Type of question
Example
Description
Examination of rare conditions, procedures, complications
Sheffield et al. Association between cholecystectomy with vs without intraoperative cholangiography and risk of common duct injury. JAMA 2013
Used Texas Medicare data to explore a rare complication to demonstrate no statistically significant association between intraoperative cholangiography and common duct injury
Trends over time in utilization or outcomes
Santry et al. Trends in bariatric surgical procedures. JAMA 2005
Used the Nationwide Inpatient Sample to demonstrate a national increasing trend in the use of bariatric surgical procedures
Regional variations in utilization
Dartmouth Atlas of Health Care
Used national Medicare data to publish numerous studies exploring large regional variation in healthcare spending and outcomes
“Real world” efficacy vs. effectiveness
Wennberg et al.: Variation in carotid endarterectomy mortality in the Medicare population. JAMA 1998
Used national Medicare data to demonstrate carotid endarectomy mortality was significantly higher than reported in randomized controlled trials
Relationship of hospital or surgeon factors on patient outcomes
Birkmeyer et al. Hospital volume and surgical mortality in the United States. NEJM 2002
Used national Medicare data to demonstrate inverse relationship between hospital volume and surgical mortality among 14 major cardiovascular and cancer operations
Ghaferi et al.: Variation in hospital mortality associated with inpatient surgery. NEJM 2009
Used NSQIP database to demonstrate low mortality hospitals in general and vascular surgery had similar complication rates, but superior ability to rescue patients from complications when compared to high mortality hospitals
Policy evaluation
Dimick et al. Bariatric surgery complications before vs. after implementation of a national policy restricting coverage to centers of excellence. JAMA 2013
Used the State Inpatient Database to demonstrate no benefit in complication rates or reoperation rates after enactment of policy to restrict bariatric surgery to designated centers of excellence
13.2.2 Defining Temporal Trends or Regional Differences in Utilization
Secondary databases are also often used to explore the utilization rates of specific surgeries to discern temporal trends or regional differences. In 2005, Santry et al. used the Nationwide Inpatient Sample (NIS) to discern a significant increase trend in the use of bariatric surgical procedures over the years 1998–2002 [2]. Regional variation in rates of procedures has been described as early as 1970. The Dartmouth Atlas of Health Care has used national Medicare data to publish numerous studies exploring large regional variations in healthcare spending and outcomes [3].
13.2.3 Examining Surgical Outcomes in the “Real World”: Efficacy vs. Effectiveness
Another natural application for the use of secondary databases is in the design of “real world” comparative effectiveness studies as opposed to efficacy studies. Efficacy studies are randomized controlled trials, generally regarded as the gold standard to practicing evidence-based medicine. These clinical trials are often performed in a narrow patient population with strict inclusion and exclusion criteria. They are also performed under ideal clinical conditions with close follow-up and ample hospital resources. These stringent criteria and ideal conditions can reduce type 1 error in randomized controlled trials. However, they threaten the external validity of the results. The large survival benefit of an intervention may not hold up in the real world, where patients and practice conditions are a lot messier. Because secondary databases tend to be population-based, these databases allow for assessment of outcomes in the real world. Wennberg et al. demonstrated this efficacy vs. effectiveness distinction examining carotid endarterectomy mortality using national Medicare data [4]. They report the mortality among Medicare beneficiaries undergoing carotid endarterectomy, a large heterogeneous cohort, was appreciably higher at low volume hospitals (2.5 %) compared to the higher volume hospitals (1.4 %) participating in two large well-designed randomized controlled trials: the North American Symptomatic Carotid Endarterectomy Trial (NASCET) and the Asymptomatic Carotid Atherosclerosis Study (ACAS).
13.2.4 Studying Outcomes Across Diverse Practice Settings
Health services researchers also use secondary databases to explore variation in outcomes across diverse practices settings. Studies can be designed to explore the effect of hospital volume or patient demographics (age, gender, race, socioeconomic status) on outcomes. Birkmeyer et al. used national Medicare data to definitively establish the inverse relationship between hospital volume and surgical mortality among six major cardiovascular procedures and eight major cancer resections [5]. Most case reports on complex operations like pancreatic resection are from high-volume institutions. Lower-volume centers would not be represented in the literature without large database studies. This study has since inspired a multitude of other studies exploring the volume-outcome relationship in many other procedures.
