Feature
Described association
Reference
Tumor-related characteristics
Lymph node involvement (N stage)
Survival outcome, RFS
Pantuck [74]
Distant metastases (M stage)
Survival outcome
Zisman et al. [18]
Fuhrman grade
Survival outcome, RFS
Fuhrman [75]
Tumor size
Survival outcome, RFS
Frank et al. [58]
Extension into perinephric fat/renal sinus fat
Survival outcome, RFS
Thompson [76]
Adrenal involvement
Survival outcome
Thompson et al. [76]
Venous involvement
Survival outcome, RFS
Martinez-Salamanca [77]
Metastatic burden
Survival outcome
Collecting duct invasion
RFS
Klatte et al. [78]
Micro-vascular invasion
Survival outcome, RFS
Kroeger et al. [32]
Sarcomatoid features
Survival outcome, RFS
de Peralta-Venturina et al. [36]
Tumor necrosis
Survival outcome
Sengupta et al. [79]
Patient-related characteristics
ECOG PS
Survival outcome, PFS, RFS
Zisman et al. [18]
Karnofsky PS
Survival outcome, PFS
Heng et al. [13]
Symptoms at presentation (local/systemic)
Survival outcome, RFS
Nutrition status
Survival outcome
Morgan et al. [20]
Disease free interval
Survival outcome
Motzer et al. [48]
Cytoreductive nephrectomy
Survival outcome
Flanigan and Mickisch et al. [39]
Blood markers
Thrombocytosis
Survival outcome
Suppiah et al. [41]
Leukocytosis
Survival outcome, PFS
Hypercalcemia
Survival outcome, PFS
Elevated alkaline phosphatase
Survival outcome, PFS
Negrier et al. [46]
Elevated CRP
Survival outcome, PFS
Karakiewicz et al. [45]
Elevated blood sedimentation rate
Survival outcome
Ljungberg et al. [44]
Elevated lactatdehydrogenase
Survival outcome, PFS
Anemia
Survival outcome
Heng et al. [13]
Clinical Features to Predict Response to Targeted Therapies
In the last 7 years, there has been a fundamental change in the treatment paradigm of mRCC. Therapies targeting key elements of the mTOR and VEGF-pathway have become the international standard of care [51]. Targeted therapies can achieve disease control rates in approximately 80 % of patients. However, primary response and duration of response to targeted therapies are very heterogeneous even among tumors with similar histological subtypes and clinicopathological features [52]. Biomarkers are urgently needed to guide clinicians to choose the most effective drug for each individual patient. This may one day be used to prevent unnecessary toxicities and cost incurred to patients who would not benefit from a drug as predicted by a biomarker, and instead allow the selection of a more efficacious drug with fewer side effects on an individual level. None of the numerous clinical, genetic, tissue and blood markers tested during the past years are ready for routine use in clinical practice. External validation of potential biomarkers is still required. The main focus of biomarker studies are molecular and genetic features which will be discussed in a separate chapter. The following paragraphs will focus on some clinical features that may be of predictive value for treatment response to targeted agents.
An interesting clinical biomarker was described by Rini et al. who demonstrated that sunitinib-induced hypertension is associated with a better objective response, PFS, and OS in mRCC. Patients with versus without hypertension had objective response rates of 54.8 versus 8.7 %, a PFS 12.5 versus 2.5 months, and OS of 30.9 versus 7.2 months [53]. Since the development of hypertension was not associated with more major adverse events than in patients without hypertension, it may be useful to guide treatment selection in targeted therapies.
The objective of other clinical studies was the influence of the body composition on treatment response to targeted therapies. A study of 475 patients demonstrated that a high BMI is correlated with improved survival with targeted agents (HR 0.67; 95 % CI: 0.49–0.91; p = 0.01) [54]. On the contrary, two other studies did not find a similar association of BMI or body surface area (BSA) with favorable response to targeted therapies. While one of the studies demonstrated a positive impact of high visceral fat area (VFA) and elevated superficial fat area (SFA) on survival, the other study found the complete opposite having used the same measurement methods [55, 56]. In summary, the heterogeneity of these results underscores the importance of external validation.
