Figure 1 Mechanisms of antibiotic resistance. Panel a, for inhibiting bacterial growth, an antibiotic (1), requires traverse the cellular envelopes either by specific transporters (2) or by diffusion through the membrane (3). In few occasions, the pre-antibiotic requires its activation by an intracellular enzyme (4). Finally, the antibiotic reaches its target at concentrations high enough to allow its inhibition (5). The presence of constitutively expressed efflux pumps (6) can decrease the intracellular concentration of the antibiotic and contributes to intrinsic resistance. Panel b, resistance can be achieved by changes that impede de entrance of the antibiotic in the cell (a, b), by enzymes that inactivate the antibiotic (c), by changes in the enzyme that activates the pre-antibiotic (d), by target modifications that impede the antibiotic interactions (e) or by overexpression of chromosomally encoded efflux pumps (f) or acquisition of these determinants by HGT, which reduces the intracellular concentration of the antibiotic.
Mutation-driven antibiotic resistance
Although commensal bacteria can serve as donors of resistance and in vivo transfer of antibiotic resistance elements has been described, the most usual mechanism of in-host adaptation to the presence of antibiotics during treatment is mutation. One important feature to understand the bacterial capability to evolve under the selection imposed by antibiotics is to know whether bacterial mutation rates can change (Martinez and Baquero, 2000). Some works have shown that this is in turn the case. Bacterial populations display different mutation frequencies and chronic infections (and likely long antibiotic treatments) select for bacterial populations with hypermutator phenotypes (Oliver et al., 2000; Martinez-Solano et al., 2008). Stable hypermutation is thus a fruitful mechanism for increasing adaptability (and antibiotic resistance) during chronic infections. Transient increases in mutation rates as the consequence of stress, including antibiotic stress and starvation, have been described (Foster, 2005) and might also be relevant for in vivo increased mutation rates leading to antibiotic resistance (Alonso et al., 1999b).
Acquisition of antibiotic resistance genes by HGT
Pathogenic bacteria were susceptible to antibiotics when these drugs were introduced for therapy and the main donors of emergent resistance genes have been environmental microorganisms (Davies, 1994). Although the number of potential resistance determinants present in environmental bacteria is huge (D’Acosta et al., 2006;; Martinez et al., 2007; Wright, 2007), just few of them are currently present in human pathogens. In some occasions, this is because the probability of contact (niche overlapping) between donor and recipient bacteria is scarce. For instance, it is unlikely that resistance genes found in the organisms from the deep terrestrial subsurface might contribute to resistance of microbial pathogens. The incorporation of the resistance gene in a gene transfer unit capable to replicate in microbial pathogens is also needed for its dissemination, which is favoured if the associated fitness costs are low. Finally, it is expected that once a resistance determinant appears for the first time, it will rapidly spread among bacterial populations under strong antibiotic selective pressure. The success of resistant bacteria in clinical environments will maintain the population containing this determinant, thus avoiding the entrance of novel resistance genes, unless the selective pressure changes (Martinez et al., 2009).
Human-driven evolution of antibiotic resistance determinants
Once resistance determinants are located in gene-transfer units, they can suffer further diversification as the consequence of strong selective pressures in clinical settings. For instance, the existence of identical genetic surroundings of the beta-lactamase genes blaCTX−M−32 and blaCTX−M−1 in the same IncN plasmids indicates evolution of this type of beta-lactamases after their integration in those plasmids (Novais et al., 2007). In another example, the beta-lactamase TEM-1, has been prevalent for several years, without any change, in resistant enteric Gram-negative bacilli (Roy et al., 1983). However, a strong adaptive radiation of TEM-1-derived enzymes have occurred (Paterson and Bonomo, 2005) after beta-lactamase inhibitors and new generations of beta-lactams were introduced for therapy. The apparition of these new TEM variants is solely due to the evolutionary process triggered by human utilization of antibiotics.
