Chapter 17 Methods in the study of clinical reasoning
This chapter presents an overview of some of the methods used in the study of clinical reasoning. It does not constitute an exhaustive overview. Rather, it presents major features of the most common approaches used in the study of clinical reasoning. In addition, we include a range of new and promising research methodologies used in clinical reasoning research and elsewhere.
From purely behaviouristic and psychometric roots, the study of clinical reasoning (see Patel et al 2005 for a review) has diversified to include different methodological commitments and techniques, developing a multiplicity of methods, and continues to do so. We have found it useful to categorize such methods along different dimensions (see Figure 17.1). One is the individual–group dimension, or the extent to which a study focuses on the individual or the group as units of analysis. A second dimension is the quantitative–qualitative dimension, or the extent to which the study makes of use quantitative or qualitative methods of data gathering and analysis. Specific studies can, of course, vary along these dimensions and may use quantitative methods to identify average differences between groups together with verbal protocol methods to characterize individual performance (Hashem et al 2003, Patel et al 2001) or use of verbal protocols in combination with interpretive methods (Ritter 2003).
QUANTITATIVE METHODS
Quantitative methodologies for investigating medical reasoning have been used in various clinical tasks. One aspect of clinical reasoning that has been investigated using group-based quantitative methods is the study of diagnosis in perceptual tasks such as X-ray or dermatological slide interpretation (Crowley et al 2003; Lesgold et al 1988; Norman et al 1989a, b). In such studies, subjects are presented with a series of X-rays or slides and then, after a period, are asked to interpret or recall the information in them. The goal is to show how variations in the subjects’ interpretations (e.g. assessed through verbal recall) relate to the variations on the experimental conditions (e.g. types of slide). These data are then quantified using descriptive statistics and subjected to standard statistical analysis (e.g. null-hypothesis testing). The same methods have been employed by others (e.g. Patel & Frederiksen 1984, Schmidt & Boshuizen 1993) to compare clinical performance by groups with different levels of expertise using verbal materials such as the clinical case as independent variable and various dependent measures such as recall (Patel & Frederiksen 1984), diagnostic accuracy (Patel & Groen 1986), probability assignments (e.g. Hasham et al 2003), or decision times (Rikers et al 2005).
Although quantitative methods are most commonly used to investigate average group differences (e.g. between experimental and control groups or between expertise levels), there is a fundamental reason for also investigating individual subjects quantitatively, namely the search for behavioural or cognitive invariants across all individuals (Runkel 1990, 2003; Simon 1990). If the overall goal of research is to understand the functioning of human beings, how they are organized such that they are capable of producing what we observe them producing, then that organization must be the same for everyone. Although unfortunately little use has been made of such methods, their addition to the methodological toolbox of the clinical reasoning researcher is welcome.
Theoretical approaches underlying individual subject research are varied, including behaviouristic, information processing, control theoretic or system dynamic approaches. Some stress the changes in overt behaviour across time while others stress the process of thinking and reasoning, the role of goals and intentions as part of people’s attempts to engage in interaction with the external environment, or the perceived consequences of such engagement (Runkel 2003). The basic idea is that humans operate according to a set of principles described by the model, which must be known in order to give an accurate account of human performance in detail.
One strategy for conducting individual subject research developed from the behaviouristic research is to look at changes across condition for each individual, where the individual serves as his or her own control, by comparing multiple measures of behaviour at baseline and after experimental intervention (Sterling & McNally 1992, Weiner & Eisen 1985). Another strategy consists of generating models of individual organization, such as a computational model, and then testing the fit of the model to individual data, using quantitative fitting measures, such as the root-mean square or correlations. Research consistent with this strategy has been associated mostly with research in artificial intelligence (e.g. Clancey 1997).
A quantitative model-based methodology that sheds light on the study of reasoning is exemplified by the decision-making approach, originating from the study of economic decisions, although widely applied to other fields, including medical decisions. Typically, researchers in decision making start with a formal model of decision making and then collect data which are compared to the model. The models can be of various types, such as simple regression models, Bayesian estimation models and decision-theoretic models. The latter are the most mathematically sophisticated (Christensen et al 1991).
