and Jordan Smoller2
(1)
Department of Epidemiology, Albert Einstein College of Medicine, Bronx, NY, USA
(2)
Department of Psychiatry and Center for Human Genetic Research, Massachusetts General Hospital, Boston, MA, USA
It is no easy task to pitch one’s way from truth to truth through besetting errors.
Peter Marc Latham 1789–1875
It is no easy task to pitch one’s way from truth to truth through besetting errors.
Peter Marc Latham
1789–1875
I wouldn’t have seen it if I didn’t believe it!
Attributed to Yogi Berra
Unfortunately, sometimes scientists see what they believe instead of believing what they see. Randomized, controlled clinical trials are intended to avoid that, and other kinds, of bias.
A randomized clinical trial is a prospective experiment to compare one or more interventions against a control group in order to determine the effectiveness of the interventions. A clinical trial may compare the value of a drug versus a placebo. A placebo is an inert substance that looks like the drug being tested. It may compare a new therapy with a currently standard therapy, surgical with medical intervention, two methods of teaching reading, and two methods of psychotherapy. The principles apply to any situation in which the issue of who is exposed to which condition is under the control of the experimenter and the method of assignment is through randomization.
6.1 Features of Randomized Clinical Trials
(1)
There is a group of patients who are designated study patients. All criteria must be set forth and met before a potential candidate can be considered eligible for the study. Any exclusions must be specified.
(2)
Any reasons for excluding a potential patient from participating in the trial must be specified prior to starting the study. Otherwise, unintentional bias may enter. For example, supposing you are comparing coronary bypass surgery with the use of a new drug for the treatment of coronary artery disease. Suppose a patient comes along who is eligible for the study and gets assigned to the surgical treatment. Suppose you now discover the patient has kidney disease. You decide to exclude him from the study because you think he may not survive the surgery with damaged kidneys. If you end up systematically excluding all the sicker patients from the surgical treatment, you may bias the results in favor of the healthier patients, who have a better chance of survival in any case. In this example, kidney disease should be an exclusion criterion applied to the patients before they are assigned to any treatment group.
(3)
Once a patient is eligible, he or she is randomly assigned to the experimental or control group. Random assignment is not “haphazard” assignment, but rather it means that each person has an equal chance of being an experimental or control patient. It is usually accomplished by the use of a table of random numbers, described later, or by computer-generated random numbers.
(4)
Clinical trials may be double-blind, in which neither the treating physician nor the patient knows whether the patient is getting the experimental treatment or the placebo; they may be single-blind, in which the treating physician knows which group the patient is in but the patient does not know. A double-blind study contains the least bias but sometimes is not possible to do for ethical or practical reasons. For example, the doctor may need to know the group to which the patient belongs so that medication may be adjusted for the welfare of the patient. There are also trials in which both patients and physicians know the treatment group, as in trials comparing radical mastectomy versus lumpectomy for treatment of breast cancer. When mortality is the outcome, the possible bias introduced is minimal, provided that exclusion criteria were specified and applied before eligibility was finally determined and that the randomization of eligible participants to treatment groups was appropriately done.
(5)
While clinical trials often compare a drug or treatment with placebo, they may also compare two treatments with each other, or a treatment and “usual care.” Trials that compare an intervention with “usual care” obviously cannot be blinded, for example, comparing a weight-loss nutritional intervention with “usual” diet; however, the assessment of effect (measurement of weight, or blood pressure, or some hypothesized effect of weight loss) should be done in a blinded fashion, with the assessor not knowing which group the participant has been assigned to.
(6)
It is essential that the control group be as similar to the treatment group as possible so that differences in outcome can be attributed to differences in treatment and not to different characteristics of the two groups. Randomization helps to achieve this comparability.
(7)
We are concerned here with Phase III trials. New drugs have to undergo Phase I and II trials, which determine toxicity, and safety and efficacy, respectively. These studies are done on small numbers of volunteers. Phase III trials are large clinical trials, large enough to provide an answer to the question of whether the drug tested is better than placebo or than a comparison drug.
