4 The Study of Risk Factors and Causation
Epidemiologists are frequently involved in studies to determine causation—that is, to find the specific cause or causes of a disease. This is a more difficult and elusive task than might be supposed, and it leaves considerable room for obfuscation, as shown in a newspaper article on cigarette smoking.1 The article quoted a spokesman for the Tobacco Institute (a trade association for cigarette manufacturers) as saying that “smoking was a risk factor, though not a cause, of a variety of diseases.”
I Types of Causal Relationships
Most scientific research seeks to identify causal relationships. The three fundamental types of causes, as discussed next in order of decreasing strength, are (A) sufficient cause, (B) necessary cause, and (C) risk factor (Box 4-1).
Box 4-1 Types of Causal Relationships
Sufficient cause: If the factor (cause) is present, the effect (disease) will always occur.
Necessary cause: The factor (cause) must be present for the effect (disease) to occur; however, a necessary cause may be present without the disease occurring.
Risk factor: If the factor is present, the probability that the effect will occur is increased.
Directly causal association: The factor exerts its effect in the absence of intermediary factors (intervening variables).
Indirectly causal association: The factor exerts its effect through intermediary factors.
Noncausal association: The relationship between two variables is statistically significant, but no causal relationship exists because the temporal relationship is incorrect (the presumed cause comes after, rather than before, the effect of interest) or because another factor is responsible for the presumed cause and the presumed effect.
B Necessary Cause
Cigarette smoking is not a necessary cause of bronchogenic lung cancer because lung cancer can and does occur in the absence of cigarette smoke. Exposure to other agents, such as radioactive materials (e.g., radon gas), arsenic, asbestos, chromium, nickel, coal tar, and some organic chemicals, has been shown to be associated with lung cancer, even in the absence of active or passive cigarette smoking.2
D Causal and Noncausal Associations
The first and most basic requirement for a causal relationship to exist is an association between the outcome of interest (e.g., a disease or death) and the presumed cause. The outcome must occur either significantly more often or significantly less often in individuals who are exposed to the presumed cause than in individuals who are not exposed. In other words, exposure to the presumed cause must make a difference, or it is not a cause. Because some differences would probably occur as a result of random variation, an association must be statistically significant, meaning that the difference must be large enough to be unlikely if the exposure really had no effect. As discussed in Chapter 10, “unlikely” is usually defined as likely to occur no more than 1 time in 20 opportunities (i.e., 5% of the time, or 0.05) by chance alone.
II Steps in Determination of Cause and Effect
Investigators must have a model of causation to guide their thinking. The scientific method for determining causation can be summarized as having three steps, which should be considered in the following order3:
These steps in epidemiologic investigation are similar in many ways to the steps followed in an investigation of murder, as discussed next.
A Investigation of Statistical Association
The relationship between smoking and lung cancer provides an example of how an association can lead to an understanding of causation. The earliest epidemiologic studies showed that smokers had an average overall death rate approximately two times that of nonsmokers; the same studies also indicated that the death rate for lung cancer among all smokers was approximately 10 times that of nonsmokers.4 These studies led to further research efforts, which clarified the role of cigarette smoking as a risk factor for lung cancer and for many other diseases as well.
In epidemiologic studies the research design must allow a statistical association to be shown, if it exists. This usually means comparing the rate of disease before and after exposure to an intervention that is designed to reduce the disease of interest, or comparing groups with and without exposure to risk factors for the disease, or comparing groups with and without treatment for the disease of interest. Statistical analysis is needed to show that the difference associated with the intervention or exposure is greater than would be expected by chance alone, and to estimate how large this difference is. Research design and statistical analysis work closely together (see Chapter 5).
If a statistically significant difference in risk of disease is observed, the investigator must first consider the direction and extent of the difference. Did therapy make patients better or worse, on average? Was the difference large enough to be etiologically or clinically important? Even if the observed difference is real and large, statistical association does not prove causation. It may seem initially that an association is causal, when in fact it is not. For example, in the era before antibiotics were developed, syphilis was treated with arsenical compounds (e.g., salvarsan), despite their toxicity. An outbreak of fever and jaundice occurred in many of the patients treated with arsenicals.5 At the time, it seemed obvious that the outbreak was caused by the arsenic. Many years later, however, medical experts realized that such outbreaks were most likely caused by an infectious agent, probably hepatitis B or C virus, spread by inadequately sterilized needles during administration of the arsenical compounds. Any statistically significant association can only be caused by one of four possibilities: true causal association, chance (see Chapter 12), random error, or systematic error (bias or its special case, confounding, as addressed later).
Several criteria, if met, increase the probability that a statistical association is true and causal6 (Box 4-2). (These criteria often can be attributed to the 19th-century philosopher John Stuart Mill.) In general, a statistical association is more likely to be causal if the criteria in Box 4-2 are true:
Box 4-2 Statistical Association and Causality
Factors that Increase Likelihood of Statistical Association Being Causal
The association shows strength; the difference in rates of disease between those with the risk factor and those without the risk factor is large.