A recent study of Tran et al. used an interesting 3-step approach for screening, confirmation and validation of blood-biomarkers [57]. In their screening study, they found a blood marker panel comprised of six proteins (IL-6, IL-8, VEGF, Osteopontin, E-selectin, HGF), which could predict survival and response to pazopanib. In the phase II and phase III approval trials of pazopanib, the findings from the training set could be externally validated (Table 28.2). Although this study was well designed and provided external validation of preliminary findings, the study did not demonstrate an improvement on existing prognostic models . Once more, the approach of Tran et al. underscores that survival outcome and treatment response are influenced by a variety of clinical and biological processes, and thus prediction models must include a marker panel rather than one single predictive factor.
Table 28.2
Clinical markers for response to targeted therapies prediction
Clinical markers | Predictive end point | Reference |
---|---|---|
BMI, BSA | Response to TT | Choueiri et al. [54] |
Hypertension | Response to sunitinib | Rini et al. [53] |
Blood markers | Predictive end point | Reference |
sVEGF and sVEGFR | OS with bevacizumab, PFS and ORR with sunitinib | |
High IL-6; IL-8, VEGF, osteopontin, E-selectin, HGF | Tumor shrinkage, PFS with pazopanib | Tran et al. [57] |
Signature of VEGF, CA-IX, collagen IV, VEGFR-2, TRAIL | PFS with sorafenib | Zurita et al. [83] |
Integration of Single Factors into Multivariable Prognostic and Predictive Models
During the last decade, several prognostication systems have combined prognostic factors. These models were developed to improve prognostication of recurrence-free survival (RFS) and CSS before or after nephrectomy. While some models were exclusively designed to improve prognostication of patients with localized RCCs, others focused on mRCC or both. There is currently no reliable model that is particularly able to predict treatment response to targeted therapies.
Multivariable Models for Localized and Metastatic RCC
Other models were established for all Union for International Cancer Control (UICC)/American Joint Committee on Cancer (AJCC) stages of patients independent of the RCC subtype. The most studied models are the University of California Los Angeles integrated staging system (UISS) and the Mayo Clinic tumor stage, tumor size, tumor grade, and necrosis (SSIGN) model [18, 58]. Both the UISS [59, 60] and the SSIGN [59] were extensively validated in numerous studies. While the UISS has the advantage that it is simple to use because it integrates standard clinicopathological variables such as the TNM stage , Fuhrman grade, and ECOG PS, the SSIGN model has reached higher accuracies in validation studies [59]. Collectively, both models have proven their applicability in clinical practice and represent useful tools in RCC survival prognostication.
Another model of Karakiewicz et al. has reached concordance indices of 88–89 % in the development and validation cohorts, respectively, and they presented the highest accuracies for postoperative survival prognostication. The model includes TNM stage, Fuhrman grade, tumor size, and symptoms at presentation as individual prognostic factors [61]. The practicability of this model has to be evaluated in clinical practice.
Patients want to be informed about their prognosis before treatment decisions are made. Karackiewicz et al. also developed a model for this purpose [62]. The training cohort and validation were comprised of 2474 and 1972 patients and included metastatic and nonmetastatic patients. It has reached impressive concordance indices of 86.8 % at 5 years, and 84.2 % at 10 years. Thus, this model may present a useful tool to inform patients and their families about their estimated prognosis before invasive treatments are done.
Multivariable Prognostic Models in Localized RCC
Currently, SRM (tumors < 4.0 cm) comprise the majority of renal lesions. It has been recognized that between 20 and 30 % of them are benign tumors. Moreover, approximately 75–80 % of the malignant SRM are indolent and do not behave aggressively in terms of tumor progression and development of metastatic disease [7, 63]. On the other hand, there is a chance that patients having undergone partial or radical nephrectomy will develop chronic kidney disease (CKD) of < 60 mL/min per 1.72 m2 in 20 and 65 % of cases, respectively, after 3 years [32]. Furthermore, renal insufficiency of > 45 mL/min and < 60 mL/min is associated with 20 % increased risk of death [64]. Therefore, it has been questioned if the majority of SRMs is overtreated by surgery, causing harm to patients due to the development of CKD. Therefore, physicians have to face two major problems: first, to prognosticate the chance of having a malignant lesion, and second, to prognosticate the likelihood that localized RCC develops metastatic disease with or without surgery. For these two purposes preoperative and postoperative prognostic models were established.
