Treatment choice
Selection factor
Expected selection bias
Expected results from biases alone
Estimated strength of effect
Chemo vs. no in several clinical scenarios
General health
Chemo prescribed only for healthier patients
Chemo patients have lower non-cancer mortality
+++
Tumor prognosis
Chemo prescribed only for patients with worse tumor prognosis
Chemo associated with increased cancer mortality
++
Surgery vs. XRT in several clinical scenarios
General health
Surgery for healthier patients
Surgery associated with lower non-cancer mortality
++
Tumor prognosis
Variable association with tumor prognosis depending on site
Variable depending on site
++
Any treatment vs. no treatment
General health
Healthier patients treated
Treatment associated with decreased non-cancer mortality
++
Tumor prognosis
Variable association with tumor prognosis depending on site
Variable depending on site
+
Surgery & XRT vs. surgery alone
General health
XRT for healthier patients
XRT associated with decreased non-cancer mortality
+
Tumor prognosis
XRT for more extensive tumor
XRT associated with increased cancer mortality
++
14.2.1.3 Measurement Bias
Measurement bias involves systematic error in measuring the exposure, outcome, or covariates in a study. Measurement error is a major concern for observational studies, particularly those using administrative data such as Medicare claims. The likelihood of measurement error differs based on the type of intervention, outcome, or covariates being measured. For example, it is fairly straightforward to define and identify surgical procedures in Medicare claims data, and costly procedures tend to be accurately coded in billing data. In contrast, it can be very difficult to identify medication use, define an exposure period, and classify patients as treated or untreated. An outcome such as survival is less likely to have measurement error than outcomes such as postoperative complications or incident disease. Similarly, comorbid conditions or risk factors such as smoking may be more difficult to measure. This is particularly true with claims data because diagnosis codes are subject to considerable error and the use of a particular diagnosis code on a claim depends on the presence of the condition, a provider’s use of the code, and the presence of other, more serious conditions. One way to estimate the validity of the exposure and outcome variables in an observational study is to compare them with a gold standard such as patient self-report or the medical record.
14.2.2 Unmeasured Confounding
Even very rich datasets such as medical records lack complete information on factors influencing selection of treatment. Perhaps the best example of the prognostic strength of missing variables is self-rated health. In most cohort studies, self-rated health is the strongest predictor of survival (after age and gender). More importantly, self-rated health remains a strong predictor in studies that include a rich array of medical, physiologic, social, and psychological variables, such as the Cardiovascular Health Survey. This means that there is a factor known by the patient and easily accessible by the physician (“How are you feeling?”) that clearly influences prognosis, that would likely influence treatment choice, and which is invisible in almost all comparative research studies using observational data.
Causal inference relies on the assumption of no unmeasured confounding; however, there is no way to test that the assumption is correct, making causal inference risky in observational studies. Investigators must do their best to identify, measure, and adjust for all potential confounders. Studies that do not use the appropriate methodology to account for observed and unobserved sources of bias and confounding produce biased effect estimates that can contribute to inappropriate treatment and policy decisions. In the next section, we discuss methods of controlling for bias in observational studies, including steps investigators can take during the design and analysis phases to minimize unmeasured confounding.
14.3 Controlling for Bias in Observational Studies
Careful study design and research methods are key for causal inference with observational data. No amount of sophisticated statistical analysis can compensate for poor study design. A helpful exercise to conduct when designing an observational study is to describe the randomized experiment the investigator would like to—but cannot—conduct, and attempt to design an observational study that emulates the experiment. Research investigators also should collaborate with statisticians, methodologists, and clinicians with relevant subject-matter knowledge during the study design and analysis process. These collaborators can provide expert input to identify issues with the research question and approach. They also can help to identify confounding variables—determinants of treatment that are also independent outcome predictors—and other potential sources of bias, and determine the expected strength and direction of the anticipated bias.