Combinatorial Evolution and Modularization in Antibiotic Resistance
Modularity is an attribute of a system that can be decomposed into a set of repeated, conserved cohesive entities that are loosely coupled (Wolf and Arkin, 2003; Pereira-Leal et al., 2006). As shown in Figure 2, the spread of a given resistance gene requires its successful integration in different hierarchical entities (from gene arrangements to bacterial clonal complexes) that display a modular structure. Each of these elements is not evenly distributed in all environments: not every phylogenetic group within a given species is equally represented in different environments; neither every plasmid is present at equal frequency among different bacterial species or subspecific groups. A given transposon is not inserted with similar frequency in each type of plasmid, nor any kind of integron in any transposon, or any antibiotic resistance gene in any integron (Souza and Eguiarte, 1997; Sherley et al., 2003; Wisplinghoff et al., 2003; Escobar-Paramo et al., 2006). Although several combinations are possible for these hierarchical entities and modules, only a number of them are found in nature. Understanding if there are some specific affinities between these modules could reveal a basic grammar of these Russian doll-within-doll modular interactions (Baquero, 2004; Gimona, 2006), thus providing the bases to predict the possibility of establishment of particular interactive functional networks, including those involved in antibiotic resistance.
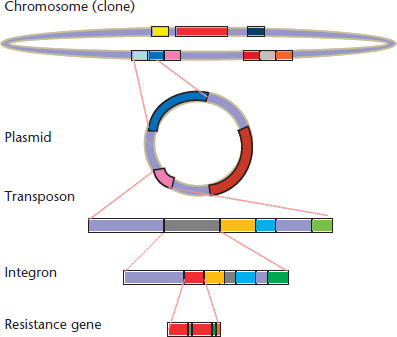
Figure 2 The modular and hierarchical structure of the genetic elements involved in antibiotic resistance. The resistance gene contains domains that are critical for its function; at its turn, the resistance gene might be captured by an integron, and the integron can be inserted in a transposon, which is part of a plasmid, and the plasmid can be transferred to a particular bacterial lineage (clone), where genetic modular interactions between the chromosome and the plasmid might occur. Note that the spread of the resistance gene depends on the evolutionary success of each one of the genetic elements up in the hierarchy.
The addition of new ‘resistance’ modules to a particular region is an important issue to understand multiresistance. Incremental modularization might occur because there is a ‘module-recruiting’ module (e.g. a recombinase in an integron; Mazel, 2006). As the incoming modules frequently provide new interactive sequences, module accretion increases the local possibilities of recruitment of new modules (Figure 3). The cumulative collection of antibiotic resistance traits within particular multimodular structures (integrons, transposons and plasmids), which act as single transmissible modules, results from this type of nested evolution.
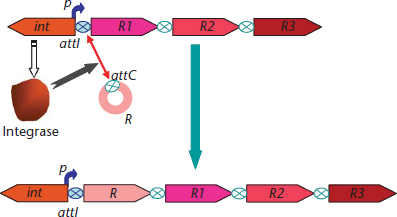
Figure 3 Integrons as gene-capture units. The integrons are composed by an integrase gene (int) and an arrangement of gene cassettes, which expression is driven by a strong promoter (p). Upstream the first gene cassettes the integrons contain a recombination site (attI) that mediates the integrase-driven recombination with the attC sites present in the gene cassettes.
The basic modularity of the genome also provides the bases for low-cost intragenomic recombination and the spread of homologous repeated genetic sequences that might contribute to antibiotic resistance. Gene conversion assures nonreciprocal transfer of information between homologous sequences inside the same genome. This might lead to minimizing costs associated with the acquisition of mutations (functionally replacing the mutated sequence) or by contrary maximizing the benefits of mutations that confer a weak advantage when present in a single copy, by spreading copies of the mutated sequence (Santoyo and Romero, 2005).
