ABL1
EGFR
GNAQ
KRAS
PTPN11
AKT1
ERBB2
GNAS
MET
RB1
ALK
ERBB4
HNF1A
MLH1
RET
APC
EZH2
HRAS
MPL
SMAD4
ATM
FBXW7
IDH1
NOTCH1
SMARCB1
BRAF
FGFR1
IDH2
NPM1
SMO
CDH1
FGFR2
JAK2
NRAS
SRC
CDKN2A
FGFR3
JAK3
PDGFRA
STK11
CSF1R
FLT3
KDR
PIK3CA
TP53
CTNNB1
GNA11
KIT
PTEN
VHL
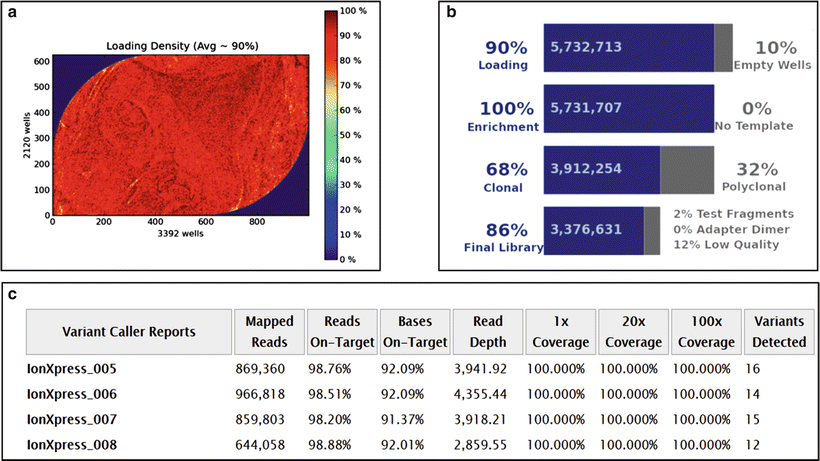
Figure 60.1
Performance specifics of a next-generation sequencing run performed on the Ion Torrent PGM platform with a 316 Chip v2 with AmpliSeq Cancer Panel v2 (Life Technologies). (a) Ion sphere particle (ISP) density indicates the distribution of the sequencing particles across the chip surface; (b) ISP summary table; (c) Variant Caller Report indicating test metrics. See Ref. 145 for details. Reprinted by permission from Elsevier Inc., Clinical Genomics: A guide to clinical next generation sequencing. Kulkarni S and Pfeifer J, Eds.
TruSeq and related assays (Illumina) [12] also target a limited set of loci in a panel of genes (Table 60.2). The assays require 150–250 ng DNA based on the sample type, and have a TAT of 3–5 days.
Table 60.2
Genes covered by the Illumina TruSeq Amplicon Cancer Panel
ABL1 | EGFR | GNAS | MLH1 | RET |
AKT1 | ERBB2 | HNF1A | MPL | SMAD4 |
ALK | ERBB4 | HRAS | NOTCH1 | SMARCB1 |
APC | FBXW7 | IDH1 | NPM1 | SMO |
ATM | FGFR1 | JAK2 | NRAS | SRC |
BRAF | FGFR2 | JAK3 | PDGFRA | STK11 |
CDH1 | FGFR3 | KDR | PIK3CA | TP53 |
CDKN2A | FLT3 | KIT | PTEN | VHL |
CSF1R | GNA11 | KRAS | PTPN11 | |
CTNNB1 | GNAQ | MET | RB1 |
Hybrid Capture-Based Methods
NGS using targeted hybridization (hybrid) capture is a sensitive and specific method to detect somatic alterations in cancer samples. With appropriate assay design, hybrid capture enables detection of all four classes of genomic alterations in cancer specimens with very high analytic sensitivity and specificity, a very low limit of detection, and very high reproducibility. Given the genomic heterogeneity that is a fundamental characteristic of cancer, particularly in solid tumors, the efficient and cost-effective targeting of multiple classes of mutations in a large number of genes in a single assay is required. Hybrid capture methodologies have the flexibility to target a wide range of genes from one gene to the exome. Currently available liquid capture kits (e.g., Ref. 13) have a target size of 1 kb to 24 Mb.
However, hybrid capture NGS tests have several disadvantages. DNA library preparation generally takes 3–5 days (compared with 1 day for amplification-based enrichment library preparation), with a large proportion of this time allocated to probe hybridization (typically 24–48 h incubation time for the hybridization step itself). Therefore, clinical hybrid capture tests have a longer TAT. Although automation can be used to decrease TAT, the equipment is expensive and thus requires a substantial initial capital investment. Hybridization-based NGS clinical tests also frequently suffer from design restrictions, including problems producing high quality sequence data from DNA regions with high GC content, repetitive sequences, and gene family members that share sequence homology (pseudogenes). The bioinformatics and interpretive component of hybrid-capture based testing has emerged as particularly problematic, since the ease with which massive amounts of sequence can be generated on the current generation of platforms can easily overwhelm a laboratory’s ability to analyze the data.
Assay Scope
Targeted Gene Panels
Gene panels for acquired mutations in cancer specimens focus on genes that are considered clinically actionable based on evidence for their diagnostic, predictive, and/or prognostic value. The gene panels may be quite narrow (e.g., only a few dozen genes) based on the specific cancer being evaluated, such as colon adenocarcinoma, lung adenocarcinoma, or gastrointestinal stromal tumor [14–16], or much broader (e.g., hundreds of genes) based on recurrently mutated genes across multiple cancer types [17, 18].
Smaller and larger gene panels each have distinct advantages and disadvantages. For clinical testing, limiting the number of targeted genes avoids an excessive number of distracting variants of unknown significance (VUSs), decreases incidental findings, and decreases TAT. Assays with a smaller target region make it cost effective to sequence at greater depth even with multiplexed samples, providing greater analytical sensitivity for detecting mutations with low variant allele frequencies (VAFs) and a lower cost. Another important advantage of small panels that target only loci with well-established clinical relevance is higher rates of reimbursement, a difference that is critical in the clinical setting where testing is funded by insurance payers rather than research grants or philanthropy. In contrast, large gene panels are more likely to include genes relevant to clinical trials or drug development, and so have much more utility in investigational settings. In the end, gene panel design is determined by examining factors such as clinical need, expected sample volume, practicality of running multiple small disease-directed panels vs a single more general cancer based panel, and sources of revenue.
