In many cases, despite all of our efforts to design a robust study and perform appropriate statistical analyses, the results from our study may not accurately reflect the true situation. This may be due to the presence of bias which can be introduced at any stage of the study, perhaps resulting from a failure to take account of important exposure (explanatory) variables.
Bias
What is It?
Bias is said to have occurred when there is a systematic difference between the results from a study and the true state of affairs. Bias may be introduced at all stages of the research process, from study design, through to analysis and publication. Bias can create a spurious association (i.e. overestimation of an effect) or mask a real one (underestimation of an effect). While appropriate statistical methods can reduce the effect of bias, they may not be able to eliminate it entirely. It is thus preferable to design a study so that bias is minimized (e.g. by taking steps to reduce recall bias in a case–control study, or by attempting to minimize loss-to-follow-up in a longitudinal study). It should be noted that increasing the sample size does not reduce bias – if anything, increasing the sample size might actually increase the impact of bias.
We have already described the biases that are most commonly encountered in clinical trials (Chapter 14), case–control studies (Chapter 15) and cohort studies (Chapter 16). However, there are many forms of bias1 which may broadly be categorized as forms of either selection or information bias. A third type of bias, caused by confounding, is discussed in the next section. Even if obvious sources of bias have been addressed, funding bias, whereby there is a tendency to report findings in the direction favoured by the funding body (such as a pharmaceutical company), and publication bias, whereby there is a tendency to publish only those papers that report positive or topical results, may mean that the results from publicly available studies are still misleading.
Selection Bias
Selection bias occurs when patients included in the study are not representative of the population to which the results will be applied, e.g. patients who agree to participate in a study may differ from those who do not agree to participate (this form of bias is a particular problem in retrospective studies when patients who have died are not included in the study). Selection bias includes the following:
- Ascertainment bias may occur when the sample included in a study is not randomly selected from the population and differs in some important respects from that population, e.g. when doctors interested in the genetics of a particular medical condition collect information on the patients in their clinic, rather than using a random sample from the population.
- Attrition bias arises when those who are lost to follow-up in a longitudinal study (Chapter 12) differ in a systematic way from those who are not lost to follow-up.
- The healthy entrant effect occurs where mortality and morbidity rates are lower in the initial stages of a longitudinal study than in the general population because the individuals included in the study are disease-free at its outset (Chapter 15).
- Response bias is caused by differences in characteristics between those who choose or volunteer to participate in a study and those who do not.
- Survivorship bias occurs when survival is compared in patients who do or who do not receive a particular intervention where this intervention only became available at some point after the start of the study so that patients have to survive long enough to be eligible to receive the intervention.
Information Bias
Information bias occurs during data collection when measurements on exposure and/or disease outcome are incorrectly recorded in a systematic manner. Information bias includes the following:
- Central tendency bias often arises when using a Likert scale (comprising a small number of graded alternative responses such as very poor, poor, no opinion, good, excellent) where responders tend to move towards the mid-point of the scale (usually ‘no opinion’ or ‘just right’).
- Lead-time bias occurs particularly in studies assessing changes in survival over time where the development of more accurate diagnostic procedures may mean that patients entered later into the study are diagnosed at an earlier stage in their disease, resulting in an apparent increase in survival from the time of diagnosis.
- Measurement bias arises when a systematic error is introduced by an inaccurate measurement tool (e.g. a set of poorly calibrated scales); it may also be introduced by digit preference or rounding error.
- Misclassification bias occurs when we incorrectly classify a categorical exposure and/or outcome variable. This may dilute or exaggerate the effect of interest, depending on whether the misclassification occurs equally in all groups or varies according to exposure group.
- Observer bias occurs when one observer tends to under-report (or over-report) a particular variable; also called assessment bias.
- Regression dilution bias may occur when fitting a regression model to describe the association between an outcome variable and one or more exposure variable(s). If there is substantial measurement error (Chapter 39) around one of the exposure variables, the associated regression parameter from the model may be attenuated.
- Reporting bias occurs when participants give answers in the direction they perceive are of interest to the researcher or under-report socially unacceptable or embarrassing behaviours or disorders (e.g. alcohol consumption or sexually transmitted disease).
Regression to the mean occurs where measurements that follow particularly low measurements tend to be higher than those recorded previously, and those that follow particularly high measurements tend to be lower (Chapter 27).
The ecological fallacy results in a bias which sometimes occurs when we reach conclusions based solely on aggregate statistics for groups within a population. We believe mistakenly that an association that we observe between variables at an aggregate level reflects the corresponding association at an individual level in the same population. This is particularly relevant when we do not have the necessary information about a variable at the patient level but only at the study level (e.g. in a meta-analysis, Chapter 43), and is common in ecological studies where we note associations between the level of disease in a population (often an entire country) which are not apparent when we consider the association at the individual level. For example, living in a more deprived area has been shown2 to be associated with an increased likelihood of being diagnosed with Stage III or IV breast cancer, but since the study used an area-based measure of low socioeconomic background, these results cannot be extended to individual women living in the area. The ecological fallacy is particularly true in meta-regression (Chapter 43).
