This distribution provides probability rule for the realisation of r heads out of n tosses. The constant p is the parameter of this distribution, which is the actual probability of one success. A second example is a normal distribution
This distribution provides probability rule for the realization of values of y when the distribution of y has a bell shape. The constants µ and σ2 are the parameters of this distribution. Parameter µ is the real mean value of y and σ2 is the real variance of y. The symbol ‘~’ is used to denote a distribution. For example, X~N(µ, σ2) indicates, X has a normal distribution with mean µ and variance σ2. As mentioned above, in general, the values of parameters are unknown. However, since a sample is assumed to imitate the population, some estimations of these parameters are possible using the observed sample values. These estimates of parameters are known as statistics. An estimate may be only a single number describing a possible value of the associated parameter (point estimate), or an interval describing a probability that the parameter will be in that interval (interval estimation). In the following section, we will discuss estimation procedure of some common parameters.
Descriptive Statistics
Descriptive statistics are summary values of the observed sample. These summary values attempt to describe the characteristics of the associated population parameters. Some common descriptive statistics are mean, median, mode, moment (or more specifically pth moment about A, defined below), variance, standard deviation, standard error, mean deviation, etc. In this discussion, the symbol will represent sum of n observed x values. For example, if x has 5 values as x1 = 1, x2 = 4, x3 = 4, x4 = 6, and x5 = 7, then
= 1 + 4 + 4 + 6 + 7 = 22. Using this notation we define, sample mean
. Mean is generally denoted by µ for population and
for sample. Population mean of a variable X is also known as the expected value of X and is denoted as E(X). Median is the middle value of the observed sample values in magnitude. For its calculation, we first arrange the observed sample values in ascending order and pick the middle value in case of odd number of observations, and mean of middle two observations in case of even number of observations. Median is generally denoted by M for population and m for sample. Mode is the observation that is repeated most number of times. For its calculation, find how many times each observation is repeated. Pick the one that has highest repetition. An observed sample may have no mode or more than one modes. The pth sample moment about A is defined as
.
Moment is generally denoted by µp for population and by mp for sample.
Sample variance =
where is the mean of observed x values.
Note that variance is the 2nd moment about mean. Variance is generally denoted by V or σ2 for population and by v or s2 for sample. Standard deviation = . Standard deviation is generally denoted by SD or σ for population and by sd or s for sample. Coefficient of variation CV =
and the corresponding estimate is cv =
Note that CV is a unit free value of standard deviation relative to the mean. Since CV is unit free, it is convenient to compare the variation of different variables in terms of CV.
Sample mean deviation =
generally denoted by MD for population and md for sample. The mth percentile denoted by pm is defined as a value of the observed sample so that m% of the observations is less than this value and (100–m)% observations are greater than this value. The value p25 is known as the first quartile (Q1) and the value p75 is known as the third quartile (Q3). Therefore, there are 25% observations smaller than Q1 and 75% of observations are greater than Q1. Similarly, there are 75% observations smaller than Q3 and 25% of observations are greater than Q3. The second quartile Q2 = p50 is the same as the median. The difference IQR = Q3 – Q1 is known as the interquartile range. The SAS Proc univariate and Proc means provide some of these summary statistics.
Since a statistic is a function of the observed sample values, it is a random variable, that is, it varies from sample to sample. Therefore, it also has a distribution with some parameters. The distribution of a statistic is known as sampling distribution. For example if X ~ N(µ, σ2) then the sample mean ~ N(µ, σ2/n), that is,
has a normal distribution with mean = µ and variance = σ2/n. The square root of this variance is the standard deviation of
. In the statistical literature the standard deviation of a statistic is known as standard error (S.E.) of the statistics. Therefore, correctly
has a distribution with mean = µ and S.E(
) =
An estimate of this S.E. is
Example 1: Suppose plasma concentrations of a test substance in 10 animals have the following values, x = 4, 2, 1, 3, 5, 6, 5, 3, 6, 3. The calculated summary statistics (using SAS Proc univariate) are as follows: = 3.8, sd = 1.69, Median = 3.5, Mode = 3.0, CV = 44.38, Mean deviation = 1.40 and p75 = 5.
Sometimes more than one variable is measured from the same experimental unit. For example, the height, weight and blood pressure may be measured for each subject in the experiment. For such data, two important summary statistics are covariance and correlation coefficient. Both of these statistics measure the pairwise dependency of one variable on another. The correlation coefficient is the standardised value of covariance and is unit free. Therefore, the correlation coefficient is easy to interpret compared to covariance. The population correlation is generally denoted by ρ(x, y) or simply by ρ, while this for sample is denoted by r(x,y) or simply by r. These two summary statistics are calculated as follows.
