Chapter Contents
Subgroup Analyses 216
What Readers Should Look for With Subgroup Analyses 218
Interim Analyses 219
Early Termination and Biased Estimates of Treatment Effects 221
Stopping for Harm or Futility 222
Other Statistical Stopping Methods 223
What Readers Should Look for With Interim Analyses 223
Conclusion 223
Subgroup analyses can pose serious multiplicity concerns. By testing enough subgroups, a false-positive result will probably emerge by chance alone. Investigators might undertake many analyses but only report the significant effects, distorting the medical literature. In general, we discourage subgroup analyses. However, if they are necessary, researchers should do statistical tests of interaction, rather than analyse every separate subgroup. Investigators cannot avoid interim analyses when data monitoring is indicated. However, repeatedly testing at every interim analysis raises multiplicity concerns, and not accounting for multiplicity escalates the false-positive error. Statistical stopping methods must be used. The O’Brien–Fleming and Peto group sequential stopping methods are easily implemented and preserve the intended α level and power. Both adopt stringent criteria (low nominal p values) during the interim analyses. Implementing a trial under these stopping rules resembles a conventional trial, with the exception that it can be terminated early should a treatment prove greatly superior. Investigators and readers, however, need to grasp that the estimated treatment effects are prone to exaggeration, a random high, with early stopping.
Subgroup analyses have specious appeal. They seem logical and intuitive and even fun—to both investigators and readers. However, this insidious appeal causes important problems. Multiplicity and naivety combine to encourage interpretational missteps in trial conduct and reporting. The subgroup treatment effects revealed in many reports might be illusory.
By contrast, investigators cannot avoid interim analyses if data monitoring is indicated. Neither can they use their normal statistical approaches at interim analyses. Statistical stopping methods, essentially statistical adjustments for warning rather than stopping, must be used in support of data monitoring. Unfortunately, those methods baffle investigators and readers alike. Statistics frequently proves confusing anyway without throwing in second-order complications of stopping methods.
Multiplicity issues from subgroup and interim analyses pose similar problems to those from multiple endpoints and treatment groups ( Chapter 19 ). Investigators frequently data-dredge by doing many subgroup analyses and undertaking repeated interim analyses. Also, researchers conduct unplanned subgroup and interim analyses. Yet some of the approaches to multiplicity problems from subgroup and interim analyses differ from those for endpoints and treatments.
Subgroup Analyses
Indiscriminate subgroup analyses pose serious multiplicity concerns. Problems reverberate throughout the medical literature. Even after many warnings, some investigators doggedly persist in undertaking excessive subgroup analyses.
Investigators define subgroups of participants by characteristics at baseline. They then do analyses to assess whether treatment effects differ in these subgroups. The major problems stem from investigators undertaking statistical tests within every subgroup examined. Combining analyses of multiple subgroups with multiple outcomes leads to a profusion of statistical tests.
Seeking positive subgroup effects (data-dredging), in the absence of overall effects, could fuel much of this activity. If enough subgroups are tested, false-positive results will arise by chance alone.
The answer to a randomized controlled trial that does not confirm one’s beliefs is not the conduct of several subanalyses until one can see what one believes. Rather, the answer is to re-examine one’s beliefs carefully.
Similarly, in a trial with a clear overall effect, subgroup testing can produce false-negative results due to chance and lack of power.
The Lancet published an illustrative example. Aspirin displayed a strongly beneficial effect in preventing death after myocardial infarction ( p < 0.00001, with a narrow confidence interval). The editors urged the researchers to include nearly 40 subgroup analyses. The investigators reluctantly agreed under the condition that they could provide a subgroup analysis of their own to illustrate their unreliability. They showed that participants born under the astrological signs Gemini or Libra had a slightly adverse effect on death from aspirin (9% increase, SD 13; not significant), whereas participants born under all other astrological signs reaped a strikingly beneficial effect (28% reduction, SD 5; p < 0.00001).
