Chapter Contents
False Claims 71
Amateurs at Work 72
Administrative Databases 74
Weak Associations: Size Matters 75
Porous Peer Review 76
Fraud 77
Conclusion 78
Observational research dominates the biomedical literature. However, few readers of that literature appreciate its tenuous scientific foundation. Most published findings of observational research are false. Of those findings that are true, most are exaggerated. Reporting is generally poor, and the inability to reproduce results is a generic problem in both the biomedical and behavioural sciences. Research with large administrative databases often produces precisely wrong results. Weak associations, below the discrimination limits of observational research, are routinely reported without caveats. Biomedical researchers are usually amateurs; most have no formal training in research methods, and the peer-review process at journals has little evidence of value. Retractions due to fraud are increasing dramatically. Observational studies have caused great harm and wasted precious resources. Indeed, an estimated 85% of the annual research investment is wasted. This chapter outlines some of the limitations of observational research and suggests some ways to address these problems.
False Claims
‘There is now enough evidence to say what many have long thought: that any claim coming from an observational study is most likely to be wrong – wrong in the sense that it will not replicate if tested rigorously’.
In 2005 Ioannidis shocked the medical world with his mathematical models showing that most reported research findings are wrong. He extended this observation by noting that of those associations that are true, most are exaggerated. The problem of false-positive claims is more acute with small studies, weak associations, more teams in pursuit of significant findings, and greater methodological bias or investigator prejudice. Indeed, large statistical associations may reflect large net bias, not any causality. At the other extreme, with massive study sizes, trivial effects (due to built-in bias) become statistically significant. Unsuspected or unmeasured residual confounding and confounding by indication handicap observational studies, and no easy remedies are available.
Others have confirmed that most observational study findings cannot be replicated. For example, 12 randomised trials tested claims from observational studies (including large ones) about diet, vitamins, and minerals. A total of 52 observational report claims were examined, and not one could be confirmed. Ironically, randomised trials found an effect in the opposite direction for 10% of the observational claims. Researchers and the lay public must grapple daily with an epidemic of false claims based on poor-quality research ( Fig. 7.1 ). Although observational studies and randomised trials in pulmonary and critical care sometimes concur, numerous interventions found beneficial in observational studies have been refuted by randomised controlled trials. Basing therapy on observational studies instead of randomised trials may be more costly in the long run and more dangerous to patients.

The medical literature is replete with bogus findings ( Panel 7.1 ). Cigarette smoking was falsely linked with suicide due to inadequate control of confounding. Betacarotene was shown to have no benefit in reducing lung-cancer risk. Selection bias led to a consistent, but incorrect, conclusion that oestrogen in menopause was associated with a reduced risk of heart disease. Poor-quality case-control studies falsely linked reserpine (an antihypertensive drug) with breast cancer and coffee drinking with pancreatic cancer. Social desirability bias (healthy controls not reporting sensitive information) linked abortion with breast cancer. Junk science led to the disappearance of a safe and effective antiemetic widely used in pregnancy. Inappropriate control groups, information bias, and failure to control for the confounding effect of sexually transmitted diseases led to the near disappearance of the intrauterine device (IUD) in the United States in the 1980s. Lack of control of confounding led researchers to believe that oral contraceptives were linked with pituitary tumours. A recent news report linking wearing high heel shoes and cancer has led both authors to abandon our stilettos for good. Better safe than sorry!
Exposure | Outcome | Explanation |
---|---|---|
Cigarette smoking | Increased risk of suicide | Smoking associated with factors predisposing to mental state that increases suicide risk |
Βeta-carotene | Reduced risk of lung cancer | Information bias and residual confounding |
Menopausal oestrogen therapy | Reduced risk of coronary artery disease | Selection bias: women who chose to use oestrogen were at lower risk of coronary artery disease |
Reserpine therapy | Increased risk of breast cancer | Flawed case-control studies; findings not replicated by later, larger studies |
Coffee drinking | Increased risk of pancreatic cancer | Gravely flawed case-control study; finding refuted by later studies |
Induced abortion | Increased risk of breast cancer | Information bias; underreporting of abortion among healthy controls |
Bendectin (pyridoxine/doxylamine) exposure | Increased risk of birth defects | Junk science |
IUD use | Increased risk of salpingitis and infertility | Wrong comparison groups, information bias (systematic overdiagnosis in IUD users), failure to control for confounding by sexually transmitted diseases |
Oral contraceptive use | Increased risk of pituitary adenoma | Confounding by indication |
Amateurs at Work
For millennia, medicine was learned through apprenticeship. By the early 1900s the deficiencies in this approach were evident. Abraham Flexner’s review of American medical schools led to the closure of many for-profit schools. Thereafter, medical schools joined forces with teaching hospitals. For the past century, medical education has featured clear objectives, formal curricula, postgraduate training, and national testing for competence.
In contrast, biomedical research continues to be learned through apprenticeship. As a result, few researchers today have any advanced training in research methods. Most young researchers learn on the job under the guidance of an older colleague, who generally has no formal research training either. As a result of the lower standards for medical research than for medical practice, most research today is suboptimal, as is its reporting. The desire to be a surgeon is insufficient to gain operating-room privileges. Not so in research. No formal training or certification is required before doing research and submitting a manuscript for publication.
