Chapter 20 Clinical reasoning in medicine
How do physicians solve diagnostic problems? What is known about the process of diagnostic clinical reasoning? In this chapter we sketch our current understanding of answers to these questions by reviewing the cognitive processes and mental structures employed in diagnostic reasoning in clinical medicine and offering a selected history of research in the area. We will not consider the parallel issues of selecting a treatment or developing a management plan. For theoretical background, we draw upon two approaches that have been particularly influential in research in this field. The first is problem solving, exemplified in the work of Newell & Simon (1972), Elstein et al (1978), Bordage and his colleagues (Bordage & Lemieux 1991, Bordage & Zacks 1984, Friedman et al 1998, Lemieux & Bordage 1992) and Norman (2005). The second is decision making, including both classical and two-system approaches, illustrated in the work of Kahneman et al (1982), Baron (2000), and the research reviewed by Mellers et al (1998), Shafir & LeBoeuf (2002) and Kahneman (2003).
Problem-solving research has usually focused on how an ill-structured problem situation is defined and structured (as by generating a set of diagnostic hypotheses). Psychological decision research has typically looked at factors affecting diagnosis or treatment choice in well defined, tightly controlled situations. A common theme in both approaches is that human rationality is limited. Nevertheless, researchers within the problem-solving paradigm have concentrated on identifying the strategies of experts in a field, with the aim of facilitating the acquisition of these strategies by learners. Behavioural decision research, on the other hand, contrasts human performance with a normative statistical model of reasoning under uncertainty. It illuminates cognitive processes by examining errors in reasoning to which even experts are not immune, and thus raises the case for decision support.
PROBLEM SOLVING: DIAGNOSIS AS HYPOTHESIS SELECTION
THE HYPOTHETICO-DEDUCTIVE METHOD
Early hypothesis generation and selective data collection
Elstein et al (1978) found that diagnostic problems are solved by a process of generating a limited number of hypotheses or problem formulations early in the workup and using them to guide subsequent data collection and integration. Each hypothesis can be used to predict what additional findings ought to be present if it were true, and then the workup is a guided search for these findings; hence, the method is hypothetico-deductive. The process of problem structuring via hypothesis generation begins with a very limited data set and occurs rapidly and automatically, even if clinicians are explicitly instructed not to generate hypotheses. Given the complexity of the clinical situation, the enormous amount of data that could potentially be obtained and the limited capacity of working memory, hypothesis generation is a psychological necessity. Novices and experienced physicians alike attempt to generate hypotheses to explain clusters of findings, although the content of the experienced group’s productions is of higher quality.
Other clinical researchers have concurred with this view (Kassirer & Gorry 1978, Kuipers & Kassirer 1984, Nendaz et al 2005, Pople 1982). It has also been favoured by medical educators (e.g. Barrows & Pickell 1991, Kassirer & Kopelman 1991), while researchers in cognitive psychology have been more sceptical. We will examine these conflicting interpretations later.
Data collection and interpretation
A clinician could collect data quite thoroughly but could nevertheless ignore, misunderstand or misinterpret a significant fraction. In contrast, a clinician might be overly economical in data collection but could interpret whatever is available quite accurately. Elstein et al (1978) found no statistically significant association between thoroughness of data collection and accuracy of data interpretation. This was an important finding for two reasons:
1. Increased emphasis upon interpretation of data. Most early research allowed subjects to select items from a large array or menu of items. This approach, exemplified in patient management problems (Feightner 1985), facilitated investigation of the amount and sequence of data collection but offered less insight into data interpretation and problem formulation. The use of standardized patients (SPs) (Swanson et al 1995, van der Vleuten & Swanson 1990) offers researchers considerable latitude in how much to focus the investigation (or student assessment) on data collection or on hypothesis generation and testing. To deepen understanding of reasoning processes, investigators in the problem-solving tradition have asked subjects to think aloud while problem solving and have then analysed their verbalizations as well as their data collection (Barrows et al 1982, Elstein et al 1978, Friedman et al 1998, Joseph & Patel 1990, Nendaz et al 2005, Neufeld et al 1981, Patel & Groen 1986). Considerable variability in acquiring and interpreting data has been found, increasing the complexity of the research task. Consequently, some researchers switched to controlling the data presented to subjects in order to concentrate on data interpretation and problem formulation (e.g. Feltovich et al 1984, Kuipers et al 1988). This shift led naturally to the second major change in research tactics.
