Fig. 18.1
Examples of MEArray platforms
While these array platforms create caricatures of in vivo microenvironments, they enable researchers to functionally define molecular components that maintain adult and embryonic stem cells, thus revealing molecular regulators and pathways of the stem cell state. We predict this type of functional cell-based dissection of combinatorial microenvironments will have particular high impact in understanding normal and malignant human stem cells, because in vivo experiments are essentially impossible. For instance, putative niche proteins and other tissue-specific proteins have been identified using MEMA, and validated in vivo in some cases, that were relevant to human embryonic [22, 39], neural [24], mammary [23, 25], and hepatic stem cells [38]. MEMA also were used to profile cell-ECM adhesion biases [40] and to optimize growth conditions of cultured cells [41]. Taking a combinatorial approach, relative to a candidate-based approach, allows screening combinations of multiple tissue-specific microenvironment proteins to identify extracellular cues that are the basis for emergent cell behaviors. Functional roles for a number of molecules known to be expressed in human mammary gland and brain, but hitherto had not been ascribed respective roles for mammary or neural stem and progenitor cell regulation, were discovered using this type of approach. The successful application of MEMA requires managing a number of technical details that are, in many cases, on the edge of discovery themselves. The remainder of this chapter will elaborate on some of the issues that arise most often when producing MEMA on 2-D substrates and provide some discussion of how we are managing them. There are relatively fewer examples of MEMA-type platforms in 3-D, perhaps because some of the high-throughput liquid handling and 3-D imaging requirements raise the barrier to entry; however, an excellent example of 3-D MEMAs is available in Ranga et al. [34].
Selecting the Printing Substrate: It Depends on the Biological Questions Being Asked
There are numerous materials used to immobilize proteins, but the primary objective remains the same where MEMA fabrication is concerned: a suitable surface coating for printing proteins upon should provide high adsorption capacity, low cell attachment in areas not printed with proteins (i.e., non-fouling), and low spot-to-spot variation. Other important considerations include the capacity to retain protein structure, functionality, and binding sites.
The most commonly used approaches are to chemically modify surfaces of glass slides, e.g., with aldehydes or epoxies, or to coat them with very thin layers of polymers such as polydimethylsiloxane (PDMS) . Slides with these surfaces adsorb proteins with either covalent bonds or strong electrostatic interactions, respectively. Covalent modifications provide irreversible attachment; however, protein 3-D structures may not be well maintained. Unintended cell attachment also can be problematic with the chemically modified glass and with the hydrophobic PDMS without the addition of non-fouling coatings, like Pluronics F108 or bovine serum albumin. Another option is to coat glass surfaces with polyacrylamide (PA) or poly(ethylene glycol) (PEG) hydrogels. These hydrogels physically absorb proteins through relatively weak electrostatic interactions, which retain most of the native protein conformation, but there is higher variation in protein-binding capacity [42]. One of the most convenient properties of PA and PEG gels is their native non-fouling character, which removes any problems of nonspecific cell attachment.
Rigidity of the substrate is another important property to consider. PDMS is inexpensive, and its elastic modulus is easy to manipulate by altering the cure: polymer ratio, covering a range of elastic modulus similar to cartilage, skin, and tendon (0.6–3.5 MPa). PEG represents a range of elastic moduli from 500 kPa to 1.6 GPa. PA is another inexpensive substrate, which can be tuned from 150 Pa to 150 kPa, which is closer to the biological microenvironment for soft tissues like brain and breast [43]. Which substrate for protein immobilization should be used ultimately depends upon the characteristics of the cells used, the tissue being mimicked, and the outcomes being measured.