Spread of Resistance: Bacterial Clones and Horizontally Transferred Elements
Bacterial species are usually subdivided in subspecific lineages displaying different evolutionary histories. Most isolates in a clonal population belong to one of a limited number of genotypic clusters (clonal complexes) that emerge from the rise in frequency and subsequent radial diversification of clonal founders. Rise in frequency is in most cases the consequence of selective events favouring the outburst and spread of particular clonal complexes in specific environmental circumstances, each clone corresponding to a fitness peak (an ecotype). This means that the clonal structure of a bacterial population might reflect the changing variety of environments colonized as well as the challenges suffered by this bacterial species, including the exposure to antibiotics. In this sense, clones might behave as adaptive modules of a hierarchical superior entity, a ‘regional community structure’, integrating ‘optimal clonal compositions’ able to provide alternative stable states if the habitat changes. Hosts of the same class (e.g. different human hosts) tend to harbour similar regional community structures of bacterial clones. Clones not belonging to such macrostructures are not readily accepted (Figure 4), and might have severe constraints to circulate among related hosts, except in the case of immature (newborns, pathological status) or damaged (antibiotics, altered nutrition) microbial ecosystems. This point is critical to understand the risks of dissemination of antibiotic resistance genes between animals, humans and the environment. Indeed, the success of a given resistance gene depends on most cases of the ability of spreading of the clone, or the mobile element, hosting such resistance. Mobile elements containing resistance genes, as plasmids, might circulate more effectively in such a genetically highly homogeneous multiclonal structure, leading to typical complex endemic antibiotic resistance situations also termed resistance ‘allodemics’ (Baquero et al., 2002).
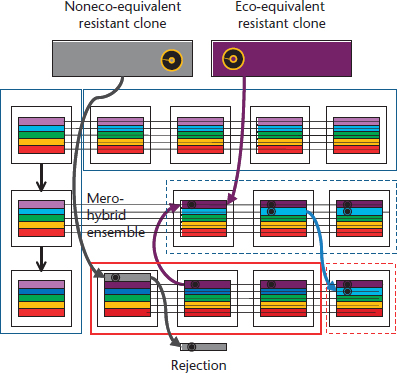
Figure 4 Optimal genetic compositions of bacterial communities inside the hosts. Each white square represents an individual that is hosting an equilibrated array of different bacterial organisms (rainbow horizontal bar pattern, in the figure). This array can be perpetuated in the host by vertical transmission (mother–child, vertical column at the left) or by horizontal transmission to hosts sharing the same environment (upper section in the figure). Small variations in the bacterial organisms are permitted (eco-equivalent bacterial types still maintaining the rainbow pattern), as it is shown by different tones of green or violet, which might freely circulate among all ‘rainbow’ hosts. Eco-equivalent types are those that have the same or very similar function in the community of types. The introduction of these types originated from a similar community leads to the formation of near-to-the optimal mero-hybrid groups, that is, ensembles that have a part from a related group. If the genetic element containing the resistance gene (black circle) is located in a bacterium (or clone) that does not belong to the optimal array for this type of hosts (grey), it can be acquired by the host, but the probabilities of rejection are high (lower section of the figure). On the contrary, if the resistance gene is acquired by any of the members of the rainbow consortium, its possibilities of spreading and becoming endemic in these hosts are high. Large boxes represent the different ecosystems.
The study of pre-antibiotic collections of plasmids shows that the appearance of resistance genes in plasmids has only occurred during the last decades (Datta and Hughes, 1983). The diversity of the main plasmid families remains relatively limited, illustrating the success in continuous adaptation of old plasmids to antibiotic challenge. An example is the recent dissemination of old plasmids due to the incorporation to their genetic sequence of genes encoding for extended-spectrum beta-lactamases (Novais et al., 2007).