2. Study of clinical judgement separated from data collection. Controlling the database facilitates analysis at the price of fidelity to clinical realities. This strategy is the most widely used in current research on clinical reasoning, the shift reflecting the influence of the paradigm of decision-making research. Sometimes clinical information is presented sequentially to a subject, so that the case unfolds in a simulation of real time, but the subject is given few or no options in data collection (Chapman et al 1996). The analysis can focus on memory organization, knowledge utilization, data interpretation or problem representation (e.g. Bordage & Lemieux 1991, Groves et al 2003, Joseph & Patel 1990, Moskowitz et al 1988). In other studies, clinicians are given all the data at once and asked to make a diagnostic or treatment decision (Elstein et al 1992, Patel & Groen 1986).
Case specificity
Problem-solving expertise varies greatly across cases and is highly dependent on the clinician’s mastery of the particular domain. Differences between clinicians are to be found more in their understanding of the problem and their problem representations than in the reasoning strategies employed (Elstein et al 1978). Thus it makes more sense to talk about reasons for success and failure in a particular case than about generic traits or strategies of expert diagnosticians.
For evaluators in medical and other health professional education, this finding raises the practical problem of how many case simulations are needed to make a case-based examination a reliable and valid assessment of problem-solving skill. Test developers are now much more concerned about the number and content of clinical simulations in an examination than they were prior to this discovery (e.g. Page et al 1990, van der Vleuten & Swanson 1990).
DIAGNOSIS AS CATEGORIZATION OR PATTERN RECOGNITION
The finding of case specificity also challenged the hypothetico-deductive model as an adequate account of the process of clinical reasoning. Both successful and unsuccessful diagnosticians employed a hypothesis-testing strategy, and diagnostic accuracy depended more on mastery of the content in a domain than on the strategy employed. By the mid-1980s, the view of diagnostic reasoning as complex and systematic generation and testing of hypotheses was being criticized. Patel, Norman and their associates (e.g. Brooks et al 1991, Eva et al 1998, Groen & Patel 1985, Schmidt et al 1990) pointed out that the clinical reasoning of experts in familiar situations frequently does not display explicit hypothesis testing. It is rapid, automatic and often non-verbal. Not all cases seen by an experienced physician appear to require hypothetico-deductive reasoning (Davidoff 1996).
Expert reasoning in familiar situations looks more like pattern recognition or direct automatic retrieval from a well-structured network of stored knowledge (Groen & Patel 1985). Since experienced clinicians have a better sense of clinical realities and the likely diagnostic possibilities, they can also more efficiently generate an early set of plausible hypotheses to avoid fruitless and expensive pursuit of unlikely diagnoses. The research emphasis has shifted from the problem-solving process to the organization of knowledge in the long-term memory of experienced clinicians (Norman 1988).
Categorization of a new case can be based either on retrieval of and matching to specific instances or examplars, or to a more abstract prototype. In instance-based recognition, a new instance is classified by resemblance to memory of a past case (Brooks et al 1991, Medin & Schaffer 1978, Norman et al 1992, Schmidt et al 1990). This model is supported by the finding that clinical diagnosis is strongly affected by the context of events (for example the location of a skin rash on the body), even when this context is normatively irrelevant. Expert–novice differences are mainly explicable in terms of the size of the knowledge store of prior instances available for pattern recognition. This theory of clinical reasoning has been developed with particular reference to pathology, dermatology and radiology, where the clinical data are predominantly visual.
According to prototype models, clinical experience facilitates the construction of abstractions or prototypes (Bordage & Zacks 1984, Rosch & Mervis 1975). Better diagnosticians have constructed more diversified and abstract sets of semantic relations to represent the links between clinical features or aspects of the problem (Bordage & Lemieux 1991, Lemieux & Bordage 1992). Experts in a domain are more able to relate findings to each other and to potential diagnoses, and to identify what additional findings are needed to complete a picture (Elstein et al 1993). These capabilities suggest that experts are working with more abstract representations and are not simply trying to match a new case to a previous instance, although that matching process may occur with simple cases.