MEMA Data Analysis: Seeing the Forest for the Trees
A main goal of MEMA-type experiments is to provide causal links between cellular responses and specific microenvironments. Both inter- and intra-microenvironment heterogeneity of cellular responses are to be expected and can be instructive about the continuum of phenotypic plasticity within the experimental system. Measuring heterogeneity of drug responses in a diversity of contexts may result in more realistic expectations of drug responses in vivo. By incorporating sufficient numbers of replicate features into the design of a MEMA, significant associations between microenvironments and cell phenotypes can be identified, but the high dimensionality of the data is a hindrance to extraction of meaningful information. Most MEMA platforms use fluorescent probes to visualize biochemical and functional phenotypes and fluorescent and phase microscopy to capture morphological and colorimetric phenotypes. There are no specialized high-throughput imaging systems for this type of work currently available; however, microarray scanners and programmable, motorized epifluorescence or laser scanning confocal microscopes have been successfully used to acquire the necessary images [23, 34]. Micrographs of cells attached to the arrayed microenvironments can be treated as ensemble data, i.e., averaging the signal from many cells on one spot in a manner similar to DNA arrays, or as single-cell data when used in combination with cell segmentation algorithms. Even in cases where MEMA are designed to have fairly low complexity, e.g., 100 or fewer unique microenvironment combinations, the analytical challenges are significant. The complexity of the information space generated from MEMA experiments increases rapidly when taking into consideration multiple microenvironmental properties such as rigidity, geometry, and molecular composition. In practice, the statistical analysis of MEMA experiments is a rate-limiting step for this technology, and there are multiple solutions for addressing this challenge. The basic data processing workflow for MEMA experiments includes: signal normalization, identifying functionally similar microenvironments by clustering, dimension reduction, data visualization, and further pathway analysis. Table 18.1 shows some suggested software packages that aid with analyses of MEMA-type data, with comments on specific strengths and weaknesses.
Table 18.1
Software for processing microarray data
Software | Application | Advantages | Limitations |
---|---|---|---|
ImageJ | Image processing | Easy to use, batch processing | Needs Java to improve automation |
Fiji (ImageJ 2) | Image processing | Built-in plugins specifically for biological data, batch processing | Needs Java to improve automation |
Cell Profiler | Image processing | No coding needed and better native automation compared to ImageJ or Fiji | Less customization compared to Matlab |
Matlab | Image processing | Highly customizable for image processing | Needs intensive coding |
Excel | Data processing | Easy to use and very limited coding needed | Difficult to process large data sets. Limited visualization choices |
R | Data processing | Handles very large data sets | Needs intensive coding |
Python | Data processing | Easier to use compared to C ++ and can be integrated with other software, such as R | Needs intensive coding |
Data Normalization
All microarray-like data contain some useful information and a significant degree of noise; thus, proper normalization is crucial. The data analysis begins with measuring fluorescence intensity or colorimetric density of each target protein in cells on each array feature. In this context, intensity typically reflects the relative abundance of the target protein. Intensities are impacted by factors such as the characteristics of the dye (antibody), spatial location, and uneven surfaces of the slides that cause inconsistent background [44]. Unlike DNA microarrays, which load the same amount of cDNA onto the array and then uses total intensity as an internal reference, the number of cells attached on MEMA features varies by microenvironment. Thus, we may use the average of the total signal from all cells on all array features as a reference for normalization of arrays of the same treatment condition. A signal emanating from cells on a control microenvironment, which is known a priori to reproducibly bias toward a given phenotype, can be used as a reference [23]. An alternative is to use spots that contain the same amount of fluorescence molecules and should have the same intensity as an internal control printed on each array.
Statistical Considerations
The main purpose of MEMA experiments is to identify the specific microenvironments that modulate certain cellular functions by comparing cellular phenotypes between treatments and controls. Table 18.2 shows some methods, which have been used for processing MEMA data. Compared to using Student’s t-test, a widely used statistical test in biological research, Dunnett’s test is a better option for correcting false P values due to multiple comparisons and identifying microenvironments that impose phenotypes that are significantly different from the control [23]. The Z-score standardization is a simple method used to identify meaningful groups that are distinct from the global mean. Z-scores have been used successfully to identify and optimize better culture conditions for rare cell populations [45]. However, the Z-score has several limitations, like skewing of values due to outliers within a data set as well as decreased accuracy when cell numbers are reduced. Moreover, the Z-score is based on the assumption that the data fit a Gaussian distribution, which is not the case in many biological systems. Thus, Guyon et al. proposed the Φ-score as a cell-to-cell phenotypic scoring method for selecting the hit discovery in cell-based assays. The Φ-score ranks cells instead of averaging them and shows performance that surpasses the Z-score for coping with the above limitations. Indeed the Φ-score can be more sensitive (more true hits) and more specific (fewer false positives) compared to other conventional methods [46].