Anecdotal reports of support from astrologers to the contrary, this chance zodiac finding has generated little interest from the medical community. The authors concluded from their subgroup analyses that
All these subgroup analyses should, perhaps, be taken less as evidence about who benefits than as evidence that such analyses are potentially misleading.
These and other thoughtful investigators stress that usually the most reliable estimate of effect for a particular subgroup is the overall effect (essentially all the subgroups combined) rather than the observed effect in that subgroup. We agree.
Proper analysis dissipates much of the multiplicity problem with subgroup analyses. Frequently, investigators improperly test every subgroup, which opens the door to chance findings. For example, breaking down age at baseline into four categories yields four tests just on that characteristic ( Panel 20.1 ). A proper analysis uses a statistical test of interaction, which involves assessing whether the treatment effect on an outcome depends on the participant’s subgroup. A test of interaction assesses whether the observed differences in outcome effects across subgroups could be ascribed to chance variation. That not only tests the proper question but also produces a single test instead of four, substantially addressing the multiplicity problem. Investigators have questioned interaction tests based on lack of power. However, interaction tests provide proper caution. They recognise the limited information available in the subgroups and have emerged as the most effective statistical method to restrain inappropriate subgroup findings while still having the ability to detect interactive effects, if present.
Febrile Morbidity | ||||
---|---|---|---|---|
Yes | No | Total | Rate Ratio (95% CI) | |
Age 20–24 Years | ||||
New antibiotic | 11 | 84 | 95 | 1.4 (0.6–3.2) |
Standard antibiotic | 8 | 86 | 94 | |
Age 25–29 Years | ||||
New antibiotic | 8 | 69 | 77 | 1.2 (0.4–3.1) |
Standard antibiotic | 7 | 72 | 79 | |
Age 30–34 Years | ||||
New antibiotic | 3 | 48 | 51 | 0.3 (0.1–0.9) |
Standard antibiotic | 11 | 38 | 49 | |
Age 35–39 Years | ||||
New antibiotic | 10 | 32 | 42 | 1.1 (0.5–2.5) |
Standard antibiotic | 9 | 33 | 42 | |
Total | ||||
New antibiotic | 32 | 233 | 265 | 0.9 (0.6–1.4) |
Standard antibiotic | 35 | 229 | 264 |
The test for statistical interaction (Breslow–Day) is nonsignificant ( p = 0.103), suggesting that a statistically significant subgroup finding in the 30–34 years age stratum is attributable to chance. However, that result, if inappropriately highlighted, would be an example of a superfluous subgroup salvage of an otherwise indeterminate (neutral) trial.
Another problem with subgroup analyses is that investigators can do many analyses and only report the significant ones, which bestows more credibility on them than they deserve—a misleading practice and, if intentional, unethical. This situation is analogous to what we judge a major problem with multiple endpoints.
Subgroup analyses remain a problem in published work. In a review of 50 reports from general medical journals ( New England Journal of Medicine, The Lancet, JAMA, and BMJ ), 70% reported subgroup analyses. Of those in which the number of analyses could be established, almost 40% did at least six subgroup analyses—one reported 24. Fewer than half used statistical tests of interaction. Furthermore, the reports did not provide information on whether the subgroup analyses were predefined or post hoc. The authors of the review suspected that ‘some investigators selectively report only the more interesting subgroup analyses, thereby leaving the reader (and us) unaware of how many less-exciting subgroup analyses were looked at and not mentioned’. Disappointingly, most trials reporting subgroup analyses noted a subgroup difference that was highlighted in the conclusions —so much for cautious interpretation!
