The focus of previous chapters has been on diseases that are caused primarily by mutations in single genes or by abnormalities of single chromosomes. Much progress has been made in identifying specific mutations that cause these diseases, leading to better risk estimates and in some cases more effective treatment. However, these conditions form only a small portion of the total burden of human genetic disease. A much larger component of our disease burden is composed of congenital malformations and common adult diseases, such as cancer, heart disease, and diabetes. Although they are usually not the result of single-gene mutations or chromosome abnormalities, these diseases have significant genetic components. They are the result of a complex interplay of multiple genetic and environmental factors. Because of the significance of common diseases in health care, it is vital to understand the ways in which genes contribute to causing them.
Principles of Multifactorial Inheritance
The Multifactorial Model
Traits in which variation is thought to be caused by the combined effects of multiple genes are called polygenic (“many genes”). When environmental factors are also believed to cause variation in the trait, which is usually the case, the term multifactorial is used. Many quantitative traits (those such as blood pressure that are measured on a continuous numerical scale) are multifactorial. Because they are caused by the additive effects of many genetic and environmental factors, these traits tend to follow a normal, or bell-shaped, distribution in populations.
Let us use an example to illustrate this concept. To begin with the simplest case, suppose (unrealistically) that height is determined by a single gene with two alleles, A and a. Allele A tends to make people tall, and allele a tends to make them short. If there is no dominance at this locus, then the three possible genotypes, AA, Aa, and aa, will produce three phenotypes: tall, intermediate, and short. Assume that the allele frequencies of A and a are each 0.50. If we assemble a population of individuals, we will observe the height distribution depicted in Fig. 12.1A .

Now suppose, a bit more realistically, that height is determined by two loci instead of one. The second locus also has two alleles, B (tall) and b (short), and they affect height in exactly the same way as alleles A and a do. There are now nine possible genotypes in our population: aabb, aaBb, aaBB, Aabb, AaBb, AaBB, AAbb, AABb, and AABB. Because an individual might have zero, one, two, three, or four “tall” alleles, there are now five distinct phenotypes ( Fig. 12.1B ). Although the height distribution in our population is not yet normal, it approaches a normal distribution more closely than in the single-gene case.
We now extend our example so that many genes and environmental factors influence height, each having a small effect. Then there are many possible phenotypes, each differing slightly, and the height distribution approaches the bell-shaped curve shown in Fig. 12.1C . Genome-wide association studies (GWAS, see Chapter 8 ) have identified more than 700 variants in more than 400 loci associated with human height, affirming that this is indeed a polygenic, multifactorial trait. The loci underlying variation in a quantitative trait such as height are termed quantitative trait loci (QTL).
It should be emphasized that the individual genes underlying a multifactorial trait such as height follow the Mendelian principles of segregation and independent assortment, just like any other genes. The only difference is that many of them act together to influence the trait.
Blood pressure is another example of a multifactorial trait. There is a correlation between parents’ blood pressures (systolic and diastolic) and those of their children, and this correlation is due in part to genes. But blood pressure is also influenced by environmental factors, such as diet and stress. One of the goals of genetic research is identification of the genes responsible for multifactorial traits such as blood pressure and of the interactions of those genes with environmental factors.
Many traits are thought to be influenced by multiple genes as well as by environmental factors. These traits are said to be multifactorial. When they can be measured on a continuous scale, they often follow a normal distribution.
The Threshold Model
A number of diseases do not follow the bell-shaped distribution. Instead, they appear to be either present or absent in individuals. Yet they do not follow the patterns expected of single-gene diseases. A commonly used explanation is that there is an underlying liability distribution for these diseases in a population ( Fig. 12.2 ). Persons who are on the low end of the distribution have little chance of developing the disease in question (i.e., they have few of the alleles or environmental factors that would cause the disease). Those who are closer to the high end of the distribution have more of the disease-causing alleles and environmental factors and are more likely to develop the disease. For multifactorial diseases that are either present or absent, a threshold of liability must be crossed before the disease is expressed. Below the threshold, the person appears unaffected; above it, they are affected by the disease.

A disease that is thought to correspond to this threshold model is pyloric stenosis, a disorder that manifests shortly after birth and is caused by a narrowing or obstruction of the pylorus, the area between the stomach and intestine. Chronic vomiting, constipation, weight loss, and electrolyte imbalance result from the condition, which can be corrected by surgery or sometimes resolves spontaneously. The prevalence of pyloric stenosis among whites is about 3/1000 live births. It is much more common in males than in females, affecting 1/200 males and 1/1000 females. It is thought that this difference in prevalence reflects two thresholds in the liability distribution; a lower one in males and a higher one in females (see Fig. 12.2 ). A lower male threshold implies that fewer disease-causing factors are required to generate the disorder in males.
