The Systematic Review
What Is It?
A systematic review1 is a formalized and stringent process of combining the information from all relevant studies (both published and unpublished) of the same health condition; these studies are usually clinical trials (Chapter 14) of the same or similar treatments but may be observational studies (Chapters 15 and 16). A systematic review is an integral part of evidence-based medicine (EBM; Chapter 40) which applies the results of the best available evidence, together with clinical expertise, to the care of patients. So important is its role in EBM that it has become the focus of an international network of clinicians, methodologists and consumers who have formed the Cochrane Collaboration. This has produced the Cochrane Library containing regularly updated evidence-based healthcare databases including the Cochrane Database of Systematic Reviews; full access to these reviews requires subscription but the abstracts are freely available on the internet (www.cochrane.org/reviews).
What Does it Achieve?
- Refinement and reduction – large quantities of information are refined and reduced to a manageable size.
- Efficiency – the systematic review is usually quicker and less costly to perform than a new study. It may prevent others embarking on unnecessary studies, and can shorten the time lag between medical developments and their implementation.
- Generalizability and consistency – results can often be generalized to a wider patient population in a broader setting than would be possible from a single study. Consistencies in the results from different studies can be assessed, and any inconsistencies determined.
- Reliability – the systematic review aims to reduce errors, and so tends to improve the reliability and accuracy of recommendations when compared with haphazard reviews or single studies.
- Power and precision – the quantitative systematic review (see meta-analysis below) has greater power (Chapter 18) to detect effects of interest and provides more precise estimates of them than a single study.
Meta-Analysis
What is It?
A meta-analysis or overview is a particular type of systematic review that focuses on the numerical results. The main aim of a meta-analysis is to combine the results from several independent studies to produce, if appropriate, an estimate of the overall or average effect of interest (e.g. the relative risk, RR; Chapter 15). The direction and magnitude of this average effect, together with a consideration of the associated confidence interval and hypothesis test result, may be used to make decisions about the therapy under investigation, the management of patients and/or the role of the factor of interest, as appropriate.
Statistical Approach
- numerical, the effect may be the difference in treatment means. A zero difference implies no treatment effect;
- binary (e.g. died/survived), we consider the risks, say, of the outcome (e.g. death) in the treatment groups. The effect may be the difference in risks or their ratio, the RR. If the difference in risks equals zero or RR = 1, then there is no treatment effect.
- We can perform a hypothesis test of homogeneity to investigate whether the variation in the individual effects is compatible with chance alone. However, this test has low power (Chapter 18) to detect heterogeneity if there are few studies in the meta-analysis and may, conversely, give a highly significant result if it comprises many large studies, even when the heterogeneity is unlikely to affect the conclusions.
- An index, I2, which does not depend on the number of studies, the type of outcome data or the choice of treatment effect (e.g. RR), can be used to quantify the impact of heterogeneity and assess inconsistency2 (see Example). I2 represents the percentage of the total variation across studies due to heterogeneity; it takes values from 0% to 100%, with a value of 0% indicating no observed heterogeneity. If there is evidence of statistical heterogeneity, we should proceed cautiously, investigate the reasons for its presence and modify our approach accordingly (see Point 3).
- Perform a random effects meta-analysis. This assumes that the separate studies represent a random sample from a population of studies which has a mean treatment effect about which the individual study effects vary. The variance of the average effect of interest incorporates both within- and between-study variability and therefore the standard error of the estimate is greater, the confidence interval for the true average effect wider and its P-value larger (i.e. it is less likely to be statistically significant) than the comparable quantities obtained from a fixed effects meta-analysis.
- Stratify the studies into subgroups of those with similar characteristics and perform a separate (usually fixed effects) meta-analysis in each stratum.
- Perform a meta-regression3 which aims to estimate the effect of interest, after adjusting for differences between studies, and to determine which covariates account for the heterogeneity. The dependent variable is the estimated effect of interest for a study (e.g. the RR) and the explanatory variables are one or more study-level characteristics (e.g. the average age of the population, the average duration of treatment, whether the hospital is in an urban or rural setting). The most usual form of meta-regression is a random effects meta-regression which takes account of the between-study variability by including it as a component of error in the model (this is a form of random effects model – see Chapter 42). Unfortunately, because the ‘sample size’ for the meta-regression is the number of studies (rather than the number of patients in each study), many analyses are insufficiently powered to detect important effects. Furthermore, it may be impossible to separate the effects of different covariates if collinearity is present (Chapter 34), as is often the case, resulting in misleading conclusions. We should also be aware of the ecological fallacy (Chapter 34) which may lead us to believe mistakenly that an association that we observe between variables at an aggregate level reflects the corresponding association at an individual level in the same population.