Suppose x and y are two variables measured from same experimental unit, then the sample covariance
and the correlation coefficient
where n is the number of observations. It can be shown that the value of r is in (–1 to 1). A positive value of r implies a direct relation between x and y, that is, as x increases y also increases and vice versa. A negative value of r implies a reverse relation between x and y, that is, as x increases y decreases and vice versa. A value of r = 1 implies a perfect correlation between x and y with direct relation between x and y. In this case a graph between x and y falls on a straight line with positive slope. Whereas a value of r = –1 implies a perfect correlation between x and y with reverse relation between x and y. In this case a graph between x and y falls on a straight line with negative slope.
Example 2: Consider the following dataset obtained for age-related changes in plasma immunoglobulin M (IgM) level after immunisation determined in Wistar rats. The observed values are shown in Table 17.1. The details of the calculations of covariance and correlation coefficient are given in Table 17.2.

Number of observation, n = 11.
Table 17.2. Calculation of covariance and correlation coefficient
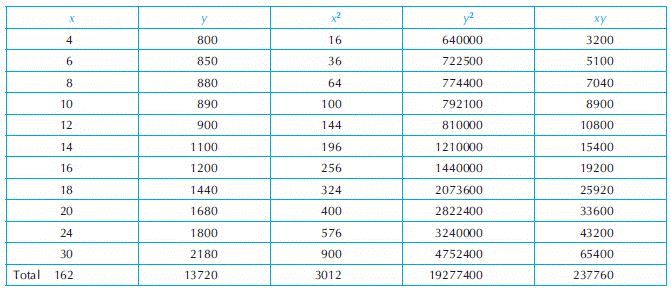
Results show that age and immunoglobulin have almost a perfect correlation. The SAS Proc corr can provide these estimates of covariance and correlation.
Estimations and Confidence Intervals
As mentioned before, the values of parameters are generally unknown. Some estimations of these parameters are possible using the observed sample values. In general, a sample proportion is the estimate of population proportion, sample mean is the estimate of population mean, sample variance is the estimate of the population variance, etc. For example, if we assume that the 10 observations in Example 1 are from a normal distribution N(µ,σ2), then the estimate of µ is calculated from the 10 observations, and estimate of variance σ2 is s2 calculated from the 10 observations. Sample estimates should have some desired properties, for example, unbiasedness, consistency, efficiency, sufficiency. Due to limitation of space, these properties will not be discussed here. Statistically, an estimate with more of these properties is assumed to be better.
Since a statistics is a function of the observed sample values, it is a random variable, that is, it varies from sample to sample. Therefore, a point estimate may be very close or far from the real value of the associated parameter. This difference cannot be enumerated by such a single value. An alternative to this point estimate is an interval estimate. This estimate calculates two values known as lower (l) and the upper (u) limits in such a way that the actual value of the parameter will be within these limits with a high probability. The interval defined by these two limits is known as the confidence interval, C.I.= (u,l). For example, if X is distributed as N(µ, σ2) then l = and u =
are the lower and the upper limits for the (1 – α)% confidence interval for µ. In a different notation, the (1 – α)% C.I. for µ is
. However, to be more accurate
from t-distribution with n – 1 degree of freedom (d.f.) should be used instead of
that is,
. The (1 – α)% C.I. for σ2 is l =
and u =
, where
is the χ2 value with n – 1 d.f. with α/2% tail to the left, and
is the χ2 value with n – 1 d.f. and (1 – α/2)% tail to the left.
The interpretation of this confidence interval is that if the calculation of confidence interval is repeated 100 times by taking sets of 100 independent samples using the above formula, then (1 – α)% times the actual value of µ is expected to be captured by the calculated intervals. If α = 0.05 then z1–α/2 = 1.96 is the 97.5% tail probability of standard normal distribution z~N(0.1). In this case the calculated confidence interval is known as the 95% confidence interval. Theoretically, confidence intervals can be calculated for any parameter. However, for some parameters the process may be quite complicated.
Example 3: Consider observations 3.9, 4.2, 4.2, 3.8, 3.6, 4.4, 4.1, 3.4, 4.0, 3.5, 3.7 and 4.2 came from N(µ, σ2). Suppose that both population mean µ and variance σ2 are unknown and we would like to calculate an 80% confidence interval for the mean µ.
