Figure 10.1. Case and control study designs.
Cohort Study
Studies a group of people and observes the development of the disease, usually after the study participants have been genotyped (Figure 10.2). Thus, there are fewer issues with control selection, which is the main weakness in case or control studies.14
Challenges
When the Human Genome Project was launched, hopes were high to rapidly discover genes relevant to disease. However, genetic information or associations do not directly transfer into new targets for drugs. Substantial challenges remain in this field, including aspects of diagnostic technology, biological complexity and phenotypical alterations not related to genotype.
- Association studies are based on medical data that are often determined subjectively, rather than in an objective, quantitative way. A good example is pathology scores, which have been shown to vary vastly among different pathologists. Thus, currently available diagnostic markers used in association studies are limited in quantity and objectivity. There is much room for novel diagnostic technology to be established or further developed to provide quantitative markers that can be better meshed with genetic data in a correlative fashion.
- Obstacles are in the vast complexity of cellular mechanisms where a single gene rarely acts on its own, but rather in concert with others and their products connected by intracellular pathways or networks. Thus, a critical point is to decipher these networks of interactions and to determine key hubs that are most likely targets for drug candidates. This is the area of systems biology, developing complex models which reflect metabolic or signalling processes in cells that can predict how the network would change in case of variations or drug intervention.
- Many diseases such as cancer, diabetes mellitus and dementia are chronic diseases that occur more frequently in old age. The incidence of these diseases is low until mid-age, but rises exponentially thereafter. In a way, it is the aging organism that increases sensitivity for genetic variants to turn into drivers for disease. What looms behind this insight is a complete rethinking of current practice of a drug treatment ‘after the fact’ towards a preventive strategy for healthy aging. Personalised screens are the first step to determine risk factors; however, scientific insight into the interaction of the aging process with genetic variations is only at its beginning, as there is substantial complexity in the aging process.
For the medical sciences and its commercial enterprises to progress, one should keep an open-minded view of the research landscape and how pharmacogenomics is related to other developments, schools of thought and technologies.
Exploring Research Areas/Ideas
Thus far, pharmacogenomics studies have focused their efforts in very specific areas for several reasons. These few classes of drugs are widely used, media-prominent and often have strong and dire adverse effects. Antihypertensive drugs, psychiatric medications and chemotherapeutic agents all fit to one or more of these descriptions. That being said, pharmacogenomics still offers a nearly bottomless pit of opportunities. Genomics is still a developing field and thus there are plenty of diseases that have not been studied yet, for the simple reason that there is too much to do! There are plenty of drugs in use that need to be studied, not to mention the potential for pharmacogenomic studies to be done during pharmaceutical research and development of newer drugs.
As previously mentioned, the best way to find ideas is to explore the current research in the field. After reading several journal articles, things will click! Meshing your personal experience and expertise with pharmacogenomics could yield a novel, interesting study. Searching the National Centre for Biotechnology Information (NCBI) PubMed database, HuGE Navigator (http://hugenavigator.net, human genome epidemiology network) or Google Scholar (also see Chapter 21) will all yield new breaking research in the area of pharmacogenomics. Talking to professors of genetics at universities and using your personal network of research colleagues is also helpful as the equipment needed to run genetic association studies is sometimes scarce and always expensive (this will be further discussed in the ‘Tools’ section of this review).
The NIH’s CRISP database (http://crisp.cit.nih.gov) is indispensible as it identifies grants that are being awarded and thus provides a less-filtered view of research than journals do. While journals publish breakthrough research, they are lagging behind the grants being awarded, since the lag between refining a process and publication may be a year or more.15
Seeking Support
Currently, especially given industry involvement and increased consumer interest, there is a lot of interest and grants to be won in the pharmacogenomics arena. Given the techniques explained in Section H, you should be able to write a stellar proposal and gain some support for your research idea. One of the minor difficulties that can be discussed in the proposal is that there are no intermediate publishing points in most pharmacogenomics research. Whereas the search for a novel oncogene may be published at the point of discovery, the point of mechanism identification and some others, a pharmacogenomics study is over and published mostly after concluding that an association is true. The CRISP database is also a good place to compare the quality of your proposal to the ones that have been granted funds in the past.
Communications
Among the many genetic association studies that have been published over the last several years, there has been a large spectrum in quality of data, presentation and writing. Because of the complexity of many of the data that pharmacogenomics studies present, there has been an increasing focus on the style of presentation of data, leading to the popularity of data gurus such as Edward Tufte.16 He has shown that there are clever and very effective ways of displaying complex information. However, there are some tables and graphs that have become very standard for pharmacogenomics and genome-association studies: linkage disequilibrium (LD) plots, contingency tables and call rate/frequency charts to name a few. We will discuss some of the most popular below.