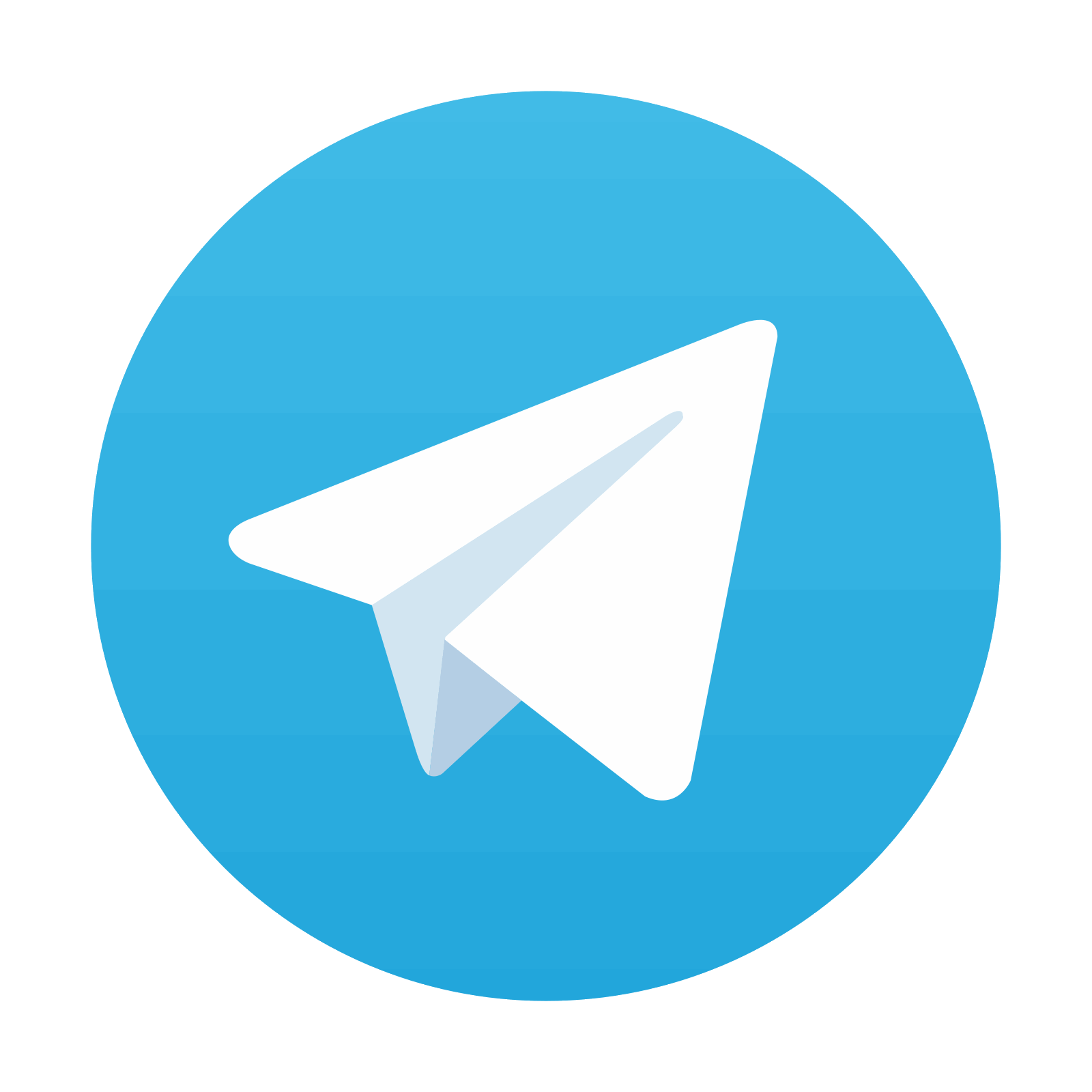
Stay updated, free articles. Join our Telegram channel

Full access? Get Clinical Tree
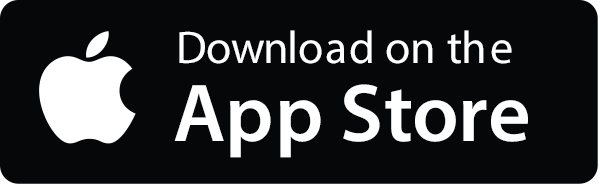
