Chapter 12 Enough can never be said to emphasize the importance of effective quality control (QC) and quality assurance practices throughout an entire modeling effort. It takes just one experience of identifying a fundamental problem in the analysis dataset or with a key modeling assumption moments before a regulatory deadline or before a presentation of results for senior management to convince any modeler of this truth. Sometimes in our haste to show progress or achieve some measure of results to share, we skip over or postpone steps in the process designed to prevent such stressful situations. At these times, the value of this message could not be more clear (Grasela et al. 2009; Bonate et al. 2012). There is a myriad of process steps involved in a model development effort, from rationalization of the need for the modeling effort, to conceptualization of the data analysis plan, to creation of the analysis dataset, to performing the modeling analysis steps, to completion of the regulatory deliverable. Each step needs to be carefully checked in parallel with performing the step, if at all possible. Delays in completion of the QC tasks only serves to increase the amount of rework that may be required and the possible ramifications to the program due to critical error. In Chapter 5, the development of the analysis plan is described as perhaps the most important step of the entire data analysis effort. If the analytical plan is carefully considered and conceived, the first step in completing the modeling and simulation project according to a generally recognized quality standard will have been achieved. Even if the best model is a poor description of the data, the results are more likely to be accepted when performed according to an established plan than they would be in the absence of a well-thought-out plan. Beyond the review of the analysis plan document itself by a senior modeler or pharmacometrician, who can carefully evaluate the appropriateness of the proposed methodology and technical details given the data, review of the analytical plan by development team members from other areas of expertise is also essential. This vetting of the plan with team members and development team leaders not specifically expert in modeling will provide input regarding the relevance of the questions the model is intended to inform, a critical first step in assuring that the model to be developed will be of use to the team in addressing important knowledge gaps. Furthermore, input and buy-in from such team members prior to the performance of the analysis may serve to facilitate their later acceptance of the modeling results and help to forge collaborative efforts to extend the use of the model via simulation strategies. Given a comprehensive data analysis plan, QC of the analysis dataset begins with the dataset requirements. The requirements or specifications for the analysis dataset may be in the form of a detailed specifications document detailing exactly which data are to be used and how such data are to be processed to create the required structure and content of the dataset for analysis (Chowdhury 2010). Alternatively, the requirements may be a simple bullet point list of points to consider in the creation of most NONMEM-ready datasets, updated with specific details regarding the particular data to be included or excluded for the analysis at hand. Although dataset QC can be performed with either type of requirements document, it should be self-evident that the more detailed and specific the specifications are defined, the more precisely the data can be checked against those requirements during dataset QC. However, in either case, the mere existence of the specification provides the standard or guidance against which an independent reviewer could provide a QC review of the dataset. Without such a form, dataset QC would be an arbitrary task, relying on the instinct and experience of the QC checker. In small organizations or other situations where an independent reviewer is not available, the documentation of steps such as the dataset requirements is still necessary and perhaps even more important, especially in light of the inherent difficulties in checking one’s own work and thought processes. In larger organizations or when possible, prior to implementation by the programmer, the requirements form itself may be subjected to a QC review by an independent pharmacometrician. With regard to QC of the dataset in those situations where an analyst prepares the requirements and a programmer executes on the specifications to create the dataset, at least two levels of dataset QC should be performed. First, the programmer should verify that the code performed as expected and that the resulting output is consistent with expectations based on his/her understanding of the requirements. Second, the analyst (requestor) should verify that the dataset contents are consistent with his/her expectations based on what was intended in the request. Finally, an independent QC reviewer could be invoked to verify the analysis dataset content by checking each element of the raw data against the specifications document and the analysis dataset (this is sometimes called 100% QC or 100% inspection, although it is not typically intended to be performed on 100% of the data). Although there are many ways to approach this type of QC, one way would be to randomly select a small subset of the data on which to perform such verification. A reasonable number of patients to check might be three from each study (with study selected as the independent variable based on the assumption that different coding might be required for each study based on the individual design features of each study). Alternatively, some other significant feature of the coding may be used to (randomly) select a subset of patients for 100% QC to ensure that important variations are verified. If discrepancies are identified, additional programming or clarification of the requirements would be needed. Once such changes are complete, the new version of the analysis dataset would be subjected to this process again (Grasela et al. 2010a). Additional suggestions regarding dataset QC are provided in Chapter 5. One of the objectives of the exploratory data analysis (EDA) step is to verify the content of the analysis dataset prior to modeling. If carefully planned, data displays can serve to inform the modeler about the data content as well as verify the structure and content of the dataset. For example, histograms of continuous variables can be used to facilitate the identification of possible outliers, as well as to identify potential issues with inconsistent units among studies or labs. Simple frequency tables can be used very effectively to identify unusual values for data items and to confirm the coding of NONMEM-required data items that were initialized and coded within the dataset creation programs. Scatterplots of concentration versus time since first or last dose can be used to easily identify possible errors in dates or times. Several aspects of data collection for pharmacometric analyses warrant consideration from a QC perspective. Clearly, if consideration is given to the data that will be most important to pharmacometric modeling efforts prior to finalization of the data collection device or at the very least, prior to the completion of the study, the modeling team will be better positioned to meet the development program timelines with higher quality data. Once the trial or trials have begun, it may be a very cumbersome and difficult process to make changes to the data to be collected, the data management plan (if one exists), or the process of data flow. In order to maximize the potential impact of modeling and simulation efforts, its full integration into development program timelines, including cross-department collaborative efforts regarding data flow and processing must be addressed (Collins et al. 2010; Collins and Silva 2011).