Preoperative Prognostication of Recurrence, Cancer-Specific Survival and Prediction of Tumor-Biology
In an effort to preoperatively distinguish benign versus malignant and indolent versus potentially aggressive lesions in RCC < 7.0 cm, Lane et al. from the Cleveland clinic constructed two nomograms from 862 patients [63]. They used clinical presentation, age, gender, smoking history, and tumor size as components for these nomograms. They reported concordance indices of 0.64 and 0.56 for malignancy and aggressiveness prediction, respectively. Clearly, the effort to predict these two end points preoperatively has to be acknowledged, but these two nomograms need further improvement, e.g., by adding new radiological markers, biopsy results , and genetic serum markers. Another preclinical model including gender, mode of presentation, necrosis, lymphadenopathy, and tumor size by imaging of Raj et al. from the Cleveland clinic was developed based on data of two high-volume centers. The resulting nomogram reached a concordance index of 80 %. In the same cohort, the TNM classification alone had a concordance index of just 71 % (Fig. 28.1) [65].
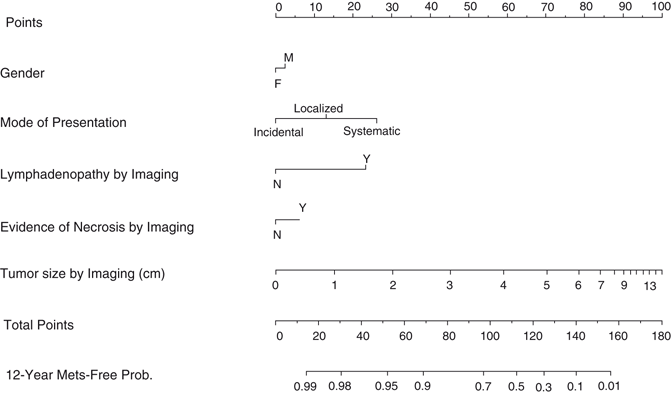
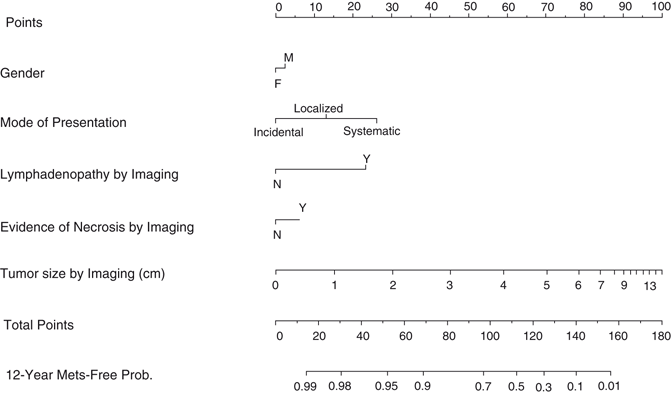
Fig. 28.1
Preoperative prognostic model of Raj et al. [65] predicts the likelihood of postoperative metastatic recurrence. Metastatic recurrence developed in 340/2517 patients with a median follow-up of 4.7 years for patients with recurrence. The nomogram predicts the 12-year probability of metastatic relapse with a concordance index of 0.80 while the TNM classification alone reached only a concordance index of 0.71. (Used with permission from [65])
Two other models from the group of Karackiewicz et al. using preoperative data were developed and validated in order to prognosticate the likelihood of lymph node and distant metastases at nephrectomy [66, 67]. The reported concordance indices were 85.2 % for distant metastases and 78.4 % for lymph node metastases. The only variables in both nomograms were symptoms at diagnosis and tumor size. The prognostication of the likelihood for metastatic disease was based on patient data with synchronous metastases. It is currently uncertain whether tumors presenting with synchronous metastases share the same biological characteristics as tumors developing metastatic disease several years after surgery.
Kutikov et al. from Fox Chase Cancer Center recently reported an interesting comprehensive approach to estimate the risk for kidney cancer death, other cancer death, and noncancer related death under consideration of other mortality risk factors [68]. In a patient cohort of 30,801 patients with localized RCC, the 5-year probability for risk of death from RCC, other cancers, and noncancer mortality were 4, 7, and 11 %, respectively (Fig. 28.2a, b). With regard to AS strategies, the approach of Kutikov et al. is important because it underscores the central role of individualized treatment decisions under consideration of other morbidities, gender, race, and age (Fig. 28.2a, b).
