Figure 17.2.1 Pockets on the surface of a protein. For the color version of this figure, go to http://www.currentprotocols.com.
Because many of these sites will be superfluous, it is important to prioritize the pocketome of a species by using various criteria including volume, area, and sphericity. Afterwards, the top rank-ordered pockets should be analyzed to determine whether they exist on important bacterial proteins. With the advent of increased computational ability, one can now scan all the protein structures in an entire genome in a systematic manner (An et al., 2005). Doing so allows for the rapid determination of those proteins that have binding sites most amenable to drug targeting. In one example, a druggability formula has been developed for the scoring of active-site pockets on a genome-wide scale for Plasmodium falciparum, the causative pathogen of malaria (Nicola et al., 2008). The formula used the following six terms: (1) absolute residue sequence identity, (2) sequence identity relative to the protein surface, (3) absolute sequence identity with the closest human homolog, (4) pocket volume, (5) pocket surface area, and (6) crystallographic resolution of the protein. By searching for closest human homolog, the goal was to avoid binding to proteins that are similar in humans, and may cause cross-reactivity. The novel protocol incorporated these variables in a unique formula for identifying drug-like pockets. In addition, pockets were visualized on the surface of the protein to determine whether the sites have meaningful biological relevance, such as affecting a catalytic region, or blocking a dimerization interface. Based on the results of this analysis, a druggable pocket was discovered on enoyl-acyl carrier protein reductase (ENR), an enzyme involved in the critical process of fatty acid biosynthesis in the pathogen. Due to its important role in the life cycle of malaria, and a lack of suitable therapeutics targeting it, ENR was chosen as the best suited for virtual-screening-based drug discovery. Once a good binding pocket is discovered on a critical protein, the next step is to search for small molecules that can bind to this pocket.
Library Generation
The easiest method to begin screening for potential small molecule binders of a new protein target is to contact one of the companies providing these commercially available compounds. There are at least 40 commercial vendors, providing >8.2 million compounds available for purchase (http://www.molsoft.com). Some of the larger databases include Ambinter (http://www.ambinter.com), Interchim (http://www.interchim.com), and Ryan Scientific Inc. (http://www.ryansci.com). Some, like the National Cancer Institute (NCI; http://cactus.nci.nih.gov/), are provided freely by the government. By using an electronic version of these libraries of compounds, it is possible to generate customized versions of the databases. For instance, in the cases when more than one vendor offers the same compounds, it is a good idea to compile a new database of nonredundant compounds before proceeding. Additionally, it is prudent to select only those compounds that have drug-like properties. This method, termed “enrichment,” enhances the probability that a compound arising from a screen will be a good hit (Sun et al., 1998; Koehler et al., 1999).
Finally, a database of virtual compounds may also be designed for screening. This offers the advantage of a wider variety of compounds, and the inclusion of compounds enriched with specific properties. However, because these virtual compounds are not available from a commercial source, it is necessary to synthesize a desired compound if it is selected as a candidate drug.
Drug Repurposing
One approach to drug discovery that has gained considerable momentum recently is the notion of repurposing. This technique essentially refers to taking an existing drug and using it for another target (Carley, 2005). The approach has a notable advantage; if the drug has already gone through clinical trials, then it is already known that the drug is nontoxic, thus eliminating the large hurdle of safety testing. Exploiting the “off-target” secondary activity of marketed drugs may help in generating drug leads to new bacterial proteins. It is feasible that new antibiotics may be discovered that already have indications for some unrelated disease. In one example of the computational repurposing procedure, antipsychotic drugs were found to have the properties needed to be optimized into prostate cancer therapeutics (Bisson et al., 2007).
High-Throughput Screening
High-Throughput Screening (HTS) is an approach used to screen a vast number of compounds against a single target. Traditionally, this type of drug discovery has been the pursuit of large pharmaceutical companies, and a few specialized academic laboratories. The technique uses a brute-force approach to collect a large amount of information about how a protein target reacts to exposure to hundreds of thousands of chemical compounds. The number of hits that can be tested with biological in vitro techniques limits this method. As expected, such an approach is very costly, due primarily to the large library of compounds, which require purchasing. In addition, the process is time-consuming, as specialized protocols need to be devised for each type of HTS experiment.
Virtual Ligand Screening
Instead of purchasing a collection of compounds and performing bench experiments to discover promising new hits, the emerging technology of computational, or “in silico,” biology is enabling the initial phases of drug discovery to be performed at the bench. The concept of applying computational methods to resolve important biological problems has advanced tremendously in the past few years; computers are getting faster and software programs are now becoming more robust.