The liability threshold concept can explain the pattern of sibling recurrence risks for pyloric stenosis, shown in Table 12.1 . Notice that males, having a lower threshold, always have a higher risk than females. However, the recurrence risk also depends on the sex of the proband. It is higher when the proband is female than when the proband is male. This reflects the concept that females, having a higher liability threshold, must be exposed to more disease-causing factors than males in order to develop the disease. Thus a family with an affected female must have more genetic and environmental risk factors, producing a higher recurrence risk for pyloric stenosis in future offspring. In such a situation, we would expect that the highest risk category would be male relatives of female probands; Table 12.1 shows that this is indeed the case.
Relatives | Male Probands | Female Probands | ||
---|---|---|---|---|
London | Belfast | London | Belfast | |
Brothers | 3.8 | 9.6 | 9.2 | 12.5 |
Sisters | 2.7 | 3.0 | 3.8 | 3.8 |
∗ Note that the risks differ somewhat between the two populations.
A number of other congenital malformations are thought to correspond to this model. They include isolated ∗
∗ In this context, the term “isolated” means that this is the only observed disease feature (i.e., the feature is not part of a larger constellation of findings, as in cleft lip/palate secondary to trisomy 13).
cleft lip and/or cleft palate, neural tube defects (anencephaly and spina bifida), club foot (talipes), and some forms of congenital heart disease.The threshold model applies to many multifactorial diseases. It assumes that there is an underlying liability distribution in a population and that a threshold on this distribution must be passed before a disease is expressed.
Recurrence Risks and Transmission Patterns
Whereas recurrence risks can be given with confidence for single-gene diseases (50% for a completely penetrant autosomal dominant disease, 25% for autosomal recessive diseases, and so on), risk estimation is more complex for multifactorial diseases. This is because the number of genes contributing to the disease is usually not known, the precise allelic constitution of the parents is not known, and the extent of environmental effects can vary substantially. For most multifactorial diseases, empirical risks (i.e., risks based on direct observation of data) have been derived. To estimate empirical risks, a large series of families is examined in which one child (the proband) has developed the disease. The relatives of each proband are surveyed in order to calculate the percentage who have also developed the disease. For example, in North America neural tube defects are seen in about 2% to 3% of the siblings of probands with this condition ( Clinical Commentary 12.1 ). Thus the recurrence risk for parents who have had one child with a neural tube defect is 2% to 3%. For conditions that are not lethal or severely debilitating, such as cleft lip and cleft palate, recurrence risks can also be estimated for the offspring of affected parents. Because risk factors vary among diseases, empirical recurrence risks are specific for each multifactorial disease.
Neural tube defects (NTDs) include anencephaly, spina bifida, and encephalocele, as well as several other less-common forms ( Fig. 12.3 ). They are one of the most important classes of birth defects, with a newborn prevalence of approximately 1 per 1000. There is considerable variation in the prevalence of NTDs among populations, and the prevalence of NTDs has been decreasing in many parts of the United States and Europe during the past three decades.
Normally the neural tube closes at about the fourth week of gestation. A defect in closure or a subsequent reopening of the neural tube results in an NTD. Spina bifida is the most commonly observed NTD and consists of a protrusion of spinal tissue through the vertebral column (the tissue usually includes meninges, spinal cord, and nerve roots). About 75% of spina bifida patients have secondary hydrocephalus, which sometimes in turn produces intellectual disability. Paralysis or muscle weakness, lack of sphincter control, and club feet are often observed. Due to improved surgical and medical care, survival rates for spina bifida patients have improved dramatically over the past several decades with approximately 80% of patients surviving to age 17.
Anencephaly is characterized by partial or complete absence of the cranial vault and calvarium and partial or complete absence of the cerebral hemispheres. At least two thirds of anencephalic fetuses are stillborn; term deliveries do not survive more than a few hours or days. Encephalocele consists of a protrusion of the brain into an enclosed sac. It is seldom compatible with survival.
NTDs are thought to arise from a combination of genetic and environmental factors, with an estimated heritability of approximately 70%. In most populations surveyed thus far, empirical recurrence risks for siblings of affected individuals range from 2% to 5%. Consistent with a multifactorial model, the recurrence risk increases with additional affected siblings. A Hungarian study showed that the overall prevalence of NTDs in that country was 1/300 births and that the sibling recurrence risks were 3%, 12%, and 25% after one, two, and three affected offspring, respectively. Recurrence risks tend to be slightly lower in populations with lower NTD prevalence rates, as predicted by the multifactorial model. Recurrence risk data support the idea that the different major forms of NTDs are caused by similar genetic and nongenetic factors. An anencephalic conception increases the recurrence risk for subsequent spina bifida conceptions, and vice versa.