Antibiotics and Antibiotic Resistance Genes in Nonclinical Environments
Several antibiotics have been isolated from soil microorganisms. Because of this, it was widely assumed that the function of antibiotics in nature should be to inhibit the growth of competitors (Waksman and Woodruff, 1940). Following the reasoning, it has been thought that antibiotic resistance determinants should have specifically evolved in environmental microbial populations to avoid the activity of antimicrobials. In some occasions, this fight-for-life view is a reasonable explanation for the function in nature of antibiotics and their resistance determinants. However, recent works indicate that antibiotics (at least some) might be involved in intercellular signalling at the low concentrations at which they are likely encountered in most natural environments (Yim et al., 2007; Fajardo and Martinez, 2008). Under this view, it could be possible that resistance genes might serve for other purposes besides avoiding antibiotic activities in the natural ecosystems where they evolved before recent massive utilization of antimicrobials. Metabolic enzymes, with substrates structurally similar to a given antibiotic, might modify this drug and serve as antibiotic resistance determinants in clinical settings with high antibiotic loads. For instance, it has been suggested that the primary function of beta-lactamases might be the synthesis of the cell wall more than resistance to beta-lactam antibiotics (Meroueh et al., 2003). The same happens with other mechanisms of resistance that do not involve antibiotic modification. Quinolones, a synthetic family of antibiotics introduced for therapy in the 1960s, are a favourite substrate of multidrug efflux pumps. Furthermore, environmental bacteria isolated before the invention of quinolones can extrude them (Alonso et al., 1999a), indicating that resistance is not the primary function of those determinants. Similarly, the plasmid-encoded quinolone resistance determinant Qnr (Martinez-Martinez et al., 1998) is chromosomally encoded in waterborne bacteria (Sanchez et al., 2008) in habitats where the presence of quinolones is not expected, further indicating that in several occasions avoiding antibiotic activity is not the primary function of antibiotic resistance determinants. The acquisition of those determinants by pathogenic bacteria, where their main role is antibiotic resistance (Martinez, 2008) is a good example of the evolutionary process named exaptation or co-optation (Gould and Lloyd, 1999), by which one element that served for a given function in its original environment plays a different functional role if the environment changes.
This situation in which antibiotics and their resistance genes are present in nature, at the concentrations and in the host selected by millions of years of evolution, has changed in the past decades. The release of high amounts of antibiotics and their resistance genes, as the consequence of human activities, in natural, nonclinical environments might disturb microbial populations. At these high nonphysiological concentrations, antibiotics are chaos-promoting factors for microbial ecosystems because they provoke functional disorders and death of several bacteria. At the supracellular level (e.g. intestinal bacterial communities or soil microbiota) the functional loss of bacteria within a particular system can be repaired by residual populations that survive antibiotics challenge, by populations of other bacteria fulfilling a similar function, by imported populations migrating from a connected system or by the emergence of novel variant (resistant) organisms. At the level of the individual organism (e.g. bacterial cell) survival will depend on replacing those functions that the antibiotics inhibit, by importing foreign genes that can deactivate the antimicrobial or by mutation-dependent innovation that leads to antibiotic resistance. Antibiotics have a second-order evolutionary impact on suborganismal elements (e.g. plasmids, integrons and genes). Critically, antibiotics or any other agent promoting disorder may expand its impact across the whole hierarchy of evolutionary units of selection (from genes to microbial communities) (Baquero et al., 2003). For instance, local disordering events may select different types of bacterial clones in a particular environment (e.g. a hospital). Genes carried by these clones may be enriched and this amplifying selective process increases the possibilities of interaction among certain clones and genetic elements in such a way that the best combinations allowing local survival increase in number, which facilitates further adaptive possibilities (Baquero, 2004). From this perspective, antibiotic resistance might constitute an ecological risk and at the same time (deactivating the effect of antimicrobial drugs) a factor of ecological protection.
The Fate of Antibiotic Resistant Bacteria. Should Antibiotic Resistance Determinants Remain in the Absence of Selection?
