Essential properties
Desirable properties
A well-defined and readily measurable endpoint (i.e. permeability, k p, or flux (J max or J ss)
Do not extrapolate or use the model beyond its original domain, both in terms of the range of physicochemical descriptors used or in terms of the class(es) of chemicals from which the model was developed
A data set that is chemically and biologically diverse and that is equally representative of the “chemical space” associated with the model; the data set should be split into suitably representative training and test sets, which are separate and consistent
The model should be correctly used and interpreted within the context of the model’s findings and of prior knowledge in the field
Physiochemical descriptors that are, in number and type, consistent with the skin permeability endpoint (i.e. molecular features that have been shown experimentally or empirically to be significant in the permeation process)
Models and methodologies should be transparent and not based on “black box” approaches
The method of analysis should be appropriate and produce a model that is statistically valid; statistical performance should be described for each mode
Appreciation of the precision of the model when interpreting its output (in the case of models featuring algorithms); mean and standard deviation values for each significant descriptor should be provided
The model and method should have a strong mechanistic basis
The use of a single model to describe a process, rather than a series of models to describe individual parts of the process
Development of models by multidisciplinary groups of experts
Quality of the Source, or Input, Data
The biological data for skin absorption, upon which current models are based, are usually either permeability or flux. This should not, however, rule out future developments where different endpoints may be used to predict optimum absorption in, for example, superficial tissues to improve the topical delivery of a range of drugs, including for example antifungals and anti-irritants. In contrast to the general picture presented by Cronin and Schultz, the field of percutaneous absorption uses well-defined measures of biological data (permeability data). The underlying issue that is more specific to studies of skin absorption is in the quality of the data used; in particular, the consistency of experimental protocols, which include everything from selection and quality of skin to the choice of formulation and receptor solvents, is central to the development of models of skin absorption. While understanding that a model should only be applied within its limits—including its experimental limits—a key recommendation for the future development of models of skin permeability is that they adopt a standardised protocol. Ideally, this would relate to a single study, which is unlikely, and the next best option—a consideration of the approach proposed by Chilcott et al. (2005) has been shown to provide little improvement in data quality or reproducibility. Nevertheless, as issues with Flynn’s (1990) data set have shown (i.e. Johnson et al. 1995; Moss and Cronin 2002) a reduction in the number of sources of data from which a model is constructed would appear to be central to producing a valid and reliable model. Thus, permeability data from which models are constructed should ideally come from a single protocol—and from the same laboratory, or workers, if possible—to reduce potential sources of error. The protocol should be well established and validated with clear and well-defined endpoints. As Cronin and Schultz pointed out, there are numerous examples of good practice in other fields that those developing models of percutaneous absorption could learn from in this regard; in these cases, the underlying issue is the development of an open-access database resource to which data points are added only if they meet the criteria described above. However, given that a large amount of the data already used for the development of models of skin permeability use published data from a variety of sources, this will—at worst—result in data that are not comparable and—at best—that exhibit substantial variation. While such data could be used for modelling, it should be used with caution, and an understanding of the limitations placed upon it by its source.
Such restrictions will be reflected in the poor statistical fit of any models thus derived. A number of suggestions for using data of perceived poor quality were discussed by Cronin and Schultz. The modeller should consider the nature of the data set, particularly its source(s) and quality, when considering the statistical quality of the resultant model. Models should, where possible, be developed empirically and be pragmatic; that is, models can be based on a small number of parameters of known significance from previous studies—perhaps echoing the use of log P and molecular weight by Flynn, and hydrogen bonding by Roberts and colleagues (Roberts et al. 1995, 1996; Pugh et al. 1996, 2000)—rather than analysing a large number of descriptors which are known to be largely irrelevant. They also recommend robust validation of the models using distinct training and test data sets.
Outliers
Cronin and Schultz also discussed outliers—compounds poorly predicted by a model—in the development of QSARs. Generally, outliers are identified by their lack of statistical fit, and it is usually inferred that this relates to a different mechanism of action than the rest of the data analysed that are not characterised as outliers. In the context of predictive models for skin permeation, the clear example of the revised Scheuplein data, which comprised almost 15 % of Flynn’s original data set, is significant (Scheuplein et al. 1969). This has been discussed previously in Chap. 4 but highlights the issue not only with identifying and selecting outliers, but also with the chosen method of analysis, which may result in misleading methods being used and which highlights the recommendations of Moss et al. (2009) to undertake rudimentary analyses of the data sets in order to characterise their fundamental nature (i.e. whether the data follows linear or nonlinear trends) so that the correct methods of analysis can be chosen.