6.2 Purposes of Randomization
The basic principle in designing clinical trials or any scientific investigation is to avoid systematic bias. When it is not known which variables may affect the outcome of an experiment, the best way to avoid systematic bias is to assign individuals into groups randomly. Randomization is intended to insure an approximately equal distribution of variables among the various groups of individuals being studied. For instance, if you are studying the effect of an antidiabetic drug and you know that cardiac risk factors affect mortality among diabetics, you would not want all the patients in the control group to have heart disease, since that would clearly bias the results. By assigning patients randomly to the drug and the control group, you can expect that the distribution of patients with cardiac problems will be comparable in the two groups. Since there are many variables that are unknown but may have a bearing on the results, randomization is insurance against unknown and unintentional bias. Of course, when dealing with variables known to be relevant, one can take these into account by stratifying and then randomizing within the strata. For instance, age is a variable relevant to diabetes outcome. To stratify by age, you might select four age groups for your study: 35–44, 45–54, 55–64, 65 plus. Each group is considered a stratum. When a patient enters into the clinical trial, his age stratum is first determined, and then he is randomly assigned to either experimental or control groups. Sex is another variable that is often handled by stratification.
Another purpose of randomization has to do with the fact that the statistical techniques used to compare results among the groups of patients under study are valid under certain assumptions arising out of randomization. The mathematical reasons for this can be found in the more advanced texts listed in the Suggested Readings.
It should be remembered that sometimes randomization fails to result in comparable groups due to chance. This can present a major problem in the interpretation of results, since differences in outcome may reflect differences in the composition of the groups on baseline characteristics rather than the effect of intervention. Statistical methods are available to adjust for baseline characteristics that are known to be related to outcome. Some of these methods are logistic regression, Cox proportional hazards models, and multiple regression analyses.
6.3 How to Perform Randomized Assignment
Random assignment into an experimental group or a control group means that each eligible individual has an equal chance of being in each of the two groups. This is often accomplished by the use of random number tables. For example, an excerpt from such a table is shown below:
48461 | 70436 | 04282 |
76537 | 59584 | 69173 |
Its use might be as follows. All even-numbered persons are assigned to the treatment group, and all odd-numbered persons are assigned to the control groups. The first person to enter the study is given the first number in the list, the next person gets the next number, and so on. Thus, the first person is given number 48461, which is an odd number and assigns the patient to the control group. The next person is given 76537; this is also an odd number so he/she too belongs to the control group. The next three people to enter the study all have even numbers, and they are in the experimental group. In the long run, there will be an equal number of patients in each of the two groups.
6.4 Two-Tailed Tests Versus One-Tailed Test
A clinical trial is designed to test a particular hypothesis. One often sees this phrase in research articles: “Significant at the .05 level, two-tailed test.” Recall that in a previous section, we discussed the concept of the “null hypothesis,” which states that there is no difference between two groups on a measure of interest. We said that in order to test this hypothesis, we would gather data so that we could decide whether we should reject the hypothesis of no difference in favor of some alternate hypothesis. A two-tailed test versus a one-tailed test refers to the alternate hypothesis posed. For example, suppose you are interested in comparing the mean cholesterol level of a group treated with a cholesterol-lowering drug to the mean of a control group given a placebo. You would collect the appropriate data from a well-designed study, and you would set up the null hypothesis as
Ho: | Mean cholesterol in treated group = mean cholesterol in control group |
You may choose as the alternate hypothesis | |
HA: | Mean cholesterol in treated group is greater than the mean in controls |
Under this circumstance, you would reject the null hypothesis in favor of the alternate hypothesis if the observed mean for women was sufficiently greater than the observed mean for men, to lead you to the conclusion that such a great difference in that direction is not likely to have occurred by chance alone. This, then, would be a one-tailed test of the null hypothesis.