Decision theory has its roots in the work of Von Neumann & Morgenstern (1944) on game theory. The theory deals with making decisions in situations of uncertainty. The basic principle of the theory is that a rational person should maximize his or her expected utility, which is defined as the product of probability by utility. Hammond (1967) gave the following example: a businessman faces the decision of either winning $500,000 or losing $100,000, both of which have the same probability, 0.5. The expected utility in this case would be of $200,000 [0.5 (500,000) − 0.5 (100,000)]. Decision theory has been used mostly as a model for rational decision making. Previously, the theory was thought to describe actual human decision making, but empirical research on the psychological bases of decision making has falsified its claims as a descriptive theory (Tversky & Kahneman 1974). The theory also has been used as a normative theory under the assumption that the maximization of the expected utilities is rational. Under this assumption, to be rational, people’s decisions must mirror those derived from the model. If people’s decisions depart from those specified by the model, it is taken as evidence that they are not behaving rationally. This assumption, and therefore the normative character of the theory, has also been severely questioned (Allais & Hagen 1979, Bunge 1985, Hammond 1967). In short, critics argue that it is not always rational to maximize one’s expected utility and therefore the theory cannot be taken as a prescription for action.
Whatever its merits either as a descriptive or a prescriptive theory, decision theory has stimulated a great deal of research in medicine (Weinstein et al 1980) and various other domains (Carroll & Johnson 1990, Dawes 1988). The research on decision theory uses a model that serves as comparison for the empirical studies. Consistent with its assumptions, most utility models are assumed to be models of rationality such that lack of agreement between the subject’s responses and the model is taken as evidence that the subject does not make decisions rationally.
It is important to note that for such models to apply, all the information has to be available to the subject (and to the model). Also note that only the selection of alternatives (e.g. diagnoses) is illustrated by these models. Such observations have provoked some researchers (Fox 1988) to argue for an expansion in the study of decision making to include also the intermediate processes between the selection of attributes and the reaching of the decision. The argument supports the developing of knowledge-based decision methods based on the techniques of artificial intelligence, which calls for the inclusion of heuristics (e.g. means–ends analysis) and knowledge structures in the decision model. Although decision models have been used to describe human behaviour, psychological research (Tversky & Kahneman 1974) has shown that subjects do not behave according to the models. People show various kinds of biases that depart systematically from the models’ predictions.
QUALITATIVE METHODS
This section deals with what are considered to be, overall, qualitative methods – those in quadrants I and IV of Figure 17.1. The methods described in this section vary widely in terms of their origins and applications and cover think-aloud protocols, discourse analysis and ethnographic methods. The first originates in the study of problem solving and the computer simulation of thought processes (Elstein et al 1990, Newell & Simon 1972), the second in the analysis of text comprehension (Frederiksen 1975) and conversation (Schiffrin et al 2001) and the third in the analysis of complex, mostly social, situations (Suchman 1987).
VERBAL REPORTS
There are two kinds of verbal report. One kind is the ‘think-aloud’ method used in clinical reasoning and expertise research (Kassirer et al 1982). The second is the retrospective protocol, such as stimulated recall (Elstein et al 1978) and the explanation protocol (Arocha et al 2005, Patel & Arocha 1995, Patel & Groen 1986). In both cases, the researcher uses verbalizations as data, without involving introspection. That is, subjects are asked to verbalize their thoughts without ‘theorizing’ about their cognitive processes. Any theorizing is the responsibility of the experimenter and not of the subject (Ericsson & Simon 1984, Newell & Simon 1972). Analysis methods can be found in Ericsson & Simon (1984).
Think-aloud protocols
In typical think-aloud research, subjects are presented with a clinical case, most frequently in written form, which may contain anything from a single sentence to a whole patient record including the clinical interview, the physical examination results and the laboratory results (e.g. Hashem et al 2003). The subject is asked to read the information and verbalize whatever thoughts come to mind. If the subject pauses for a few seconds, the experimenter intervenes with questions such as ‘What are you thinking about?’ or, more appropriately, with demands such as ‘Please, continue’, which encourage the subject to carry on talking without introspecting.
Once the protocol has been collected, it is subjected to an analysis aimed at uncovering the cognitive processes and the information that were used. The analysis of the protocol is then compared to a reference or domain model of the task to be solved. This model is frequently taken either from an expert collaborator in the study or from printed information about the topic, such as textbooks or scholarly expositions. For instance, Kuipers & Kassirer (1984), in their study of causal reasoning, used a model of the Starling equilibrium mechanism which was compared to the protocols from subjects at different levels of expertise: medical students, residents, and expert physicians. In the same vein, Patel and her colleagues (Joseph & Patel 1990, Patel & Groen 1986) used a reference model of the clinical cases, which served as a standard for comparison with subjects’ protocols.