14.3.1 Study Design
The ideal way to minimize bias in observational studies of treatment effectiveness is to collect comprehensive patient, treatment, and outcome data suggested by relevant clinicians and methodologists. This is ideal for primary research studies; however, it is not an option for secondary data analysis on existing observational data sets. Investigators who use existing datasets cannot control how patients were identified and selected, and the analysis is limited to available variables and the way they were measured at the time of data collection. Therefore, it is critical for investigators using secondary data to consider the comprehensive list of potential patient, provider, and process of care factors as the investigator considers potential challenges to causal inference in order to evaluate the feasibility of answering the research question with the available data. We review several research practices for secondary data analysis that will help to improve causal inference.
Prior to designing a study and analyzing the data, investigators must familiarize themselves with the dataset they will be using, including how the sample was selected, how the data were collected, what variables are included and how they were defined, and the potential limitations. For example, hospital discharge data such as the Nationwide Inpatient Sample represent hospital discharges and not individual persons. Patients with multiple hospitalizations will be counted multiple times, and the dataset does not contain unique patient identifiers that allow follow-up after discharge. Administrative claims data such as Medicare data were not collected for research purposes and do not contain direct clinical information. Rather clinical information has to be inferred from diagnosis and procedure claims. In addition, diagnosis codes listed on claims were designed for reimbursement rather than surveillance purposes, and conditions may be included based on reimbursement rather than clinical importance.
Finally, sometimes a dataset contains inadequate information to investigate a particular question. For example, we wanted to use SEER data to examine breast cancer outcomes in patients with positive sentinel nodes who underwent sentinel lymph node biopsy alone or in combination with axillary lymph node dissection. After careful review of the SEER documentation for staging, lymph node status, and dissection variables, we discovered that SEER does not separately record the pathology status of sentinel lymph nodes from axillary lymph nodes. Investigators who are not familiar with their dataset may make incorrect assumptions about how data were collected or variables were defined that could jeopardize the results of their study and preclude causal inference.
Investigators need to explicitly define the intervention or treatment of interest. A well-defined causal effect is necessary for meaningful causal inference. For many interventions, there are a number of ways to define or measure exposure, which could lead to very different estimates of effectiveness. For example, in a study evaluating the effectiveness of chemotherapy, the definition of exposure to chemotherapy could specify a certain number of doses of chemotherapy within a certain time frame, or it could require only one dose at any time point after diagnosis. These definitions are likely to produce different results. Investigators using administrative claims data have to infer receipt of treatment based on claims for services and often have to develop surrogate measures of an intervention. This requires the investigator to make assumptions, and he/she must consider how results may be affected.
Another prerequisite for causal inference is a well-characterized target population. Investigators need to explicitly define the subset of the population in which the effect is being estimated and the population to whom the results may be generalized. The investigator should carefully select the study cohort and construct the treatment comparison groups and carefully define the observation period in which outcomes will be monitored. Cohort selection criteria should be specified to construct a ‘clean’ patient sample. For example, in a recent study evaluating overuse of cardiac stress testing before elective noncardiac surgery, the cohort was restricted to patients with no active cardiac conditions or clinical risk factors [9]. Cardiac stress testing was clearly not indicated in such patients; therefore, investigators could label testing as overuse. When defining an observation period, investigators must consider the length of time that is appropriate for the research question and the study outcome. For example, 2-year survival would be an adequate amount of time to assess the effectiveness of interventions for pancreatic cancer, but not for breast or prostate cancer. Finally, investigators must determine the extent to which the potential confounders identified by the research team are observable, measurable, or proxied by existing variables in the observational dataset.
14.3.2 Statistical Techniques
There are a number of statistical methods aimed at strengthening causal inference in observational studies of the comparative effectiveness of different treatments. Table 14.2 shows the most common statistical methods used to adjust for bias. Below, we briefly discuss the statistical methods with regard to their contributions to causal inference for observational data. A detailed description of these methods is beyond the scope of this chapter.
