Academic, Commercial, and Biodefense Case Studies for Collaborative Drug Discovery: Potential for Disrupting Drug Discovery
Collaborative Drug Discovery, Inc., Burlingame, CA, USA
Historically, drug discovery has evolved at fairly linear rates, with modest improvements as new technologies were developed; at worst, some have even correlated the additions of new technologies with the decrease in pharmaceutical productivity [1, 2]. This evolution at linear rates may be because (as many people believe) the easier problems were solved first and/or because of additional regulatory requirements that have been added as R&D has progressed. Even the 4.9% increase in scientific publications [3] seen each year, much like compounded interest, should be paying more handsome dividends than it does, at least for drug discovery. We know that other fields can improve dramatically when they evolve around a common standard. For example, in computer chip R&D, Moore’s law states, “The number of transistors that can be placed inexpensively on an integrated circuit doubles approximately every two years” [4]. In contrast, network-based phenomena evolve much faster per Metcalfe’s law, which states, “The value of a telecommunications network is proportional to the square of the number of connected users of the system (n2)” [5]; hence, we see astonishing exponential rates of evolution in adoption of Internet tools like Facebook [6].
We have previously described a brief history of Collaborative Drug Discovery, Inc. (CDD [7]) and our role as a provider of the first collaborative drug discovery data-hosting platform in the cloud [8]. Our years of experience developing software and efforts to promote collaboration to speed drug discovery provide a valuable perspective, which we will share through the course of this chapter. We will demonstrate how scientific teams and their projects mutually benefit from the secure exchanges of information. Both commercial and altruistic case studies clearly demonstrate how a collaborative software platform can accelerate the efficiency of drug discovery and reduce redundancy. The key question today is how these economic efficiencies can be scaled to the entire drug discovery ecosystem.
We have shown how a web-based database technology can allow anyone to collaboratively leverage others’ drug discovery results [9–13]. This enables the scientists within their natural workflow to effectively collaborate while maximizing the intellectual property rights required for commercialization. The first important prerequisite is having a scientist with a collaborative mind-set, who appreciates the value gained by collaborating with others. Efficiency is possible with the right attitude: a recognition and nuanced appreciation of the additional opportunity and challenges inherent in initiating and executing successful collaborations. A second prerequisite is the development and utilization of technologies that make collaborations work better [14]—of which there are many. Fortunately, there has been rapid progress in this field. With the enlightened use of collaborative technologies, more opportunities are realized because everyone with complementary capabilities is on the same page. As we will illustrate, complex collaborations can become manageable and scalable using technologies such as CDD and others.
Collaborative drug discovery platforms like CDD are broadly applicable because drug discovery projects have similar technical and commercialization hurdles. Apart from the perplexing, idiosyncratic features of individual molecules, targets, and assays, there are common, systematic technical challenges associated with lead identification via screening (whether high throughput, computational, or a combination of both) and lead optimization. Despite the great variation in scientific projects and assay methods at a data level for the vast majority of cases, the common standards have fortuitously become self-evident (sdf, csv, SMILES, xls, controls, IC50’s, hyperlinks, etc.), which has in turn led to the development of early bioassay ontologies [15]. These common standards developed over time, coupled with conserved drug optimization process workflows, suggest that a finite set of collaborative technologies can meet the majority of drug discovery informatics requirements. By being first movers, these collaborative tools set the standards for de facto collaboration and have formed a common foundation for collaborative science.
While in the past discovery of new drugs could be attributed to individual scientists [16], it is now incredibly rare for a scientist working in isolation to discover and develop a drug alone. Drug discovery work is collaborative, involving massive teams doing interdisciplinary preclinical and clinical research. We are also seeing not only companies outsourcing research that traditionally would have been performed in-house, but also companies collaborating with academics and other organizations, placing an extra emphasis on the need for collaborative technologies that effectively bring researchers together.
From our experiences with leading commercial, academic, and government laboratories [7], a recurrent theme has been the importance of giving each researcher control over whom they collaborate with (if indeed they need or want to). Providing facile control of each collaborator’s data access within natural workflows (with minimal activation barriers) has been a key for more effective collaborations and is therefore important for any technology in this collaborative domain. Providing fine-grained control in a secure environment for selective data sharing without multiple data uploads for prepatent and prepublication data (i.e., intellectual property and data-sharing capabilities as the data are being created) is a driver for effective collaborations. Researchers want the freedom to do what is necessary within their regular workflows or as demanded by their organizations. We should provide researchers with a convenient way to share data any time but always with the default being maximum privacy.
We believe there are at least two major “activation barriers” that need to be surmounted for facile collaboration around data. The first is reliably, accurately, and meaningfully capturing the data from the experiments. This is not as trivial as it sounds, and it is generally underappreciated. The second barrier is assuming the need to keep data private for commercialization reasons, which is generally overemphasized. In this context, it is worth remembering that, upon patenting, information is shared, albeit not directly in a reusable database format. By lowering barriers for both data archiving and selective sharing, we can begin to reap the benefits of Internet-catalyzed collaborations across the entire academic, industry, nonprofit, and government drug discovery ecosystem [17]. Technical innovation allows researchers to decide if, when, and with whom to share data. The realization of our vision to develop software for sharing data is seen in concrete examples like a leading academic high-throughput screening center simultaneously managing 26 separate, secure projects in a single collaborative “vault” (with appropriate access permissions as projects roll in and out of the screening queues).
