Fig. 8.1
RT-PCR. Following RNA purification and construction of tagged cDNAs, PCR amplification is done with quantitative results available in real time. PCR cycles required to shift from an initial linear to the exponential phase indicates the relative abundance of the original cDNA (left). Obtaining the inflection points, or slopes, of the reaction along with the known starting concentrations allows for a standard curve (right) to be developed and number of gene copies to be quantified. RT-PCR allows for comparison of gene expression between conditions, and newer computational analysis techniques allow for quantification and statistical analysis (© 2005 by Steven M. Carr after Gibson and Muse)
RNA Sequencing
As described in more detail previously, RNA sequencing encompasses a newer technique for measuring gene expression and allows for quantification of RNA molecules. Many commercial platforms of transcriptome sequencing are available. Through the use of laser scanning, this technique allows for the analysis of many sequencing reactions occurring on glass slides and yields millions of RNA sequence reads [60]. Unlike oligonucleotide arrays and PCR-based techniques, transcriptome sequencing offers improved ability to detect low-abundance transcripts, as well as detection of new polymorphisms within a transcript sequence.
Analysis and Interpretation of Microarrays and Gene Expression Profiling
The use of microarrays and genetic expression profiling patterns has increased dramatically recently in both basic science as well as clinical laboratories. The ability to compare an abundance of gene expression levels simultaneously across conditions makes these techniques quite valuable and reliable. Increasing complexity of the technique used requires one to become familiar with how to process and analyze the data derived. General areas regarding the interpretation are normalization, quality assessment, preprocessing, data analysis, multiple comparison problem, and interpretation.
Normalization
Many microarray platforms require the use of fluorescence for detection of amplified transcripts. Normalization allows for the standardization of fluorescence levels across all experiments done. Standardization in this manner allows for comparison across experiments. Normalization is important given that each microarray in itself can be thought of as an experiment unto itself. Discussed in the preparation of microarrays earlier, changes in labeling efficiency or differences in starting mRNA can greatly impact output readings [61]. Normalization deals with these differences by adjusting fluorescent intensity to mRNA bound probes allowing for comparison across arrays.
Normalization can be accomplished through scaling, quantile normalization, and locally weighted scatterplot smoothing (LOWESS). Scaling refers to adjustment of intensities across arrays by a constant factor allowing for average expression levels among arrays to be similar. Quantile normalization allows for the distribution of intensities to be adjusted across arrays. Distribution adjustment is accomplished by ranking all probe intensities from highest to lowest and assigning numerical values to the rankings. Locally weighted scatterplot smoothing allows for normalization in two color arrays [61]. The use of this normalization technique requires differing the brightness and darkness of the fluorescence labels.
Quality Assessment
Quality assessment should occur both before and after normalization. Quality assessments ensure that all preparation steps in developing array were accomplished successfully. Pre-normalization assessment should ensure that there are no mechanical issues with array prep such as scratches, bubbles, or other artifacts contained on the array. The use of certain controls in commercially available steps that are done during sample prep help ensure all steps in array prep were accomplished. Quality assessment should continue post-normalization to evaluate a microarray relative to other arrays in the same experiment. Post-normalization quality assessment identifies outlier samples or significant differences in batches of microarrays. This analysis allows for statistical adjustment of the significant outliers or for them to be excluded altogether from further analysis.
Preprocessing
Preprocessing allows for data acquired to conform to a normal distribution as most statistical analyses rely on this. Usually gene expression profiles require conversion to a logarithmic scale to achieve normal distribution. The use of preprocessing can identify and allow for exclusion of low-quality probe sets as well as genes that have relatively low variability across all samples in the array.
Data Analysis
Microarrays using fluorescence detection typically provide an image file for analysis with raw data files containing upwards of 1 GB of data [60]. The use of large databases allows easier analysis techniques as well as storage of clinical or experimental variables pertaining to samples and quality assessed preprocessed gene expression levels. Analysis of the data can be accomplished a variety of ways from simple statistical methods to development of new algorithms. There exist many commercially available computer programs and computational software that allow for the ability to analyze differential expression, network analysis, class prediction, and class discovery.
Determination of differential expression is likely the most used analyses on microarray data. Differential expression allows for the identification of variability of gene expression in one condition compared to another and is usually accomplished through the use of t-test, analysis of variance (ANOVA), or linear modeling. Network analysis allows for identification of potential new interactions between genes and their expression [62]. The use of various algorithms allows for inferences to be made regarding one gene’s interaction with others. The use of class prediction analysis requires samples from two conditions to be split into a training set as well as a test set. The training set of samples allows for a list of genes that separate the two conditions, and the test set allows for determination of the accuracy of the prior. Class discovery analysis allows for the possible identification of novel phenotypes. Determination of closely correlated samples can be done based only on gene expression regardless of clinical phenotype.