Table 14.2
Statistical methods to reduce confounding in observational studies
Statistical method | Purpose/use |
---|---|
Multivariate regression | Estimate conditional expectation of dependent variable given independent variables |
Propensity score analysis (stratification, matching, inverse probability weighting, regression adjustment) | Reduce imbalance in treatment and control groups based on observed variables |
Instrumental variable analysis | Adjust for unobserved confounding |
14.3.2.1 Multivariate Regression
Multivariate regression is the conventional method of data analysis in observational studies. Regression models may take many forms, depending on the distribution of the response variable and structure of the dataset. The most commonly used regression models include linear regression for continuous outcomes (e.g., the effect of age on FEV1), logistic regression for categorical outcomes (e.g., the effect of intraoperative cholangiography on bile duct injury), Cox proportional hazards models for time-to-event outcomes (e.g., effect of adjuvant chemotherapy on survival), and Poisson regression for count data (e.g., the effect of INR level on ischemic stroke rates).
Regression analysis is used to disentangle the effect of the relationship of interest from the contribution of the covariates that may affect the outcome. Regression can control for differences between treatment groups by providing estimates of the treatment effect when the other covariates are held fixed. However, in order to control for a covariate, it must be measurable in the observational dataset; therefore, multivariate regression analysis is unable to control for the effects of unmeasured confounders.
14.3.2.2 Stratification or Restriction Prior to Multivariate Regression
Stratification may be used as a method to adjust for a measurable prognostic factor that differs systematically between treatment groups, that is, a potential confounder. Patients are grouped into strata of the prognostic variable, and the treatment effect is estimated by comparing treated and untreated patients within each stratum. This method yields effect measures for each stratum of the prognostic variable, known as conditional effect measures. They do not indicate the average treatment effect in the entire population. Sometimes investigators estimate the treatment effect in only some of the strata defined by the prognostic factor, a form of stratification known as restriction.
Stratification and restriction create subgroups that are more homogeneous, sometimes enabling the investigator to identify the presence of confounding. For example, a study assessing the short-term outcomes of incidental appendectomy during open cholecystectomy used restriction to evaluate the consistency and plausibility of their results [10]. Table 14.3 shows the unadjusted and adjusted associations between incidental appendectomy and adverse outcomes in the overall cohort and in restricted subgroups. Unadjusted comparisons showed paradoxical reductions in mortality and length of stay associated with incidental appendectomy. Multivariate models adjusting for potential confounders, such as comorbidity and nonelective surgery, showed increased risk of nonfatal complications with incidental appendectomy but no differences in mortality or length of stay. The investigators believed that unmeasured differences between the appendectomy and no appendectomy groups were more likely to exist in high risk patients, confounding the estimates for the overall sample. After restricting the analysis to subgroups of patients with low surgical risk, incidental appendectomy was consistently associated with a small but definite increase in adverse postoperative outcomes.