We are clearly at the start of a new era for drug discovery, one of specialization, integration, and collaboration. It is an era driven by technologies that provide data availability, such as PubChem [18] and ChemSpider [19, 20] (for all open data), and better collaborative technologies like HEOS® (for all private data) [21, 22] and CDD (for private, collaborative, and open data) [9, 12, 13]. These technologies have become widely adopted, supporting thousands of researchers around the world who routinely trust these hosted systems, which in turn sets the foundation for a more effective paradigm. Drug discovery research can directly benefit from previous and complementary collaborative efforts, while collaborative informatics provides cohesiveness when scaling these efforts. Researchers can now potentially balance appropriate ownership of intellectual property (IP) with maximum collective learning. We should see new discoveries in turn occurring more rapidly as scientists collaborate, from which we can all benefit. The various case studies detailed later represent a wide array from the commercial, academic, government, and biodefense arenas to demonstrate how researchers across all types of organizations can form a collaborative team. We hope that the reader will join us in collaborative drug discovery soon and help us in our mission to positively disrupt drug discovery.
Academic Collaborative Drug Discovery Case Studies
Antimalarial Resistance Reversal
This was our first published case study [13] and involved a four-way collaboration. Academic and ex-industry scientists with complementary capabilities in the United States and Africa came together for the purpose of identifying antimalarial resistance reversal agents in combination with chloroquine [13]. Professor Kelly Chibale (University of Cape Town, South Africa) and his existing collaborators (including Dr. Peter Smith at University of Cape Town) had finite resources, budgets, and time. Dr. Chibale had done his postdoctoral work at the University of California, San Francisco (UCSF), and he knew other members of the CDD community at UCSF (e.g., Professors Phillip Rosenthal and James McKerrow) who also were working on small molecule drug discovery against malaria and other neglected infectious diseases like Chagas disease and African sleeping sickness. Given their personal and professional connections, they were willing to pool and share existing resources and compounds. Selected samples were shipped on dry ice from UCSF to Dr. Peter Smith’s laboratory at the University of Cape Town to screen for reversal of chloroquine resistance activity. New compounds were identified that almost completely reversed the chloroquine resistance. There was another discovery to come, however, using a set of 1720 known drugs and their therapeutic indications kindly provided by Dr. Christopher Lipinski (retired, Pfizer) and available in CDD Public for the use and benefit of researchers throughout the world. Within this data set, a further 18 matches were found with the same substructure that Prof. Chibale had identified as being responsible for reversal of chloroquine resistance activity. These known drugs were then purchased, shipped, and tested by Dr. Smith for antimalarial resistance reversal activity in combination with chloroquine. Several compounds almost completely reversed chloroquine resistance in vitro (sevenfold); these included the FDA-approved drugs pimozide, vinblastine, sertraline, and dihydroergotamine mesylate. The discovery of effective compounds that already have known human safety profiles and FDA approval can save years or even decades in the race to overcome malaria drug resistance, relative to starting a new project from scratch. This represents a potential in silico drug repurposing strategy, which we have discussed elsewhere as a way to accelerate drug discovery [23, 24]. It is worth highlighting that these public–private and private–private data sharing collaborations required multiple parties all providing complementary pieces, as well as the CDD platform that brought them together and facilitated it.
Tuberculosis Research
This second academic case study deals with another neglected disease and involved building a new community of Tuberculosis (TB) Drug Discovery Researchers funded by the Bill & Melinda Gates Foundation. With finite resources and software capabilities, CDD needed to balance the ability to support anyone working on TB drug discovery while accelerating the progress of the most promising hits and leads within a pipeline drawn from academic, nonprofit, government, and corporate laboratories distributed across the globe. The scaling challenges inherent in this project led to CDD’s current policy of freely hosting any data that researchers are willing to share publicly (via CDD Public) while charging for privacy, collaborative capabilities, import, and export. While several respected information technology leaders encouraged us to make CDD open source and offer services (following a Linux type model), a credible alternative business model has emerged that ensures sustainability of an increasingly valuable commercial software offering, balanced with an equally valuable free content offering for the general public.
Within the TB arena in the first 2 years of this project, 250 users from 58 labs used the CDD collaborative platform. Perhaps somewhat surprisingly, big pharmaceutical corporations have also been among the more forward-thinking participants in this project in terms of actively and publicly sharing private neglected disease structure–activity relationship (SAR) data. The project led to CDD publicly hosting GlaxoSmithKline malaria and Novartis TB SAR data—harbingers of the winds of change impacting our field.
The CDD TB database became an integral part of the workflow for most of these TB groups, enabling them to better understand their data and exploit their finite resources more effectively. The technologies and support enabled them to selectively compare their results, avoid duplicate work, and formulate better future research priorities. These collaborations allowed previously disjointed efforts to begin to coalesce into a “virtual pharmaceutical organization.” The participation of the largest global pharmaceutical companies and their willingness to facilitate the exchange of data between themselves and the academic and other nonprofit research groups through both open and secure private channels is an important, and historically irreversible, collaborative drug discovery development.
