8 Statistical Foundations of Clinical Decisions
Access the most relevant research data.
Decide which studies are most trustworthy and applicable to the clinical question under consideration.
Use appropriate methods available to determine the best diagnostic and therapeutic approaches to each problem.
There is no controversy about the need to improve clinical decision making and maximize the quality of care. Opinions do differ, however regarding the extent to which the tools discussed in this chapter are likely to help in actual clinical decision making. Some individuals and medical centers already use these methods to guide the care of individual patients. Others acknowledge that the tools can help to formulate policy and analyze the cost-effectiveness of medical interventions, such as immunizations,1,2 but they may not use the techniques for making decisions about individual patients. Even when the most highly regarded means are used to procure evidence, such as double-blind, placebo-controlled clinical trials, the applicability of that evidence to an individual patient is uncertain and a matter of judgment.
I Bayes Theorem
Although it is useful to know the sensitivity and specificity of a test, when a clinician decides to use a certain test on a patient, the following two clinical questions require answers (see Chapter 7):
If the test results are positive, what is the probability that the patient has the disease?
If the test results are negative, what is the probability that the patient does not have the disease?
Many clinicians, even those who understand sensitivity, specificity, and predictive values, throw in the towel when it comes to Bayes theorem. A close look at the previous equation reveals, however, that Bayes theorem is merely the formula for the positive predictive value (PPV), a value discussed in Chapter 7 and illustrated there in a standard 2 × 2 table (see Table 7-1).
The numerator of Bayes theorem merely describes cell a (the true-positive results) in Table 7-1. The probability of being in cell a is equal to the prevalence times the sensitivity, where p(D+) is the prevalence (expressed as the probability of being in the diseased column) and where p(T+ | D+) is the sensitivity (the probability of being in the top, test-positive, row, given the fact of being in the diseased column). The denominator of Bayes theorem consists of two terms, the first of which describes cell a (the true-positive results), and the second of which describes cell b (the false-positive results) in Table 7-1. In the second term of the denominator, the probability of the false-positive error rate, or p(T+ | D−), is multiplied by the prevalence of nondiseased persons, or p(D−). As outlined in Chapter 7, the true-positive results (a) divided by the true-positive plus false-positive results (a + b) gives a/(a + b), which is the positive predictive value.
A Community Screening Programs
In a population with a low prevalence of a particular disease, most of the positive results in a screening program for the disease likely would be falsely positive (see Chapter 7). Although this fact does not automatically invalidate a screening program, it raises some concerns about cost-effectiveness, which can be explored using Bayes theorem.
A program employing the tuberculin tine test to screen children for tuberculosis (TB) is discussed as an example (based on actual experience).3 This test uses small amounts of tuberculin antigen on the tips of tiny prongs called tines. The tines pierce the skin on the forearm and leave some antigen behind. The skin is examined 48 hours later, and the presence of an inflammatory reaction in the area where the tines entered is considered a positive result. If the sensitivity and specificity of the test and the prevalence of TB in the community are known, Bayes theorem can be used to predict what proportion of the children with positive test results will have true-positive results (i.e., will actually be infected with Mycobacterium tuberculosis).
Box 8-1 shows how the calculations are made. Suppose a test has a sensitivity of 96% and a specificity of 94%. If the prevalence of TB in the community is 1%, only 13.9% of those with a positive test result would be likely to be infected with TB. Clinicians involved in community health programs can quickly develop a table that lists different levels of test sensitivity, test specificity, and disease prevalence that shows how these levels affect the proportion of positive results that are likely to be true-positive results. Although this calculation is fairly straightforward and extremely useful, it is not used often in the early stages of planning for screening programs. Before a new test is used, particularly for screening a large population, it is best to apply the test’s sensitivity and specificity to the anticipated prevalence of the condition in the population. This helps avoid awkward surprises and is useful in the planning of appropriate follow-up for test-positive individuals. If the primary concern is simply to determine the overall performance of a test, however, likelihood ratios, which are independent of prevalence, are recommended (see Chapter 7).