The best approach to discovering a molecule is by designing one that can fit into a region on the protein receptor where it normally binds other proteins. If the receptor cannot bind other proteins, it will no longer be active. Whereas the laboratory approach tests a few potential drugs by trial-and-error, the computational approach screens millions of compounds within a few days. Thus, the approach called Virtual Ligand Screening (VLS) offers many advantages over traditional HTS methods (Table 17.2.2). Primarily, VLS is much cheaper and less time consuming than high throughput screening (HTS).
Table 17.2.2 VLS Procedure
Procedure | Goal |
1. Receptor Modeling | Predict the correct receptor pocket model. |
2. Library Generation | Create a sufficiently large and diverse set of relevant compounds. |
3. Flexible Docking | Correct prediction of binding geometry between protein and ligand. |
4. Ligand Scoring | Filter top-ranked compounds to those most drug-like, avoiding toxicity, and eliminating false positives. |
5. Hit list post-processing | Improve compounds by similarity searching and chemical derivatization. |
VLS employs a flexible docking protocol similar to the one described above; except instead of docking a single ligand to a protein-binding site, multiple ligands are docked. Because this method of small molecule docking is done on a much larger scale, a supercomputing Beowulf cluster is typically used for the analysis. This type of computing cluster combines hundreds or thousands of central processing units (CPUs) together and allows the docking simulations to be run in parallel, so as to increase the throughput of data.
The VLS method is important in discovering small molecules for a better understanding of the comprehensive aspect of proteins. This method also allows researchers to narrow the search to a relatively small set of compounds. When a few molecules are determined to be potential hits, these molecules are purchased in order to be experimentally validated in enzymatic and cell-based assays. This approach has been used in the past to successfully identify small molecule ligands/inhibitors of HIV-1 TAR (Filikov et al., 2000), the human retinoic acid receptor-α (Schapira et al., 2001), anthrax lethal factor (Forino et al., 2005), and P. falciparum ENR (Nicola et al., 2007).
Scoring
Once a list of hits is returned from the VLS run, the next step is to remove false positives from this list. In order to do this effectively, a proper scoring function should be employed. Scoring functions are used to assign an affinity to each possible geometric arrangement of the receptor-ligand complex. This affinity typically takes the form of free-binding energy between a ligand and receptor (Bock and Gough, 2002; Roche et al., 2002). Other approaches include theoretical assessment of solubility, lipophilicity, and other pharmacological properties.
As part of the filtering process, it is important to ensure the compounds tested will be bioavailable and nontoxic to cells. This can be performed using an in silico ADMET (absorption, distribution, metabolism, excretion, toxicity) filter. This type of analysis analyzes all the structural groups of the compounds and compares them to a database of known problematic substituents. Compounds containing these so-called chemical “structure alerts” (Ashby, 1985) can then be eliminated from the list of top-scoring hits.
Optimization
Once a few promising antibacterial compounds are validated in a laboratory, they are examined for their potential to be fine-tuned in order to enhance their ideal properties. Traditionally, this step involves a medicinal chemist who understands the reactivity of the compound of interest. More recently, however, computational tools are being used for this analysis as well. Two general approaches are employed for this procedure: similarity searching and chemical derivatization.
Similarity search
A similarity search is simply a screen of the newly discovered compound hits against the same, or a different, database of compounds. The reasoning behind this step is to increase the likelihood of identifying other compounds with similar structures to the originals, but perhaps containing substituents leading to improved binding. As with the initial compounds, it is important to validate these additional compounds with enzymatic and cellular assays to verify their improved potency.
Chemical derivatization
As an alternative, or in parallel to similarity searching, the compounds may be improved through chemical “derivatization.” This technique seeks to improve the binding of the compounds within the active site by “growing” them to achieve a better lock-and-key fit. Visually observing the docked conformation of each compound, and then rationally manipulating certain chemical moieties to design better derivatives, accomplish this better lock-and-key fit. Aside from manual improvement, this type of derivatization can also be done systematically and rapidly using a computational approach. The goal with this approach is to ensure the new compounds have an increased chemical and steric compatibility with the binding pocket. This, in turn, may help improve their efficacy and specificity to develop the compounds into more potent inhibitors.
Validation
Experimental assays
As with all computational analyses, it is important that the predicted antibiotic hits are validated with experimental tests. The narrowed list of hits arising after the scoring and filtering procedures can be purchased from their respective commercial vendor. Once obtained, the compounds should be tested in known experimental assays. They can be validated for activity using in vitro assays and selecting for molecules with good binding affinities. Typically, these experiments are conducted by a research laboratory that has experience with the studied bacterial target.
If the compounds are not available commercially, as may be the case when the compounds are virtual or derivatized, it may be necessary to synthesize the new molecules.
X-ray structure determination
To ensure binding is specific to the binding site of interest, it is ideal to solve the crystal structures of the compounds in complex with the target bacterial protein. A three-dimensional view of the complex has a two-fold purpose; it will (1) validate the predicted docking poses of the compounds to the binding pocket, and (2) allow the further engineering of an inhibitor with greater complementarity within the binding cavity.
