Sample evaluation—targeted genomic enrichment
Sample ID
112111-3
Phenotype
High-frequency SNHL
Exons targeted
1,357
Bases targeted
351,522 base pairs
Metric
Result (reference range)
Total reads
28.2 million (14–32 million)
% Reads mapped
97.2 % (94.3–98.7 %)
% Reads overlapping target
61.0 % (58.1–63.9 %)
Targets covered at 1×
98.8 % (98.6–98.9 %)
Targets covered at 10×
98.2 % (97.5–98.2 %)
Total variants identified
335 (300–400)
Rare variants (≤1 %)
87 (60–100)
Exonic or splice site variants
19 (10–30)
Non-synonymous/indel/splice site variants
7
Variants of unknown significance
4
Known disease-causing mutations
0
Candidate mutations for deafness
2
Variants are typically annotated with the following: gene, location (exonic/intronic/splice site/intergenic/noncoding RNA), nucleotide change, amino acid change, presence in publicly available databases (1000 Genomes, dbSNP), and scores in pathogenicity prediction algorithms (Polyphen, PhyloP, SIFT). Variants are then prioritized to identify those most likely to be disease causing; variants previously reported as disease-causing mutations (DCMs) are most likely to be causative. In general, if no DCMs are identified, the prioritization strategy adheres to two generally accepted tenants of human disease genetics: (1) DCMs will be rare; and (2) DCMs will have a significant functional impact on the protein. An algorithm for variant prioritization is included in Fig. 14.1.
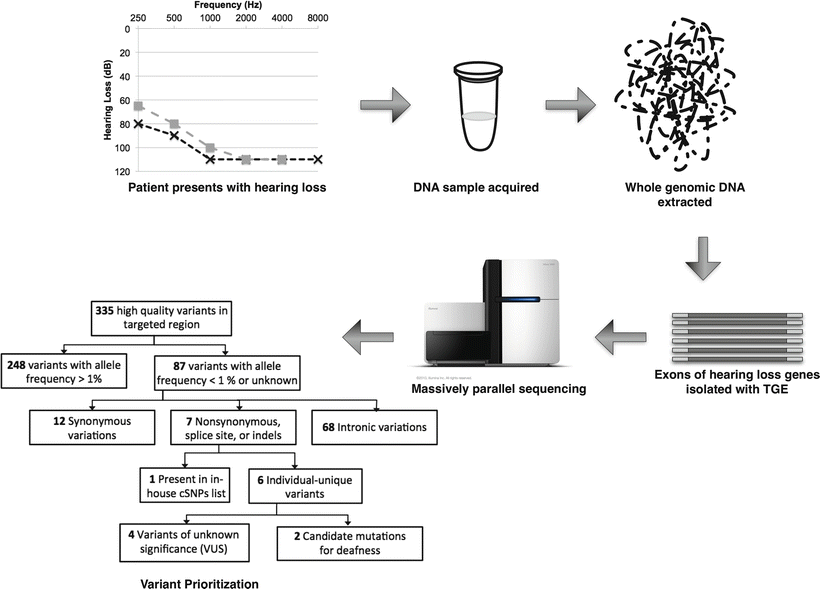
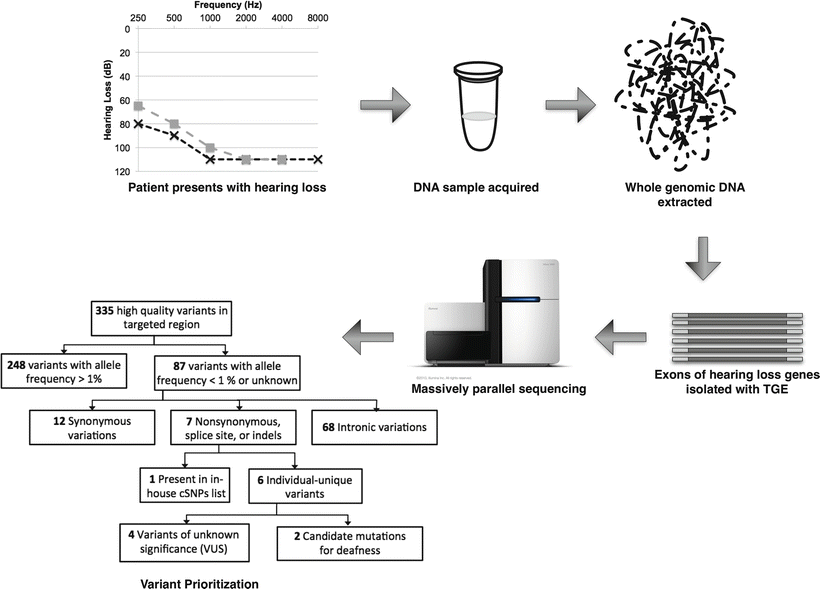
Figure 14.1
Overview of multi-gene screening panel for deafness using targeted genomic enrichment (TGE). After DNA is isolated, exons of interest (every exon of every known hearing loss gene) are isolated via TGE. Massively parallel sequencing yields millions of sequencing reads that are assessed for quality and aligned to the reference human genome. Variants are compared to the reference genome for annotation and prioritization to identify those changes that are most likely to be deafness causing, as described in detail in the text
Determining the population frequency of a variant is greatly facilitated by dbSNP and the 1000 Genomes Project, where millions of genomic variants from large sequencing projects are publicly available. Of particular importance are well-curated, locus-specific databases and laboratory-developed variant databases, which can provide significant data on variant frequency for TGE assays. The functional effect of a genetic variant is most easily interpretable when it is non-synonymous, affects a splice site, or is an insertion/deletion that causes a frameshift. Variants located outside the coding sequence are more difficult to interpret.
