Chapter 24 ISD models typically divide the instructional design process into five phases: (1) analysis, (2) design, (3) development, (4) implementation, and (5) evaluation. In this so-called ADDIE model (see Fig. 24.1), the evaluation phase is mainly summative, while formative evaluation may be conducted during all phases. Though the model appears to be linear, it does not have to be followed rigidly. Often, the model is repeatedly used to develop related units of instruction (iteration), phases are skipped because particular information is already available (layers of necessity) or later phases provide inputs that make it necessary to reconsider earlier phases (zigzag design). It is thus best seen as a project management tool that helps designers think about the different steps that must be taken. Moreover, the ADDIE model does not suggest or follow specific learning theories: it can be used for all instructional design projects irrespective of the preferred learning paradigm. In the first phase of the ADDIE model (Fig. 24.1), the focus is on the analysis of the desired learning outcomes and on the analysis of fixed conditions. With regard to fixed conditions, analyses pertain to the analysis of the context (availability of equipment, time and money, culture, setting such as school, military or work organization, etc.), the analysis of the target group (prior knowledge, general schooling, age, learning styles, handicaps, etc.), and the analysis of tasks and subject matter (tools and objects required, conditions for performance, risks, etc.). Close to 100 ID models have been described in the literature (for overviews, see Reigeluth 1983, 1999, Reigeluth & Carr-Chellman 2009) and on the internet (see, e.g. www.instructionaldesign.org, http://carbon.ucdenver.edu/~mryder/itc/idmodels). ID models differ from each other in several dimensions. One dimension pertains to the learning paradigm they adhere to, which may reflect, for example, a behaviorist, cognitive or social-constructivist perspective. A second dimension, discussed in the next section, is between models directed at the level of message design, lesson design and course and curriculum design. A third dimension pertains to outcomes-based models and whole-task models. Outcomes-based models typically focus on one particular domain of learning, such as the cognitive domain, psychomotor domain or affective domain (Bloom 1956), which roughly corresponds with the triplet knowledge, skills and attitudes. In one particular domain of learning, desired outcomes are analyzed in terms of distinct objectives or learning goals, after which instructional strategies are selected for reaching each of the separate objectives. Gagné (1985) introduced a widely used taxonomy in the cognitive domain. His taxonomy makes a distinction between verbal information, intellectual skills, cognitive strategies, attitudes and psychomotor skills. The intellectual skills are at the heart of the taxonomy and include five subcategories: Many researchers introduced alternative classifications of objectives. But a common premise of all outcomes-based models is that different objectives can best be reached by the application of particular instructional strategies (the conditions of learning; Gagné 1985). The optimal strategy is chosen for each objective; the objectives are usually taught one by one and the overall educational goal is believed to be met after all separate objectives have been taught. For instance, if complex skills or professional competences are taught, each objective corresponds with one enabling or constituent skill, and sequencing the objectives naturally results in a part-task sequence. Thus, the learner is taught only one or a very limited number of constituent skills at the same time. New constituent skills are gradually added to practice, and it is not until the end of the instruction – if at all – that the learner has the opportunity to practise the whole complex skill. Outcomes-based instructional design models are very effective for teaching objectives that have little to do with each other, that is, require little coordination. But in the early 1990s, authors in the field of instructional design started to question the value of outcomes-based models for reaching ‘integrative’ goals or objectives (e.g. Gagné & Merrill 1990). For complex skills or professional competencies, which are dominant in the medical domain, there are many interactions between the different aspects of task performance and their related objectives: with high demands on coordination. Then, an outcomes-based approach yields instruction that is fragmented and piecemeal and thus does not work. Whole-task models provide an alternative because they pay explicit attention to the coordination of all task aspects. Whole-task models explicitly aim at integrative goals, or complex learning. They take a holistic rather than atomistic perspective on instructional design (van Merriënboer 1997). First, complex contents and tasks are not split over different domains of learning (e.g. knowledge is taught in lectures, skills are taught in a skills lab and attitudes are taught in role plays), but knowledge, skills and attitudes are developed simultaneously by having the learners work on whole, integrative tasks. Second, complex contents and tasks are not reduced into simpler elements up to a level where the single elements (i.e. isolated objectives) can be transferred to learners through presentation and/or practice, but they are taught from simple-to-complex wholes in such a way that relationships between the elements are retained. Thus, whole-task models basically try to deal with complexity without losing sight of the relationships between elements. Rather than starting from a specification of objectives, instructional design starts with the identification of a representative set of real-life tasks and an analysis of the cognitive schemas that people need in order to perform those tasks (also called cognitive task analysis or CTA; Clark et al 2008). Cognitive schemas can be seen as the building blocks of cognition and integrate knowledge, skills and attitudes. The process of competence development can be described as the construction and automation of increasingly more complex cognitive schemas. Subprocesses of schema construction are inductive learning and elaboration. Learners induce new cognitive schemas and modify existing ones as a result of their concrete experiences with a varied set of tasks. They elaborate their cognitive schemas by connecting newly presented information to the things they already know.
Instructional design
The ADDIE model
The universe of ID models
Outcomes-based models
Whole-task models
Stay updated, free articles. Join our Telegram channel
Full access? Get Clinical Tree
Instructional design
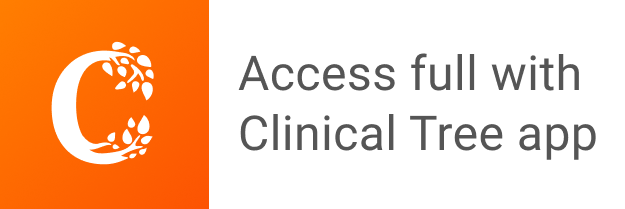