Introduction
An essential facet of statistics is the ability to summarize the important features of the analysis. We must know what to include and how to display our results in a manner that enables others to obtain relevant and important information easily and draw correct conclusions. This chapter describes the key features of presentation.
Numerical Results
- Give figures only to the degree of accuracy that is appropriate (as a guideline, one significant figure more than the raw data). If analysing the data by hand, only round up or down at the end of the calculations.
- Give the number of items on which any summary measure (e.g. a percentage) is based.
- Describe any outliers and explain how they are handled (Chapter 3).
- Include the units of measurement.
- When interest is focused on a parameter (e.g. the mean, regression coefficient), always indicate the precision of its estimate. We recommend using a confidence interval for this but the standard error is also acceptable. Avoid using the ± symbol, as in mean ± SEM (Chapter 10), because by adding and subtracting the SEM, we create a 67% confidence interval that can be misleading for those used to 95% confidence intervals. It is better to show the standard error in brackets after the parameter estimate, e.g. mean = 16.6 g (SEM 0.5 g).
- When interest is focused on the distribution of observations, always indicate a measure of the ‘spread’ of the data. The range of values that excludes outliers (typically, the range of values containing the central 95% of the observations (Chapter 6)) is a useful descriptor. If the data are Normally distributed, this range is approximated by the sample mean ±1.96 × standard deviation (Chapter 7). The mean and the standard deviation can be quoted instead, e.g. mean = 35.9 mm (SD 2.8 mm), but this leaves the reader to evaluate the range.
Tables
- Do not give too much information in a table.
- Include a concise, informative and unambiguous title.
- Label each row and column.
- Remember that it is easier to scan information down columns rather than across rows.
Diagrams
- Keep a diagram simple and avoid unnecessary frills (e.g. making a pie chart three-dimensional).
- Include a concise, informative and unambiguous title.
- Label all axes, segments and bars, and explain the meaning of symbols.
- Avoid distorting results by exaggerating the scale on an axis.
- Indicate where two or more observations lie in the same position on a scatter diagram, e.g. by using a different symbol.
- Ensure that all the relevant information is contained in the diagram (e.g. link paired observations).
Presenting Results in a Paper
When presenting results in a paper, we should ensure that the paper contains enough information for the reader to understand what has been done. He or she should be able to reproduce the results, given the appropriate computer package and data. All aspects of the design of the study and the statistical methodology must be fully described. Guidelines for the presentation of study results are now available for many types of study design, including randomized trials (the CONSORT Statement, see also Chapter 14), observational studies (the STROBE Statement) and meta-analyses (the QUOROM Statement). In 2008, the EQUATOR (Enhancing the Quality and Transparency of Health Research) Network was initiated with the objectives of providing resources and training for the reporting of health research as well as assistance in the development, dissemination, and implementation of reporting guidelines. Its website (www.equator-network.org) provides links to all relevant guidelines.
Results of a Hypothesis Test
- Include a relevant diagram, if appropriate.
- Indicate the hypotheses of interest.
- Name the test and state whether it is one- or two-tailed.
- Justify the assumptions (if any) underlying the test (e.g. Normality, constant variance (Chapter 35)), and describe any transformations (Chapter 9) required to meet these assumptions (e.g. taking logarithms).
- Specify the observed value of the test statistic, its distribution (and degrees of freedom, if relevant) and, if possible, the exact P-value (e.g. P = 0.03) rather than an interval estimate of it (e.g. 0.01 < P < 0.05) or a star system (e.g. *, **, *** for increasing levels of significance). Avoid writing ‘n.s.’ when P > 0.05; an exact P-value is preferable even when the result is non-significant.
- Include an estimate of the relevant effect of interest (e.g. the difference in means for the two-sample t-test, or the mean difference for the paired t-test) with a confidence interval (preferably) or standard error.
- Draw conclusions from the results (e.g. reject the null hypothesis), interpret any confidence intervals and explain their implications.
Results of a Regression Analysis
Here we include simple (Chapters 27 and 28) and multiple linear regression (Chapter 29), logistic regression (Chapter 30), Poisson regression (Chapter 31), proportional hazards regression (Chapter 44) and regression methods for clustered data (Chapter 42). Full details of these analyses are explained in the associated chapters.
- Include relevant diagrams (e.g. a scatter plot with the fitted line for simple regression).
- Clearly state which is the dependent variable and which is (are) the explanatory variable(s).
- Justify underlying assumptions and explain the results of regression diagnostics, if appropriate.
- Describe any transformations, and explain their purpose.
- Where appropriate, describe the possible numerical values taken by any categorical variable (e.g. male = 0, female = 1), how dummy variables were created (Chapter 29), and the units of numerical variables.
- Give an indication of the goodness of fit of the model (e.g. quote R2 (Chapter 29) or LRS (Chapter 32)).
- If appropriate (e.g. in multiple regression), give the results of the overall F-test from the ANOVA table.