Exomes and Genomes
Exome and genome sequencing often are applied to the study of cancer as a discovery tool in the investigative setting. Exome or genome sequencing is helpful for detection of CNVs and is especially well suited to detection of structural variants (SV), which often involve noncoding DNA breakpoints. However, the use of exome and whole genome in routine clinical practice has several limitations. First, because of the high depth of coverage (about 1,000×) required for sensitive and specific identification of somatic variants in cancer samples due to admixing of benign and malignant cells within the tumor, clonal heterogeneity of the tumor cells, and variation in coverage across different regions of DNA, the cost of exome or genome sequencing is often prohibitive in clinical practice. Second, the utility of sequencing genes without established clinical significance for cancer patient management is an issue. Beyond the genes evaluated by focused panels, there are relatively few loci for which sufficient evidence of clinical significance exists to support interpretation of functional or therapeutic consequences for the variants identified; thus, most variants identified are VUSs and do not meaningfully contribute to patient management. Third, intensive bioinformatics analysis is required to manage the vast amounts of data generated by such large scale sequencing.
Determination of Somatic Status with or Without Paired Tumor-Normal Tissue Analyses
Determination of Somatic Status Without Paired Normal Tissue
Many factors complicate predictions regarding the germline vs somatic status of a variant, as well as estimates of the percentage of tumor cells that harbor the variant, for sequence changes identified from a cancer sample when a normal tissue sample from the same patient is not available or tested for comparison. Most approaches to this problem rely on the VAF, which is essentially the percentage of sequence reads that have the variant. However, when evaluating the VAF for variants identified from cancer samples, it is important to remember that tumor samples in general, and solid tumor samples in particular, are inherently heterogeneous, consisting not only of the tumor cells but also of associated inflammatory cells, stromal cells, endothelial cells, and normal parenchymal cells (as discussed in more detail below). Since the relative proportion of these various cell types is highly variable between different tumor samples, and even between different areas of the same tumor, the VAF from the cancer specimen is an uncertain guide as to whether a variant is a somatic mutation present in the majority of the tumor cells, only a small subclone of the tumor cells, or even a germline variant. For example, a heterozygous mutation present in a sample that consists almost entirely of tumor cells could have the same VAF (i.e., 0.5, often indicated as 50 %) as a homozygous mutation present in half of the tumor cells, or as a germline variant (Fig. 60.2). Interpretation of VAFs is further complicated by a complex interplay of various classes of mutation affecting the same locus. For example, a SNV in a gene that is amplified in a small subclone of the tumor could produce the same VAF as a heterozygous mutation present in the majority of tumor cells. The use of VAFs as a basis for evaluating the percentage of tumor cells that contain a variant, or to infer the somatic vs germline status for an identified variant, is further complicated by analytic factors inherent to capture and/or amplification techniques that introduce technical sources of bias.
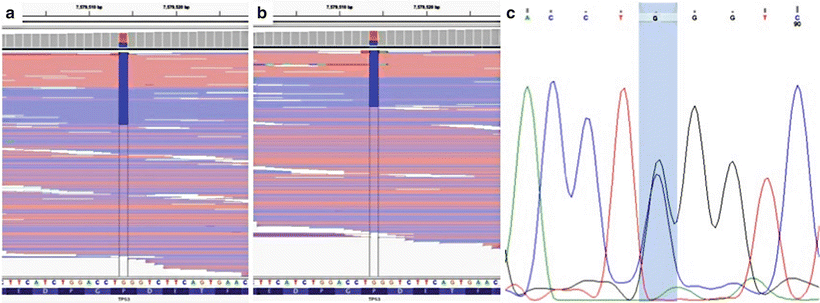
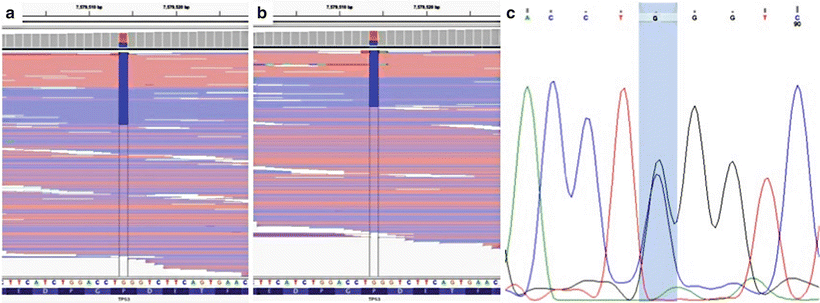
Figure 60.2
Ambiguity of variant allele frequencies (VAFs). Next-generation sequencing (NGS) was performed on two separate tumor samples from one patient; a TP53 variant was identified in both samples (chr17:7579514G.C). The patient’s oropharyngeal squamous cell carcinoma (SCC) contained the variant with a VAF of 0.30 (a). The same variant was present in the lung SCC with a VAF of 0.32 (b). Sanger sequencing was performed on nonneoplastic tissue from the same patient, which demonstrated that the variant was heterozygous in the germline (c), and copy number analysis at this position showed no copy number alterations (not shown). Thus, based on the VAF alone, this variant may have been incorrectly interpreted as a somatic mutation that was shared between the two tumors. Reprinted by permission from Elsevier Inc., Clinical Genomics: A guide to clinical next generation sequencing. Kulkarni S and Pfeifer J, Eds.
Paired Tumor-Normal Analysis
For NGS analysis of cancer specimens, paired tumor-normal testing can sometimes provide insight into the significance of a VUS obtained from tumor tissue. However, the laboratory decision to perform paired tumor-normal testing, whether ad hoc based on the NGS findings in a particular case or as the routine approach for all cases, depends on a number of factors including the cost, the size of the target region, and the anticipated clinical use of the sequence results. In general, for NGS assays focused on a limited panel of genes designed to identify mutations that are the targets of specific drug therapies, paired tumor-normal testing provides little additional information that impacts patient care. However, for very large gene panels, exome, and genome sequencing, paired tumor-normal testing is an integral part of NGS analysis, essentially in order to filter out germline variants. Unfortunately, current reimbursement paradigms do not support clinical sequencing of non-tumor samples for comparison to tumor samples.