NTDs can usually be diagnosed prenatally, sometimes by ultrasound and usually by an elevation in α-fetoprotein (AFP) in the maternal serum or amniotic fluid (see Chapter 13 ). A spina bifida lesion can be either open or closed (i.e., covered with a layer of skin). Open spina bifida is more likely to be detected by an AFP assay.
A major epidemiological finding is that mothers who supplement their diet with folic acid at the time of conception are less likely to produce children with NTDs. This result has been replicated in several different populations and is thus well confirmed. It has been estimated that approximately 50% to 70% of NTDs can be avoided simply by dietary folic acid supplementation. (Traditional prenatal vitamin supplements would not have an effect because administration does not usually begin until well after the time that the neural tube closes.) Since mothers would be likely to ingest similar amounts of folic acid from one pregnancy to the next, folic acid deficiency could well account for at least part of the elevated sibling recurrence risk for NTDs.
Dietary folic acid is an important example of a nongenetic factor that contributes to familial clustering of a disease. However, it is likely that there is genetic variation in response to folic acid, which helps to explain why most mothers with folic acid deficiency do not bear children with NTDs and why some who ingest adequate amounts of folic acid nonetheless bear children with NTDs. To address this issue, researchers are testing for associations between NTDs and variants in several genes whose products (e.g., methylene tetrahydrofolate reductase) are involved in folic acid metabolism (see Clinical Commentary 15.6 in Chapter 15 for further information on dietary folic acid supplementation and NTD prevention).

In contrast to most single-gene diseases, recurrence risks for multifactorial diseases can change substantially from one population to another (notice the differences between the London and Belfast populations in Table 12.1 ). This is because allele frequencies as well as environmental factors can differ among populations.
Empirical recurrence risks for multifactorial diseases are based on studies of large collections of families. These risks are specific to a given population.
It is sometimes difficult to differentiate polygenic or multifactorial diseases from single-gene diseases that have reduced penetrance or variable expression. Large data sets and good family history data are necessary to make the distinction. Several criteria are usually used to define multifactorial inheritance.
- ▪
The recurrence risk is higher if more than one family member is affected. For example, the sibling recurrence risk for a ventricular septal defect (VSD, a type of congenital heart defect) is 3% if one sibling has had a VSD but increases to approximately 10% if two siblings have had VSDs. In contrast, the recurrence risk for single-gene diseases remains the same regardless of the number of affected siblings. This increase does not mean that the family’s risk has actually changed. Rather, it means that we now have more information about the family’s true risk; because they have had two affected children, they are probably located higher on the liability distribution than a family with only one affected child. In other words, they have more risk factors (genetic and/or environmental) and are more likely to produce an affected child.
- ▪
If the expression of the disease in the proband is more severe, the recurrence risk is higher. This is again consistent with the liability model, because a more severe expression indicates that the affected person is at the extreme tail of the liability distribution (see Fig. 12.2 ). His or her relatives are thus at a higher risk to inherit disease-causing genes. For example, the occurrence of a bilateral (both sides) cleft lip/palate confers a higher recurrence risk on family members than does the occurrence of a unilateral (one side) cleft.
- ▪
The recurrence risk is higher if the proband is of the less commonly affected sex (see, for example, the previous discussion of pyloric stenosis). This is because an affected individual of the less susceptible sex is usually at a more extreme position on the liability distribution.
- ▪
The recurrence risk for the disease usually decreases rapidly in more remotely related relatives ( Table 12.2 ). Although the recurrence risk for single-gene diseases decreases by 50% with each degree of relationship (e.g., an autosomal dominant disease has a 50% recurrence risk for offspring of affected persons, 25% for nieces or nephews, 12.5% for first cousins, and so on), it decreases much more quickly for multifactorial diseases. This reflects the fact that many genetic and environmental factors must combine to produce a trait. All of the necessary risk factors are unlikely to be present in less closely related family members.
Table 12.2
Recurrence Risks for First-, Second-, and Third-Degree Relatives of Probands
Disease
Prevalence in General Population
Degree of Relation
First Degree
Second Degree
Third degree
Cleft lip/palate
0.001
0.04
0.007
0.003
Club foot
0.001
0.025
0.005
0.002
Congenital hip dislocation
0.002
0.005
0.006
0.004
- ▪
If the prevalence of the disease in a population is f (which varies between zero and one), the risk for offspring and siblings of probands is approximately <SPAN role=presentation tabIndex=0 id=MathJax-Element-1-Frame class=MathJax style="POSITION: relative" data-mathml='f’>f√f
f
. This does not hold true for single-gene traits, because their recurrence risks are largely independent of population prevalence. It is not an absolute rule for multifactorial traits either, but many such diseases do tend to conform to this prediction. Examination of the risks given in Table 12.2 shows that these three diseases follow the prediction fairly well.