Confounding
What Is It?
Confounding occurs when we find a spurious association between a potential risk factor and a disease outcome or miss a real association between them because we have failed to adjust for any confounding variables. A confounding variable or confounder
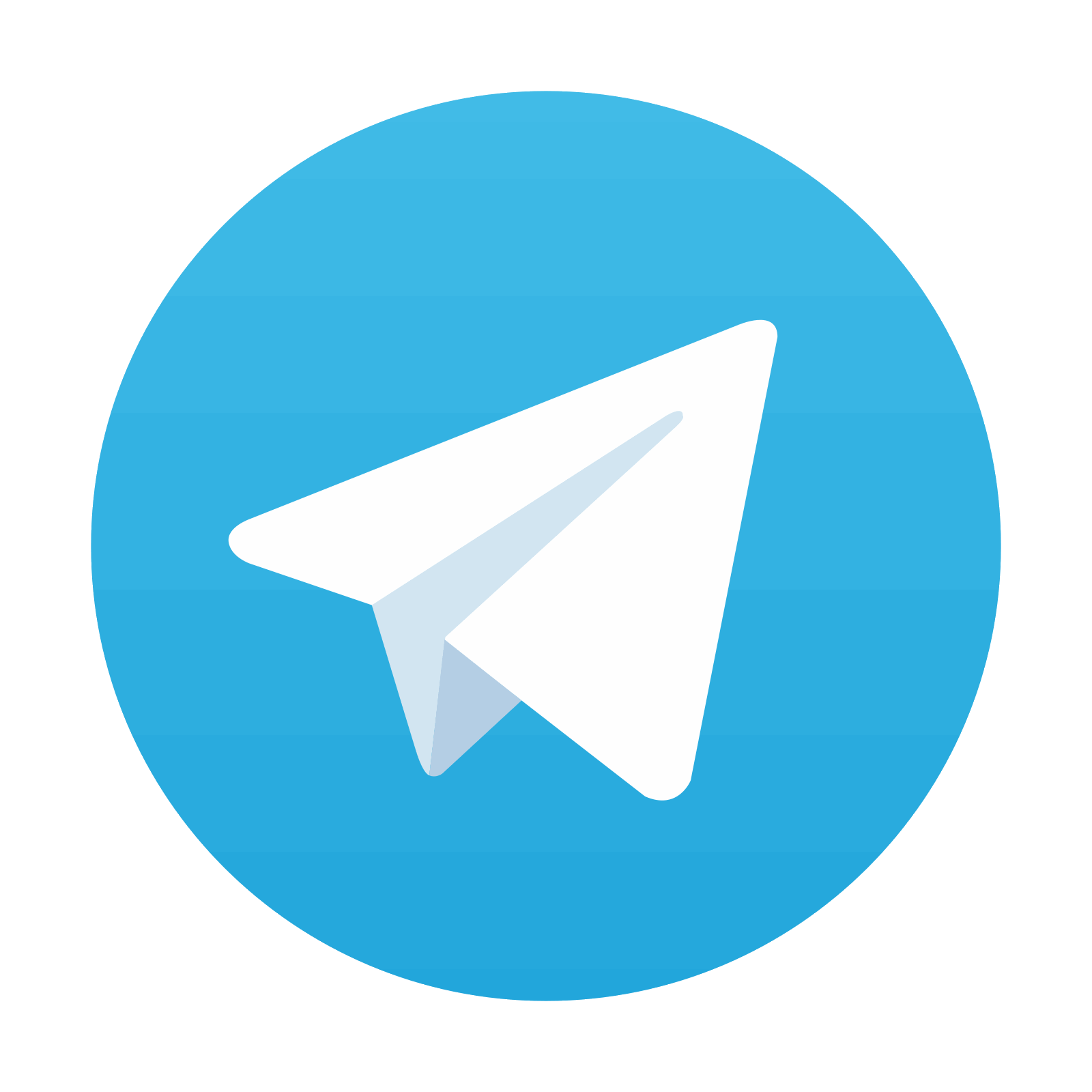
Stay updated, free articles. Join our Telegram channel

Full access? Get Clinical Tree
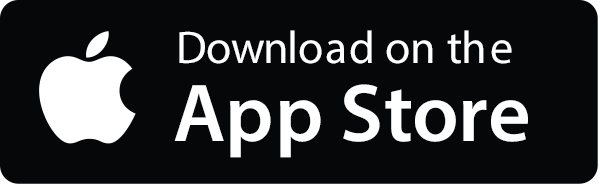