Box 8-1 Use of Bayes Theorem or 2 × 2 Table to Determine Positive Predictive Value of Hypothetical Tuberculin Screening Program
Part 1 Beginning Data
Sensitivity of tuberculin tine test | = 96% | = 0.96 |
False-negative error rate of test | = 4% | = 0.04 |
Specificity of test | = 94% | = 0.94 |
False-positive error rate of test | = 6% | = 0.06 |
Prevalence of tuberculosis in community | = 1% | = 0.01 |
Data from Jekel JF, Greenberg RA, Drake BM: Influence of the prevalence of infection on tuberculin skin testing programs. Public Health Reports 84:883–886, 1969.
B Individual Patient Care
Suppose a clinician is uncertain about a patient’s diagnosis, obtains a test result for a certain disease, and the test is positive. Even if the clinician knows the sensitivity and specificity of the test, this does not solve the problem, because to calculate the positive predictive value, whether using Bayes theorem or a 2 × 2 table (e.g., Table 7-1), it is necessary to know the prevalence of the disease. In a clinical setting, the prevalence can be considered the expected prevalence in the population of which the patient is part. The actual prevalence is usually unknown, but often a reasonable estimate can be made.
Under the circumstances, Bayes theorem could be used to help interpret the positive test. A second estimate of disease probability in this patient could be calculated. It is called the posterior probability, reflecting that it is made after the test results are known. Calculation of the posterior probability is based on the sensitivity and specificity of the test that was performed and on the prior probability of disease before the test was performed, which in this case was 2%. Suppose the serum calcium test had 90% sensitivity and 95% specificity (which implies it had a false-positive error rate of 5%; specificity + false-positive error rate = 100%). When this information is used in the Bayes equation, as shown in Box 8-2, the result is a posterior probability of 27%. This means that the patient is now in a group of patients with a substantial possibility, but still far from certainty, of parathyroid disease. In Box 8-2, the result is the same (i.e., 27%) when a 2 × 2 table is used. This is true because, as discussed previously, the probability based on the Bayes theorem is identical to the positive predictive value.
Box 8-2 Use of Bayes Theorem or 2 × 2 Table to Determine Posterior Probability and Positive Predictive Value in Clinical Setting (Hypothetical Data)
Part 1 Beginning Data (Before Performing First Test)
Sensitivity of first test | = 90% | = 0.90 |
Specificity of first test | = 95% | = 0.95 |
Prior probability of disease | = 2% | = 0.02 |
In light of the 27% posterior probability, the clinician decides to order a serum parathyroid hormone concentration test with simultaneous measurement of serum calcium, even though this test is expensive. If the parathyroid hormone test had a sensitivity of 95% and a specificity of 98%, and the results turned out to be positive, the Bayes theorem could be used again to calculate the probability of parathyroid disease in this patient. This time, however, the posterior probability for the first test (27%) would be used as the prior probability for the second test. The result of the calculation, as shown in Box 8-3, is a new probability of 94%. The patient likely does have hyperparathyroidism, although lack of true, numerical certainty even at this stage is noteworthy.
Box 8-3 Use of Bayes Theorem or 2 × 2 Table to Determine Second Posterior Probability and Second Positive Predictive Value in Clinical Setting
Part 1 Beginning Data (Before Performing the Second Test)
Sensitivity of second test | = 95% | = 0.95 |
Specificity of second test | = 98% | = 0.98 |
Prior probability of disease (see Box 8-2) | = 27% | = 0.27 |
C Influence of the Sequence of Testing
With an increasing number of diagnostic tests available in clinical medicine, the clinician now needs to consider whether to do many tests simultaneously or to do them sequentially. As outlined in Chapter 7, tests used to “rule out” a diagnosis should have a high degree of sensitivity, whereas tests used to “rule in” a diagnosis should have a high degree of specificity (see Box 7-1). The sequential approach is best done as follows:
1. Starting with the most sensitive test.
2. Continuing with increasingly specific tests if the previous test yields positive results.
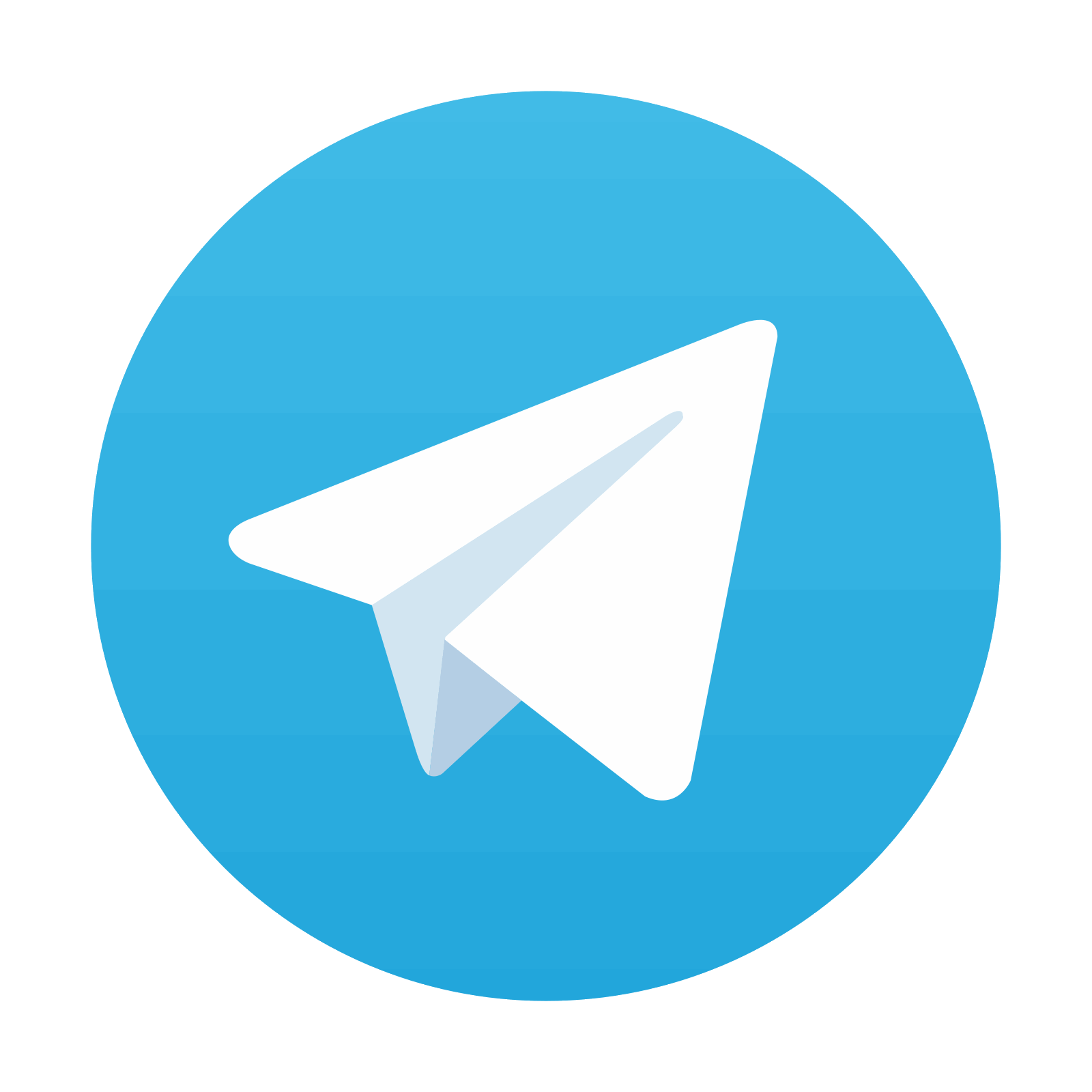
Stay updated, free articles. Join our Telegram channel

Full access? Get Clinical Tree
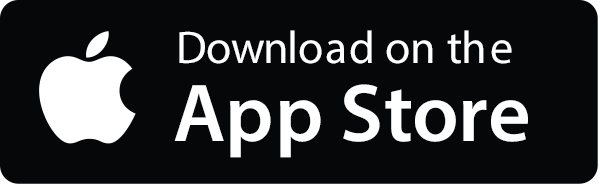