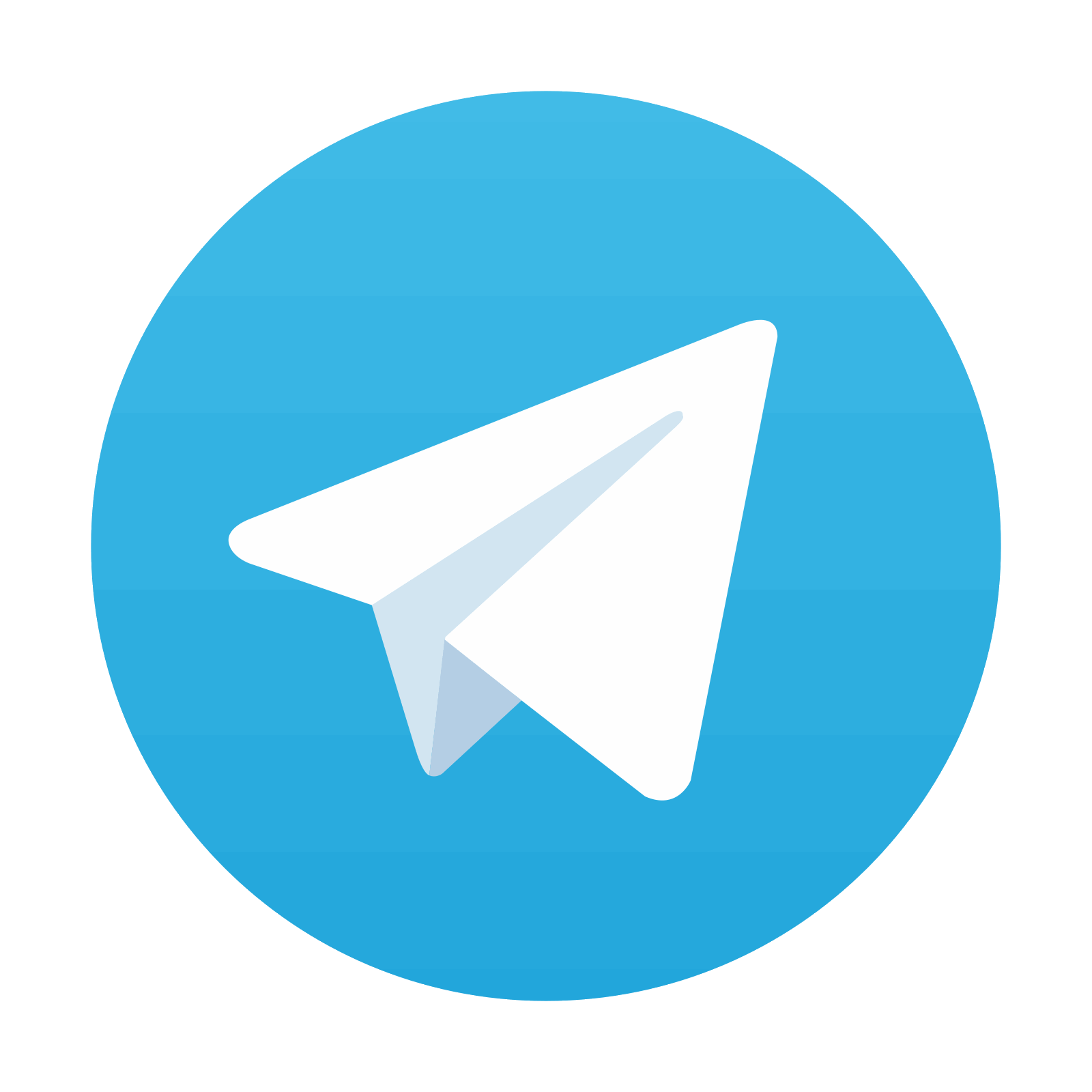
Stay updated, free articles. Join our Telegram channel

Full access? Get Clinical Tree
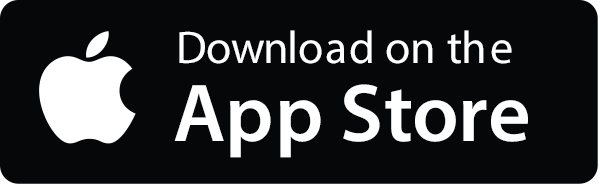
