Fig. 15.1
Forest plot depicting pooled random effects meta-analysis and subgroup estimates according to dual versus single ring structure of wound protector
For RCTs, absolute measures, such as the risk difference (RD), also called the absolute risk reduction, (ARR) and number of patients needed to treat (NNT) can be calculated. The RD is defined as the risk in the treatment group minus the risk in the control group, which quantifies the absolute change in risk due to the treatment. In general, a negative risk difference favors the treatment group. The NNT is defined as the inverse of the risk difference and is the number of patients that need to be treated with the intervention to prevent one event. In the case where the risk difference is positive (does not favor the treatment group), the inverse will provide the number needed to harm (NNH) [2].
For example, a meta-analysis comparing stent treatments of infragenicular vessels in for chronic lower limb ischemia found that primary patency was significantly higher with the drug eluting stent compared to patients treated with bare metal stent (OR 4.51, 95 % CI 2.90 to 7.02, and NNT 3.5) [15]. Drug eluting stent increased the odds of vessel patency 4.5 fold higher than the metal stent and 3.5 patients had to receive a drug eluting stent to prevent loss of patency in one patient in the control arm.
The meta-analysis technique for combining data also utilizes a weighted average of the results. Larger trials are given more weight since the results of smaller trials are more likely to be affected by chance [4, 8]. Either the fixed-effects or random-effects model can be used for determining the overall effect. The fixed-effects model assumes that all studies are estimating the same common treatment effect; therefore if each study were infinitely large an identical treatment effect could be calculated. The random-effects model assumes that each study is estimating a different treatment effect and hence yields wider confidence intervals (CI). A meta-analysis should be analyzed using both models. If there is not a difference between the models, then the studies are unlikely to have significant statistical heterogeneity. If there is a considerable difference between the two models, then the most conservative estimate should be reported, which is usually the random effects model [2].
There are additional types of meta-analysis. One approach is to run the analysis based on individual patient data. While this method requires a greater amount of resources, it lessens the degree of publication and selection bias, thus potentially resulting in more accurate results. Another example is the cumulative meta-analysis, which involves repeating the meta-analysis as new study findings become available and allows for the accrual of data over time [5]. It can also retrospectively pinpoint the time when a treatment effect achieved statistical significance.
Meta-analysis can be performed using both observational or RCT data. Ideally, limiting the meta-analysis to only RCT data will produce results with a higher level of scientific evidence. Randomized data will be less likely to have significant selection bias or other confounding factors. Pooling non-randomized data has many limitations that must be considered in the final assessment of the results. Furthermore, a general rule of thumb is that observational data should not be combined with randomized data within an analysis.
While in general a meta-analysis produces an overall conclusion with more power than looking at the individual studies, results must be interpreted with consideration of the study question, selection criteria, method of data collection, and statistical analysis [4].
The main limitation of a meta-analysis is the potential for multiple types of bias. Pooling data from different sources unavoidably includes biases of the individual studies [16, 17]. Moreover, despite the establishment of study selection criteria, authors may tend to incorporate studies that support their view, leading to selection bias [3, 4, 16–19]. There is also potential for bias in identification of studies because they are often selected by investigators familiar with field who have individual opinions [16]. Language bias may exist when literature searches failure to include foreign studies, because significant results are more likely to be published in English [4, 16]. Studies with significant findings tend to be cited and published more frequently, and those with negative or non-significant findings are less likely to be published, resulting in possible citation bias and publication bias [4, 16]. Since studies with significant results are more likely to be indexed in the literature database, database bias is another concern [4, 16]. Studies which have not been published in traditional journals, like a dissertation or a chapter, are referred to as “fugitive” literature and are less likely to be identified through the traditional database search. Finally, multiple publication bias can occur if several publications are generated from a multi-center trial or a large trial reporting on a variety of outcomes. If the same set of patients is included twice in the meta-analysis, the treatment effect can be overestimated [16]. These potent bias factors can affect the conclusions and must be considered during interpretation of the results.
To combat these sources of bias, several tools are available. First, a sensitivity analysis can help examine for bias by exploring the robustness of the findings under different assumptions [16]. Exclusion of studies based on specified criteria (e.g. low quality, small sample size, or studies stopped early due to an interim analysis) should not significantly change the overall effect if the results of the meta-analysis are not significantly influenced by these studies. Second, the degree of study heterogeneity is another major limitation and the random-effects model should be used when appropriate [2].
A third approach to measure potential bias is the funnel plot, which is a scatter plot illustrating each study’s effect with reference to their sample size. The underlying principle is that as the sample size of individual studies increases, the precision of the overall estimate or effect difference improves. This is shown graphically as smaller studies would distribute widely while the spread of large studies should be narrow. The plot should show a symmetrical inverted funnel if there is minimal or no bias, as demonstrated in Fig. 15.2. By the same logic, the plot would be asymmetrical and skewed when bias exists.


Fig. 15.2
Illustration of a funnel plot for a hypothetical meta-analysis comparing hernia recurrence incidence following Procedure X versus Procedure Y. The y-axis reflects individual study weights as the log of the effect estimate, SE (log OR). The x-axis represents the odds ratio (OR) for each study. The symmetry of the plot distribution suggests absence of publication bias
One standardized method of assessing and reporting the potential for bias is the Cochrane Risk of Bias [20]. RCTs included in a meta-analysis are evaluated for seven potential biases: random sequence generation, allocation assignment, blinding of participants and personnel, blinding of outcome assessment, incomplete outcome data, selective reporting and other bias. Each item is scored on a scale of high, indeterminate, or low bias. Reporting this potential for bias helps the reader assess the overall level of bias for the selected studies of interest (Fig. 15.3).