Risks for multifactorial diseases usually increase if more family members are affected, if the disease has more severe expression, and if the affected proband is a member of the less commonly affected sex. Recurrence risks decrease rapidly with more remote degrees of relationship. In general, the sibling recurrence risk is approximately equal to the square root of the prevalence of the disease in the population.
Multifactorial versus Single-Gene Inheritance
It is important to clarify the difference between a multifactorial disease and a single-gene disease in which there is locus heterogeneity. In the former case, a disease is caused by the simultaneous influence of multiple genetic and environmental factors, each of which has a relatively small effect. In contrast, a disease with locus heterogeneity, such as osteogenesis imperfecta, requires only a single mutation to cause it. Because of locus heterogeneity, a single mutation at either of two or more loci can cause disease; some affected persons have one mutation while others have the other mutation.
In some cases, a trait may be influenced by the combination of both a single gene with large effects and a multifactorial background in which additional genes and environmental factors have small individual effects ( Fig. 12.4 ). Imagine that variation in height, for example, is caused by a single locus (termed a major gene ) and a multifactorial component. Individuals with the AA genotype tend to be taller, those with the aa genotype tend to be shorter, and those with Aa tend to be intermediate. But additional variation is caused by other factors (the multifactorial component). Thus those with the aa genotype vary in height from 130 cm to about 170 cm, those with the Aa genotype vary from 150 cm to 190 cm, and those with the AA genotype vary from 170 to 210 cm. There is substantial overlap among the three major genotypes because of the influence of the multifactorial background. The total distribution of height, which is bell-shaped, is caused by the superposition of the three distributions about each genotype.

Many of the diseases to be discussed later can be caused by a major gene and/or multifactorial inheritance. That is, there are subsets of the population in which diseases such as colon cancer, breast cancer, or heart disease are inherited as single-gene disorders (with additional variation in disease susceptibility contributed by other genetic and environmental factors). These subsets usually account for only a small percentage of the total number of disease cases. It is nevertheless important to identify the responsible major genes, because their function can provide important clues to the pathophysiology and treatment of the disease.
Multifactorial diseases can be distinguished from single-gene disorders caused by mutations at different loci (locus heterogeneity). Sometimes a disease has both single-gene and multifactorial components.
Nature and Nurture: Disentangling the Effects of Genes and Environment
Family members share genes and a common environment. Family resemblance in traits such as blood pressure therefore reflects both genetic and environmental commonality (“nature” and “nurture,” respectively). For centuries, people have debated the relative importance of these two types of factors. It is a mistake, of course, to view them as mutually exclusive. Few traits are influenced only by genes or only by environment. Most are influenced by both.
Determining the relative influence of genetic and environmental factors can lead to a better understanding of disease etiology. It can also help in the planning of public health strategies. A disease in which hereditary influence is relatively small, such as lung cancer, may be prevented most effectively through emphasis on lifestyle changes (avoidance of tobacco). When a disease has a relatively larger hereditary component, as in breast cancer, examination of family history should be emphasized in addition to lifestyle modification.
In the following sections, we review two research strategies that are often used to estimate the relative influence of genes and environment: twin studies and adoption studies. We then discuss methods that aim to delineate the individual genes responsible for multifactorial diseases.
Twin Studies
Twins occur with a frequency of about 1/100 births in populations of European ancestry. They are slightly more common among Africans and a bit less common among Asians. Monozygotic (MZ, or identical) twins originate when the developing embryo divides to form two separate but genetically identical embryos. Because they are genetically identical, MZ twins are an example of natural clones. Their physical appearances can be strikingly similar ( Fig. 12.5 ). Dizygotic (DZ, or fraternal) twins are the result of a double ovulation followed by the fertilization of each egg by a different sperm. †
† While MZ twinning rates are quite constant across populations, DZ twinning rates vary somewhat. DZ twinning increases with maternal age until about age 40 years, after which the rate declines. The frequency of DZ twinning has increased dramatically in countries during the past several decades because of the use of ovulation-inducing drugs and in vitro fertilization.
Thus DZ twins are genetically no more similar than siblings. Because two different sperm cells are required to fertilize the two eggs, it is possible for each DZ twin to have a different father.
Because MZ twins are genetically identical, any differences between them should be due only to environmental effects. MZ twins should thus resemble each other very closely for traits that are strongly influenced by genes. DZ twins provide a convenient comparison; their environmental differences should be similar to those of MZ twins, but their genetic differences are as great as those between siblings. Twin studies thus usually consist of comparisons between MZ and DZ twins. If both members of a twin pair share a trait (e.g., cleft lip), they are said to be concordant. If they do not share the trait, they are discordant. For a trait determined completely by genes, MZ twins should always be concordant, and DZ twins should be concordant less often. Like siblings, DZ twins share only 50% of their DNA because each parent transmits half of his or her DNA to each offspring. Concordance rates can differ between opposite-sex DZ twin pairs and same-sex DZ pairs for some traits, such as those that have different frequencies in males and females. For such traits, only same-sex DZ twin pairs should be used when comparing MZ and DZ concordance rates.