Multiple Comparison Problem
Unique issues arise when dealing with statistical analyses of such large datasets. With increasing number of statistical tests performed, there is higher likelihood of a false-positive result. Consider that making a Type I error is defined by the p-value assigned, typically 0.05 or a 1 in 20 chance of this error occurring. Given that microarrays can compare thousands of genes analysis of 5,000 genes with a p-value of 0.05, there would be 250 Type I errors, typically an unacceptable number of false positives.
Methods are available to assist in lowering the probability for Type I errors. The Bonferroni correction, Benjamini-Hochberg false discovery rate, and the Q-value are methods to decrease Type I rates. The Bonferroni correction lowers the Type I error probability by adjusting the p-value. Use of the Bonferroni correction requires the desired p-value to be divided by the number of total number of tests making it significantly less likely to have a Type I error but also less likely to identify real differences. An additional approach is to attempt to control the false-positive rate with the Benjamini-Hochberg false discovery rate (FDR) [63]. FDR is the percentage of expected false-positive rate based on p-value compared to total number of tests ran. Use of the Q-value expands on the FDR and allows for some toleration of false positives while still recognizing the impact of the larger proportion (i.e., 95 % for p-value of 0.05) [64]. The Q-value indicates the false-positive rate at the level a gene expression is deemed to be significant statistically [64].
Interpretation
Interpretation of the vast amount of information gained from microarrays in biologically contextual ways remains a challenge. The use of large microarray datasets allows for large comparisons to be made among expression signatures [65], gene probes [66] as well as some encompassing phenotypic data [67–69]. Though many genes’ expression and networking may be obtained from arrays, identifying those that are meaningful from a biologic phenotypic point may be difficult to detect. Enrichment ranking and gene set enrichment analysis (GSEA) are examples to assist in linking expression profiles to phenotypic expression [67, 68]. Additionally the use of heat maps using columns of samples and genetic rows allow for analysis of groups of genes or samples that share genetic profiles [70].
Basic Science and Clinical Applications of RNA and DNA Analysis
The depth and amount of data obtained from techniques described above, at times overwhelming, can provide great insight into biomolecular processes, disease origination, prognosis, potential therapeutics, and novel genetic permutations. Application of these techniques in basic science labs has led to great translational impact in certain medical fields. Pathology, which once relied solely on experience and histopathology for diagnosis and prognostication, has seen genetic profiles offer assistance in certain malignancies. Both surgical and medical oncology have benefited from genetic analysis of both hematologic and solid tumor genetic profiling for both classification, treatment, prognostication, and to help guide patients in difficult decision making. Commercially available profiling in breast cancer (Oncotype Dx™, Genomic Health, Inc., Redwood City, California) has greatly enhanced discussion regarding the benefit of chemotherapy in certain breast cancers.
The impact that genetic analysis has had in basic science research is broad and beyond the scope of this chapter. However, without the great application of the techniques described above, the impact of recent discoveries would have proved difficult. Techniques described here allow for the discovery of genetic pathways both known and unknown. Expansion of the techniques described allow for the wealth of knowledge and techniques in genetically engineering models of research. Today one may find it difficult to read thorough a peer-reviewed journal and not find the use of knockout or transgenetically engineered organisms or in vitro models; also the use of RT-PCR, microarrays, or sequencing data and techniques permeates all phases of basic science research at one time or another.
Conclusion
At its core basic science research has been trying to unlock the secrets contained within genomes since the beginning. From the discovery of chromosomes in the mid-nineteenth century to Watson and Crick’s amazing work in the 1950s to the sequencing of the human genome in 2001, our understanding of the ultimate life building block is still growing and by no means complete. For example, the original sequencing of the human genome took nearly a decade and countless millions of dollars to complete; today sequencing of an entire human’s genome can be accomplished in hours and likely in the not so distant future minutes. Current advances as well as those not known yet will augment our means to gain understanding of an amazingly complex system.
References
1.
International Human Genome Sequencing Consortium. Finishing the euchromatic sequence of the human genome. Nature. 2004;431(7011):931–45.CrossRef
2.
3.
4.
5.
Mathews CK, van Holde KE, Appling DR, Anthony-Cahill SJ. Biochemistry. 4th ed. Toronto: Prentice Hall; 2012.
6.
7.
8.
9.