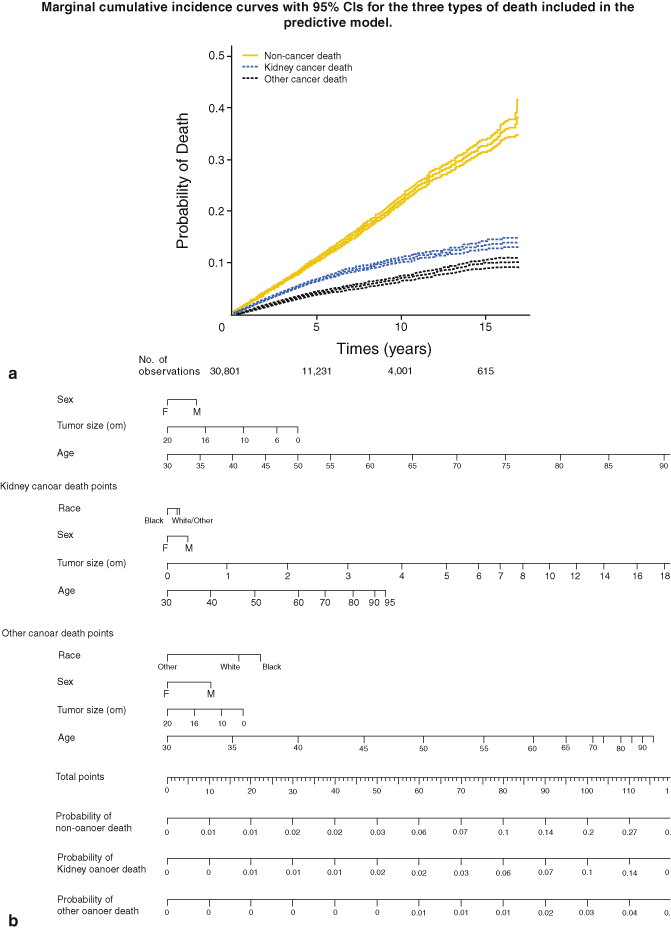
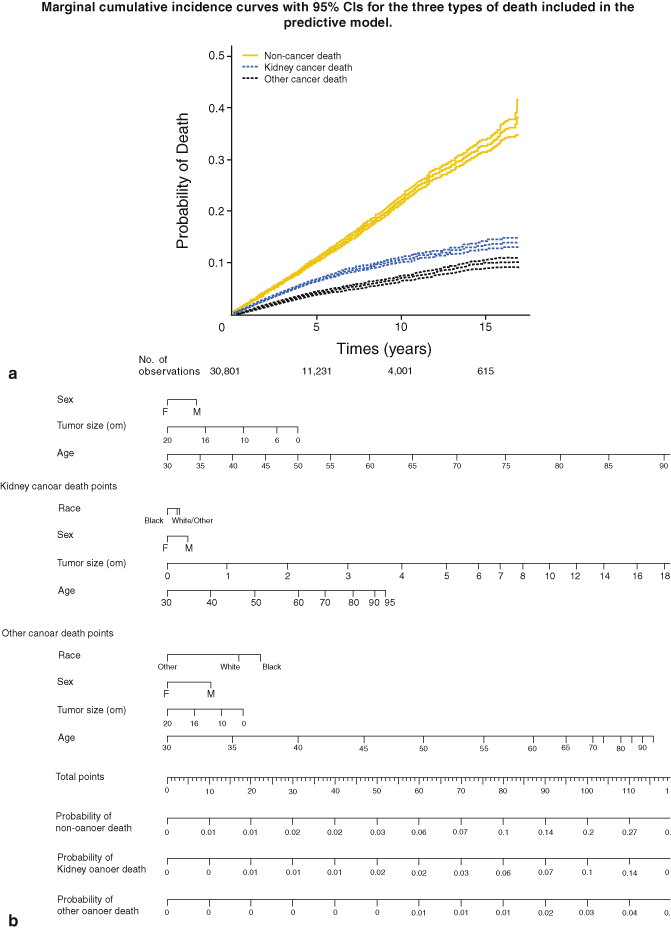
Fig. 28.2
a Cumulative mortality incidence rates and 95 % confidence intervals for noncancer-related, kidney-cancer-related , and other cancer-related death. b Nomogram that prognosticates the 5-year probability according to competing risk criteria. (Used with permission from [68])
All preoperative nomograms currently available were developed based on patient cohorts who were treated by radical or partial nephrectomy. In the future, it will be one major challenge to integrate clinical, histological, molecular, and genetic features of prospectively collected data of patients managed with AS. A comprehensive overview on preoperative prognostic models in localized RCC is given in Table 28.3.