In general, we discourage subgroup analyses. If properly undertaken, they are not necessarily wrong. Sometimes they make biological sense or are mandated by sponsors, both public and industry. Four clinical indications for subgroup analyses have been proposed: ‘if there is a potentially large difference between groups in terms of harm that results from treatment; if pathophysiology makes patients from groups differ in their response to treatment; if there are clinically important questions relating to the practical application of treatment; and if there are doubts about the potential benefits of an intervention that results in underuse of this treatment in specific subgroups (for example in elderly patients)’. If done, they should be confined to the primary outcome and a limited number of subgroups. Those planned should be prespecified in the protocol. Investigators must report all subgroup analyses done, not just the significant ones. Importantly, they should use statistical tests of interaction to assess whether a treatment effect differs among subgroups rather than individual tests within each subgroup. This approach alleviates major concerns with multiple comparisons. Rarely should subgroup analyses affect the trial’s conclusions.
Subgroup analyses are particularly prone to over interpretation, and one is tempted to suggest ‘don’t do it’ (or at least ‘don’t believe it’) for many trials, but this suggestion is probably contrary to human nature.
Methodologists have been too restrained in criticising improperly undertaken subgroup analyses. Stronger denunciation is needed.
What readers should look for with subgroup analyses
Readers should be wary of trials that report many subgroup analyses, unless the investigators provide valid reasons. Also, beware of trials that provide a small number of subgroup analyses. They might have done many and just cherry-picked the interesting and significant ones. Consequently, faulty reporting could mean that trials with few subgroup analyses are even worse than the trials with many. Investigators have more credence if they state that they reported all the analyses done. Furthermore, researchers should label nonprespecified subgroup analyses as hypothesis generating rather than confirming. Such findings should not appear in the conclusions.
Readers should expect interaction tests for subgroup effects. Discount analyses built on tests within subgroups. Even with a significant interaction test, readers should base interpretation of the findings on biological plausibility, on prespecification of analyses, and on the statistical strength of the information. Generally, adjustments for multiplicity are unnecessary when investigators use interaction tests. However, in view of the frequently frivolous data-dredging pursuits involved, the argument for statistical adjustments is stronger than that for multiple endpoints. Moreover, if investigators do not use interaction tests and report tests on every individual subgroup, multiplicity adjustments are appropriate. Most subgroup findings tend to exaggerate reality. Be especially suspicious of investigators highlighting a subgroup treatment effect in a trial with no overall treatment effect. They are usually superfluous subgroup salvages of otherwise indeterminate (neutral) trials (see Panel 20.1 ). ‘When the overall result of a major RCT is neutral, it is tempting to search across subgroups to see if there is a particular subgroup in which the treatment effect is favorable. In this context, subgroup claims require an especially cautious interpretation in a journal publication’.
Readers should be the most suspicious of results in which the primary comparison is neutral, the interaction test is statistically significant, and the treatment effects in the subgroups are in opposite directions. This situation is described in a drug versus placebo RCT where the overall result is neutral. ‘Against a background of low-dose aspirin, 15,603 patients at high risk of atherothrombotic events were randomized to clopidogrel or placebo. Over a median 28 months, incidence of the primary endpoint (CV death, MI, or stroke) was 6.8% versus 7.3% (p = 0.22). But, in symptomatic patients (78% of all patients), the findings for clopidogrel looked better: 6.9% versus 7.9% (p = 0.046). In contrast, the results trended in the opposition [sic] direction in asymptomatic patients: 6.6% versus 5.5% (p = 0.02). The interaction test had p = 0.045, and the authors’ conclusions included a claim of benefit for clopidogrel in symptomatic patients’. Such qualitative interactions, where the treatment effects are in opposite directions among subgroups, are usually biologically implausible and seldom occur in clinical medicine. An editorial stated that ‘the charisma of extracting favourable subgroups should be resisted’, and the New England Journal of Medicine stiffened its policy on reporting subgroup analyses.
