Figure 1.1Diagrammatic ternary phase diagram for studying the relationship between oil, water and surfactant for self-emulsifying lipid-based drug delivery systems. (Adapted from JB Cannon, MA Long. Emulsions, microemulsions, and lipid-based drug delivery systems for drug solubilization and delivery—Part II: Oral applications. In: R. Liu, ed., Water-Insoluble Drug Formulation, 2nd edition, CRC Press, Boca Raton, FL, 2008, Chapter 11, pp. 227–254.)
The broader adoption of SEDDS may in part be impeded by the limited solubility of some hydrophobic drugs in preconcentrates. Based on research with simvastatin SNEDDS, it has been suggested that supersaturation of the preconcentrate may be a feasible approach to increase drug loads and bioavailability.34 Supersaturated preconcentrates containing up to 200% simvastatin (compared to 75% conventional loading) were formed by subjecting the preconcentrates to heating and cooling cycles. In beagle dogs, the 200% super SNEDDS exhibited markedly greater bioavailability than the same dose in multiple units of the conventional SNEDDS.
The broader adoption of SEDDS in capsules may also be impeded by lack of stability on aging and possible adverse interactions of some preconcentrates with soft or hard shells. To remedy this problem, solid SEDDSs have been proposed, formed by such processes as spray drying or freeze drying the preconcentrate, and physical adsorption onto silica and silicate-based carriers.35,36 The resultant powdery formulations, mixed as needed with suitable excipients, may be encapsulated or tableted.
Systematic Approaches for Understanding Hard Shell Capsule Formulation and Filling
Modeling of Plug Formation
That most powder formulations are filled on machines that form powder plugs by compression and eject the plugs into capsules has stimulated interest in exploring filling machine operating characteristics and low-force compression physics. Advanced through the instrumentation of capsule filling machines and laboratory simulation of plug formation, discussed in Chapter 7, this research has revealed that several powder compression models (e.g., Heckel, Shaxby–Evans, and Kawakita) can be used to parameterize the low-force compression profiles related to capsule filling, as discussed by Podczeck in Chapter 9. Based in part on understandings developed through such research, a theoretical model was developed by Khawam, discussed in Chapter 10, that may prove to be useful as an in silico analysis tool for developing formulations for dosator machines that can be used in conjunction with other development tools, for example, experimental design/response surface analysis.
Modeling in Support of Formulation Development
More than ever before, pharmaceutical scientists are adopting systematic approaches to the design, formulation, and optimization of dosage forms. Driven in part by the QbD initiative of the US Food and Drug Administration (FDA) and supported by enhanced understandings of biopharmaceutic principles, for example, the Biopharmaceutical Drug Classification System, and software-driven optimization and decision-making tools, pharmaceutical scientists have the ability to make logical and deliberate formulation design decisions. Using such tools to model the interplay of formulation and process variables not only can enhance formulators’ understanding of how formulation and process factors affect product performance but also can lead to an optimized drug delivery system with respect to its design criteria.
The current regulatory climate clearly favors such systematic development approaches. Systematic approaches can establish a research database that can help justify SUPAC (scale-up and post-approval changes) changes to regulatory agencies. Experimental design and modeling also supports FDA’s QbD and risk-based cGMP initiatives by revealing the relationships between product quality attributes and critical material and process attributes.37,38 An understanding of these relationships can lead to the identification of the Design Space (DS), a particularly useful QbD tool. The DS is formally defined as “The multidimensional combination and interaction of input variables (e.g., material attributes) and process parameters that have been demonstrated to provide assurance of quality.”39 Thus, these operational ranges determine the DS space within which movement is not considered a change for regulatory purposes.
Among the tools being employed by today’s formulation scientists are multivariate analysis and response surface methodology40,41 and artificial intelligence.42–44 A discussion of the mathematical principles and statistical bases for these tools is beyond the scope of this discussion. For these, the interested reader is referred to Fridrun Podczeck’s chapter entitled “Aims and Objectives of Experimental Design and Optimization in Formulation and Process Development”45 and the Peng and Augsburger chapter on “Knowledge-Based Systems and Other AI Applications for Tableting,”46 both of which appear in Pharmaceutical Dosage Forms: Tablets. However, several interesting examples of applications to hard shell formulation development have been selected for discussion here.