Quality Control
12.1 Introduction
12.2 QC of the Data Analysis Plan
12.3 Analysis Dataset Creation
12.3.1 Exploratory Data Analysis and Its Role in Dataset QC
12.3.2 QC in Data Collection
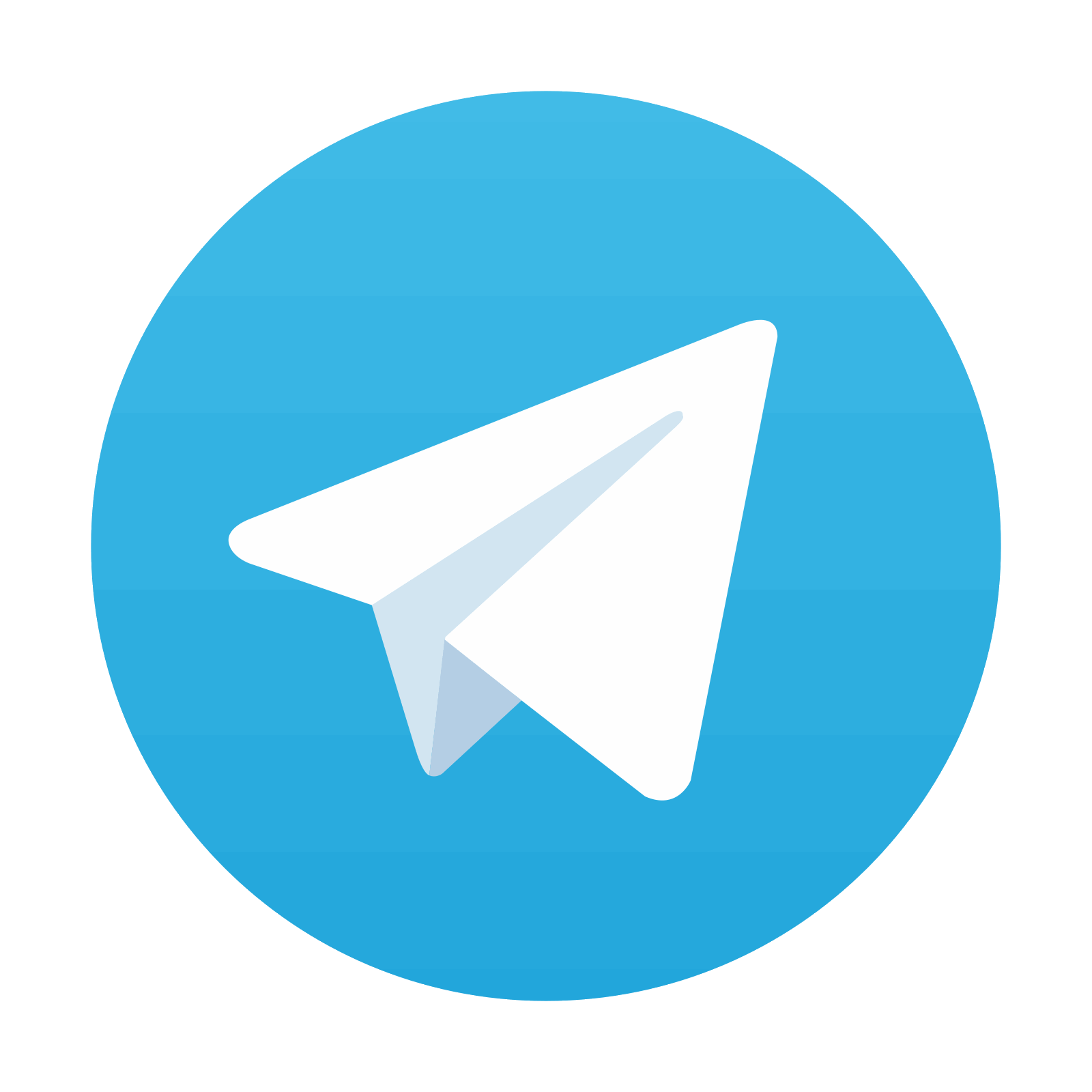
Stay updated, free articles. Join our Telegram channel

Full access? Get Clinical Tree
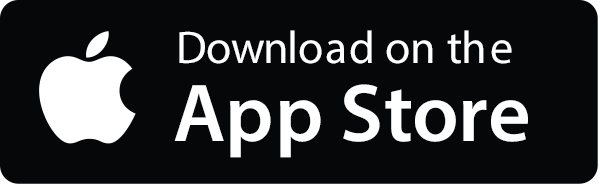
