What is Linear Regression?
To investigate the relationship between two numerical variables, x and y, we measure the values of x and y on each of the n individuals in our sample. We plot the points on a scatter diagram (Chapters 4 and 26), and say that we have a linear relationship if the data approximate a straight line. If we believe y is dependent on x, with a change in y being attributed to a change in x, rather than the other way round, we can determine the linear regression line (the regression of y on x) that best describes the straight line relationship between the two variables. In general, we describe the regression as univariable because we are concerned with only one x variable in the analysis; this contrasts with multivariable regression which involves two or more x’s (see Chapters 29–31).
The Regression Line
The mathematical equation which estimates the simple linear regression line is:
Y = a + bx
- x is called the independent, predictor or explanatory variable;
- for a given value of x, Y is the value of y (called the dependent, outcome or response variable) which lies on the estimated line. It is an estimate of the value we expect for y (i.e. its mean) if we know the value of x, and is called the fitted value of y;
- a is the intercept of the estimated line; it is the value of Y when x = 0 (Fig. 27.1);
- b is the slope or gradient of the estimated line; it represents the amount by which Y increases on average if we increase x by one unit (Fig. 27.1).
Figure 27.1 Estimated linear regression line showing the intercept, a, and the slope, b (the mean increase in Y for a unit increase in x).

a and b are called the regression coefficients of the estimated line, although this term is often reserved only for b. We show how to evaluate these coefficients in Chapter 28. Simple linear regression can be extended to include more than one explanatory variable; in this case, it is known as multivariable or multiple linear regression (Chapter 29).
Method of Least Squares
We perform regression analysis using a sample of observations. a and b are the sample estimates of the true parameters, α and β, which define the linear regression line in the population. a and b are determined by the method of least squares (often called ordinary least squares, OLS) in such a way that the ‘fit’ of the line Y = a + bx to the points in the scatter diagram is optimal. We assess this by considering the residuals (the vertical distance of each point from the line, i.e. residual = observed y − fitted Y (Fig. 27.2). The line of best fit is chosen so that the sum of the squared residuals is a minimum.
Figure 27.2 Estimated linear regression line showing the residual (vertical dotted line) for each point.

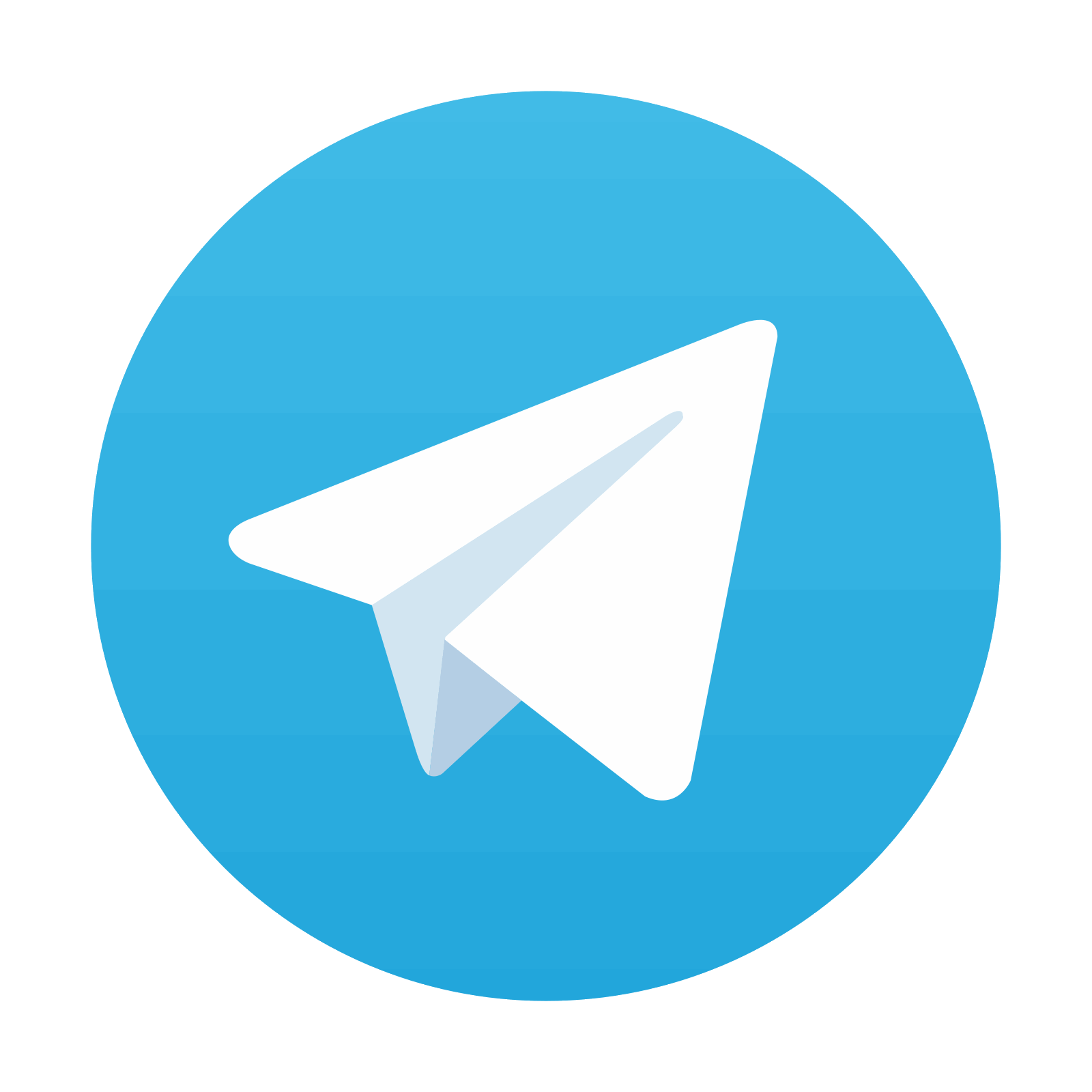
Stay updated, free articles. Join our Telegram channel

Full access? Get Clinical Tree
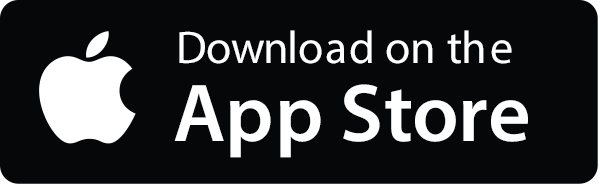