- Provide estimates of all the coefficients in the model (including those which are not significant, if applicable) together with the confidence intervals for the coefficients or standard errors of their estimates. In logistic regression (Chapter 30), Poisson regression (Chapter 31) and proportional hazards regression (Chapter 44), convert the coefficients to estimated odds ratios, relative rates or relative hazards (with confidence intervals). Interpret the relevant coefficients.
- Show the results of the hypothesis tests on the coefficients (i.e. include the test statistics and the P-values). Draw appropriate conclusions from these tests.
Complex Analyses
There are no simple rules for the presentation of the more complex forms of statistical analysis. Be sure to describe the design of the study fully (e.g. the factors in the analysis of variance and whether there is a hierarchical arrangement), and include a validation of underlying assumptions, relevant descriptive statistics (with confidence intervals), test statistics and P-values. A brief description of what the analysis is doing helps the uninitiated; this should be accompanied by a reference for further details. Specify which computer package has been used.
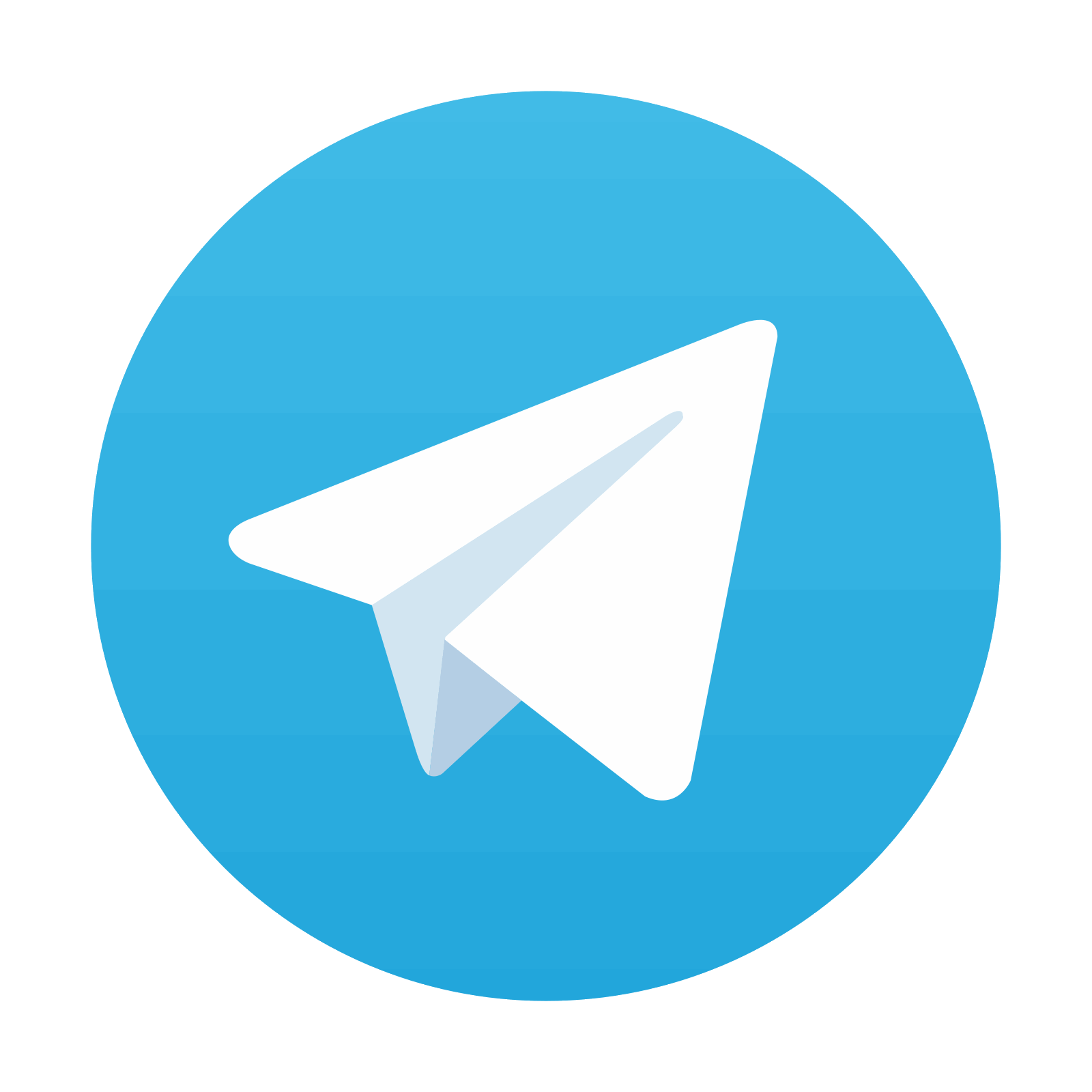
Stay updated, free articles. Join our Telegram channel

Full access? Get Clinical Tree
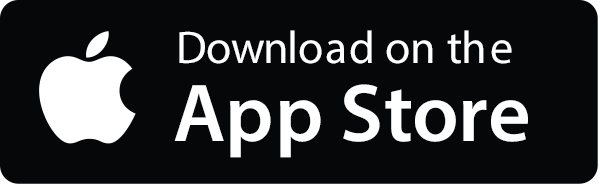