Multivariate Analysis and Response Surface Methodology
One example of response surface analysis applied to capsules examines the critical formulation variables for a piroxicam capsule formulation.41 This example is particularly interesting because it links in vitro outcomes with in vivo outcomes in human subjects. Piroxicam is a BCS Class II drug (low solubility and high permeability) drug. Thirty-two batches of 20-mg piroxicam capsules were manufactured according to a resolution V central composite (face-centered) experimental design. Five formulation (independent) variables were studied at each of three levels (see Table 1.1 and additional comments in Chapter 15). Colloidal silica (glidant) and sodium starch glycolate (disintegrant) were fixed at 0.1% and 5%, respectively, in all batches. Because it is a BCS II drug, the effect of piroxicam particle size on drug dissolution was of particular importance. This aspect was evaluated by comparing the piroxicam powder as received (termed unmilled in the study) with the same lot of piroxicam that was remilled (termed milled in the study). The remilling of piroxicam substantially reduced its particle size, as reflected in a nearly threefold increase in its specific surface area from 1.79 to 4.91 m2/g. The capsules were filled with a consistent plug compression force using an instrumented Zanasi LZ-64 automatic capsule filling machine. USP monograph dissolution profiles (% piroxicam dissolved vs. time) were obtained for the batches. Statistical analysis revealed a number of interesting insights. For example, the percent lactose, and an interaction between piroxicam particle size and sodium lauryl sulfate level were the most important variables affecting the percentage of piroxicam dissolved at 10 min. However, the most important variable affecting the percent dissolved in 45 min was the wetting agent, sodium lauryl sulfate, followed closely by piroxicam particle size. When the piroxicam is milled, the sodium lauryl sulfate level has comparatively little effect on dissolution (see Figure 1.2), presumably because the milled drug is more rapidly dissolving because of its larger specific surface area. The apparent reduced significance of the level of lactose at 45 min may be attributed to its complete dissolution by that time. Overall, the magnesium stearate level and its blending time were either not significant or among the least significant factors affecting piroxicam dissolution in these formulations. This observation may be largely explained by the ability of sodium lauryl sulfate to overcome the hydrophobicity of magnesium stearate. The water solubility of lactose and the presence of the disintegrant, sodium starch glycolate, may also help overcome hydrophobic lubricant effects.
Formulation or Process Variable | Level Studied | ||
---|---|---|---|
Low | Medium | High | |
Surfactant: sodium lauryl sulfate | 0% | 0.5% | 1.0% |
Lubricant: magnesium stearate | 0.5% | 1.0% | 1.5% |
Lubricant blend time | 2 min | 10 min | 18 min |
Filler (expressed as % lactose in binary mixtures with microcrystalline cellulose) | 0% | 50% | 100% |
Drug particle size (expressed as % milled [4.91 M2/g] piroxicam in binary mixtures with unmilled [1.79 M2/g] piroxicam) | 0% | 50% | 100% |
Source: DA Piscitelli, S Bigora, C Propst, S Goskonda, P Schwartz, L Lesko, LL Augsburger, D Young. The impact of formulation and process changes on in vitro dissolution and bioequivalence of piroxicam capsules. Pharm Devel Tech 1998; 3(4): 443–452.

Figure 1.2Effect of piroxicam-specific surface area, sodium lauryl sulfate (SLS), and lactose on dissolution. (Plotted from University of Maryland–FDA data [Food and Drug Administration, RFP 223-91-3401].) Q10 = percent dissolved in 10 min; Q45 = percent dissolved in 45 min; % SLS = percent sodium lauryl sulfate; % lactose = percentage of lactose in a binary filler consisting of a blend of lactose and microcrystalline cellulose.