Fig. 15.3
Cochrane risk of bias for hypothetical bariatric surgery randomized controlled trials. Two trials are depicted with ratings of high, indeterminate, or low for each of the seven bias categories
Other areas of criticism involve the interpretation of meta-analysis results. One potential problem can occur when a meta-analyst neglects to consider important covariates, which could lead to misinterpretation of the results [18, 19]. For example, in a study involving cerebrospinal fluid drainage (CSFD) in thoracic and thoracoabdominal aortic surgical repair, the expertise of the surgical team varies among the included studies and could play a critical factor in the outcomes of interest – prevention of paraplegia [21]. Some argue that the inherent degree of study heterogeneity does not permit the pooling of data to produce a valid conclusion [16, 17]. Also, the strength and precision of a meta-analysis is in question when the results contradicts a large, well-performed RCT [16, 17]. As such, results of any individual study or trial may be overlooked in place of the pooled results. However, it is arguable that findings falling outside the group mean are likely a product of chance and may not reflect the true effect difference, which provides the rationale for formally pooling similar studies. Even if a real difference exists in an individual trial, the results of the group will likely be the best overall estimate (also known as Stein’s Paradox) [22].
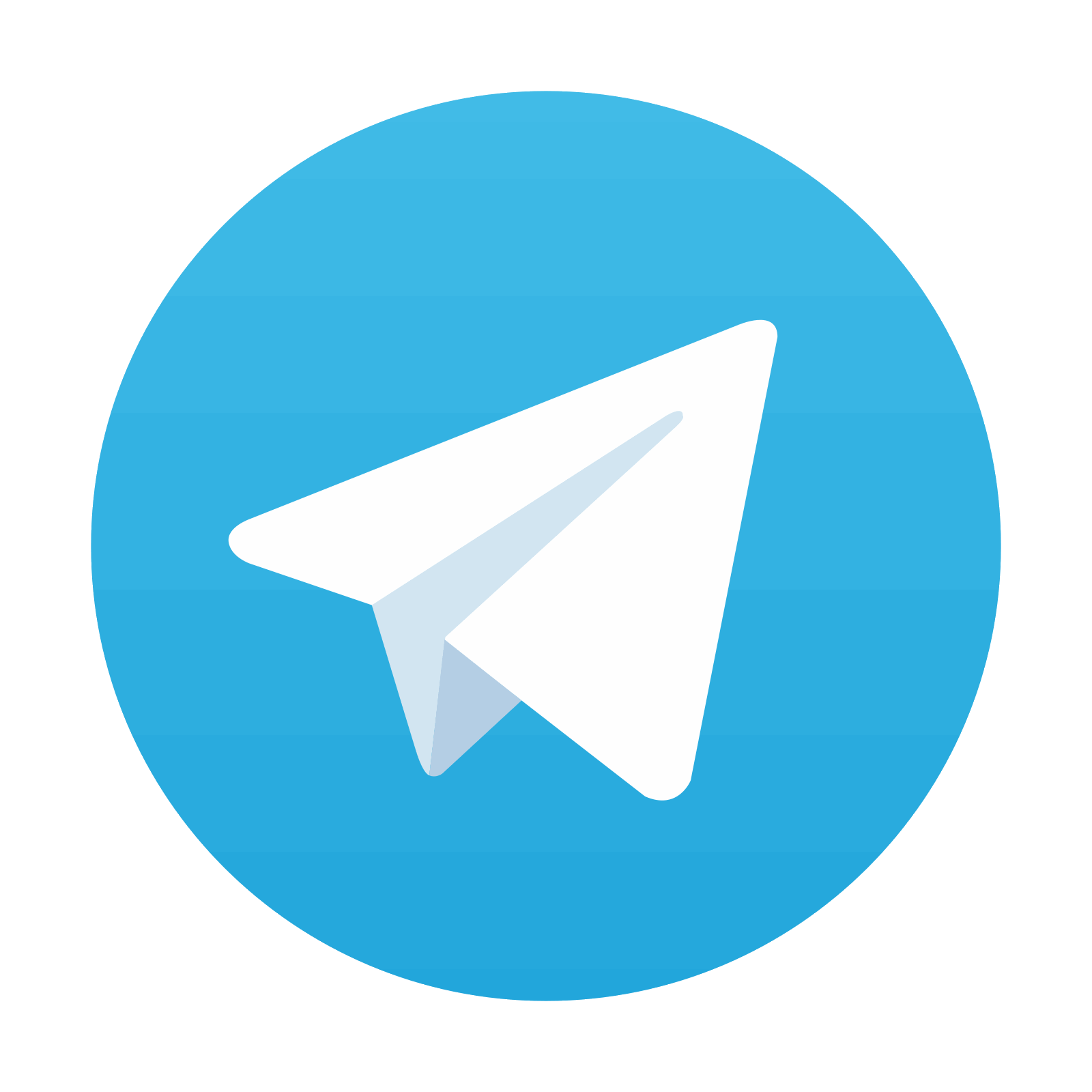
Stay updated, free articles. Join our Telegram channel

Full access? Get Clinical Tree
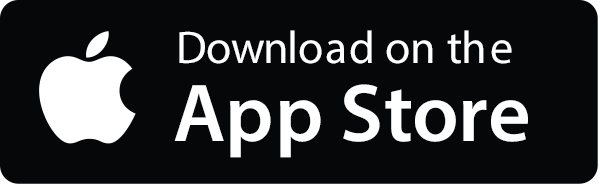