The poor quality of biomedical research and its reporting is well documented. Many reports make no mention of limitations of the study. Even in high-profile general medical journals, description of control for confounding bias remains poor. Statistical errors include multiple unplanned comparisons to find something statistically significant (‘ p -value hacking’), single imputation of missing data, ignoring regression to the mean, and inferring causation from statistical associations in observational studies. Discussion sections of manuscripts, where limitations and caveats should be discussed, are often chaotic and loosely tethered to the results. Structured discussion sections, akin to structured abstracts, might promote transparency regarding the limitations of observational reports.
Many clinical practice recommendations cannot be supported by the published literature. Because of these deficiencies, international guidelines now exist for reporting observational studies. The Strengthening the Reporting of Observational Studies in Epidemiology (STROBE) guidelines are available on the Internet ( http://www.strobe-statement.org/index.php?id = strobe-home, accessed 9 March 2017) and on the Equator Network website ( www.equator-network.org , accessed 9 March 2017). Compliance with the checklist for various types of observational studies will promote transparency concerning methods.
Moreover, over the past decade, formal approaches to grading the quality of evidence and strength of recommendations have gained popularity. The Grading of Recommendations Assessment, Development and Evaluation (GRADE) system evaluates the quality of evidence, including susceptibility to bias, then makes either strong or weak recommendations based on that evidence.
Administrative Databases
Big data invite big problems. The term ‘big data’ denotes large, complex, and linkable information. Poring over databases built for insurance or other purposes has become a burgeoning industry in medical research. Although such databases can monitor trends over time or provide crude measures of frequency, most databases are simply inadequate for credible epidemiological research. Eager researchers often start data dredging without a specified hypothesis and written plan of analysis. The process may degenerate into a scavenger hunt, derisively termed ‘risk factorology’. As Ioannidis has lamented, ‘Risk factor epidemiology has excelled in salami-sliced data-dredged articles’. The resultant spurious associations and false alarms needlessly frighten the public and fill courtrooms with bogus, but remunerative, litigation.
The advantages of administrative databases are readily apparent: the data are already gathered, computerised, and often vast in scope. The advantages of speed, previous data entry, and precision from large numbers are offset by two insurmountable problems: lack of validation of diagnosis and lack of information about potentially confounding factors.
Accurate coding of patient outcomes is mandatory for study validity. Indeed, the US Food and Drug Administration cautions that for drug epidemiology studies using electronic databases, confirmation of the diagnosis requires going to the patient’s medical record: ‘Although validation can be performed using different techniques, the determination of the positive predictive value of a code-based (e.g., ICD) operational outcome definition often involves selecting all or a sample of cases with the codes of interest from the data source and conducting a review of their primary medical data (generally medical charts) to determine whether or not each patient actually experienced the coded event’.
Diagnoses in many administrative databases are invalid, which precludes their use in epidemiological research. For example, an analysis of the Danish Patient Registry suggested differing risk of venous thromboembolism by type of progestin in oral contraceptives. In contrast, large, rigorous, targeted cohort studies have consistently found the risk comparable with all progestins. One explanation is that the diagnosis of venous thromboembolism in that database is often wrong. Indeed, the positive predictive value for diseases and treatments in this database ranges from < 15% to 100%. The problem of invalid diagnoses in administrative databases is widespread. It includes insurance, healthcare, and vital statistics databases.
The second deficiency of administrative databases is the usual lack of information about important potential confounding factors. For example, database studies of venous thrombosis commonly lack information on body mass index, family history of thrombosis, and socioeconomic status. Missing data pose other irremediable challenges. Insurance claims data are often incomplete and error ridden. Diet may play a role in the aetiology of many diseases, including cancer. What information on fruit and vegetable consumption is found on insurance databases or national registries? Missing data are the norm in electronic health records, and these missing data are not missing at random.
Another danger of administrative database studies is ‘mass significance’. Because of large sample sizes, almost any weak association, real or bogus, has a narrow confidence interval and an impressive statistical significance level with lots of zeroes after the decimal point. Big data can find significant differences of no consequence. Trivial, often spurious, associations have achieved statistical significance but have no clinical relevance. Because of inaccurate diagnoses and lack of control for potential confounding, these studies may have precision but not validity. Stated another way, they may be precisely wrong. Administrative databases have a role to play, but the notion that bigger is better in clinical research is demonstrably false.
Weak Associations: Size Matters
Many researchers are unaware of the limitations of their craft. All observational research (and poorly done randomised controlled trials) are susceptible to bias. Even after attempts to minimise selection and information biases and after control for known potential confounding factors, bias often remains. These biases can easily account for small associations. As a result, weak associations (which dominate in published studies) must be viewed with circumspection and humility. Weak associations, defined as relative risks between 0.5 and 2.0, in a cohort study can readily be accounted for by residual bias ( Fig. 7.2 ). Because case-control studies are more susceptible to bias than are cohort studies, the bar must be set higher. In case-control studies, weak associations can be viewed as odds ratios between 0.33 and 3.0 ( Fig. 7.3 ). Results that fall within these zones may be due to bias. Results that fall outside these bounds in either direction may deserve attention.
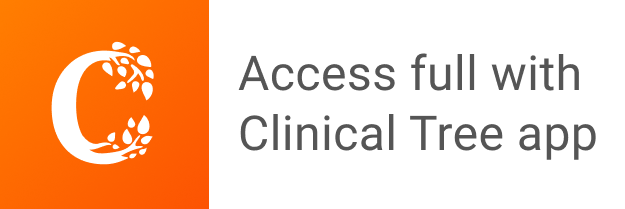