Three formulations from this study having Fast, Moderate, and Slow dissolution were chosen for comparison to a lot of the innovator’s product in a human bioavailability study.41 The resulting pharmacokinetic data provided still another opportunity to examine the effects of formulation variables. Using the following previously developed deconvolution-based model (Equation 1.1),47 Polli developed an in vitro–in vivo relationship (IVIVR) that pointed up the role of formulation in overcoming piroxicam’s low solubility/high permeability properties.48

Fd is the fraction of the dose dissolved in time t, Fa is the fraction of the dose of the drug absorbed in time t, fa is the fraction of the total amount of drug absorbed at t = ∞, and α is the ratio of the apparent first-order permeation rate constant, kp1, to the first-order dissolution rate constant, kd. The model makes the following simplifying assumptions: apparent first-order permeability, first-order dissolution under sink conditions, drug is stable in the gastrointestinal lumen or at the gastrointestinal wall, in vitro and in vivo dissolution profiles are identical, and the absence of a lag time for permeation or dissolution. The term α is of particular interest to this discussion. If an analysis reveals α ≫ 1, the permeation rate constant is much greater than the dissolution rate constant and drug absorption is expected to be dissolution rate-limited. If α ≪ 1, then the permeation rate constant is much less than the dissolution rate constant, signifying that the rate-limiting step to absorption is intestinal permeation.
The values of α determined for the Slow, Moderate, and Fast formulations and a lot of the Innovator product are given in Table 1.2. An examination of the data indicates that piroxicam exhibits generally dissolution rate-limited (i.e., α > 1) absorption, which is consistent with piroxicam’s BCS classification. For the Slow formulation, α = 6.50, which indicates that dissolution was dominating over permeability about sevenfold in controlling overall piroxicam absorption. What is particularly interesting about the analysis in Table 1.2 are the formulation implications. For example, the use of milled piroxicam, 1% sodium lauryl sulfate, and lactose filler so promoted dissolution in the Fast formulation that kd≅kp1 and α is slightly less than 1, indicating that because of formulation design, the Fast product is slightly more permeability rate-limited than dissolution rate-limited, thereby overcoming piroxicam’s biopharmaceutic properties. It is also interesting that the in vitro dissolution test (USP) proved more sensitive to the piroxicam formulation variables than the biodata. Despite their differences in dissolution rate, the Fast, Moderate, and Slow products were found bioequivalent to each other and to the lot of Innovator product studied. It is possible that either the formulation variables studied did not affect in vivo dissolution or the differences were not discernible because of the long biological half-life of piroxicam.49
Formulation | α=kp1kd,±SE |
---|---|
Fast (Q45 = 95) | 0.896 ± 0.138 |
Moderate (Q45 = 87) | 1.54 ± 0.24 |
Innovator (Q45 = 80) | 3.42 ± 0.84 |
Slow (Q45 = 65) | 6.50 ± 2.17 |
Source: JE Polli. Analysis of in vitro–in vivo data. In: GL Amidon, JR Robinson, RL Williams, eds. Scientific Foundations for Regulating Drug Products. Arlington, VA, AAPS Press, 1997, pp. 335–351.
Note: Q45 = percent labeled content dissolved in 45 min; SE = standard error.
Statistical methods have also been applied to the formulation development of dry powder inhalation (DPI) capsules. Nearly half of marketed DPIs are single-dose delivery systems consisting of a powder formulation in a hard shell gelatin capsule. In practice, the capsule is punctured in a specially designed inhalation device and the powder is inhaled by the patient. In one example, Faulhammer et al.50 undertook a study aimed at development of a predictive model for the filling of the typically low fill-weight capsule inhalation products with a dosator nozzle. Based on an assessment of the effects of the critical process parameters (CPPs) and critical material attributes (CMAs) on fill weight and weight variability, they developed and validated a predictive model for fill weight variation for two groups of powders: those that flow well and those that are cohesive. The model provided a “good approximation” of fill weight variability for each powder group and permitted the establishment of a DS for different types of inhalation grade lactose in low-fill weight capsule filling.
In another study of low-fill weight capsule filling, Faulhammer et al.51 examined the effect of CMAs on the critical quality attributes (CQAs) of capsules filled on a dosator machine using six different grades of microcrystalline cellulose representing a wide range in physical properties. An experimental design was developed using four filling machine process parameters as controllable variables: dosator size, dosing chamber length (sometimes referred to as the piston height setting), powder layer height (often also called powder bed height), and filling speed. The data were analyzed by multivariate analysis with partial least squares regression (PLA). The Faulhammer group also used experimental design to evaluate the effect of these dosator machine process parameters on the capsules with various forms of lactose.52 A partial least square (PLS) method was used to examine the correlations between the material properties and process parameters and capsule fill weight and weight variability.