MULTIPLE REASONING STRATEGIES
Norman et al (1994) found that experienced physicians used a hypothetico-deductive strategy with difficult cases only, a view supported by Davidoff (1996). When a case is perceived to be less challenging, quicker and easier methods are used, such as pattern recognition or feature matching. Thus, controversy about the methods used in diagnostic reasoning can be resolved by positing that the method selected depends upon perceived characteristics of the problem. There is an interaction between the clinician’s level of skill and the perceived difficulty of the task (Elstein 1994). Easy cases are solved by pattern recognition and going directly from data to diagnostic classification – what Groen & Patel (1985) called forward reasoning. Difficult cases need systematic hypothesis generation and testing. Whether a problem is easy or difficult depends in part on the knowledge and experience of the clinician who is trying to solve it (Figure 20.1).
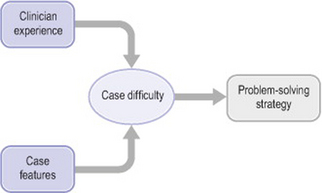
Figure 20.1 Impact on problem-solving strategy of case difficulty, clinician experience and case features
Both Norman and Eva have since championed the view that clinicians apply multiple reasoning strategies as necessary to approach diagnostic problems (Eva 2005, Norman 2005). Their research suggests that training physicians in multiple knowledge representations and reasoning modes may yield the best overall performance.
ERRORS IN HYPOTHESIS-GENERATION AND RESTRUCTURING
Neither pattern recognition nor hypothesis testing is an error-proof strategy, nor are they always consistent with statistical rules of inference with imperfect information. Errors that can occur in difficult cases in internal medicine were illustrated and discussed by Kassirer & Kopelman (1991) and classes of error were reviewed by Graber et al (2002). The frequency of errors in actual practice is unknown, but considering a number of studies as a whole, an error rate of 15% might be a good first approximation.
Looking at an instance of diagnostic reasoning retrospectively, it is easy to see that a clinician could err either by oversimplifying a complex problem or by taking a problem that could appropriately have been dealt with routinely and using a more effortful strategy of competing hypotheses. It has been far more difficult for researchers and teachers to prescribe an appropriate strategy in advance. Because so much depends on the interaction between case and clinician, prescriptive guidelines for the proper amount of hypothesis generation and testing are still unavailable for the student clinician. Perhaps the most useful advice is to emulate the hypothesis-testing strategy used by experienced clinicians when they are having difficulty, since novices will experience as problematic many situations that the former solve by routine pattern-recognition methods. In an era that emphasizes cost-effective clinical practice, gathering data unrelated to diagnostic hypotheses will be discouraged.
Many diagnostic problems are so complex that the correct solution is not contained within the initial set of hypotheses. Restructuring and reformulating must occur through time as data are obtained and the clinical picture evolves. However, as any problem solver works with a particular set of hypotheses, psychological commitment takes place and it becomes more difficult to restructure the problem (Janis & Mann 1977). Ideally, one might want to work purely inductively, reasoning only from the facts, but this strategy is never employed because it is inefficient and produces high levels of cognitive strain (Elstein et al 1978). It is much easier to solve a problem where some boundaries and hypotheses provide the needed framework. On the other hand, early problem formulation may also bias the clinician’s thinking (Barrows et al 1982, Voytovich et al 1985). Errors in interpreting the diagnostic value of clinical information have been found by several research teams (Elstein et al 1978, Friedman et al 1998, Gruppen et al 1991, Wolf et al 1985).
DECISION MAKING: DIAGNOSIS AS OPINION REVISION
BAYES’ THEOREM
In the literature on medical decision making, reaching a diagnosis is conceptualized as a process of reasoning about uncertainty, updating an opinion with imperfect information (the clinical evidence). As new information is obtained, the probability of each diagnostic possibility is continuously revised. Each post-test probability becomes the pre-test probability for the next stage of the inference process. Bayes’ theorem, the formal mathematical rule for this operation (Hunink et al 2001, Sox et al 1988), states that the post-test probability is a function of two variables, pre-test probability and the strength of the new diagnostic evidence. The pre-test probability can be either the known prevalence of the disease or the clinician’s belief about the probability of disease before new information is acquired. The strength of the evidence is measured by a likelihood ratio, the ratio of the probabilities of observing a particular finding in patients with and without the disease of interest. This framework directs attention to two major classes of errors in clinical reasoning: errors in a clinician’s beliefs about pre-test probability or errors in assessing the strength of the evidence. Bayes’ theorem is a normative rule for diagnostic reasoning; it tells us how we should reason, but it does not claim that we actually revise our opinions in this way. Indeed, from the Bayesian viewpoint, the psychological study of diagnostic reasoning centres on errors in both components, which are discussed below (Kempainen et al 2003 provide a similar review).