Visualizing the crystal structure of the compound in the binding site of the target allows for a detailed analysis of the binding geometry. This information is very valuable when suggesting any necessary modifications, as when conducting chemical derivatization. Clearly, many of these steps may be performed in a different order, or in parallel when seeking to arrive at the best compound rapidly. Moreover, the series of steps lends itself to multiple iterations, whereby validated compounds can undergo a new round of optimization and validation, etc. (Fig. 17.2.2).
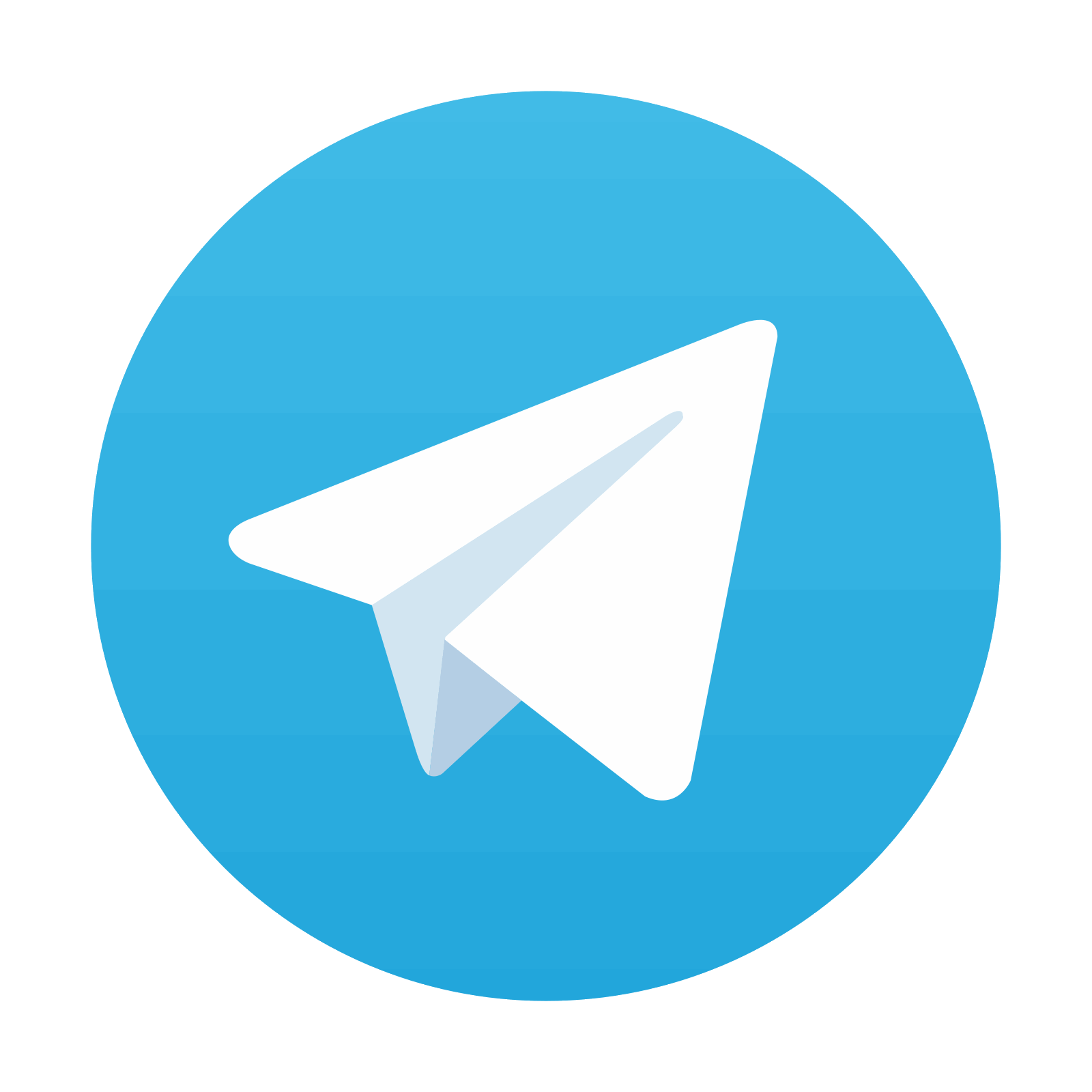
Stay updated, free articles. Join our Telegram channel

Full access? Get Clinical Tree
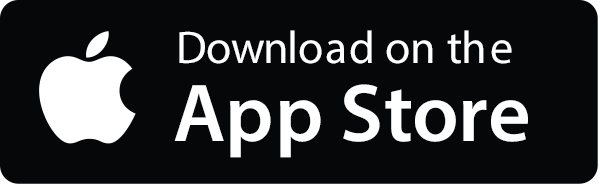