For think-aloud reports to be valid, it is necessary that some conditions be met. The conditions pertain to the type of task that should be used, the kinds of instruction given to the subject, and the familiarity of the subject with the task. Ericsson & Simon (1984) developed an extensive description of these conditions, and there is also independent research that has shown the validity of the methods (White 1988). The theory of protocol analysis is based on the assumption that verbalizations reflect a subject’s search through a problem space of hypotheses and data.
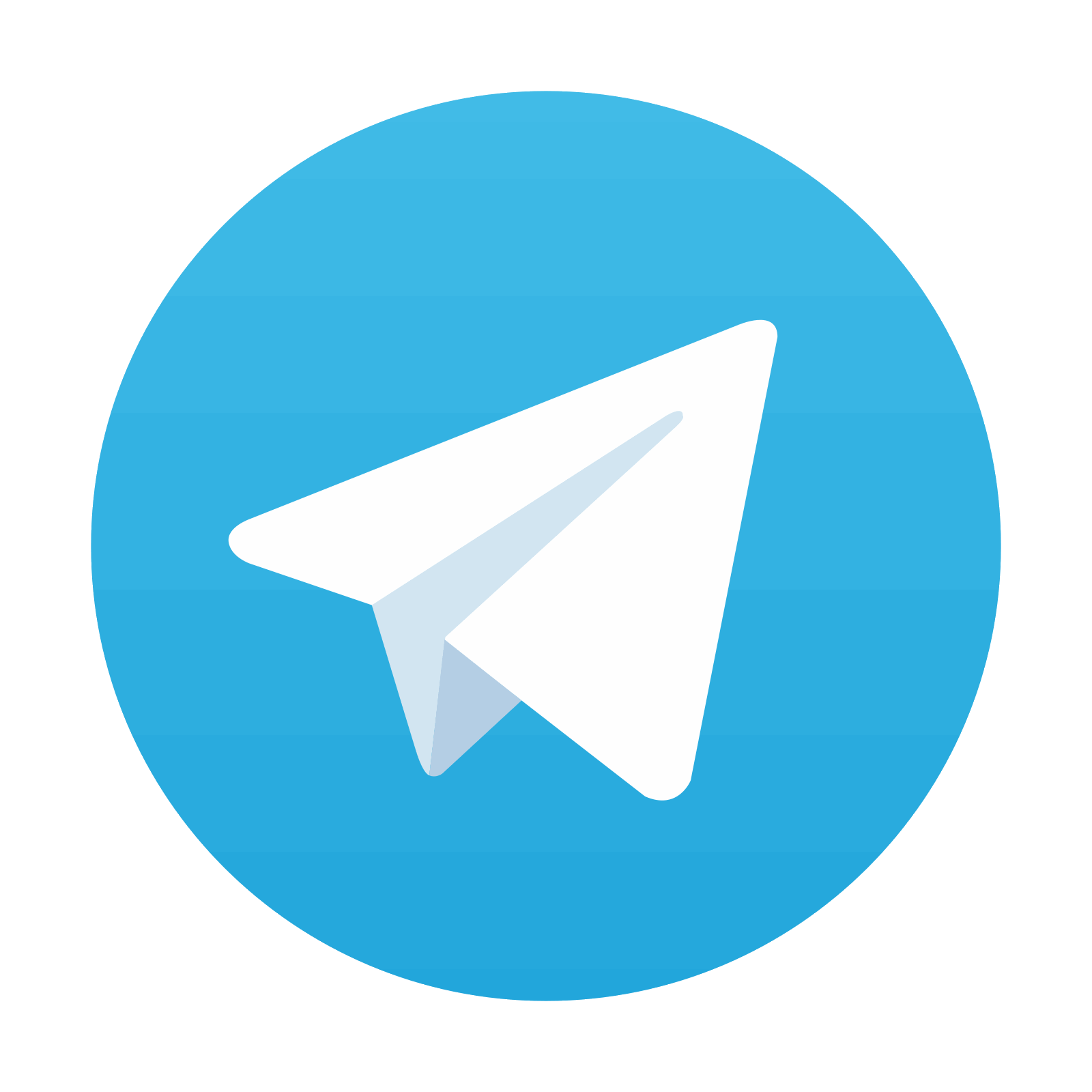
Stay updated, free articles. Join our Telegram channel

Full access? Get Clinical Tree
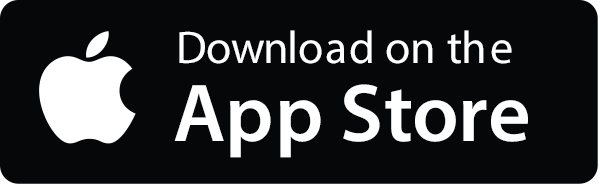