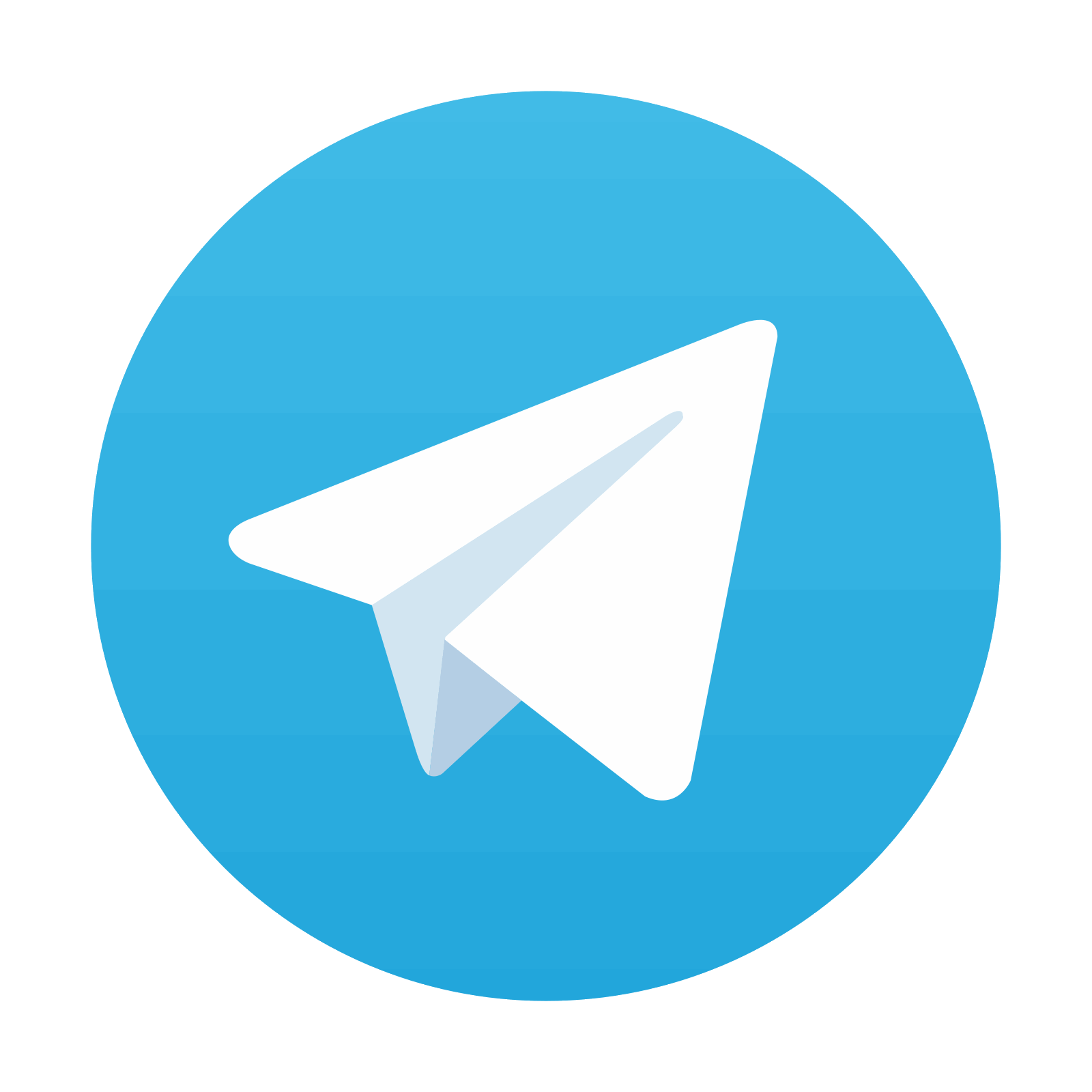
Stay updated, free articles. Join our Telegram channel

Full access? Get Clinical Tree
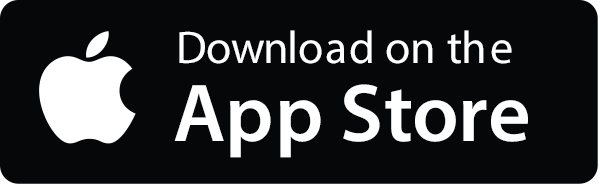