The association shows consistency; the difference is always observed if the risk factor is present.
The association shows specificity; the difference does not appear if the risk factor is absent.
The association has biologic plausibility; the association makes sense, based on what is known about the natural history of the disease.
The association exhibits a dose-response relationship; the risk of disease is greater with stronger exposure to the risk factor.
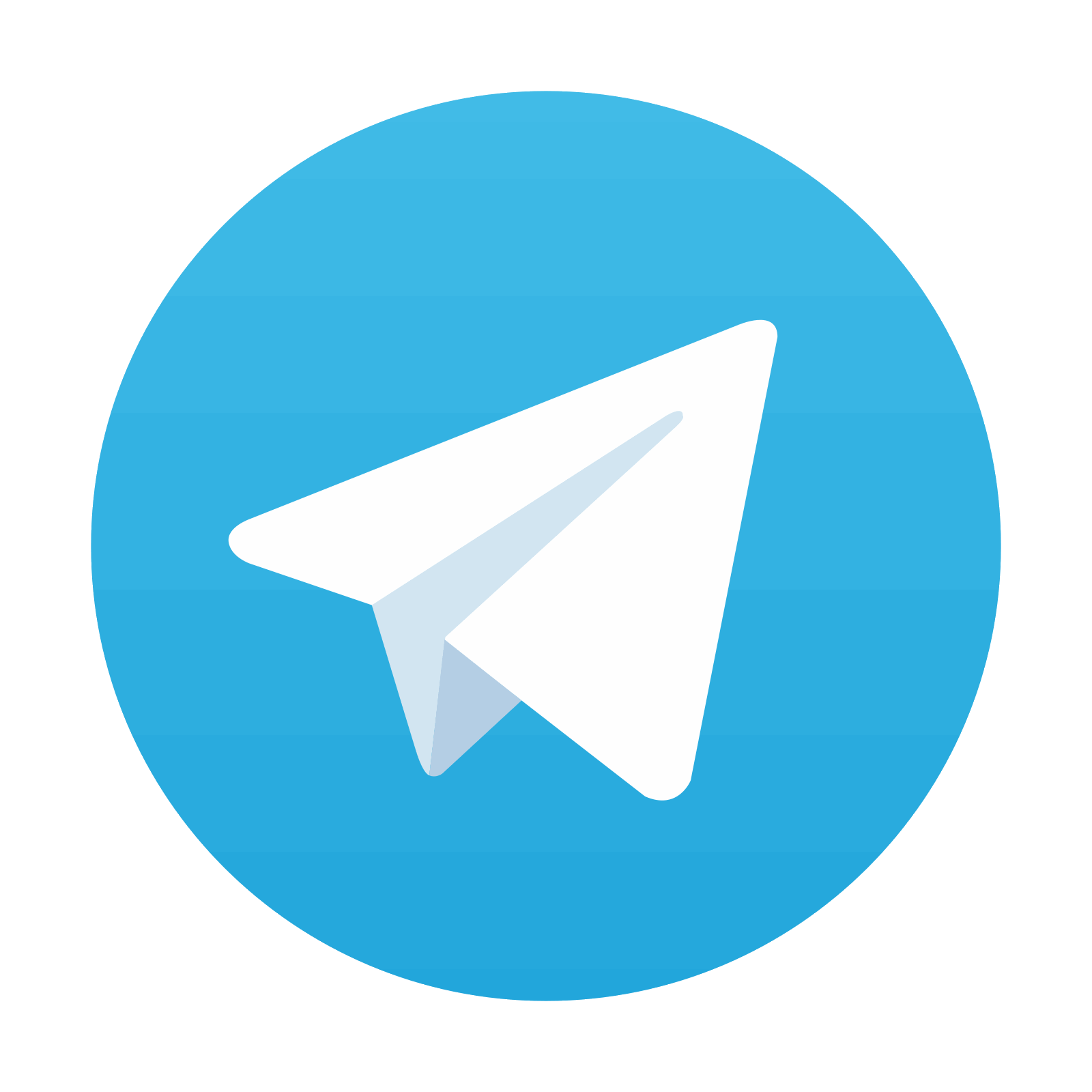
Stay updated, free articles. Join our Telegram channel

Full access? Get Clinical Tree
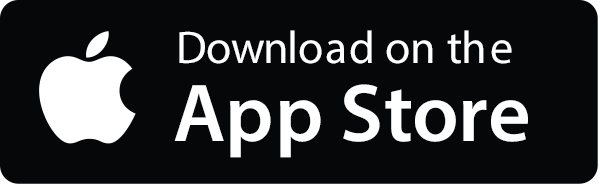