We have
Thus,
From t-table t0.9,11 = 1.363 and the 80% confidence interval on µ is
Suppose µ1 and µ2 are population means from two populations. Also let x1, …, xm, and y1, …, yn be two samples for these two populations. The (1 – α)% confidence interval on the difference of these means µ1 – µ2 can be found as
Where sp =
Central Limit Theorem
If x1, x2, …, xn is a sample of size n from a population not necessarily normal with mean µ and variance σ2, Then for large n, the sample mean has approximately a normal distribution with mean µ and variance σ2/n. In notations we say, as
. This property of large sample is known as the central limit theorem. As a consequence of this theorem, statistical tests (discussed in a later section) originally meant for normal population are also applicable to non-normal populations for large sample.
Statistical Hypothesis Testing and Sample Size Calculation
Statistical Hypothesis Testing
As pointed out earlier, population parameters are generally unknown. However, sometimes they may have hypothetical values. It is sometimes possible to test if such hypothetical values of parameters are true using a representative sample observation from this population. This statistical procedure of verification is known as the test of hypothesis. Since we deal with the parameters of a population, the procedure is known as parametric method. In later part of this section we will discuss test of hypothesis not regarding parameters of any population. The procedure then will be called non-parametric method.
Formally, we first express a hypothetical true value of the parameter in the form of a hypothesis, known as the null hypothesis (H0), and possible other values of the parameter in the form of an alternative hypothesis (H1). In notations, H0: θ = θ0 and H1: θ > θ0 or H1: θ < θ0 (both of these are known as one-sided alternative) or H1: θ ≠ θ0 (known as two-sided alternative) where θ denotes a parameter. The general idea is to calculate the difference or ratio r =
between the estimate of the parameter and the corresponding hypothetical value and make a determination on the validity of the hypothetical value of the parameter based on the magnitude of this difference or ratio. A big absolute value of d indicates the falsehood of the null hypothesis and a small value of d indicates truthfulness of the null hypothesis. Similarly, a small or large value of r indicates the falsehood of the null hypothesis and a value of r very close to 1 indicates truthfulness of the null hypothesis. An important component of this process is the selection of a cut-off point on d (or r) to decide on the truthfulness or falsehood of the null hypothesis. For this purpose the difference (or the ratio) is standardised. This standardised value is known as test statistic (Z). A cut-off point is then determined based on the distribution of this statistics. There are two types of errors associated with this process, known as the Type 1 and Type 2 errors. They are defined in Table 17.3.
Table 17.3. Type 1 and type 2 errors

These two error types are enumerated by percent of times they happen.
The Type 1 error is generally denoted by α and the Type 2 error is generally denoted by β. We want these errors to be as small as possible. The cut-off point for the test is generally fixed such that the Type 1 error is less than a pre-specified value. This cut-off point is known as the critical value and the corresponding Type 1 error (α) is known as the test level. Generally α is set to 5% or 1%. The value (1 – β) × 100 is known as the power of the test. There are two operational criteria to draw conclusion in a hypothesis testing known as (i) critical value criteria and (ii) p-value criteria. In the first criteria, for one-sided alternative with H1: θ > θ0, compare z with the critical value z1–α. If the calculated statistic z is greater than the critical value z1–α, reject the null hypothesis, accept otherwise. For one-sided alternative with H1: θ < θ0, compare z with the critical value zα. If the calculated statistic z is less than the critical value zα, reject the null hypothesis, accept otherwise. The critical values z1–α and zα are taken from standard tables. For two-sided alternative, compare |z| with the critical value z1–α/2. In the second criteria, for one-sided alternative H1: θ > θ0, calculate the p-value defined as p = P(Z > Z1-α). If this calculated p-value is smaller than the test level α reject the null hypothesis, accept otherwise. For one-sided alternative H1: θ < θ0, calculate the p-value defined as p = P(Z < zα). If this calculated p-value is smaller than the test level α reject the null hypothesis, accept otherwise. For two-sided alternative, calculate the p-value defined as p = P(|Z| > Z1–α/2). If this calculated p-value is smaller than α/2 reject the null hypothesis, accept otherwise. Clearly the two criteria are equivalent (Figure 17.1).