Recent works have shown that the same resistance genes and the genetic platforms containing them currently present in human pathogens are encountered as well in pristine environments faraway of human contact. This suggests that, once an antibiotic resistance determinant is selected, the probabilities for its maintenance even in the absence of antibiotics might be high. For instance, resistance to sulphonamides is still widespread in the UK, although these antibiotics are not currently prescribed. This is in contradiction with the idea that resistance is associated to fitness costs. To explain the reasons for the stability of some resistance mechanisms and not others, we have to remember, that in some occasions, acquisition of resistance has no-cost for bacteria, or even render a higher fitness in specific environments (Alonso et al., 2004; Luo et al., 2005; Balsalobre and de la Campa, 2008). Furthermore, even when fitness costs are high, compensatory mutations reducing bacterial burden are selected (Bjorkman et al., 2000; Paulander et al., 2007; Lofmark et al., 2008). It is also worth to remark that several antibiotic resistance plasmids encode toxin–antitoxin systems (Hayes, 2003) that impede plasmid loss and allow maintenance of resistance. Finally, antibiotic resistance genes can be associated in the same unit (e.g. an integron) with other resistance elements or with determinants that confer an ecological advantage in a given environment (e.g. siderophores, toxins or resistance to biocides or to heavy metals). Given these genetic associations, resistance can be coselected by its associated trait in the absence antibiotics.
Predictions on Trajectories in Antibiotic Resistance: Evolutionary Functions
Prediction of evolutionary trajectories should constitute the ultimate way to demonstrate the truth of hypothesis in evolutionary sciences. In this last section we are intending to offer a comprehensive list of the main factors determining or influencing the evolutionary trajectories in antibiotic resistance, and therefore components in the predictive process (Baquero, 2009). This analysis does not intend to enter into the details of any of them, but rather to offer an integrated image of the complexity of the field. For such a purpose, we consider that evolutionary paths are the result of the composite effect of number of quantitative measurable variables, just as a mathematical function. An evolutionary function is considered here as a quantitative correspondence between the frequency of a measurable, independent biological entity (e.g. the frequency of an antibiotic resistance gene in a given environment) or an event (e.g. the intensity of antibiotic selective pressure), and the probability to occur in a quantitatively correlated dependent adaptive change (e.g. evolution towards resistance) in the informative (genetic) content of another (dependent) biological entity (e.g. integron, plasmid and bacterial cell). The resulting change can be comodulated by other independent variables, so that the final probability of an evolutionary significant event to occur is the result of a composite function. Note that the array of variables, the components of the prediction, might be variable in space and time, so that the final probability has a local optimum for each local set of conditions. Such approach for obtaining evolutionary predictions of antibiotic resistance is now just a theoretical exercise. Nevertheless, it should help in identifying the parameters, interactions and components needed to describe this complex system. The enumeration of eight major operative components that can shape the trajectories of antibiotic resistance might serve as a guideline to recapitulate the factors that influence such processes.
Probability of capture of an antibiotic resistant gene by human or animal bacteria
This probability depends on the following variables: (i) the frequency of antibiotic resistance genes in the environment; (ii) the frequency of gene capture units, able to pick up the resistance genes, in the environment; (iii) the frequency of human or animal bacterial clones in the environment with a significant resistance-gene pool; (iv) the genetic connectivity between environmental and human or animal bacterial clones, as to be clinically relevant, antibiotic resistance should enter into bacteria of the human or animal environment and (v) the genetic connectivity of the incoming gene within the new host; that is, its epigenetic acceptance in the human or animal bacterial host environment.