Prober JM, Trainor GL, Dam RJ, Hobbs FW, Robertson CW, Zagursky RJ, et al. A system for rapid DNA sequencing with fluorescent chain-terminating dideoxynucleotides. Science. 1987;238(4825):336–41.PubMedCrossRef
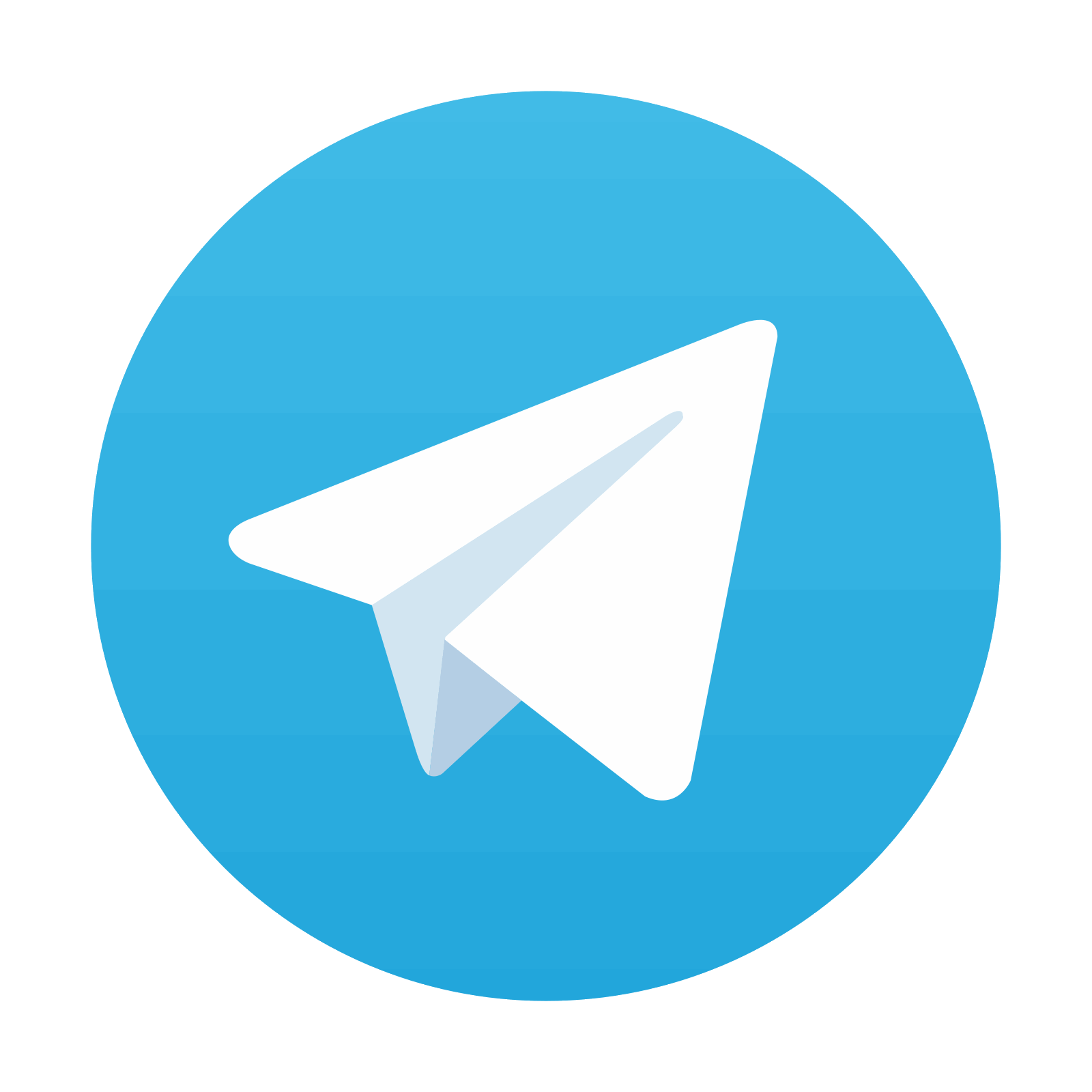
Stay updated, free articles. Join our Telegram channel

Full access? Get Clinical Tree
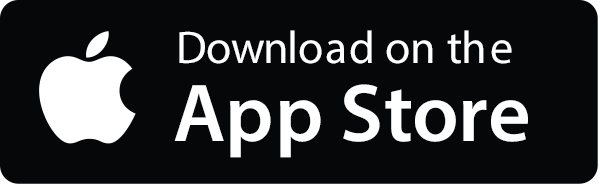