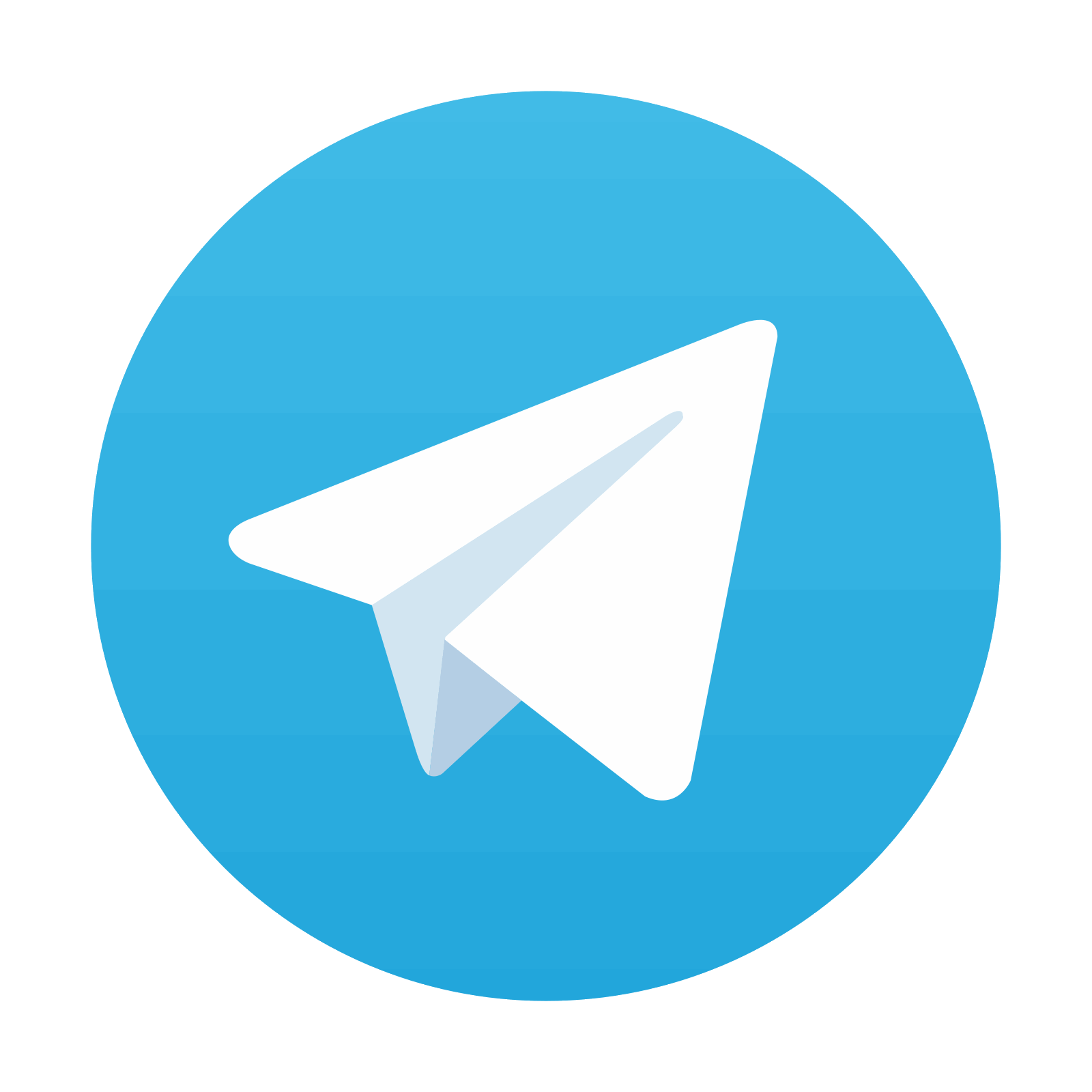
Stay updated, free articles. Join our Telegram channel

Full access? Get Clinical Tree
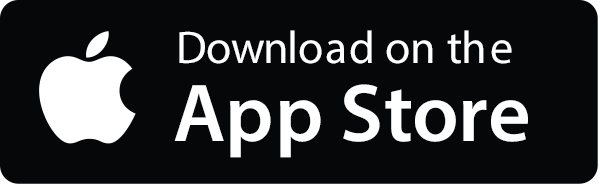