Probability of integration of the new resistant clones in particular human or animal microbiotic ensembles
A microbiotic ensemble is a set of microorganisms functionally linked by co-operating in the maintenance of a given community in a specific environment (as the gut). If an incoming bacterial clone belongs to this ensemble, or is functionally equivalent to one of its members, a successful colonization will happen; if not, the clone will be ecologically rejected. This rejection occurs because the functions potentially provided by this clone do not integrate into the network of functions exerted by the microbiotic community that assures an optimal integration with the local environment. The probability of fixing a resistance trait depends on the frequency of acquisition of incoming resistant clones by the local microbiota of the host. This frequency depends on: (i) the age of the host (in early stages of life, microbiota is more permissive for foreign bacteria); (ii) the environment–human/animal intensity of contacts (poor sanitation favours acquisition of foreign organisms); (iii) the between-human/animal intensity of contacts (e.g. crowding in hospitals or in farms) and (iv) the health status of the hosts.
Probability of antibiotic resistance gene capture by endemic, epidemic and pathogenic clones
If a given antibiotic resistance determinant is acquired by bacterial clones able to colonize many different individuals (endemic) or is successfully transmitted among many hosts (epidemic), or produces infections with increased exposure to antimicrobial agents, such resistance trait will be amplified. Such spread will depend on the: (i) frequency of endemic, epidemic and pathogenic clones at local and global levels and (ii) frequency of acquisition and maintenance of horizontally transferable genetic elements containing resistance genes in these particular clones.
Probability of refining the antibiotic resistance functions to fit with the selective landscape
Any resistance trait can evolve under antibiotic challenge (see earlier). Nevertheless, the functions of the proteins have structural constraints, and therefore only a number of evolutionary trajectories are possible (Figure 5). Evolutionary refinement might start with very small advantages. Antibiotic selective gradients can improve selection of specific mutations, with small changes in the susceptibility to antibiotics but are the hallmark for other mutations rendering high-level resistance (Negri et al., 2000). Therefore, the probability of improving the resistance gene depends on: (i) the frequency of mutation and recombination in hosting bacteria; (ii) the different possible evolutionary trajectories giving up the novel resistance phenotype; (iii) the frequency of challenging by antibiotic selective gradients able to select for variants with different levels of antibiotic resistance.
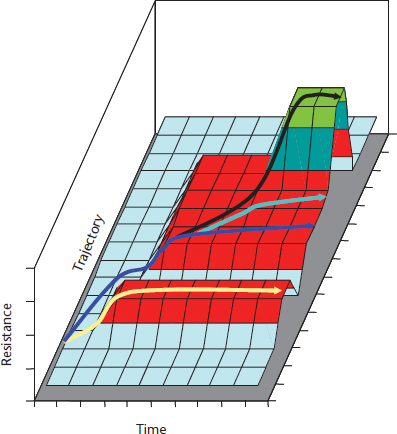
Figure 5 Evolutionary trajectories of antibiotic resistance as a hill-climbing process. Climbing gentle slopes might be required to have access to the high fitness (high levels of antibiotic resistance) peaks. In the yellow trajectory, such first step towards resistance might be limited for further developments, as it leads to a flat adaptive surface surrounded by lower fitness valleys (all possible mutations decrease resistance to the given antibiotic or to other antibiotic in the case of alternate selective challenges). Although the first event leading to resistance might occur later, in the blue trajectory, the flat adaptive surface reached after this first climbing event is larger, and resistance might spread and diversify without increasing the level of resistance (pale blue arrows). If second mutations are compatible with the first one, resistance can further increase (black arrow). In this black trajectory, the highest fitness peak is reached, but the surface on the top of the mountain is low and surrounded by steep cliffs, so that any genetic modification will reduce the fitness.