A concordance estimate would not be appropriate for quantitative traits, such as blood pressure or height. Here the intraclass correlation coefficient is used. This statistic varies between −1.0 and +1.0 and measures the degree of homogeneity of a trait in a sample of individuals. For example, we may wish to assess the degree of similarity between twins for a trait such as height. The measurements are made in a collection of twins, and correlation coefficients are estimated separately for the MZ sample and the DZ sample. If a trait were determined entirely by genes, we would expect the correlation coefficient for MZ pairs to be 1.0 (i.e., each pair of twins would have exactly the same height). A correlation coefficient of 0.0 would mean that the similarity between MZ twins for the trait in question is no greater than chance. Because DZ twins share half of their DNA, we would expect a DZ correlation coefficient of 0.50 for a trait determined entirely by genes.
Monozygotic (identical) twins are the result of an early cleavage of the embryo, whereas dizygotic (fraternal) twins are caused by the fertilization of two eggs by two sperm cells. Comparisons of concordance rates and correlations in MZ and DZ twins help to estimate the extent to which a trait is influenced by genes.
Concordance rates and correlation coefficients for a number of traits are given in Table 12.3 . The concordance rates for contagious diseases like measles are quite similar in MZ and DZ twins. This is expected, because most contagious diseases are unlikely to be influenced markedly by genes. On the other hand, the concordance rates for schizophrenia are quite dissimilar between MZ and DZ twins, indicating a sizable genetic component for this disease. The MZ correlation for dermatoglyphics (fingerprints), a series of traits determined almost entirely by genes, is close to 1.0.
Trait or Disease | Concordance Rate | ||
---|---|---|---|
MZ Twins | DZ Twins | Heritability | |
Affective disorder (bipolar) | 0.79 | 0.24 | >1.0 ‡ |
Affective disorder (unipolar) | 0.54 | 0.19 | 0.70 |
Alcoholism | >0.60 | <0.30 | 0.60 |
Autism | 0.58 | 0.23 | 0.70 |
Blood pressure (diastolic) † | 0.58 | 0.27 | 0.62 |
Blood pressure (systolic) † | 0.55 | 0.25 | 0.60 |
Body fat percentage † | 0.73 | 0.22 | >1.0 |
Body mass index † | 0.95 | 0.53 | 0.84 |
Cleft lip/palate | 0.38 | 0.08 | 0.60 |
Club foot | 0.32 | 0.03 | 0.58 |
Dermatoglyphics (finger ridge count) † | 0.95 | 0.49 | 0.92 |
Diabetes mellitus (type 1) | 0.30–0.70 | 0.05–0.10 | ∼0.80 |
Diabetes mellitus (type 2) | 0.35–0.60 | 0.15–0.20 | ∼0.60 |
Epilepsy (idiopathic) | 0.69 | 0.14 | >1.0 |
Height † | 0.94 | 0.44 | 1.0 |
IQ † | 0.76 | 0.51 | 0.50 |
Measles | 0.95 | 0.87 | 0.16 |
Multiple sclerosis | 0.28 | 0.03 | 0.50 |
Myocardial infarction (males) | 0.39 | 0.26 | 0.26 |
Myocardial infarction (females) | 0.44 | 0.14 | 0.60 |
Schizophrenia | 0.47 | 0.12 | 0.70 |
Spina bifida | 0.72 | 0.33 | 0.78 |
∗ These figures were compiled from a large variety of sources and represent primarily European and U.S. populations. Heritability is estimated here using the formula 2(c MZ − c DZ ).
† Because these are quantitative traits, correlation coefficients are given rather than concordance rates.
‡ Several heritability estimates exceed 1.0. Because it is impossible for >100% of the variance of a trait to be genetically determined, these values indicate that other factors, such as shared environmental factors, must be operating.
Correlations and concordance rates in MZ and DZ twins can be used to measure the heritability of multifactorial traits. Essentially, heritability is the percentage of population variation in a trait that is due to genes (statistically, it is the proportion of the total variance of a trait that is caused by genes). A simple formula for estimating heritability (h 2 ) from twin correlations or concordance rates is as follows:
h2=CMZ−CDZ1−CDZ
Like recurrence risks, heritability values are specific for the population in which they are estimated. However, there is usually agreement from one population to another regarding the general range of heritability estimates of most traits (e.g., the heritability of height is almost always high, and the heritability of contagious diseases is almost always low). The same is true of empirical recurrence risks.