Even before acquired mutations are considered, bioinformatics analysis of exome and genome sequence results of tumor specimens is complicated by the fact that an average person has from 140 to 420 non-silent (non-synonymous SNV, gain or loss of stop codon, frameshift or in-frame indel, or change in splice site) germline variants not present in any significant proportion of other individuals (i.e., they are variants with a minor allele frequency of < 0.5 %) [19]. Nonetheless, these variants are not expected to contribute directly to carcinogenesis and thus likely represent benign variation seen in healthy humans [20]. In addition, the number of “novel” germline variants increases dramatically for individuals from ethnicities that are less well genetically characterized, based solely on inadequate sampling of rare benign polymorphisms in those populations. Since benign polymorphisms are generally indistinguishable from tumor-associated mutations in cancer samples if matched normal tissue is not available for comparison, these polymorphisms in the patient’s background germline genetic profile cannot be separated from tumor-associated acquired mutations.
With respect to acquired somatic mutations, the number of somatic mutations in a tumor sample is highly variable between cancer types. Some cancers have < 1 mutation per megabase (Mb) of coding DNA sequence, with others having > 100 mutations per Mb [21–29]. Unlike targeted NGS analysis of relatively limited and well-described hot spot mutations and cancer genes, exome targeting captures 30–75 Mb of sequence (depending on the reagent used for capture) and identifies hundreds of nonsynonymous coding sequence variants from each cancer sample. In so-called “hypermutator phenotype” tumors characterized by unusually high rates of somatic mutation, over 1,000 somatic mutations can be identified [21, 26]. For example, lung squamous cell carcinoma, which has one of the highest described somatic mutation rates, harbors an average of 228 non-silent protein coding sequence mutations, 165 structural rearrangements, and 323 copy number changes per tumor [22]. Inclusion of the noncoding (e.g., intronic or untranslated region) sequence identified by genome sequencing hugely increases the number of variants identified; for example, a median of over 18,000 SNVs alone are found by genome sequencing of lung adenocarcinoma [27].
Comparison of tumor tissue to normal (or more accurately, non-neoplastic) tissue from the same patient is extremely valuable for determining whether an identified variant is a germline variant or a somatic mutation, and for decreasing the overall number of variants that need to be evaluated and interpreted. The simplest bioinformatics approach for paired tumor-normal NGS testing involves subtracting variants identified in the normal sample, producing a set of variants that appears to be enriched in the tumor. The subtraction approach relies on a pure normal sample, verified by tissue histology or some other method, to avoid “subtracting” variants present in even a low level of contaminating tumor cells.
One caveat to removal of germline variants from subsequent analyses is worth note, namely that some germline variants are very relevant in cancer and will be removed by this analytical method. For example, germline variants in BRCA1 or BRCA2 drive oncogenesis in families with hereditary breast cancer, and germline variants in TP53 cause cancer in families with Li–Fraumeni syndrome [30, 31]. However, ideally, standard clinical evaluation should identify those patients at risk for a hereditary cancer syndrome.
Library Complexity
The number of independent DNA template molecules (sometimes referred to as genome equivalents) sequenced in an NGS assay has a profound impact on the sensitivity and specificity of variant detection. While it is possible to perform NGS analysis using only picogram quantities of DNA [32–34], this technical feat is accomplished by simply increasing the number of amplification cycles during library preparation. However, the information content in 1,000 sequence reads derived from one genome is quite different than the information content present in 1,000 sequence reads from 1,000 different genomes. Thus, library complexity and sequence depth (see below) are independent parameters in NGS assay design.
One common way to measure library complexity is through quantitation of the number of unique, on-target reads. Sequence reads with different 5′ and 3′ termini are usually unique and thus arise from DNA from more than one genome (and more than one cell); thus, it is straightforward to estimate the complexity of a DNA library produced by a hybrid capture method since the sequence reads have different 5′ and 3′ termini reflecting the population of DNA fragments captured during the hybridization step. However, it is uncertain whether sequence reads with identical 5′ and 3′ termini have an origin from different genomes (cells) or merely represent PCR amplification bias; thus, direct measurement of the complexity in a DNA library produced by an amplification method is difficult since all the sequence reads from one amplicon will have identical 5′ and 3′ termini regardless of the population of DNA fragments from which they originated.
Accurate calculation (or even estimation) of complexity from morphologic assessment of patient specimens is difficult since all the steps of library preparation involve inefficiencies that interact in complicated ways. Cancer specimens that are highly cellular and contain a high percentage of viable tumor cells typically produce an adequately complex DNA library. Small paucicellular specimens have the potential for generating low complexity DNA libraries likely to produce biased sequence results. The complicated intratumoral heterogeneity of malignancies (see below) dictates that DNA library complexity should be maximized to achieve optimal NGS sequencing results.
Depth of Coverage
Depth of coverage is defined as the number of aligned reads that contain a given nucleotide position, and sufficient depth of coverage is critical in clinical NGS assays for identification of sequence variants with the required level of sensitivity and specificity. Many factors influence the required depth of coverage. The first variable is the sequence complexity of the target region. Target regions with homology to multiple regions of the genome, a higher number of repetitive sequence elements, pseudogenes, and increased GC content generally have decreased coverage due to technical aspects of the sequencing process [35, 36]. Second, the method used for targeted enrichment can impact coverage depth with amplification methods often providing higher depth (although the complexity of the sequence data may be uncertain, as discussed above). Third, in a multiplexed clinical test where multiple samples are sequenced simultaneously, the size of the target region (e.g., 400 kb for a typical panel of genes, vs 30–75 Mb for an exome, vs over 3 Gb for a genome) will impact the depth of coverage that can be reasonably achieved for each sample because of the defined sequencing capacity of the chip used to generate the sequence reads.