Studies using secondary datasets have also examined surgeon and/or hospital characteristics and their impact on patient outcomes. Ghaferi et al. used the NSQIP database to explore the variation in hospital mortality associated with inpatient general and vascular surgery [6]. To study variations in mortality and other patient outcomes, a broad sample of hospitals is required. Without secondary data, it would not be feasible to compare outcomes across hospitals and draw meaningful inferences. Using a large clinical registry, Ghaferi et al. were able to compare low-mortality and high-mortality hospitals and discovered similar complication rates. The distinction in low-mortality hospitals was their superior ability to prevent mortality in patients experiencing complications.
13.2.5 Evaluating Health Care Policy
As discussed above, there are many instances in which performing a randomized controlled trial for an intervention would be impractical, and using a large secondary database is the only option to assess outcomes. One example of this is in the evaluation of the impact of large-scale policy changes such as Medicare’s decision to restrict coverage of bariatric surgery to hospitals designated as centers of excellence. With such a large-scale policy decision, it would be infeasible to conduct a randomized controlled trial to evaluate this policy. Dimick et al. used the State Inpatient Database (SID) from 12 states to compare center of excellence hospitals and non-center of excellence hospitals using a difference-in-differences analytic approach incorporating existing time trends to demonstrate no benefit in rates of complications or reoperation after the enactment of this policy [7]. This is discussed in detail in elsewhere in this book.
13.3 Administrative Databases vs. Clinical Registries
13.3.1 Strengths and Weaknesses of Administrative Data
Administrative databases offer several advantages in health services research (Table 13.2). These data sets are population based, allowing for the examination of time trends or regional differences as well as real world outcomes, and have a large sample size, allowing for the study of rare conditions, as discussed above. These datasets are also relatively inexpensive and readily available. Therefore, administrative data are a good source of preliminary data for a grant. Another benefit of administrative data is the ability to link patients across episodes of care and institutions. For example, this allows for more accurate tracking of readmissions than clinical data, which often relies on hospitals to keep track of their own readmissions. This may be easy enough if a patient is readmitted to the same surgeon or service, but more complicated if a patient is readmitted to a different provider or service within the same hospital, or a completely different hospital altogether.
Table 13.2
Strengths and weakness of administrative databases and clinical registries
Administrative databases | |
---|---|
Strength | Weakness |
Population-based | Lack clinical detail |
Inexpensive, readily available | Inaccurate/variable coding |
Linkable across episodes | Lags in availability |
Large sample size | Collected for billing purposes |
Clinical registries | |
Strength | Weakness |
Clinical granularity | Lags in availability |
Large sample size | Relies on abstractors for reliability |
Resource-intensive |
The primary weaknesses in administrative data lie in the inaccuracy and variability in coding. Administrative databases were developed primarily for billing purposes. As a result, primary procedure and diagnosis codes, demographics, length-of-stay and outcomes such as mortality and readmission are recorded with good accuracy. However, administrative data often lack clinical granularity beyond these data points. Patient comorbidities, often used to adequately risk-adjust patients, rely on the varying quality of secondary diagnoses coding. Furthermore, the coding of complications, an important outcome measure in surgery, has also been criticized for clinical inaccuracy. The surgical health services researcher must have effective strategies to address these weaknesses as discussed below.
13.3.2 Identifying Comorbidities in Administrative Data
Popular methods to perform comorbidity risk adjustment include the Charlson Index and the Elixhauser method [8, 9]. The Charlson Index assigns a specific point value for certain comorbidities to predict 10-year survival. It has been adapted for use with International Classification of Disease, 9th Revision, Clinical Modification (ICD-9-CM) codes by various groups, with the most popular proposed by Deyo et al. [10]. The Elixhauser method uses a list of 30 comorbidities that can be identified by ICD-9-CM codes. These comorbidities are not simplified by an index, as each comorbidity can affect outcomes differently among different patient groups. Rather, each comorbidity is used as an indicator variable in logistic regression when performing risk adjustment. Both methods for risk adjustment are widely used and have been previously validated for use with administrative data. However, limitations still exist in using these methods. Though both the Elixhauser method and Charlson Index can discern the presence of comorbidities, the severity of comorbidities is not discernable. Also, in situations where confounding by indication based on clinical severity are important, administrative data may not adequately identify patients with higher clinical severity. Administrative data will not yield the appropriate clinical detail to perform rigorous comparative effectiveness studies without a more advanced method for causal inference.
13.3.3 Identifying Complications in Administrative Data
The Complications Screening Program developed by Iezzoni et al. is commonly used to identify complications from administrative data [11]. Using specific ICD-9-CM codes, the following postoperative complications can be identified: pulmonary failure, pneumonia, myocardial infarction, deep venous thrombosis/pulmonary embolism, acute renal failure, hemorrhage, surgical site infection, and gastrointestinal hemorrhage. This method has also been previously validated by chart review and shown to be in good agreement. Again, however, we caution the reader in using these methods in studies for which other complications not targeted in the Complications Screening Program (e.g. anastomotic leak, urinary tract infection, central line associated blood stream infection, etc.) may be important outcome measures. Furthermore, the severity of these complications cannot be ascertained with administrative data.