Frequently, several rare variants cannot be further prioritized based on database comparison and gene effect assessment. In silico pathogenicity algorithms can be used to predict the significance of these variants. For example, evolutionary conservation of the variant location (BLOSUM), comparison of the physical characteristics of the most common and the variant amino acids (Align-GVGD), and protein-specific annotation and functional consequence of changes (SIFT and PolyPhen) can provide insight into the possible pathogenicity of VUS. These in silico methods do not replace in vitro or in vivo experimentation, but provide the only mechanism to assess the large numbers of variants expeditiously. When these four specific methods are concurrent on pathogenicity, the positive predictive value is >94 % [9]. The alternative, to invest significant time and effort completing functional assays, would reduce the clinical usefulness of genetic testing in many instances.
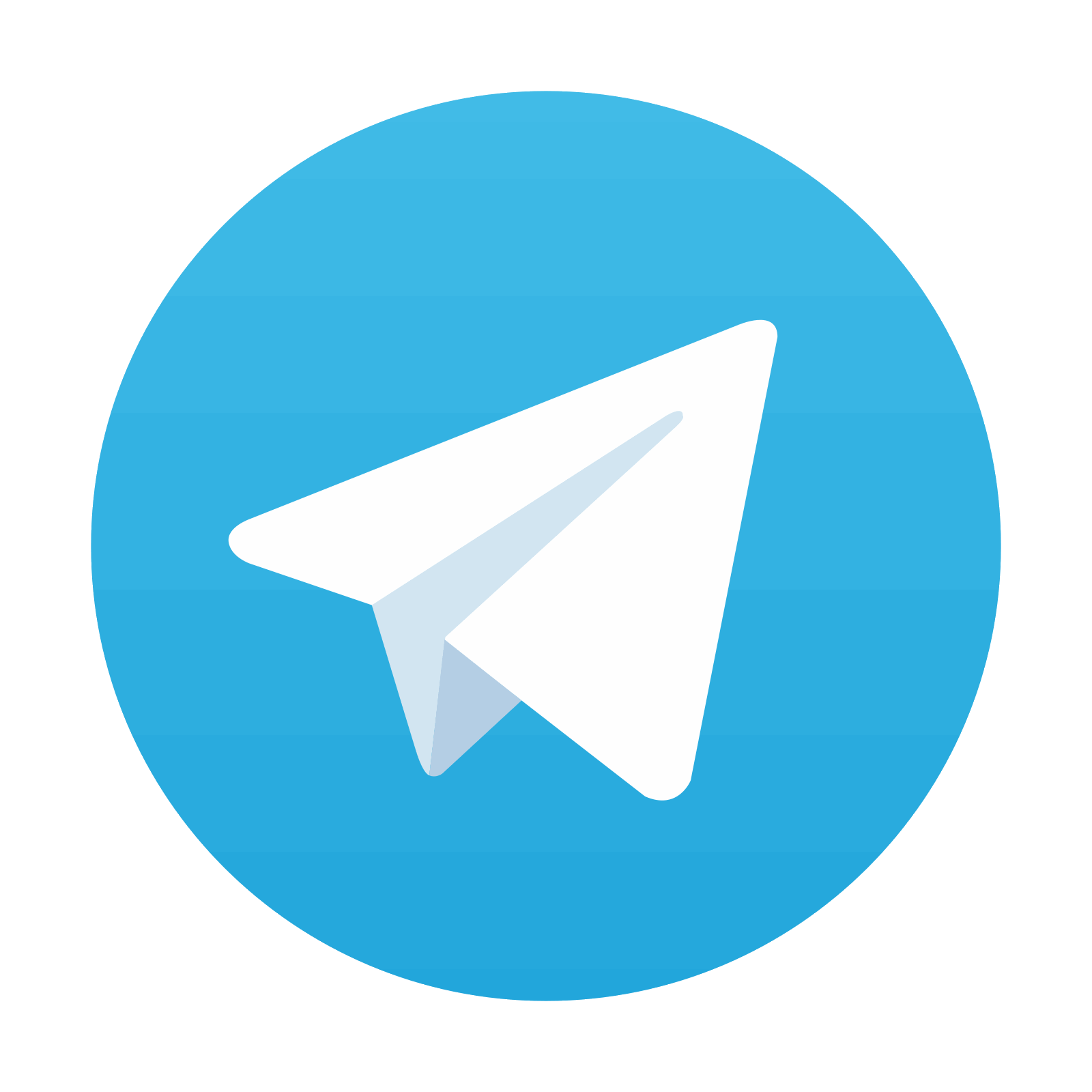
Stay updated, free articles. Join our Telegram channel

Full access? Get Clinical Tree
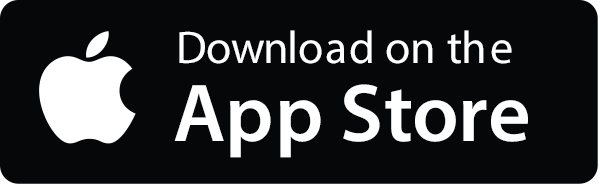