If, however, your alternate hypothesis was that the mean cholesterol level for females is different from the mean cholesterol level for males, then you would reject the null hypothesis in favor of the alternate either if the mean for women was sufficiently greater than the mean for men or if the mean for women was sufficiently lower than the mean for men. The direction of the difference is not specified. In medical research, it is more common to use a two-tailed test of significance since we often do not know in which direction a difference may turn out to be, even though we may think we know before we start the experiment. In any case, it is important to report whether we are using a one-tailed or a two-tailed test.
6.5 Clinical Trial as “Gold Standard”
Sometimes observational study evidence can lead to misleading conclusions about the efficacy or safety of a treatment, only to be overturned by clinical trial evidence, with enormous public health implications. The Women’s Health Initiative (WHI) clinical trial of hormone therapy is a dramatic example of that.22 Estrogen was approved by the FDA for relief of postmenopausal symptoms in 1942, aggressively marketed in the mid-1960s, and after 1980, generally combined with progestin for women with a uterus because it was found that progestin offset the risks of estrogen for uterine cancer. In the meantime, many large prospective follow-up studies almost uniformly showed that estrogen reduced heart diseases by 30–50 %. In the 1993, WHI mounted a large clinical trial to really answer the question of long-term risks and benefits of hormone therapy. One part was the study of estrogen alone for women had had a hysterectomy and thus didn’t need progestin to protect their uterus, and another part was of estrogen plus progestin (E + P) for women with an intact uterus.
The E + P trial was a randomized, double-blind, placebo-controlled clinical trial meant to run for an average of 8.5 years. It included 16,608 women ages 50–79; such a large sample size was deemed necessary to obtain adequate power. The trial was stopped in 2002, 3 years before its planned completion, because the Data and Safety Monitoring Board or DSMB (as described in Chapter 10) found estrogen plus progestin caused an excess of breast cancer, and surprisingly, there was a significant and entirely unexpected excess of heart attacks in the E + P group compared to placebo! Final results, reported in subsequent papers, showed that the adverse effects (a 24 % increase in invasive breast cancer, 31 % increase in strokes, 29 % increase in coronary heart disease, and more than a twofold increase in pulmonary embolism and in dementia) offset the benefits (a 37 % decrease in colorectal cancer and 34 % decrease in hip fractures), so that taken together, the number of excess harmful events per year was substantial. Since there were six million women taking this preparation in the United States alone, and millions more globally, these results have important implications for women other than those in the trial itself.
Why such different results from a clinical trial than from observational longitudinal studies? The most likely explanation is selection bias. Women who were taking hormones and then followed to observe their rates of heart disease were, in virtually all the observational studies, healthier, thinner, more active, more educated, and less overweight, than their non-hormone-taking counterparts, and their healthier lifestyle and better baseline health status, rather than the hormones per se, was what accounted for their lower rates of heart disease.
The question now is answered using the “gold standard,” the clinical trial: estrogen plus progestin does not protect against heart disease and in fact increases the risk. As noted before, the impact of this research is great since so many millions of women were using the preparation tested.
6.6 Regression Toward the Mean
When you select from a population those individuals who have high blood pressure and then at a later time measure their blood pressure again, the average of the second measurements will tend to be lower than the average of the first measurements and will be closer to the mean of the original population from which these individuals were drawn. If between the first and second measurements you have instituted some treatment, you may incorrectly attribute the decline of average blood pressure in the group to the effects of treatment, whereas part of that decline may be due to the phenomenon called regression toward the mean. (That is one reason why a placebo control group is most important for comparison of effects of treatment above and beyond that caused by regression to the mean.) Regression to the mean occurs when you select out a group because individuals have values that fall above some criterion level, as in screening. It is due to variability of measurement error. Consider blood pressure.