The relationship between depth of coverage and the reproducibility of variant detection from a given sample is straightforward: a higher number of high-quality sequence reads lends confidence to the base called at a particular location, whether the base call from the sequenced sample is the same as the reference base (no variant identified) or is a non-reference base (variant identified), and thus increases assay sensitivity and specificity [35–38]. However, the depth of coverage required to make accurate variant calls also is dependent upon the type of variant being evaluated, and whether the variant is germline or acquired. In general, a lower depth of coverage is acceptable for constitutional testing where germline alterations are more easily identified since they are in either a heterozygous or homozygous state, and all DNA has the same sequence, except for mosaicism. A minimum of 30× coverage with balanced reads (forward and reverse reads equally represented) is usually sufficient for germline testing [39, 40]. However, much higher read depths are necessary to confidently identify somatic variants in tumor specimens due to tissue and tumor heterogeneity (see below); an overall coverage of approximately 1,000× is optimal [7]. For NGS of mitochondrial DNA, an average coverage of > 20,000 is required to reliably detect heteroplasmic variants present at 1.5 % [41].
The need for high read depths reflects the complexity involved in somatic variant detection. As discussed below in more detail, tumor biopsy specimens represent a heterogeneous mixture of tissue encompassing malignant cells, as well as supporting stromal cells, inflammatory cells, and uninvolved tissue; malignant cells harboring somatic variation can become diluted out in this admixture. Of additional consideration, intratumoral heterogeneity creates tumor subclones so that only a small proportion of the total tumor cell population may have a given mutation. Thus, the read depth of the assay should be sufficiently high to compensate for this variation.
Preanalytic Issues
Specimen Requirements
The amount of specimen DNA required for clinical NGS testing can be from a variety of patient sample sources including peripheral blood, bone marrow aspirates, buccal swabs, surgical resections, needle biopsies, and fine needle aspirations (FNAs). For solid tumors the most frequently available specimen type is FFPE tumor tissue. Fortunately, FFPE specimens as well as fresh tumor samples are both amenable to NGS analysis by current NGS technologies. However, DNA from FFPE will be a suboptimal substrate for use with emerging approaches that make it possible to determine the sequence of over a thousand bases per individual DNA template molecule, which will improve the analysis of currently difficult regions such as pseudogenes or repetitive sequences.
While it is well established that formaldehyde reacts with DNA and proteins to form covalent crosslinks, engenders oxidation and deamination reactions, and leads to the formation of cyclic based derivatives [42–46], with higher depths of coverage, the rate of sequence artifacts from FFPE samples is quite small compared with paired fresh samples from the same tumor, and is in fact several orders of magnitude below the cutoff for reporting variants in routine clinical practice [47]. Similarly, several studies have demonstrated that, for both amplification and hybrid capture methods, alcohol fixation does not induce sequence artifacts at a clinically significant rate [48, 49]. The lack of a significant rate of NGS sequence artifacts has been shown for both ethanol-fixed specimens (of the type used in Papanicolaou stains) as well as methanol-fixed specimens (of the type used in Romanowsky stains such as Diff-Quik), which allows the increasingly common use of cytology specimens for clinical NGS tests. Since exposure to acid efficiently hydrolyzes phosphate diester links (and also damages nucleotides leading to abasic sites) in both DNA and RNA, acid decalcification renders tissue samples unacceptable for NGS analysis [50]. When decalcification is required, calcium chelating agents such as EDTA should be used since they have no significant impact on nucleic acids.
Histopathologic Review
Prior to DNA extraction from the tumor specimen, the specimen slides corresponding to the tumor used for NGS testing should be reviewed by an anatomic pathologist to ensure the presence of viable (non-necrotic) malignant tissue, and to assess the quality and quantity of the material submitted for testing. The pathologic assessment is an important quality control step since it permits evaluation of possible analytic confounders, including the percentage of nonneoplastic tissue, necrosis, cautery artifact, and so on, and thus helps ensure that the specimen is adequate for the validated assay. More specifically, if a cutoff of 10 % VAF is used for clinical reporting of a variant, then areas with more than 20 % tumor cellularity should be used to ensure that heterozygous variants present in all the tumor cells will likely be detected. Obtaining an estimation of the percentage of tumor cells present in the tissue section relative to total number of cells is useful during interpretation of the sequencing data in regard to VAF. Unfortunately, although a pathologist’s review of cancer samples is required to select the regions of tumor with high cellularity and viability, the estimates of percent tumor cells present are unreliable [51, 52].
It is important to recognize that the percentage of nonneoplastic tissue (also known as tissue heterogeneity) is different from intratumoral heterogeneity. Tissue heterogeneity refers to the fact that no tumor specimen is composed of 100 % neoplastic cells. Instead, cancer samples contain a varying proportion of nonneoplastic cells including stromal cells (benign parenchymal cells and fibroblasts), inflammatory cells (primarily neutrophils, lymphocytes, and macrophages), and endothelial cells (of blood vessels and lymphatics). Intratumoral heterogeneity is a term used to refer to the fact that malignant neoplasms usually demonstrate clonal heterogeneity [53, 54]. Consequently, even with a relatively pure tumor sample identified by histopathologic review, the number, type, and frequency of sequence variants detected in that sample may or may not be an accurate reflection of the range and frequency of the variants elsewhere in the tumor.
DNA Extraction
Total DNA yield from a cancer specimen is commonly measured by either spectrophotometry or fluorometry. Metrics including A 260/A 280 and A 260/A 230 are commonly used to estimate nucleic acid purity, and agarose gel electrophoresis can be performed to ensure the presence of high molecular weight genomic DNA. However, the presence of high molecular weight DNA is not necessarily mandatory for NGS, as demonstrated by the fact that both amplification-based and hybrid capture-based methods work well with FFPE samples that contain damaged nucleic acids due to formalin-fixation during routine processing [47]. While sample processing is generally standardized within a laboratory, many variables can significantly impact nucleic acid quality, such as fixation time, storage conditions, and acid decalcification, and in turn can affect subsequent library preparation and sequencing. The use of acid decalcification is especially problematic since acid exposure rapidly destroys nucleic acids [50]; decalcification with a chelating agent (EDTA) is preferred [55].
Analytic Issues
NGS tests require three components, specifically: the sequencing instrument; the laboratory procedures including extraction of nucleic acids and DNA library preparation; and the bioinformatics processes for base calling, reference genome alignment, variant identification, variant annotation, and variant interpretation. The general features of all three of these components as they apply to NGS analysis of cancer specimens are similar to those for constitutional testing, and are covered in Chap. 61. However, some additional issues must be considered in the analysis of tumor samples, specifically in the bioinformatics analysis.