Some of CDD’s most advanced features have debuted within this TB community. For example, one laboratory used CDD’s software to manage a portfolio of distributed projects, much as a major pharmaceutical company manages internal or external projects. CDD provided this “Projects” functionality to all users. Projects allows for secure, selective data sharing or data partitioning with fine-grained controls of individual objects within secure vaults. Each vault is a separate database with customizable business rules for registration, field definitions, protocol definitions, assay readouts, assay-specific normalization options, and so on. Moreover, data within each secure vault are further partitioned into separate projects with carefully controlled personal access permissions for an extra level of data control.
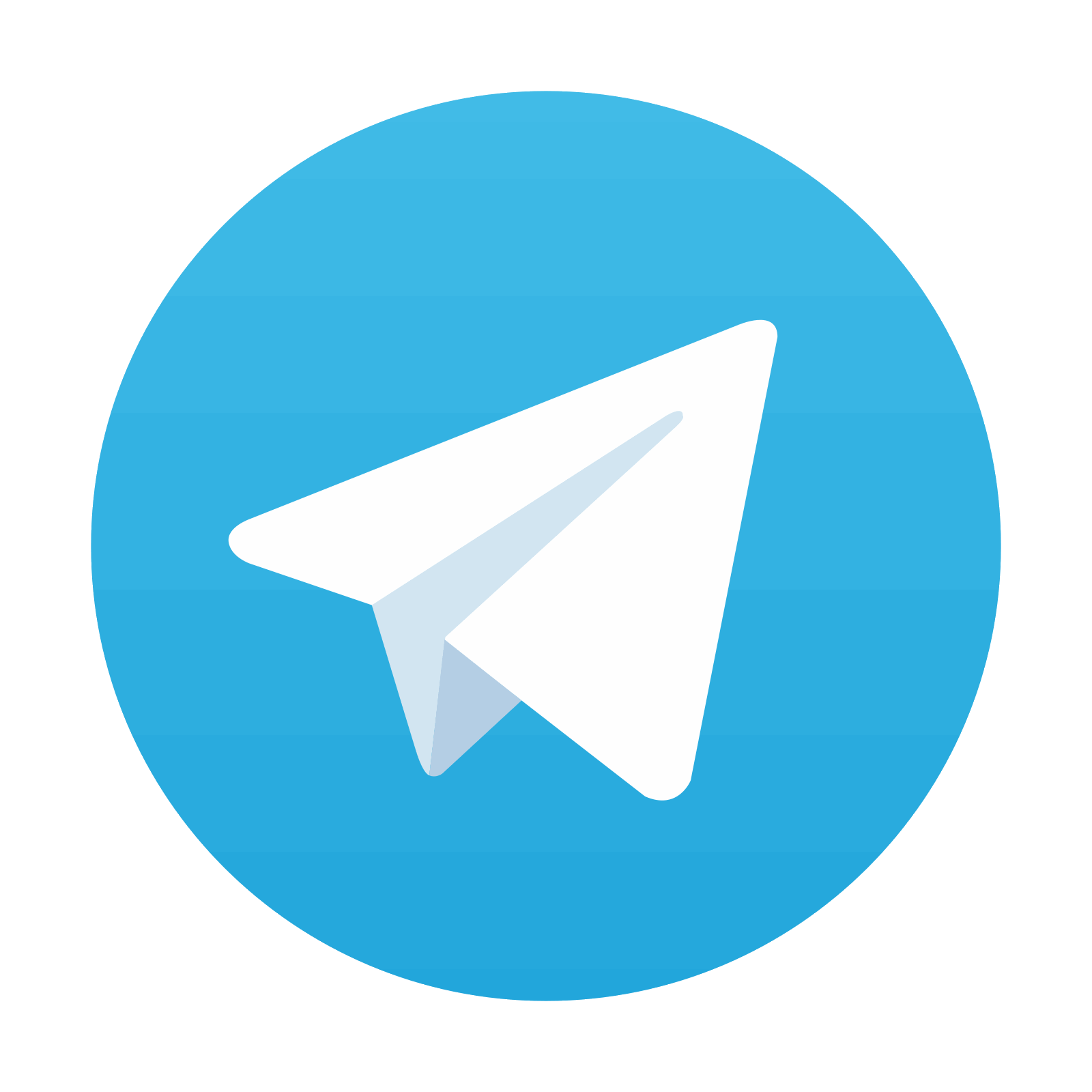
Stay updated, free articles. Join our Telegram channel

Full access? Get Clinical Tree
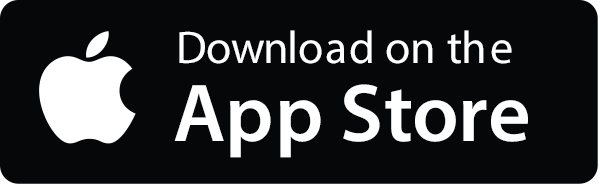