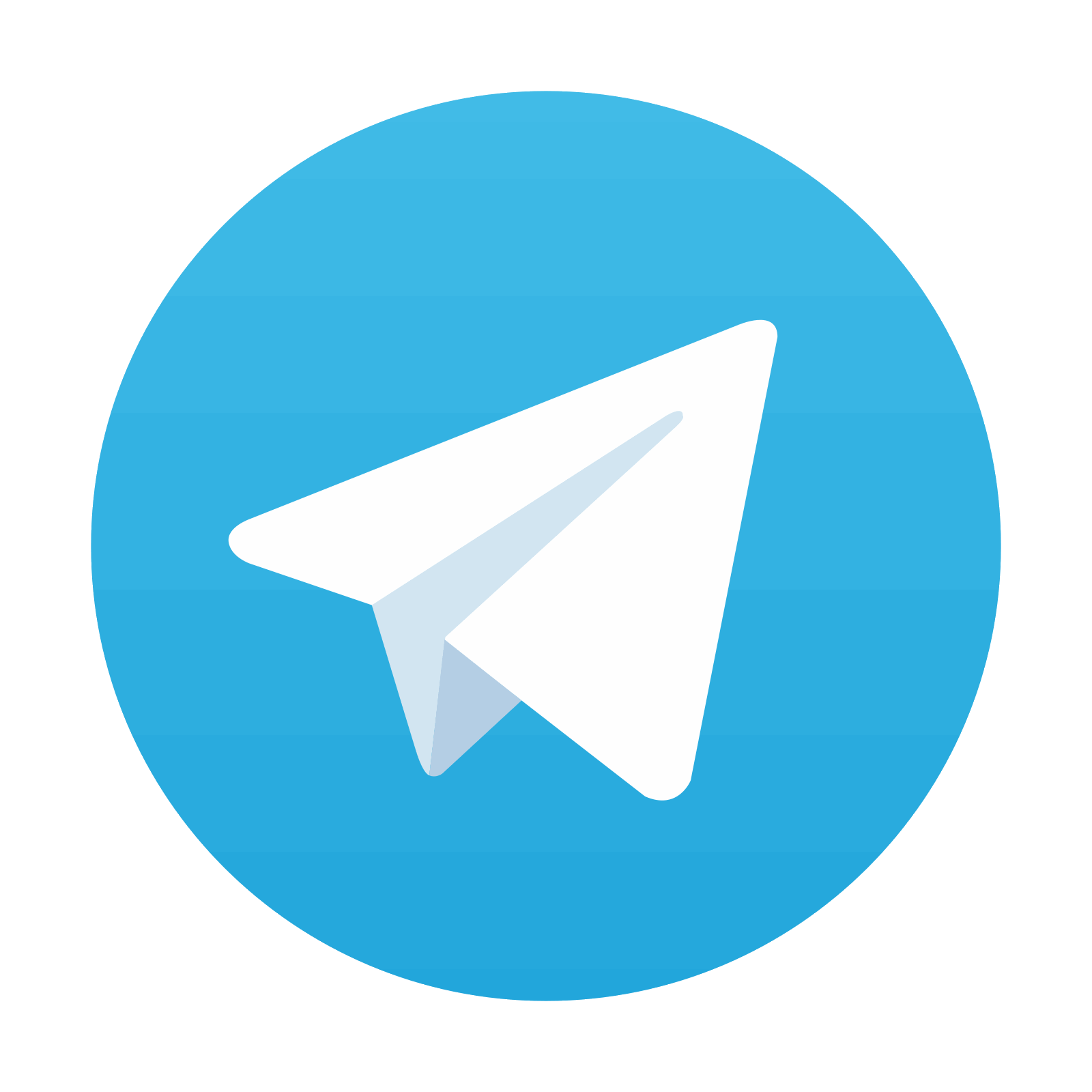
Stay updated, free articles. Join our Telegram channel

Full access? Get Clinical Tree
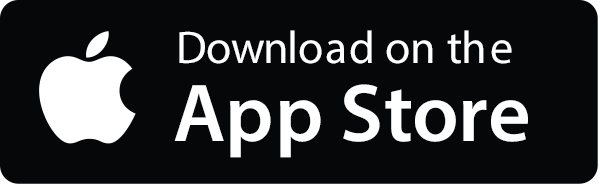