Table 28.3
Prognostic models in localized RCCs
Preoperative models | ||||
---|---|---|---|---|
Patient number(Test set; validation cohort) | Endpoint | Follow-up | Concordance index | Reference(year) |
296 | Recurrence after surgery | 48 months (5–129) | 65.1 % | Yaycioglu (2001) [84] |
660 | Recurrence after surgery | 42 months (2–180) | 67.2 % | Cindolo (2003) [85] |
851 | Malignancy and tumor aggressiveness | 1.4 years | 64.4 % (malignancy); 55.7 % (aggressiveness) | Lane (2007) [63] |
2522; 2136 | Lymph node metastases at surgery | n.r. | 78.8 % | Hutterer (2007) [67] |
2517 | Recurrence after surgery | 4.7 years (patients without evidence of metastatic recurrence) | 80 % | Raj (2008) [65] |
2660; 2716 | Visceral metastases at surgery | n.r. | 85 % | Hutterer (2008) [66] |
30801 | CSS, OC, NCS | 3.8 years (0–203 months) | n.r. | Kutikov (2010) [68] |
Postoperative models | ||||
601 | RFS | 40 months (patients without evidence of metastatic recurrence) | 74.0 % | Kattan (2001) [25] |
1864 | RFS to abdominal, thoracic, and bone metastases | 6.4 years (0.1–31) | 80.5 % (abdominal), 82.6 % (thoracic), 80.0 %(bone) | Frank (2003) [69] |
1671 | RFS | 5.4 years (0–31 years) | 81.9 % | Leibovich (2003) [35] |
701 | RFS | 32 months (patients without evidence of metastatic recurrence) | 82.0 % | Sorbellini (2005) [70] |
559 | RFS solitary, chest, abdominal, bone, brain recurrence | 26 months | n.r. | Lam (2005) [86] |
170 | RFS | 7.1 years (0.1–16.9) | 90.4 % | Klatte (2009) [71] |
Recurrence-Free Survival and Cancer-Specific Survival after Nephrectomy
It is important to individualize follow-up protocols and to assign patients at high risk for disease recurrence to clinical trials for adjuvant therapies after surgical treatment of non-mRCC. Several models address this issue (Table 28.3). Most of them are currently based on clinicopathological features only, without consideration of molecular or genetic markers. The Leibovich prognostic score from the Mayo Clinic is probably the most often applied prognostic model for localized ccRCC [35]. The model separates three groups with high, intermediate, and low risk for metastatic relapse after nephrectomy by integrating tumor stage , regional lymph node status, tumor size , nuclear grade, and histologic tumor necrosis into a prognostic risk score. Another model of the same institution was constructed with 11 clinicopathological characteristics in order to prognosticate recurrence in specific organ sites. The authors reported excellent concordance indices of 80.5, 82.6, and 80.8 % for the prediction of abdominal, thoracic, and bone metastases, respectively [69]. While these are reasonable concordance indices, there is the question about the practicability of this model in clinical practice since so many variables are necessary for prognostication.
A fundamentally simpler nomogram was presented by Sorbellini et al. [70] from the Memorial Sloan Kettering Cancer Center (MSKCC). This nomogram reached a concordance index of 82.0 % and is based on tumor size, T stage, grade, necrosis, MVI, and presentation of symptoms. The sorbellini nomogram was externally validated in opposite to many other nomograms .
In a proof of principle study Klatte et al. presented a nomogram which combines clinicopathological features and histological markers in order to predict RFS in primary localized RCCs (Fig. 28.2a, b) [71]. The nomogram reached an impressive concordance index of 90.4 %. The model included T stage, ECOG PS, and five histological markers (Ki-67, p53, endothelial VEGFR-1, epithelial VEGFR-1, and epithelial VEGF-D). While Ki-67 and expression of p53 are routinely evaluable markers, distinguishing between epithelial and endothelial VEGF and VEGF-R expression may be challenging in real life practice. However, in biomarker research, it is important to rigorously integrate new biomarkers into existing prognostic tools and to demonstrate the advantages of these markers in order to improve the current practice of prognostication [72]. This model has to be externally validated before it can be used in clinical practice.