Sample Size Calculation
For any statistical hypothesis both Type 1 and Type 2 errors are sensitive to the sample size. In general, as the sample size becomes large both Type 1 and Type 2 errors become small. However, as the sample size becomes large, the cost, time and complexity of conducting the experiment grow. Therefore, for any experiment, a reasonable sample size is desirable. In practice, an acceptable Type 1 error and an acceptable Type 2 error are first determined and then the sample size is calculated to satisfy these two requirements. There are mathematical formulas that can calculate a sample size satisfying the required Type 1 and Type 2 errors. Because of the limitation of space, the derivation procedure of these mathematical formulas has not been discussed.
An example of these mathematical formulae for testing a single mean is as follows. Consider testing the hypothesis H0: µ = µ0 against the alternative H1: µ = µ1. Suppose that the intended Type 1 error = α and Type 2 error = β, then the sample size n that satisfies these requirements is calculated using the formula
where z(1-α) and z(1-β) are the (1 – α)% and (1 – β)% tail values of z, respectively. For example, consider a test with, H0: µ = 370 against H1: µ = 365. Assume σ = 20, α = 0.025, and β = 0.05 or power = 95%. We have z(1 – α) = 1.96 and z(1 – β) = 1.64. Therefore,
Thus, to perform a 0.05 level test for null hypothesis, H0: µ = 370 against H1: µ = 365 with a power of 95% we need a sample of about 208 subjects.
Also, for test for equality of two population means, with equal sample size from each population, we have the following formula. The null hypothesis H0: µ1 = µ2 and the alternative hypothesis . Suppose that the intended Type 1 error = α and Type 2 error = β, then the sample size n (from each population) that satisfies these requirements is
Presently, many statistical software packages are available to calculate the sample size under different conditions and requirements. Some of these softwares are nQuary, Power analysis, Sample size calculator, PASS, EAST, SAS, etc. These softwares are generally simple to use and highly accurate.
In the following sections we will discuss the test procedure for some common parametric and non-parametric methods for test of hypothesis.
Parametric Method
Test for Single Proportion
Assumptions: The inherent population is binomial with parameter p. The test level = α.
Observation: Sample x1, x2, …, xn of size n, where each xi is either 0 or 1.
Null hypothesis: H0: p = p0.
Alternative hypothesis: H1: p > p0 (one-sided alternative).
Test statistic
Conclusion: Reject H0 if where
is the
percentile of standard normal distribution (Z). If the alternative is H1: p < p0 then reject
. If the alternative is two-sided, that is, H1:p ≠ p0. Reject H0 if
(two tail test), where
is the
percentile of z.
Example 4: In an experiment 5 female mice were given a special diet to study its effect on the gender of their pups. During the study period there were 5 litters with a total of 32 pups (12 males and 20 females). The null hypothesis is that the diet did not affect the gender of the pups. Let p represent the proportion of males. If diet does not have any effect on gender, then p should be 0.5. Therefore, we have H0: p = 0.5 and H1: p < 0.5, n = 30, = –1.0954. From normal table, we have z0.05 = –1.64. Since –1.0954 > z0.05 we accept the null hypothesis, that is, the treatment did not have any effect on the gender of the pups.
Test for Two Proportions
Assumptions: The inherent populations are binomial. The test level = α.
Observation: Sample 1, x1, x2, …, xm of size m, and Sample 2, y1, y2, …, yn of size n where each xi and yj are either 0 or 1.
Null hypothesis: H0: p1 = p2.
Alternative hypothesis: .
Test statistic:
where is the pooled estimate of variance of p. The
is calculated as
Conclusion: Reject H0 if where
is the 1-α/2 percentile of t distribution with m + n – 2 degrees of freedom. If sample sizes are large alternatively reject H0 if
where z1-α/2 is the 1-α/2 percentile of z.
Example 5: Consider an example in which two treatments were applied on two groups of animals to evaluate the effect of treatment on survival. At the end of the experiment there were 425 deaths out of 500 animals in Group 1, and 459 deaths out of 500 animals in Group 2. We would like to test the equality of the proportions of deaths in the two treatment groups at test level 0.05. The null hypothesis is, H0: there is no difference between two treatments with regard to survival of animals.
Since sample size is very large we can use z test. From normal table z0.975 = 1.96. Since 3.35 > z0.975 we reject the null hypothesis and conclude that there is a statistically significant difference in survival of animals in the two treatment groups.
Test for Dose–Response Relationship (Trend) of m Incidence Rates (m Proportions)
Cochran-Armitage Test: Consider an experiment in which 40 animals were randomly but equally divided into four groups. Group 1 was control. Groups 2, 3 and 4 received, respectively, low, mid and high doses of an experimental drug. At the end of the treatment the presence and absence of a certain disease was observed. Table 17.4 shows the data.