Table 14.3
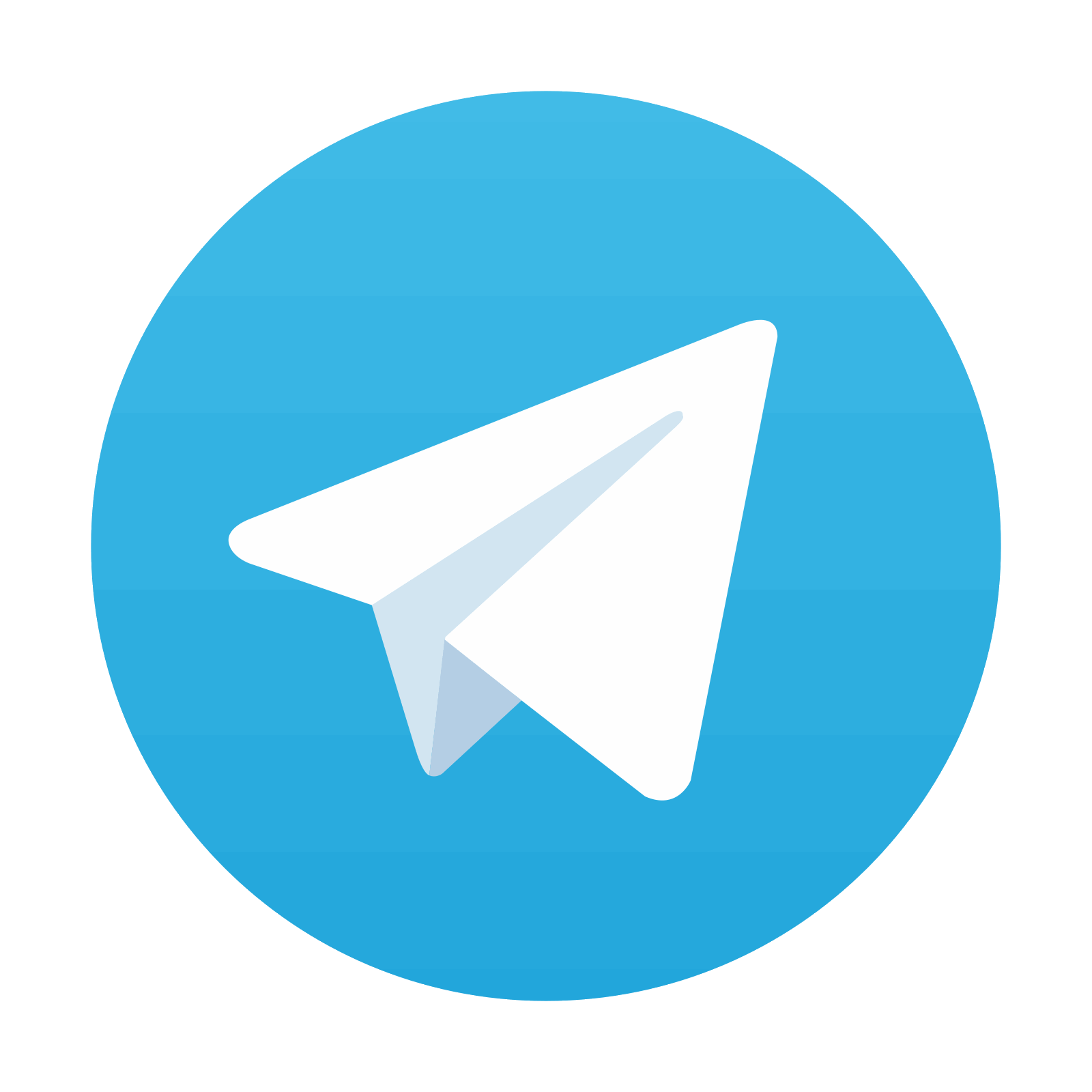
Outcomes of patients undergoing open cholecystectomy with vs. without incidental appendectomy for the overall patient cohort and low-risk subgroups