Table 18.2
Data analysis and visualization techniques used with MEArray-type data
Methods | Type | Advantages | Limitations | References |
---|---|---|---|---|
Z-score | Normalization | Easy to implement even in excel | Sensitive to outlier values, and less accurate in cases with few cell numbers | [45] |
Φ-score | Normalization | Overcomes the limitations of Z-score | Needs specialized software for implementation, such as R | [46] |
Dunnett’s test | Statistical test | Overcomes problems with type I errors (false positives) due to multiple comparisons to a single control | Does not make all pair-wise comparisons | [23] |
PCA | Dimension reduction | A simple method to PCA to identify patterns due to variance | Only reflects linear relationships | [47] |
ICA | Filter noise and data separation | An alternative method to identify patterns and filter noise | Data needs to be non-Gaussian distribution and independent to each other | [48] |
IPCA | Filter noise and dimension reduction | A method combined PCA and ICA to identify patterns | Similar to ICA, certain assumptions are needed | [49] |
SPADE | Visualization | Identifies patterns in high dimensional data | Lower resolution compared to ViSNE, needs further statistical tests for validation | [50] |
ViSNE | Visualization | Similar to SPADE but has higher resolution. Can reflect nonlinear relationships | Needs further statistical tests for validation | [51] |
Clustering methods commonly used for DNA microarray data sets, such as hierarchical or k-means clustering, also are used with MEMA data to separate meaningful groups. Konagaya et al. interrogated a relatively small number of growth factor combinations to optimize neural progenitor cell culture microenvironments and then used hierarchical cluster analysis to reveal three major clusters of microenvironment combinations that facilitated growth versus astrocyte or neuron differentiation [41]. Although these analyses can reveal the meaningful groups within simple data sets, like traditional two-color DNA microarray data, the difficulty and challenge of data clustering arise rapidly in multidimensional data sets [52]. The phrase, “the curse of dimensionality” [53], described the general phenomenon that data analysis techniques, which work well at lower dimensions, are often unable to perform as well when the dimensionality of data are increased. To overcome some of these difficulties, dimension reduction techniques have been developed.
Dimension Reduction and Data Visualization
Due to improvements in computational processing power, we now are able to better deal with high-dimensional data and with algorithms that do not make painful compromises in the name of efficiency. Dimension reduction essentially distills vast amounts of information into snap shots that are emblematic of the underlying biology.
Principal component analysis (PCA) is used for dimension reduction and can reveal the most variable factors that contribute to certain phenotypes [47]. However, not all biological questions are related to the variables with highest variance in the data set, and in these cases, PCA is less able to identify the contributing factors. Thus, independent component analysis (ICA) is an alternative to PCA, particularly when some certain characteristics of the data are known, allowing the assumption that the observed data are separated into groups that are independent of each other [48]. An example of a case where ICA has been applied is the cocktail party problem, describing the human ability to selectively recognize speech sounds that are often assumed to be independent from each other in noisy environments [54]. However, the need to make assumptions about the data and to choose the number of components analyzed is a limitation of ICA, particularly in high-dimensional data sets where we may not fully understand the relationships between variables. Due to this limitation, Yao et al. proposed independent principal component analysis (IPCA) combining the advantages of PCA and ICA, where they applied PCA as a preprocessing step to extract components for subsequent analysis and then applied ICA to filter out noise [49].They assumed that microarray-based gene expression measurements that follow a Gaussian distribution represented noise (i.e., most of the genes are not expected to change at a given condition), and they showed that IPCA was better able to reveal patterns within those biological data [49]. All of these approaches are used in microarray analysis, but they often suffer from preserving important information during data reconstruction when trying to analyze high-dimensional single-cell data. Linear techniques such as PCA focus on separating dissimilar data points far away in low-dimensional representations after data transformation. However, biological data is often nonlinear, and for high-dimensional data, it is usually more important to keep similar data points close together in low-dimensional representations, which is typically not feasible with linear mapping techniques [55].