As with all NGS testing, after the sequencing reads are generated from the DNA extracted from a tumor specimen, bioinformatics tools are used to align the reads against a reference genome and identify differences between the tumor DNA sequence and the reference sequence. Given the intrinsic genomic instability of malignancies, and often complicated intratumoral heterogeneity due to the presence of various tumor subclones, maximum clinical utility of NGS testing of cancer specimens can only be achieved using a bioinformatics pipeline designed to detect all four classes of genomic variants (SNVs, indels, CNVs, and SVS) at allele frequencies that are physiologically relevant. The four main classes of variants each require different computational approaches for sensitive and specific identification (assuming the assay is designed to permit their detection) [56], and since various bioinformatics pipelines are known to yield different variant calls for the different classes of variants, and even for specific variants, optimization of the bioinformatics pipeline used for a clinical NGS test is imperative [57].
Single Nucleotide Variations
SNVs occur when a single nucleotide (e.g., A, T, C, or G) is altered in the DNA sequence; note that single base pair insertions and deletions are technically not SNVs but rather indels. SNVs are by far the most common class of sequence variant, and the high density of polymorphic SNVs segregating in the human population (about 1 SNV is present per 800 bases between a single diploid individual and the reference genome) makes them ideal markers for genetic mapping [58]. Inherited SNVs are generally classified as single nucleotide polymorphisms (SNPs) if they are present at a moderately high frequency in the population (greater than 1 %), although many inherited SNVs exist at lower population allele frequencies yet are nonetheless benign polymorphisms with no known disease association. While SNPs are polymorphisms that have no direct (or clearly established indirect) association with a specific disease, SNVs that are correlated with disease often are referred to as single base pair mutations or point mutations. The biologic impact of SNVs in protein-coding regions depends on whether the change is synonymous (silent) or nonsynonymous (of which the two types are missense mutations and nonsense mutations). In noncoding regions, as well as some coding regions, SNVs may affect RNA processing or gene regulation [59–62]. Nonetheless, selection pressure reduces the overall frequency of single base pair substitutions in coding DNA and in associated regulatory sequences, with the result that the overall SNV rate in protein-coding DNA is much less than that of noncoding DNA. In addition, clinical testing does not usually consider the noncoding regions of the genome, although these regions are being studied and clearly can cause disease.
The platforms and bioinformatics pipelines of NGS are well suited to the detection of SNVs, although the error rates of different platforms must be considered during platform selection and assay design (Fig. 60.3). In fact, the earliest clinical applications of NGS were designed to detect SNVs in inherited and acquired diseases, and for this reason the bioinformatics pipelines required for sensitive and specific detection of single base substitutions are among the most advanced in clinical NGS. The expanding catalog of clinically relevant point mutations has been an especially important driver of development of NGS assays [21, 63, 64]. Indeed, to date, NGS tests have been successfully implemented in several clinical laboratories for detecting SNVs [7–9, 14, 16, 17].
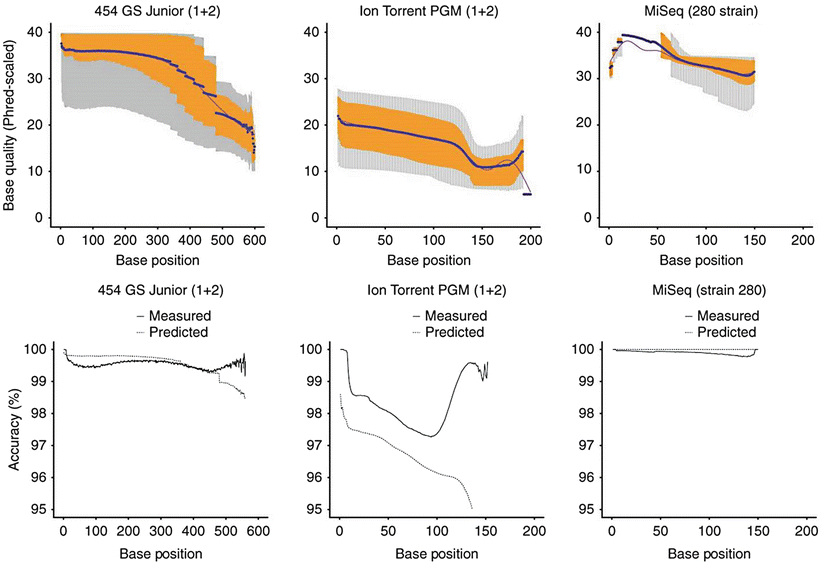
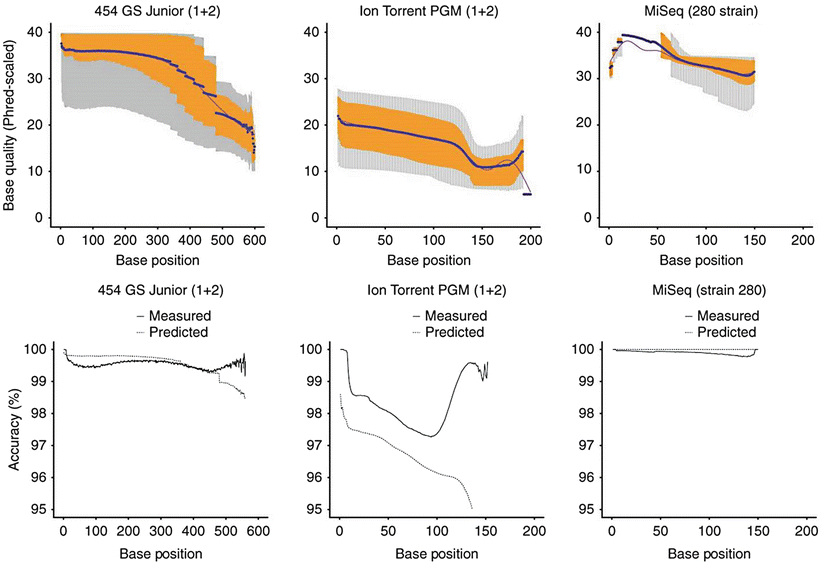
Figure 60.3
Comparison of three major platforms currently used in clinical NGS assays. Since the platforms vary with respect to their chemistry, it is not surprising that they each have different intrinsic error rates which affect the performance for single nucleotide variant (SNV) detection. In one comprehensive study evaluating sequencing platform differences [141], the MiSeq instrument (Illumina) had the lowest substitution error rate (about 0.1 substitutions per 100 bases). The Ion Torrent PGM (Life Technologies) had a substitution error rate over ten-fold greater, which steadily decreased across the read length; however, increased accuracy could be achieved by “clipping” read ends determined to be of low quality [142]. The substitution error rate of the 454GS Junior (454 Life Sciences, A Roche Company, Branford, CT) was intermediate between the MiSeq and Ion Torrent PGM. In terms of indel detection, admittedly only one of many factors that must be considered in selecting an NGS platform, the reversible dye terminator approach of the MiSeq has a lower indel error rate (<0.001 indels per 100 bases sequenced) compared with pH detection of the Ion Torrent PGM (1.5 indels per 100 bases), for reasons discussed in the text [143]. From Loman NJ, Misra RV, Dallman TJ et al. (2012) Performance comparison of benchtop high-throughput sequencing platforms. Nat Biotechnol 30:434-9. Reprinted by permission from Nature Publishing Group