Our interest is to test the dose–response relationship (trend) of incidence rates of the diseased animals. The null hypothesis is, H0: there is no dose–response relationship on the proportions of diseased animals. The Cochran–Armitage test statistic is defined as the following:
where xi is the ith dose, ni is the number of animals with disease in the ith dose group, Ni is the number of animals at risk in the ith dose group, The statistic χ2 has a χ2 distribution with 1 degree of freedom. The critical value χα,(1)2 can be obtained from the χ2 table.
Example 6: For the data in the above table χ2 = 11.06, with α = 0.05 the critical χ20.95,(1) value is 3.84. Since 11.06 > χ20.95,(1), we reject the null hypothesis (that there is no dose–response relationship) and conclude that there exists a statistically significant dose–response relationship in the proportions of diseased animals.
Test for Single Mean
Assumptions: The inherent population is normal. The test level = α.
Observation: Sample x1, x2, …, xn of size n.
Null hypothesis: H0: µ = µ0.
Alternative hypothesis: H1: µ > µ0.
Test statistic: if σ2 is known and
is unknown
where s =
Conclusion: Reject H0 if is known, and
is unknown, where z1-α is the 1–αth percentile of z distribution,
is the 1–α percentile of t distribution with n – 1 d.f., which can be obtained from a t-table. If the alternative is two-sided, for example
, reject H0 if
is known, and
if σ2 is unknown.
Example 7: Suppose we have the following 10 observations from an experiment: 10.2, 11.0, 12.8, 10.8, 11.1, 9.9, 12.1, 10.2, 11.6 and 11.4. We would like to test the hypothesis H0: µ = 11 against the alternative H1: µ > 11 with lest level α = 0.05. The population variance σ2 is assumed to be unknown. We have n = 10,
From the table we accept the null hypothesis and conclude that the population mean, from which the sample came, is 11.
In the above discussion the population was assumed to be normal. Therefore, before applying this test it is necessary to test the normality assumption of the population. Several descriptive methods can be used to check for normality. A brief description of some of these methods is as follows:

Example 8: An example of drawing a normal plot is as follows. Suppose we have 11 observations 36.3, 32.7, 40.5, 36.2, 38.5, 36.3, 41.0, 37.0, 37.1, 39.9 and 41.0. We want to plot a normal probability plot (Figure 17.2). Calculations are shown in Table 17.5.
The plot does not look like a straight line. Therefore, the observations do not appear to be coming from a normal population. However, in this example the sample size is too small. For an accurate conclusion the sample size needs to be reasonably large.
Test for Equality of Two Means from Two Populations
Assumptions: The two inherent populations are normal with equal variances. The test level = α.
Observation: Sample x11, x12,… of size n1 from Population 1 and x21, x22,…
of size n2 from Population 2.
Null hypothesis: H0: µ1 = µ2.
Alternative hypothesis: H1: µ1 > µ2.
Test statistic: z = if the common variance σ2 is known, and
Conclusion: Reject H0 if z ≥ z1-α if the common variance σ2 is known, and if the common variance σ2 is unknown, where z1-α is the 1-α percentile of z distribution and
is the 1-α percentile of t distribution with n1 + n2 – 2 d.f. If the alternative is two-sided, for example
reject
if the common variance σ2 is known, and
if the common variance σ2 is unknown.
Example 9: Suppose we have 60 observations from each of the two populations with mean vales of µ1 and µ2, respectively. Suppose the common population variance σ2 is unknown. Let following be the summary statistics from the samples: The null hypothesis H0: µ1 = µ2, and alternate hypothesis
, also let α = 0.05. We have,
From table t0.975,(158) = 1.96. Since t > t0.975,(158) = we reject H0, that is, we reject µ1 = µ2.
In the above discussion, the variances of the two populations were assumed to be equal. Therefore, before applying this test it is necessary to test the equality of the population variances. A test for the equality of variances is given in a later section. If the test reveals that the population variances are not equal, the problem is popularly known as the Behrens-Fisher problem. In this case the test statistic is defined as
and the degrees of freedom is estimated as
Test for Equality of Several Means
Before describing the test procedure for several means, we need to discuss a statistical technique known as analysis of variance. The discussion will be very brief. A complete description of this topic needs much deeper statistical discussion which is outside the scope of this chapter. The interested readers are referred to the book by Hogg and Tanis.1 Suppose m different doses of a drug are tested on m groups of similar patients. The drug is expected to be effective and change the disease level. The data may be written as shown in Table 17.6.