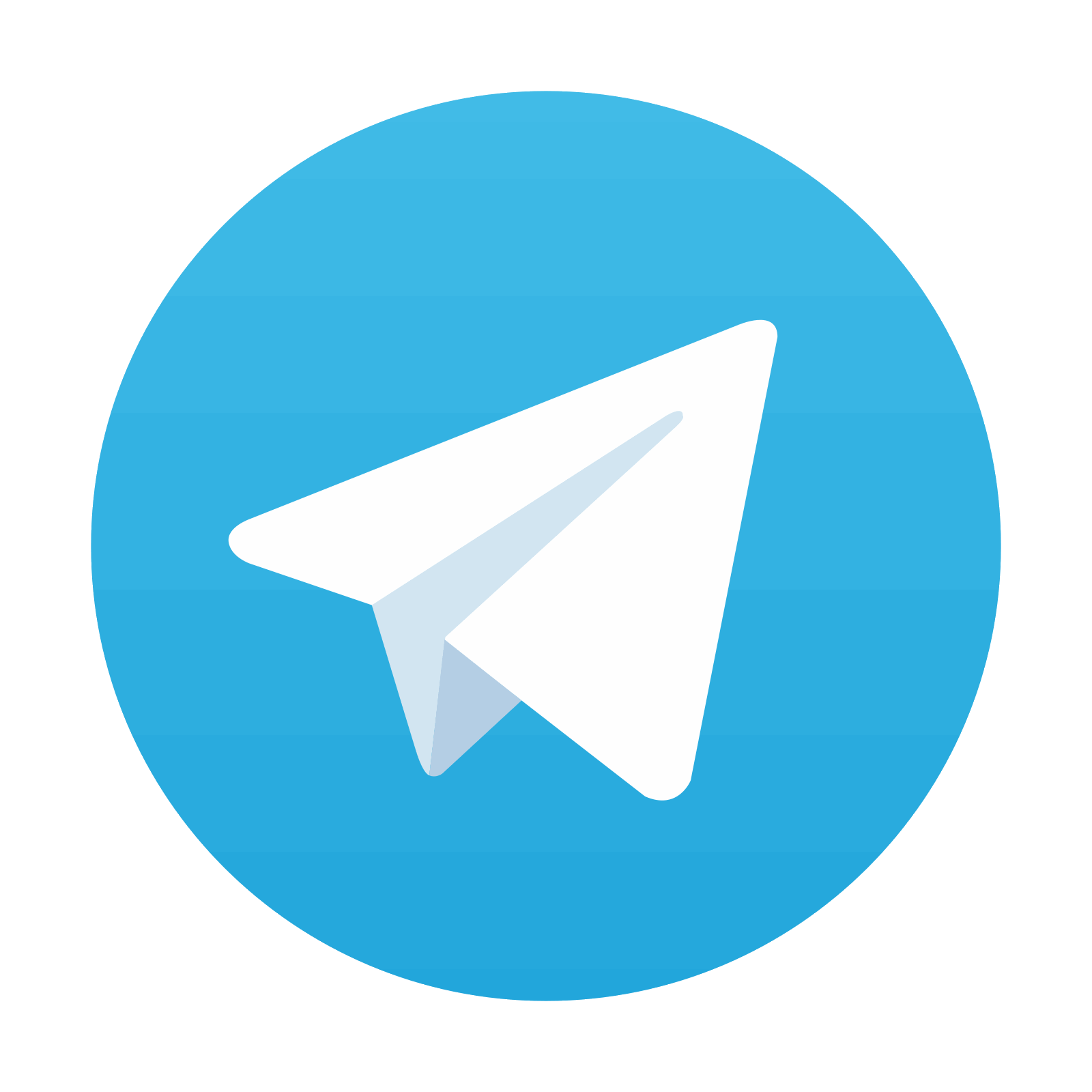
Stay updated, free articles. Join our Telegram channel

Full access? Get Clinical Tree
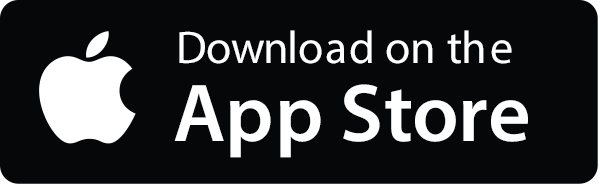