From a bioinformatics perspective, many popular NGS analysis programs for SNV detection are designed for constitutional genome analysis where variants occur in 0 % (wild type), 50 % (heterozygous), or 100 % (homozygous) of the reads. These prior probabilities are often built into the algorithms, and consequently, SNVs with VAFs falling too far outside the expected range for homozygous and heterozygous variants are often ignored as false positives. Thus, sensitive and specific bioinformatics approaches for somatically acquired SNVs require either significant revision of the software packages designed for constitutional testing or new algorithms altogether. Some bioinformatics tools are optimized for very sensitive detection of SNVs in NGS data, but these tools require high coverage depth for acceptable performance and rely on spiked in control samples used to calibrate run-dependent error models [37], features that must be accounted for in assay design. The published comparative performance of the various bioinformatics tools for SNV detection provides some guidance to clinical laboratories for design and implementation of NGS assays for somatic mutations [37, 65].
A number of on-line tools can be used to predict the impact of a SNV and evaluate whether a SNV has a documented disease association. However, given the lack of standardized annotation formats, and variability in the level of review that was performed to establish the associations between a specific genotype and a specific phenotype, putative associations must be carefully reviewed in the context of the published medical literature.
Insertions and Deletions
By definition, indels are an insertion and/or deletion of one or more nucleotides into genomic DNA and include events less than 1 kb in length, although most indels are only several bp to several dozen base pair (bp) in length. Of note, many indels are not necessarily the direct result of DNA damage, per se, but instead originate from DNA polymerase errors or incorrect DNA repair following a genomic insult. As a result, indels may be complex (e.g., include both inserted and deleted bases) and often involve areas with repetitive sequences, factors that can make identification difficult. Indels can have widely variable consequences, including altered gene transcription, altered RNA splicing, in frame mutations (synonymous, missense, and nonsense mutations), frameshift mutations (that can be silent, or result in production of a protein with altered structure and function), and change the length of repetitive regions which can lead to the clinical phenomenon known as anticipation (more severe and earlier onset disease with each generation).
Indel detection is very important in clinical NGS of cancer specimens since indels are implicated as the driving mechanism for many oncologic diseases. Additionally, indels are a common mechanism of kinase activation in cancer, a feature exploited clinically by targeted therapy with kinase inhibitors. While the sequencing techniques and bioinformatics tools used for NGS analysis both influence the sensitivity and specificity of indel detection, several specific factors inherent to indels as a mutation class also complicate their detection, including size, DNA sequence context (including the fact that indels commonly occur in repetitive DNA sequences), and variant annotation. The bioinformatics tools optimized for detection of SNVs or other classes of mutation are not optimized to detect indels, and therefore specific tools for indel detection are required.
Since alignment of indel-containing sequence reads is technically challenging, significant improvement in bioinformatics detection of indels can be achieved simply by using algorithms specifically designed for the task. One such specialized approach is called local realignment, which essentially tweaks the local alignment of bases within each mapped read so as to minimize the number of base mismatches [66].
Probabilistic modeling based on mapped sequence reads can be used to identify indels that are up to approximately 15 % the length of an individual sequence read, but not longer. This level of sensitivity is suitable for the detection of many clinically relevant indels like EGFR exon 19 activating indels. However, probabilistic methods do not provide an acceptable sensitivity for detection of other insertions such as FLT3 internal tandem duplications (ITDs) that range from 15 bp to over 300 bp in length [67], the presence of which is used clinically to predict prognosis and guide treatment in patients with cytogenetically normal AML [68].
Split-read analysis approaches to indel detection utilize algorithms that focus on split and soft-clipped reads (sometimes called one-end anchored reads) to identify possible breakpoints in NGS sequence data. The reads can either be analyzed using a pattern-growth algorithm whereby unmapped reads are broken into smaller pieces and realigned separately to identify possible indels, and/or by de novo assembly whereby unmapped reads are reassembled into a contiguous sequence (contig) based on their overlaps with each other [67, 69]. Importantly, evaluation of split and soft-clipped reads allows for the identification of the full size spectrum of indels, and the approach is not subject to the same read length constraints as probabilistic methods [67].
Indel Annotation
A major issue with clinical indel detection is annotation (i.e., how the size, composition, and genomic location of the indel is written). As discussed above, indels often occur in repetitive sequences, and thus multiple possible annotations can describe the same resulting sequence (see Fig. 60.4). Left-alignment prior to indel calling can decrease this problem by combining the multiple possible annotations into a single left-aligned annotation [66]. Importantly, left-alignment does not necessarily facilitate comparison of the identified indel to existing databases and literature in which indels are often not annotated in left-aligned format. In fact, left-alignment may preclude correlation of a potentially relevant indel with existing databases during interpretation.