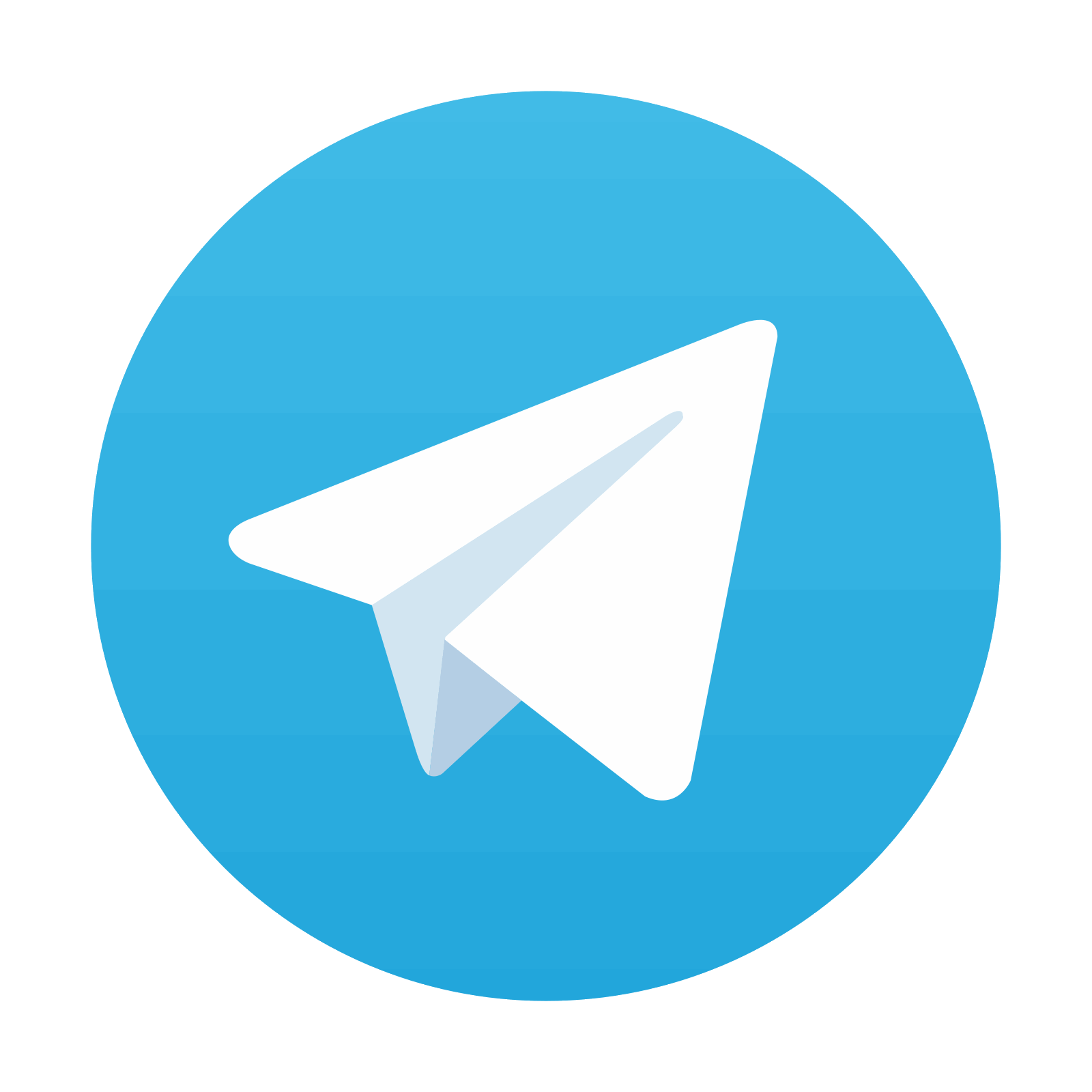
Stay updated, free articles. Join our Telegram channel

Full access? Get Clinical Tree
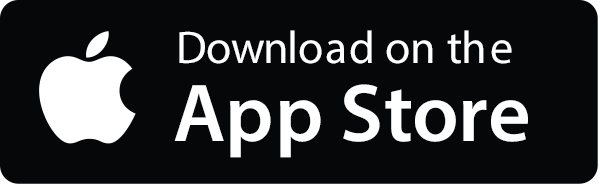