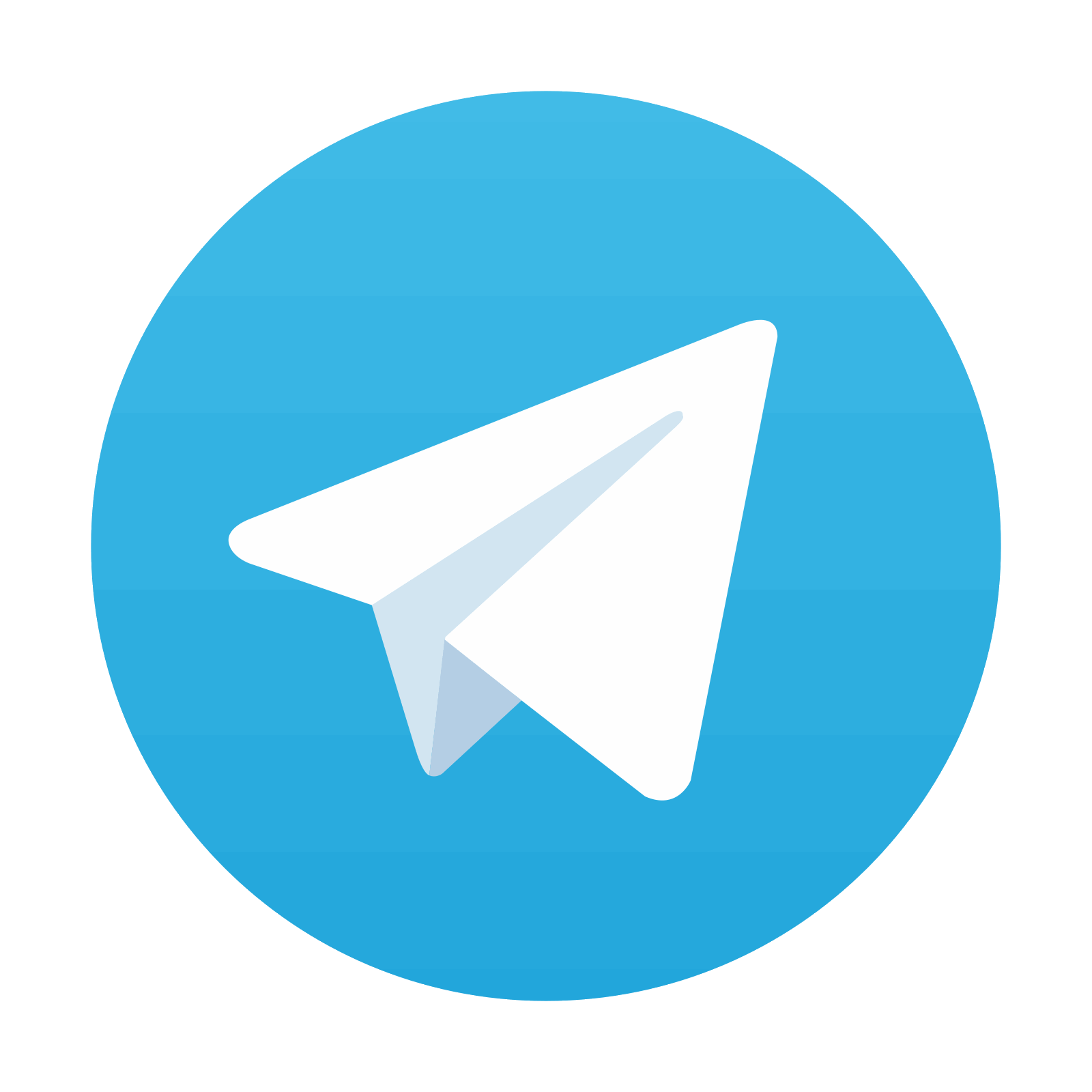
Stay updated, free articles. Join our Telegram channel

Full access? Get Clinical Tree
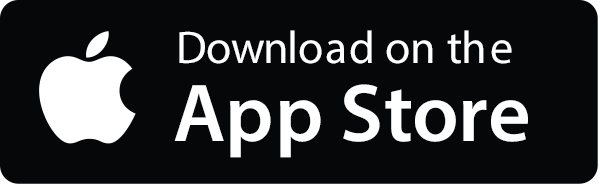