Methods to highlight outliers include their identification based on their high standard residuals from regression-based techniques; following this, they are often removed individually either by subjective comment—perhaps informed by empirical insights of how, for example, a particular chemical might permeate the skin—or by whether they sit above or below an arbitrary cut-off point (i.e. a particular residual value returned from statistical analysis). When carried out correctly, the removal of outliers—and the identification of which chemicals were removed for this reason—will improve the quality and relevance of a model. In some cases, it may be relevant to analyse a model both before and after the removal of outliers, as those compounds which are genuine outliers will, if removed, result in a minimal change to the model. However, the situation with Johnson et al’s (1995) reanalysis of the Flynn data set, which highlights issues of data quality, should be borne in mind when considering such manipulations of the model.
Biological Data
Cronin and Schultz make the obvious, but often overlooked, comment that biological data are inherently variable and subject to error and that standard protocols may often be difficult to develop. In the case of skin absorption, while it is reasonable to comment that methods for the measurement of in vitro percutaneous absorption are reasonably standardised, significant differences do exist. As such experiments are the main source of data from which models are constructed, it is difficult to remove such variation from models of skin permeation. Therefore, models of skin permeation should be presented as mean values from a series of replicates, with the standard deviation or standard error also quoted—a good example of this practice is shown in the review by Geinoz et al. (2004). Thus, an extension of this consideration is that, once it is accepted that biological measurements are associated with error, it should be considered that certain protocols might result in more error than others. This may be considered when collating literature data into a single data set.
Descriptor Selection and Interpretation, and Data Set Design
In selecting which descriptors to use for an analysis, care should be taken to avoid repetition, colinearity and therefore relevance, which has been observed previously with topological indices such as molecular connectivity (Basak et al. 2000; Patel and Cronin 2001). To avoid such issues, any regression analysis that is based on multivariate methods should not be based on colinear descriptors, as this will result in an artificially high regression coefficient (Romanelli et al. 2000). This is achieved by the analysis of the correlation matrix output from any regression analysis, but decisions based on what is or is not an acceptable level of covariance are somewhat subjective but should be as low as possible, but must be significantly lower than the statistical fit of the model itself, and the r 2 value (adjusted for degrees of freedom) should be reported (Cronin and Schultz 2003).
While poor data set design can result in issues of colinearity and introduce bias into the data it is, in the case of percutaneous absorption, extremely difficult to find sufficient data in the literature to compile a data set that is completely without some form of bias. In particular, most data relate to chemicals that have low to intermediate lipophilicities (i.e. 1.0 < log P < 3.0, or MW < 500) and, as such, the range and relevance of any resultant models may be of limited value—this is discussed in detail in Chap. 9 (Moss et al. 2006).
More broadly, poor data set design may result in the inclusion of colinear descriptors which are of little relevance to the underlying mechanism of the process being modelled. This is more common with related chemicals (i.e. homologous series) and may be addressed by using as diverse a data set as available. This is, of course, somewhat idealistic, but it does highlight the relevance of models of skin permeability and their limitations. Statistical methods, including the use of principal component analysis (Moss et al. 2009; Sun et al. 2011) or correlation matrices and an examination of the intercorrelations between variables, should be assessed in any method to which such statistical measures are relevant. Thus, issues of colinearity may be reduced by the selection of relevant fundamental physicochemical descriptors which will allow clear and unambiguous mechanistic inferences to be drawn.
Statistical Analysis of Data
Although Cronin and Schultz (2003) focused on QSAR-based modelling approaches, their comments on statistical analysis are broadly relevant and impact on considerations of other methods. Over-fitting of data may be an issue which is related to the method of analysis, and potentially also of the variance associated with data of a biological origin. This is also an issue for nonlinear methods and was discussed in Chap. 7, where artificial neural networks in particular have been shown to over-fit data.
Nevertheless, most biological processes are inherently nonlinear, and, in the context of fundamental physicochemical parameters, it is clear that this is the case with skin absorption where both highly hydrophilic and highly hydrophobic chemicals are poor skin permeants. Thus, Cronin and Schultz commented that global modelling is unlikely to successful without some consideration of nonlinearity. This is perhaps reflected in the improved models obtained—compared to linear methods—when various researchers have employed nonlinear methods. These methods have been discussed in Chaps. 4–7.