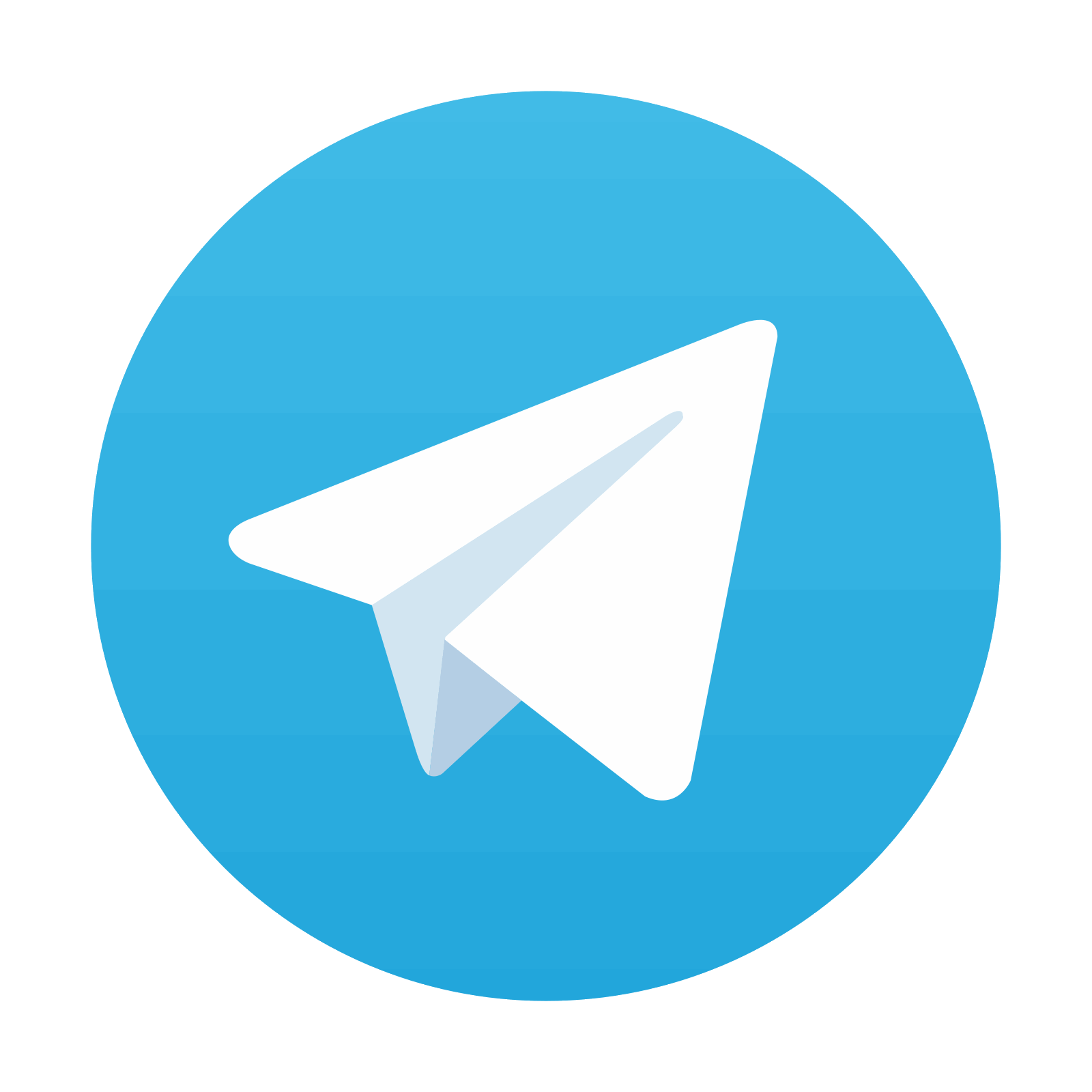
Stay updated, free articles. Join our Telegram channel

Full access? Get Clinical Tree
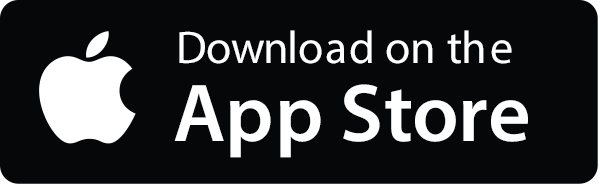