Comparisons of correlations and concordance rates in MZ and DZ twins allow the estimation of heritability, a measure of the percentage of population variation in a disease that can be attributed to genes.
At one time twins were thought to provide a perfect “natural laboratory” in which to determine the relative influences of genetics and environment. But several difficulties arise. One of the most important is the assumption that the environments of MZ and DZ twins are equally similar. MZ twins are often treated more similarly than DZ twins. A greater similarity in environment can make MZ twins more concordant for a trait, inflating the apparent influence of genes. In addition, MZ twins may be more likely to seek the same type of environment, further reinforcing environmental similarity. On the other hand, it has been suggested that some MZ twins tend to develop personality differences in an attempt to assert their individuality.
Another difficulty is that the uterine environments of different pairs of MZ twins can be more or less similar, depending on whether there are two amnions and two chorions, two amnions and one shared chorion, or one shared amnion and one shared chorion. In addition, somatic mutations can occur during mitotic divisions of the cells of MZ twin embryos after cleavage occurs. Thus the MZ twins might not be quite “identical,” especially if a mutation occurred early in the development of one of the twins. Finally, methylation patterns, which can influence the transcription of specific genes, become more dissimilar in MZ twin pairs as they age. This dissimilarity is greater when the twins adopt markedly different habits and lifestyles (e.g., when one twin smokes cigarettes and the other does not).
Of the various problems with the twin method, the greater degree of environmental sharing among MZ twins is perhaps the most serious. One way to circumvent this problem, at least in part, is to study MZ twins who were raised in separate environments. Concordance among these twin pairs should be caused by genetic, rather than environmental similarities. As one might expect, it is not easy to find such twin pairs. A major effort to do so has been undertaken by researchers at the University of Minnesota, whose studies have shown a remarkable congruence among MZ twins reared apart, even for many behavioral traits. However, these studies must be viewed with caution, because the sample sizes are relatively small and because many of the twin pairs had at least some contact with each other before they were studied.
Although twin studies provide valuable information, they are also affected by certain biases. The most serious is greater environmental similarity between MZ twins than between DZ twins. Other biases include somatic mutations that might affect only one MZ twin and differences in the uterine environments of twins.
Adoption Studies
Studies of adopted children are also used to estimate the genetic contribution to a multifactorial trait. Offspring who were born to parents who have a disease, but who were adopted by parents lacking the disease can be studied to find out whether the offspring develop the disease. In some cases, these adopted persons develop the disease more often than do children in a comparative control population (i.e., adopted children who were born to parents who do not have the disease). This provides evidence that genes may be involved in causing the disease, because the adopted children do not share an environment with their affected natural parents. For example, schizophrenia is seen in 8% to 10% of adopted children whose natural parent had schizophrenia, whereas it is seen in only 1% of adopted children of unaffected parents.
As with twin studies, several precautions must be exercised in interpreting the results of adoption studies. First, prenatal environmental influences could have long-lasting effects on an adopted child. Second, children are sometimes adopted after they are several years old, ensuring that some nongenetic influences have been imparted by the natural parents. Finally, adoption agencies sometimes try to match the adoptive parents with the natural parents in terms of attributes such as socioeconomic status. All of these factors could exaggerate the apparent influence of biological inheritance.
Adoption studies provide a second means of estimating the influence of genes on multifactorial diseases. They consist of comparing disease rates among the adopted offspring of affected parents with the rates among adopted offspring of unaffected parents. As with the twin method, several biases can influence these studies.
These reservations, as well as those summarized for twin studies, underscore the need for caution in basing conclusions on twin and adoption studies. These approaches do not provide definitive measures of the role of genes in multifactorial disease, nor can they identify specific genes responsible for disease. Instead, they provide a preliminary indication of the extent to which a multifactorial disease may be influenced by genetic factors. Methods for the direct detection of genes underlying multifactorial traits are summarized in Box 12.1 .
As discussed in the text, twin and adoption studies are not designed to reveal specific genes that cause multifactorial diseases. The identification of specific causative genes is an important goal, because only then can we begin to understand the underlying biology of the disease and undertake to correct the defect. For complex multifactorial traits, this is a formidable task because of locus heterogeneity, the interactions of multiple genes, decreased penetrance, age-dependent onset, and phenocopies (persons who have a phenotype, such as breast cancer, but who do not carry a known disease-causing mutation, such as a BRCA1 alteration). Fortunately, recent technological advances are rapidly making this goal more attainable. Here we discuss several approaches that are used to identify the genes underlying multifactorial traits.