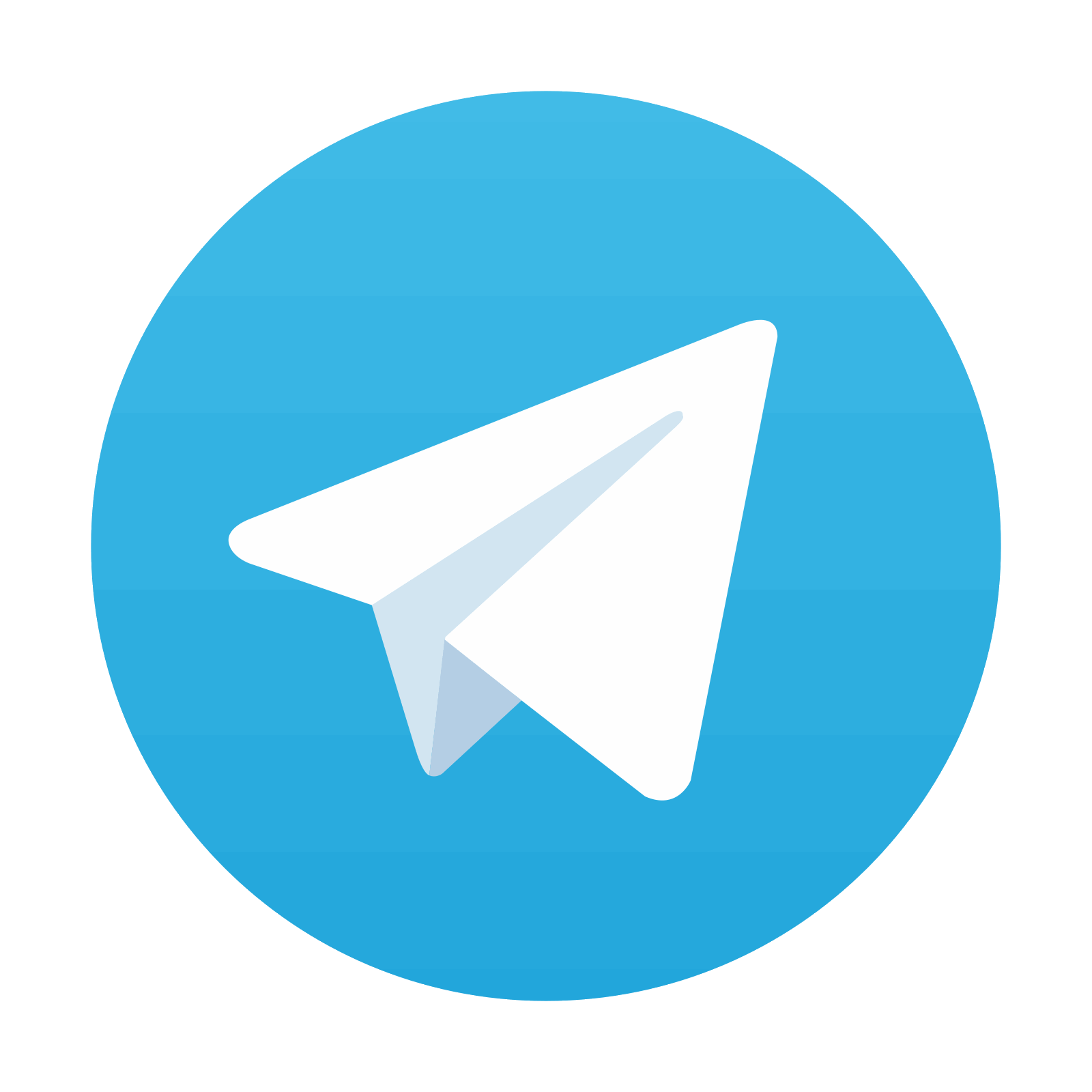
Stay updated, free articles. Join our Telegram channel

Full access? Get Clinical Tree
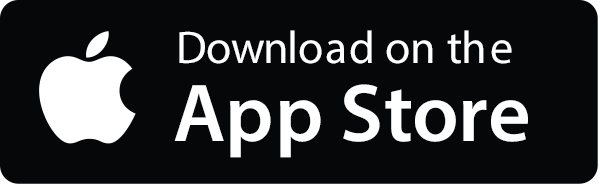
