We often gather sample data in order to assess how much evidence there is against a specific hypothesis about the population. When performing descriptive analyses (Chapters 4–6) we may see trends that appear to support or refute this hypothesis. However, we do not know if these trends reflect real associations or are simply a result of random fluctuations caused by the variability present in any data set. We use a process known as hypothesis testing (or significance testing) to quantify our belief against a particular hypothesis.
This chapter describes the format of hypothesis testing in general; details of specific hypothesis tests are given in subsequent chapters. For easy reference, each hypothesis test is contained in a similarly formatted box.
Defining the Null and Alternative Hypotheses
We usually test the null hypothesis (H0) which assumes no effect (e.g. the difference in means equals zero) in the population. For example, if we are interested in comparing smoking rates in men and women in the population, the null hypothesis would be:
We then define the alternative hypothesis (H1) which holds if the null hypothesis is not true. The alternative hypothesis relates more directly to the theory we wish to investigate. So, in the example, we might have:
We have not specified any direction for the difference in smoking rates, i.e. we have not stated whether men have higher or lower rates than women in the population. This leads to what is known as a two-tailed test because we allow for either eventuality, and is recommended as we are rarely certain, in advance, of the direction of any difference, if one exists. In some, very rare, circumstances, we may carry out a one-tailed test in which a direction of effect is specified in H1. This might apply if we are considering a disease from which all untreated individuals die (a new drug cannot make things worse) or if we are conducting a trial of equivalence or non-inferiority (see last section in this chapter).
Obtaining the Test Statistic
After collecting the data, we substitute values from our sample into a formula, specific to the test we are using, to determine a value for the test statistic. This reflects the amount of evidence in the data against the null hypothesis – usually, the larger the value, ignoring its sign, the greater the evidence.
Obtaining the P-Value
All test statistics follow known theoretical probability distributions (Chapters 7 and 8). We relate the value of the test statistic obtained from the sample to the known distribution to obtain the P-value, the area in both (or occasionally one) tails of the probability distribution. Most computer packages provide the two-tailed P-value automatically. The P-value is the probability of obtaining our results, or something more extreme, if the null hypothesis is true. The null hypothesis relates to the population of interest, rather than the sample. Therefore, the null hypothesis is either true or false and we cannot interpret the P-value as the probability that the null hypothesis is true.
Using the P-Value
We must make a decision about how much evidence we require to enable us to decide to reject the null hypothesis in favour of the alternative. The smaller the P-value, the greater the evidence against the null hypothesis.
- Conventionally, we consider that if the P-value is less than 0.05, there is sufficient evidence to reject the null hypothesis, as there is only a small chance of the results occurring if the null hypothesis were true. We then reject the null hypothesis and say that the results are significant at the 5% level (Fig. 17.1).
- In contrast, if the P-value is equal to or greater than 0.05, we usually conclude that there is insufficient evidence to reject the null hypothesis. We do not reject the null hypothesis, and we say that the results are not significant at the 5% level (Fig. 17.1). This does not mean that the null hypothesis is true; simply that we do not have enough evidence to reject it.
Figure 17.1 Probability distribution of the test statistic showing a two-tailed probability, P = 0.05.

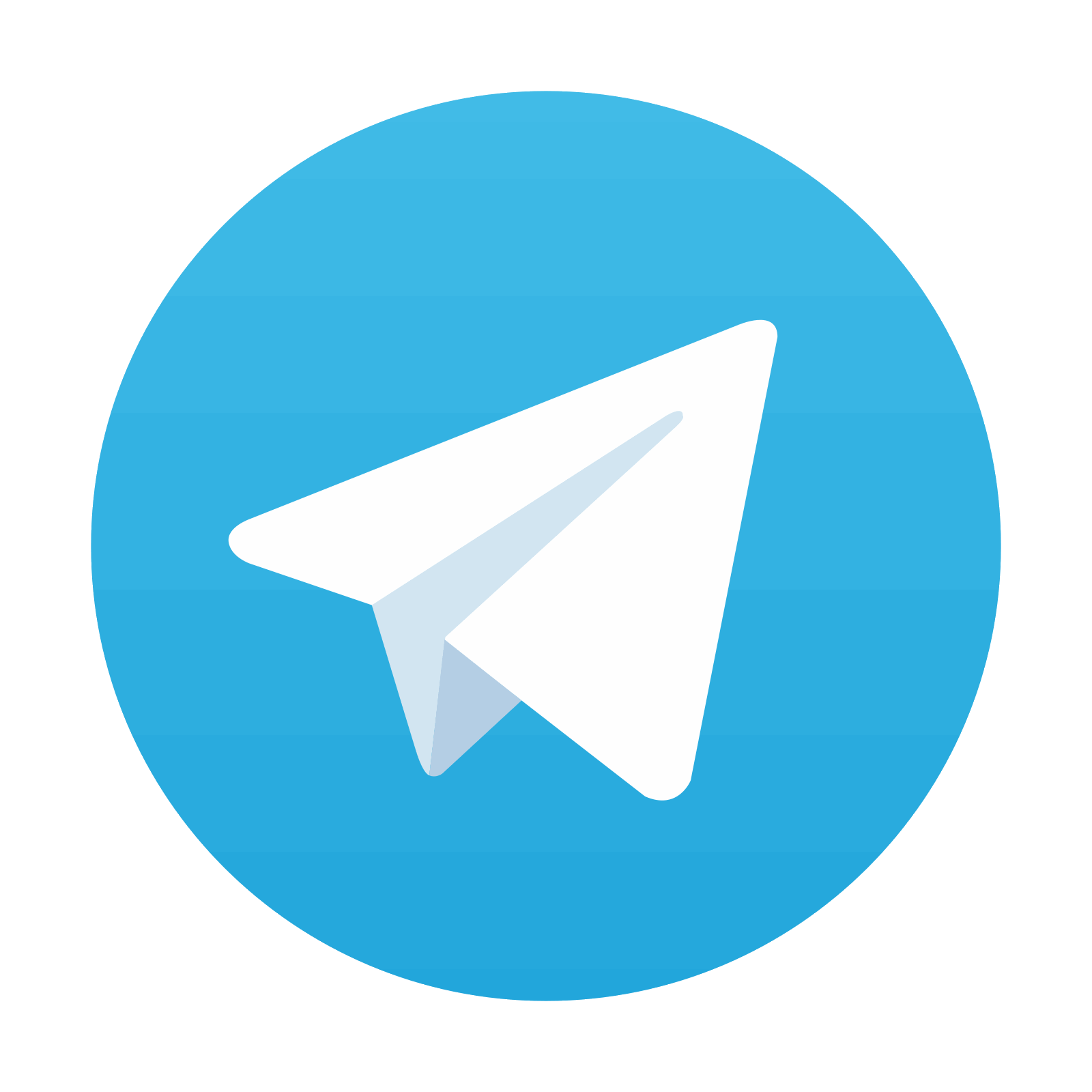
Stay updated, free articles. Join our Telegram channel

Full access? Get Clinical Tree
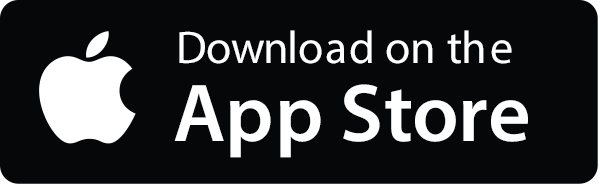