One way to search for these genes is to use conventional linkage analysis, as described in Chapter 8 . Disease families are collected, a single-gene mode of inheritance is assumed, and linkage analysis is undertaken with a large series of marker polymorphisms that span the genome (this is termed a genome scan ). If a sufficiently large LOD score (see Chapter 8 ) is obtained with a polymorphism, it is assumed that the region around this polymorphism might contain a disease-causing gene. This approach is sometimes successful, especially when there are subsets of families in which a single-gene mode of inheritance is seen (e.g., autosomal dominant, autosomal recessive). This was the case, for example, with familial breast cancer and familial Alzheimer disease, where some families presented a clear autosomal dominant mode of inheritance.
With many multifactorial disorders, however, such subsets are not readily apparent. Because of obstacles such as heterogeneity and phenocopies, traditional linkage analyses are often ineffective. An alternative approach involves comparing pairs or groups of relatives who are all affected by the same disease and assessing the extent to which different regions of the genome are shared among them . The logic of this approach is simple: if two relatives are both affected by a genetic disease, we would expect to see increased sharing of polymorphisms or DNA sequence in the genomic region that contains a susceptibility gene. For example, first-degree relatives, such as siblings or parents and offspring, are expected to share 50% of their genome, but if affected pairs share a significantly larger proportion of DNA sequence in a specific genomic region, then that region may contain a causal gene. This approach has the advantage that one does not have to assume a specific mode of inheritance. In addition, the method is unaffected by incomplete penetrance, because the analysis is restricted only to affected individuals. The method is sometimes made more powerful by selecting subjects with extreme values of a trait (e.g., sibling pairs with very high blood pressure) to enrich the sample for genes likely to contribute to the trait. A variation on this approach is to sample pairs of relatives that are highly discordant for a trait (e.g., one with very high blood pressure and one with very low blood pressure), and then to look for genomic regions in which there is less allele sharing than expected.
During the past two decades, genome-wide association studies (GWAS, see Chapter 8 ) have become common in searches for genes that cause complex diseases. GWAS became more practical after the Human Genome Project developed dense sets of single nucleotide polymorphisms (SNPs) that span the human genome. It is now common to use microarrays that can assay millions of SNPs or high-throughput DNA sequencing strategies in a collection of cases and controls. GWAS are carried out using both SNPs as well as larger CNVs, which can contain multiple genes. Because the differences in the frequencies of disease-causing variants can be quite small, thousands or even tens of thousands of cases and controls are often tested in these studies. As in all case-control studies, it is vital to match cases and controls adequately for confounding factors such as ancestral background (see discussion in Chapter 8 ). Sophisticated methods have been developed to detect differences in ancestry and to correct for them statistically. A major challenge with GWAS is that the variants detected by this technique typically have only a small effect on disease risk (5%–20%), and their biological significance is usually difficult to interpret. Confidence in the plausibility of disease-associated genes increases if an appreciable number of the genes associated with a disease encode products in the same disease-associated pathway (e.g., the folate metabolism pathway for neural tube defects, or the dopaminergic pathway for schizophrenia).
GWAS data are now often combined with other types of genome-wide data, such as RNAseq ( Chapter 3 ), to test whether disease-associated variants are also associated with altered levels of mRNA expression in an appropriate tissue. DNA variants associated with variation in mRNA expression of a gene are termed expression quantitative trait loci , or eQTLs ). For example, some DNA variants associated with human height are also associated with variation in the mRNA expression levels of genes that affect fibroblast growth factor signaling, adding credence to their biological role in influencing height.
As the cost of exome and whole-genome sequencing has decreased, it has become increasingly common to compare DNA sequences in affected cases and matched controls. As discussed in Chapter 8 , this approach has the advantage that it can detect all variants, including rare alleles that differ in the two groups. Because these alleles are rare, large sample sizes must be used to detect their effects. This problem can be overcome somewhat by adding the effects of all disease-associated alleles at a given locus together (this is termed a burden test ). In addition, there has been a resurgence of interest in sequencing families, in which even a rare allele is likely to be observed in multiple cases. This approach has been successful, for example, in identifying new genes associated with late-onset Alzheimer disease.
In addition to searching for variants in protein-coding DNA, there is now considerable interest in identifying noncoding regulatory variants that may contribute to complex diseases. Indeed, about 80% of the SNPs associated with complex diseases in GWAS are found in noncoding DNA. This motivates whole-genome sequencing studies. Our understanding of variation in regulatory sequences such as enhancers and promoters is limited, however, but initial results (e.g., the association between enhancer elements and prostate cancer, discussed in the text) suggest that much will be learned by analyzing noncoding variation in the genome. Studies of epigenetic regulation (e.g., methylation and chromatin modification patterns, which can be correlated with GWAS results) are also beginning to yield new insights into the causes of common, multifactorial diseases.