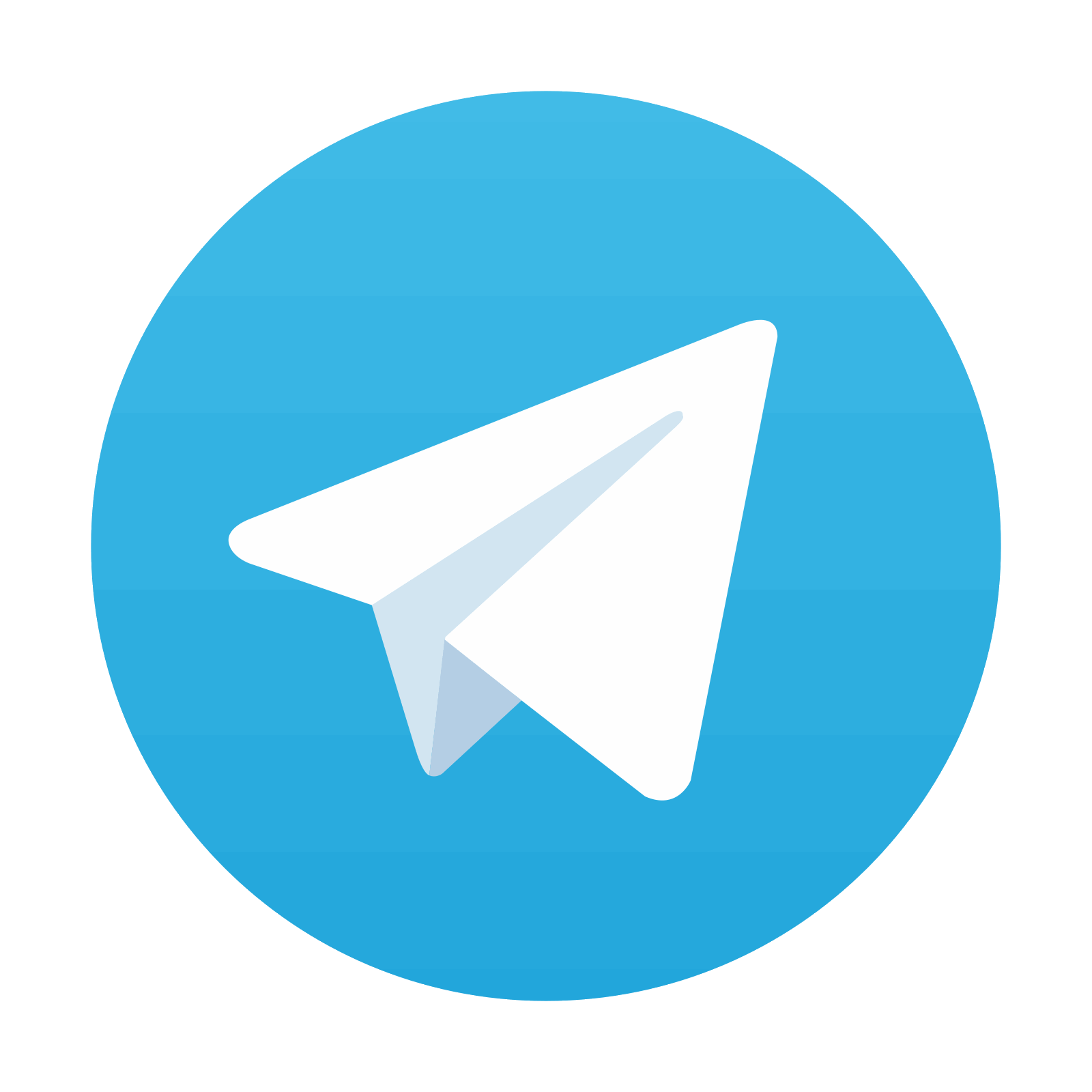
Stay updated, free articles. Join our Telegram channel

Full access? Get Clinical Tree
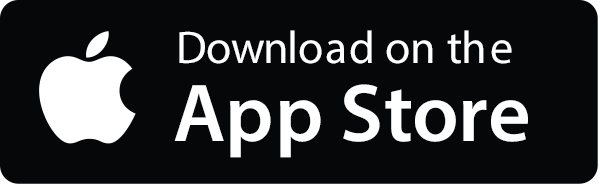

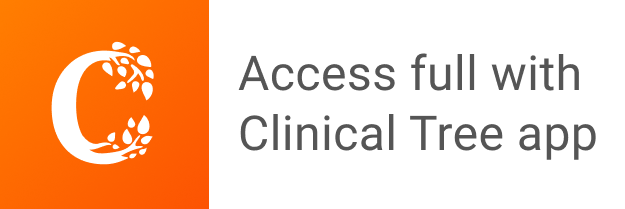