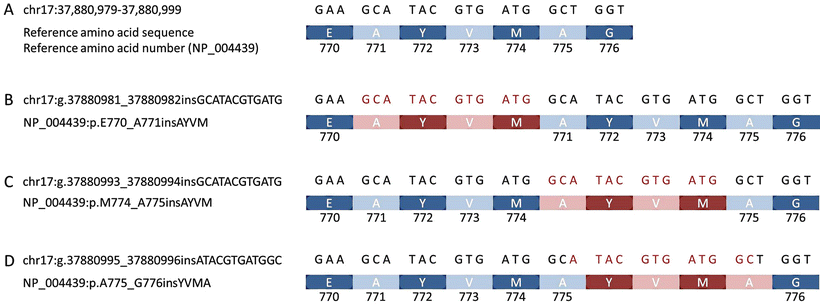
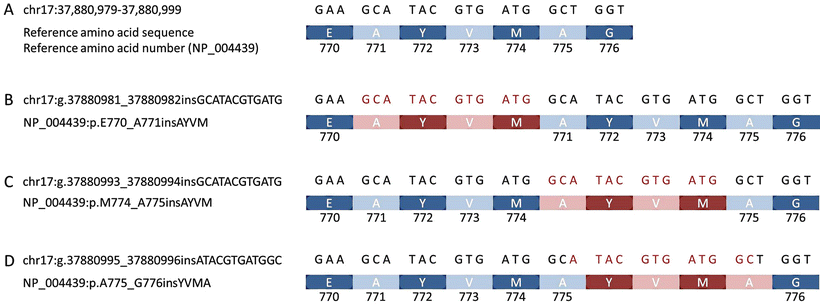
Figure 60.4
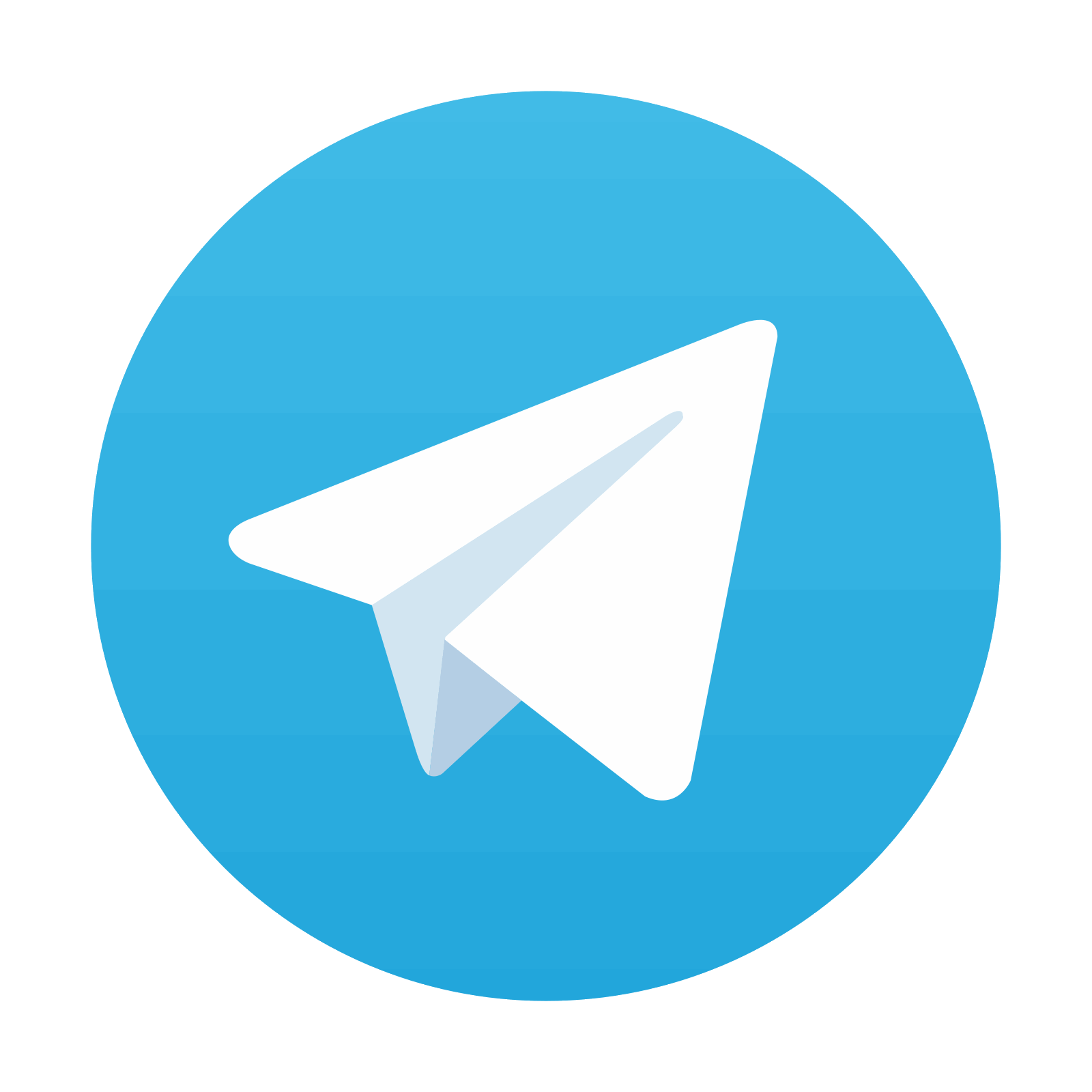
Redundant annotations for indels. The most common activating ERBB2 indel in lung cancer results from duplication of 12 nucleotides in exon 20, resulting in insertion of four amino acids in the protein sequence, but can be annotated in multiple ways. The reference genomic nucleotide sequence and resulting amino acid sequence at the beginning of exon 20, with amino acid numbering according to the NP_00439 isoform of the ERBB2/HER2 protein, are shown (a). Three possible annotations for the activating ERBB2 indel are shown (b, c, and d; inserted nucleotides and amino acids shown in red, reference shown in blue). In (b and c), the inserted nucleotide sequence is the same (GCATACGTGATG), but the site of insertion is different. In (b), the insertion is made at the beginning of the reference AYVM sequence, with the genomic annotation chr17:g.37880981_377880982ins12 and protein annotation NP_004439:p.E770_A771insAYVM. In (c), the insertion is made after the reference AYVM sequence, shifting the indel annotations to chr17:g.37880993_37880994ins12 and NP_004439:p.M774_A775insAYVM. However, the resulting nucleotide and amino acid sequences are exactly the same in (b) and (c). However, the same insertion also can be annotated with what seems to be a completely different inserted sequence (d). Note that the amino acid before and after the reference YVM is an alanine. However, the reference A771 is encoded by the genomic sequence GCA, whereas the reference A775 is encoded by the genomic sequence GCT. Insertion of the sequence ATACGTGATGGC, splitting the GC and T that normally encode A775 (i.e., genomic annotation chr17:g.37880995_37880996insATACGTGATGGC) keeps an A at amino acid number 775 (though now encoded by GCA instead of the reference GCT). The inserted nucleotides result in insertion of amino acids YVMA between reference amino acid positions 775 and 776 (i.e., protein annotation NP_00439:p.A775_G776insYVMA). The inserted A (just prior to G776) is derived from the last two inserted nucleotides (GC) and the T that was split off from what was originally A775. Although at first glance the various annotations listed in parts (b), (c), and (d) seem different, all result in the same final nucleotide and amino acid sequence. Of note, none of these possible annotations is technically correct based on the Human Genome Variation Society recommendations for mutation nomenclature [144], in which the variant is most appropriately annotated as a duplication (dup): chr17:g.378800982_37880993dup and NP_004439:p.A771_M774dup. Reprinted by permission from Elsevier Inc., Clinical Genomics: A guide to clinical next generation sequencing. Kulkarni S and Pfeifer J, Eds.