However, one issue with some nonlinear methods—discussed in Chap. 7—is their inherent lack of transparency. Thus, while machine learning and related methods currently appear to offer improved predictions of percutaneous absorption, they lack transparency and have limited portability. It may therefore be the case that despite advances described in Chap. 7, the modeller should use the most transparent, portable and readily interpretable method available as it may offer greater utility, particularly in terms of mechanistic insight, than “better” models.
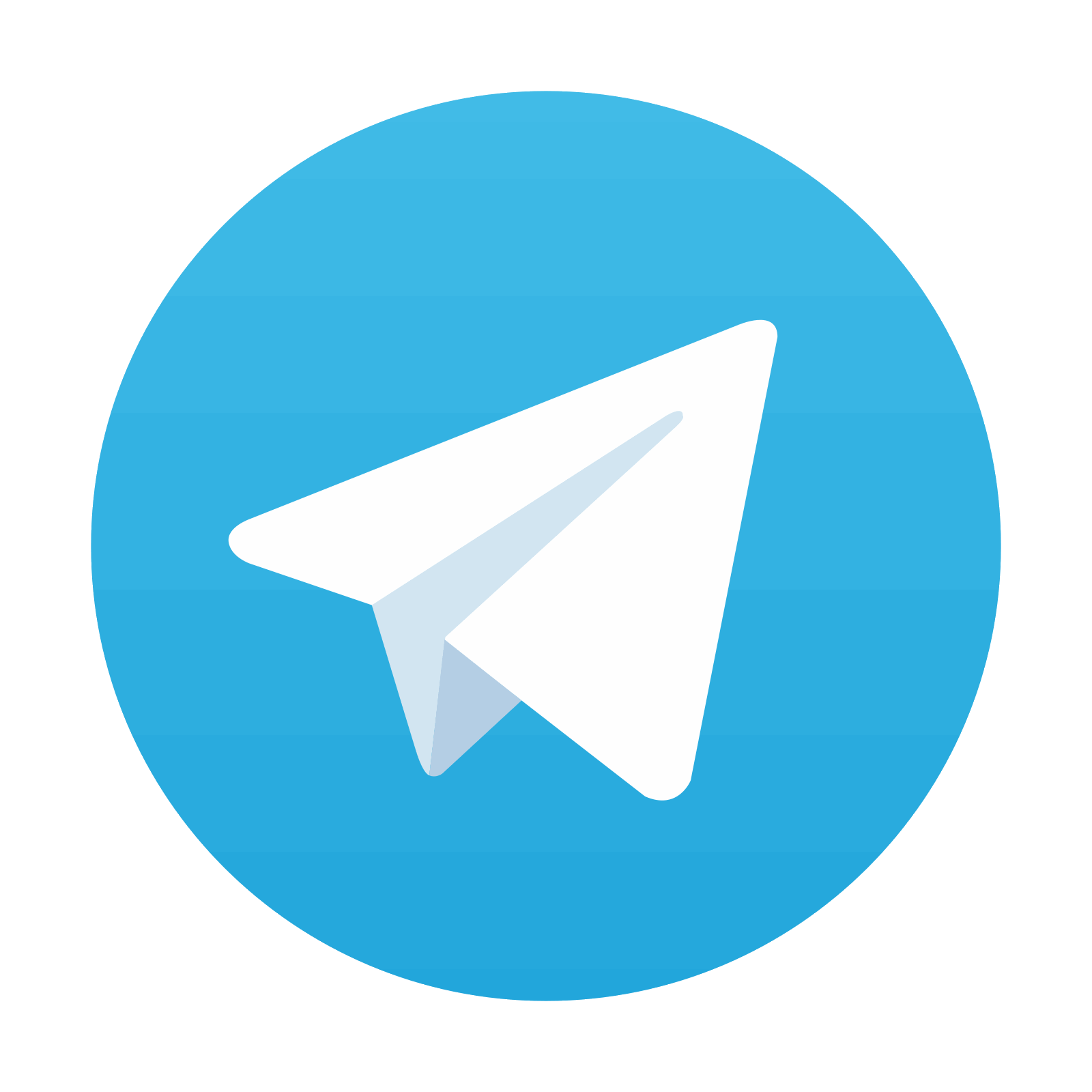
Stay updated, free articles. Join our Telegram channel

Full access? Get Clinical Tree
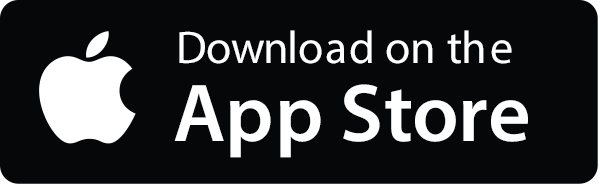