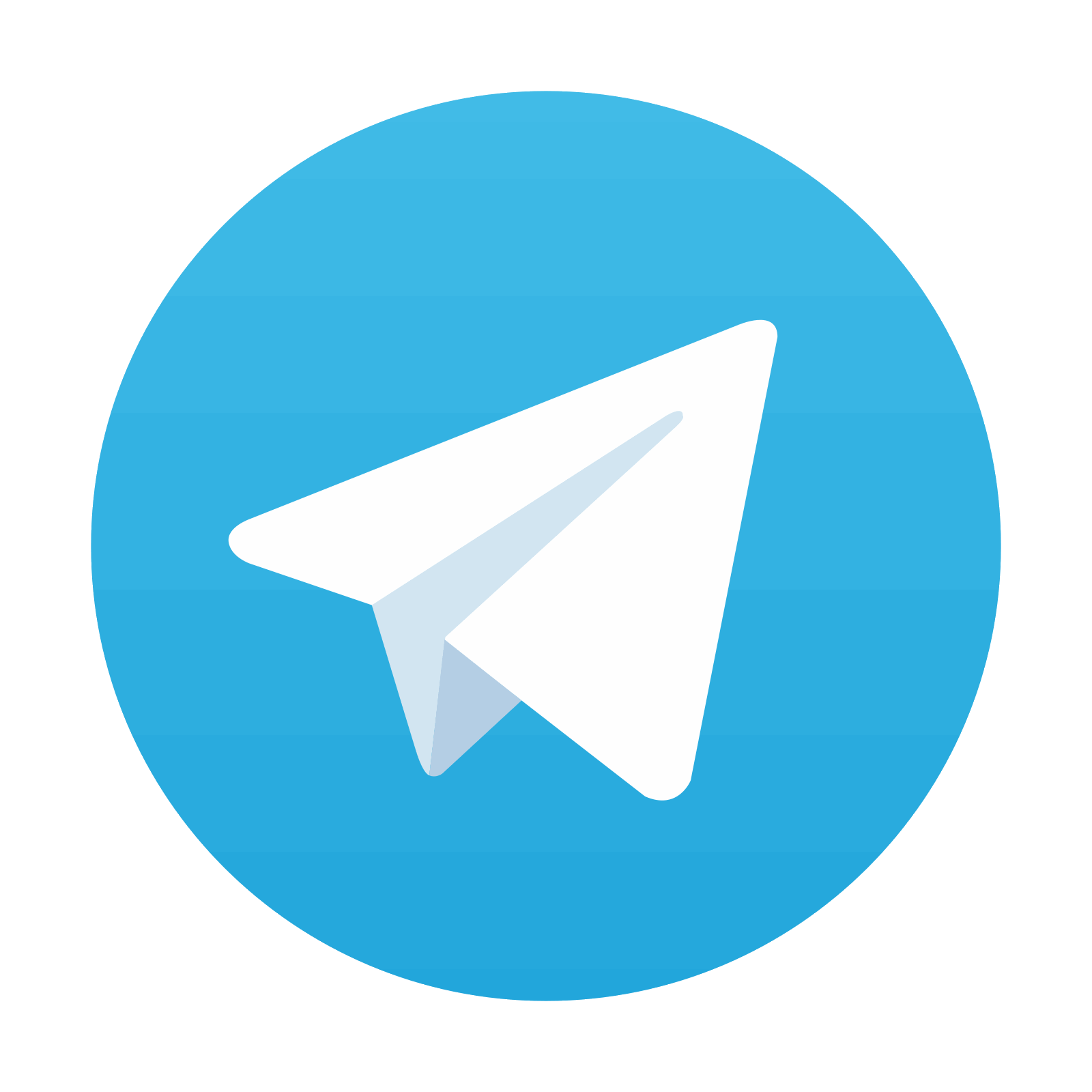
Stay updated, free articles. Join our Telegram channel

Full access? Get Clinical Tree
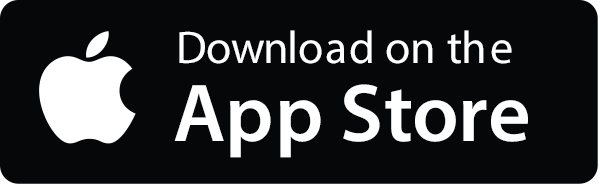