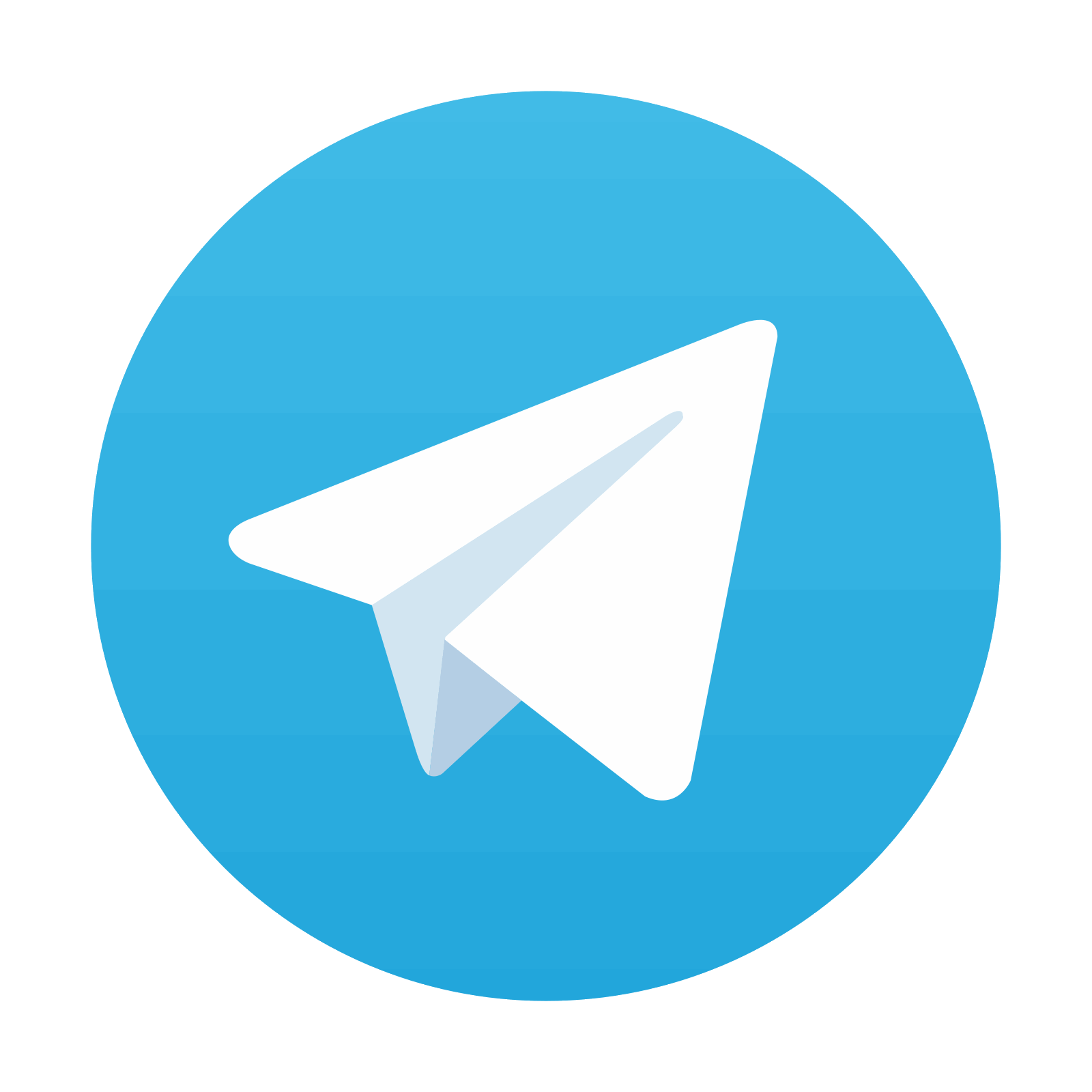
Stay updated, free articles. Join our Telegram channel

Full access? Get Clinical Tree
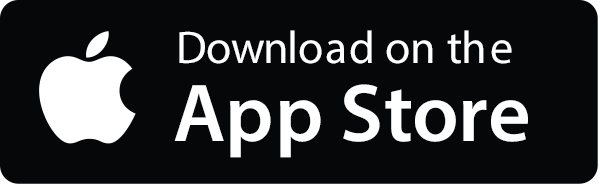

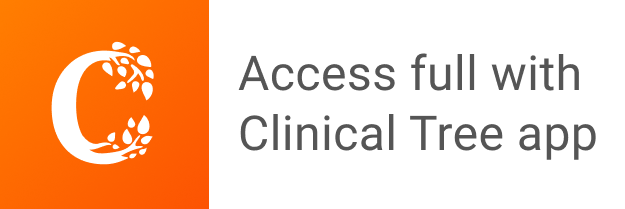