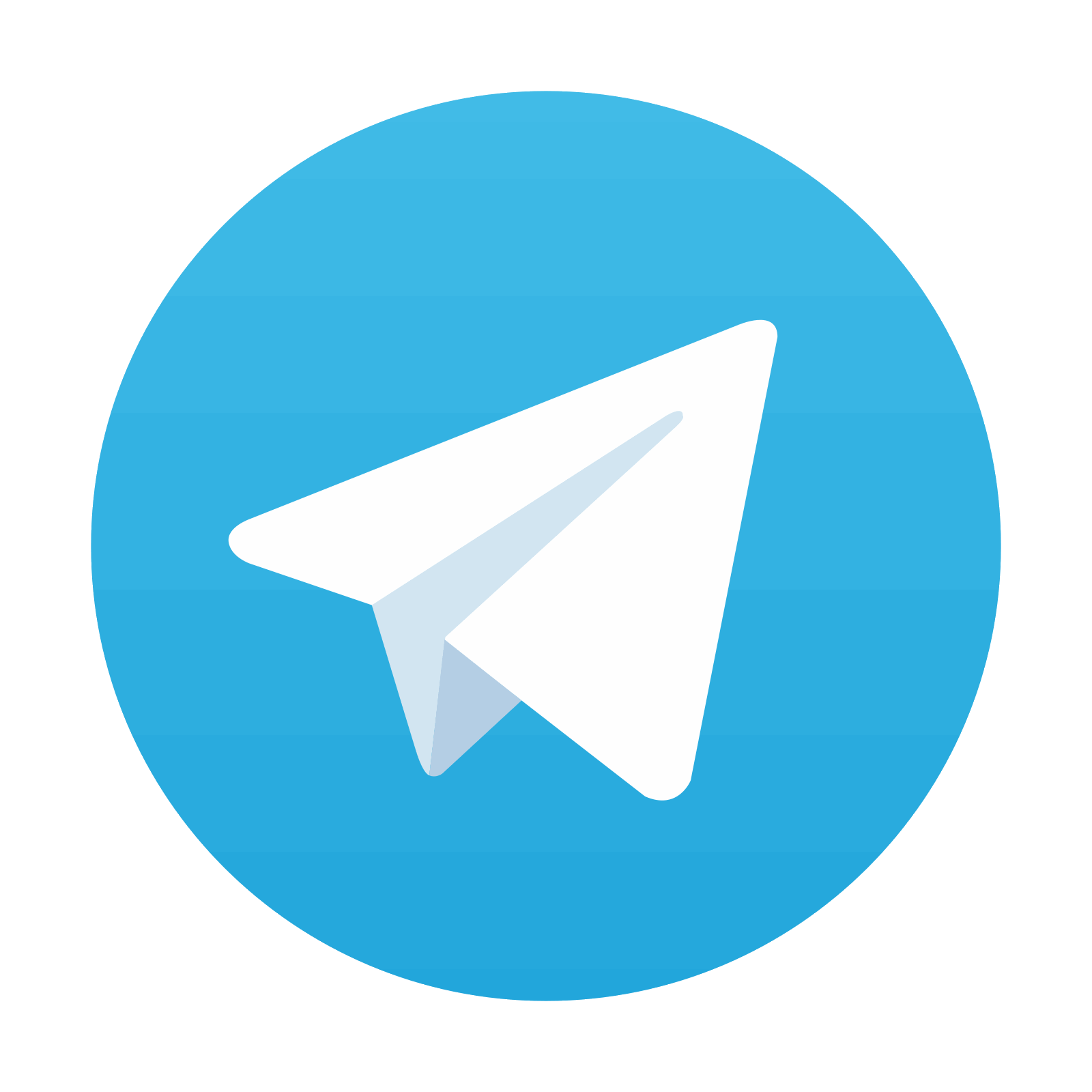
Stay updated, free articles. Join our Telegram channel

Full access? Get Clinical Tree
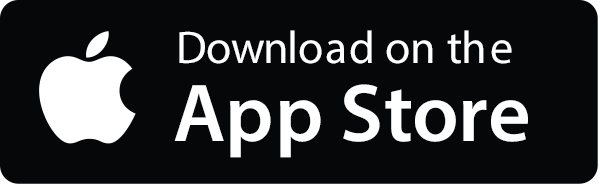

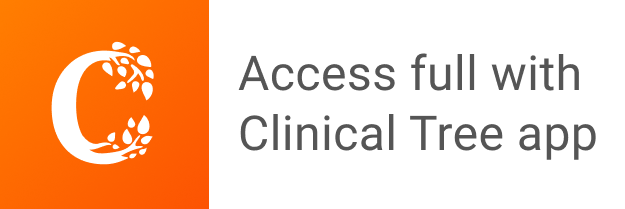