Fig. 2.1
The structure of the therapy imaging and model management system (TIMMS) that may provide much of the IT framework for personalized medicine. The kernel for workflow and knowledge and decision management, the patient specific models repository and the process models repository are the central components for the development of digital patient models through the process of patient-specific modeling (Lemke and Golubnitschaja The EPMA Journal 2014, 5, p. 8). (Legend: ICT Information and Communication Technology; WF Workflow; K +D knowledge and decision; Rep Representation; Mechatr Mechatronic)
1.
Standardized interfaces for communication and mechatronics (such as robotics), thereby creating a unified environment for the input and output of data (including the representation and display of information and images, as well as the electromechanical control of surgical and navigational devices);
2.
Creation and maintenance of a Digital Patient Model (DPM) based on generic Patient-Specific Models (PSMs) modified by the addition of patient-specific data, thereby providing a multi-scalar, comprehensive, precise, personalized representation of the patient;
3.
Creation and maintenance of a system for Process Modeling of all aspects of the surgical workflow, to ensure efficiency, learning, and safety throughout operative procedures;
4.
Real-time knowledge management and decision support system (Kernel for Workflow and Knowledge and Decision Management) thereby promoting optimized diagnostic, prognostic, and therapeutic decisions throughout the treatment workflow. The key role here is to assist and optimize the practice of medicine in the face of incomplete medical knowledge, which is an inherent part of clinical practice.
5.
Validation system, thereby providing quality assurance, patient safety, system security, and processing of medical evidence.
There are seven TIMMS engines that may be defined as software modules that can be executed on an appropriate computing machine to provide functionalities. These engines relate to imaging and biosensor data acquisition, modeling, simulation, workflow and knowledge and decision management, visualization, intervention, and validation.
The TIMMS engines, repositories, and IT infrastructure initially were designed to facilitate model-guided surgical therapy. Subsequently, the TIMMS structures and functions have been generalized to apply to all forms of model-based medical management (suitable for patients with cancer, diabetes, etc.) and may be abbreviated MIMMS (Medical Imaging and Model Management System).
For the purposes of developing a working TIMMS or MIMMS for PPPM, it is necessary to understand the functions of the central TIMMS engine (Kernel for Workflow and Knowledge and Decision Management; Kernel), the Modeling Engine, and their substrate, the DPM.
The Kernel provides the strategic intelligence for therapeutic planning and workflow execution. Often this module (or parts thereof) is integrated into some of the other engines, as the need demands. This important computing Kernel (or “brain”) of the system may use different forms of logic, different database structuring, agents, machine learning, and other forms of artificial intelligence, depending on the specific applications of the procedure or procedures being performed. Agents may be defined as software modules, containing some form of intelligence, which, with some degree of autonomy and adaptability, carry out functions or tasks. A full description of the role of the Kernel is beyond the scope of this book, but attention will be focused on the design, development, and management of the DPM. The use-case, as developed in this book, will focus on the requirements for a DPM to facilitate the management of patients with HCC.
2.1.2 Digital Patient Model
In every day terms, the DPM may be thought of as a representation of the patient, composed of both the static and dynamic features of the human organism, maintained within a multi-layered database structure [2]. Certain features are relatively stable, such as the DNA sequence within the chromosomes, or a radiograph of an extremity, while other features are in a constant state of fluctuation, such as pulse and respiration. In addition, the clinical significance of physiologic fluctuations may vary in different clinical settings. Thus, the DPM must be capable of managing a constellation of interacting static and fluctuating causes and effects of varying degrees of certainty, predictability, and significance. As the database of knowledge underlying the creation and maintenance of DPMs increases, a statistically valid tool that increases in accuracy as more data are added (to be discussed below), will be available for assistance in medical and surgical management in the face of incomplete medical knowledge.
Ideally, the DPM has the capabilities to capture patient specific information in a way that facilitates evaluation and application of that information:
a.
The DPM can receive and process information from an unlimited number of sources, and at a rate required for real-time activity.
b.
The DPM is comprehensive, yet flexible enough to be extensible at any time.
c.
The information contained within the DPM can be extracted by a wide variety of selection parameters, templates, and constellations.
d.
Extraction is flexible and controllable by the end-user and researchers.
e.
Extraction may take in a variety of formats or representations—charts, tables, lists, images, graphs, and models.
f.
The DPM allows and facilitates simulations, prognosis, prediction, and therapy planning. One of the key functions of the DPM is to allow simulated evaluation of hypothetical, alternative therapies to predict and optimize patient outcomes, and to anticipate adverse effects of the proposed therapies.
g.
The DPM allows comparisons and compilations with other DPMs to collect Model-Based Medical Evidence, Population-Based Medical Evidence, and Disease-Based Medical Evidence.
h.
The DPM facilitates actions with workflows, algorithms, and databases.
Features of the patient that are amenable to graphical representation are maintained as references within the DPM database structure to Picture Archiving and Communications Systems (PACS) and repositories that allow access to the actual images, for example, through functionalities of a suitable IT infrastructure for simulations and interventions. This allows medical images to be loaded into advanced medical workstations for purposes of treatment planning and simulation, as well as allowing real-time interaction with hardware and software for image-guided interventions, such as radiation therapy and minimally invasive therapies.
Those features of the DPM that are measurable and/or have cause-and-effect relationships, and can be quantified may be dealt with in a different manner than non-quantifiable features, such as constitution and appearance. In Bayesian terms, these quantifiable features, or entities, may be thought of as a dynamic set of data elements, i.e. attributes with interconnected and fluctuating probability distributions.
The probability distribution of each attribute or variable reflects and represents the state of uncertainty associated with the knowledge about a particular feature of an individual patient. The existence of relationships among attributes, represented by appropriate links and their binding strength, are also subject to probability distributions. The value of each attribute probability distribution lies within a statistically definable range of normal and abnormal values. The boundaries of the values for each attribute, and the volatility of the changes of these values, vary in health and disease, and at different ages, and may be subject to further alterations based on the body’s homeostatic mechanisms, as well as constitutional, genetic and epigenetic, and environmental factors, including prior medical and surgical interventions. It is therefore reasonable to assume that the described situation surrounding the patient is amenable to be represented by a form of Bayesian Network. However, standard Bayesian Networks that have been utilized in previous medical decision support and knowledge management systems are inadequate for this purpose. A Multi-Entity Bayesian Network (MEBN) [3] is available that can overcome the limitations of standard Bayesian Networks.
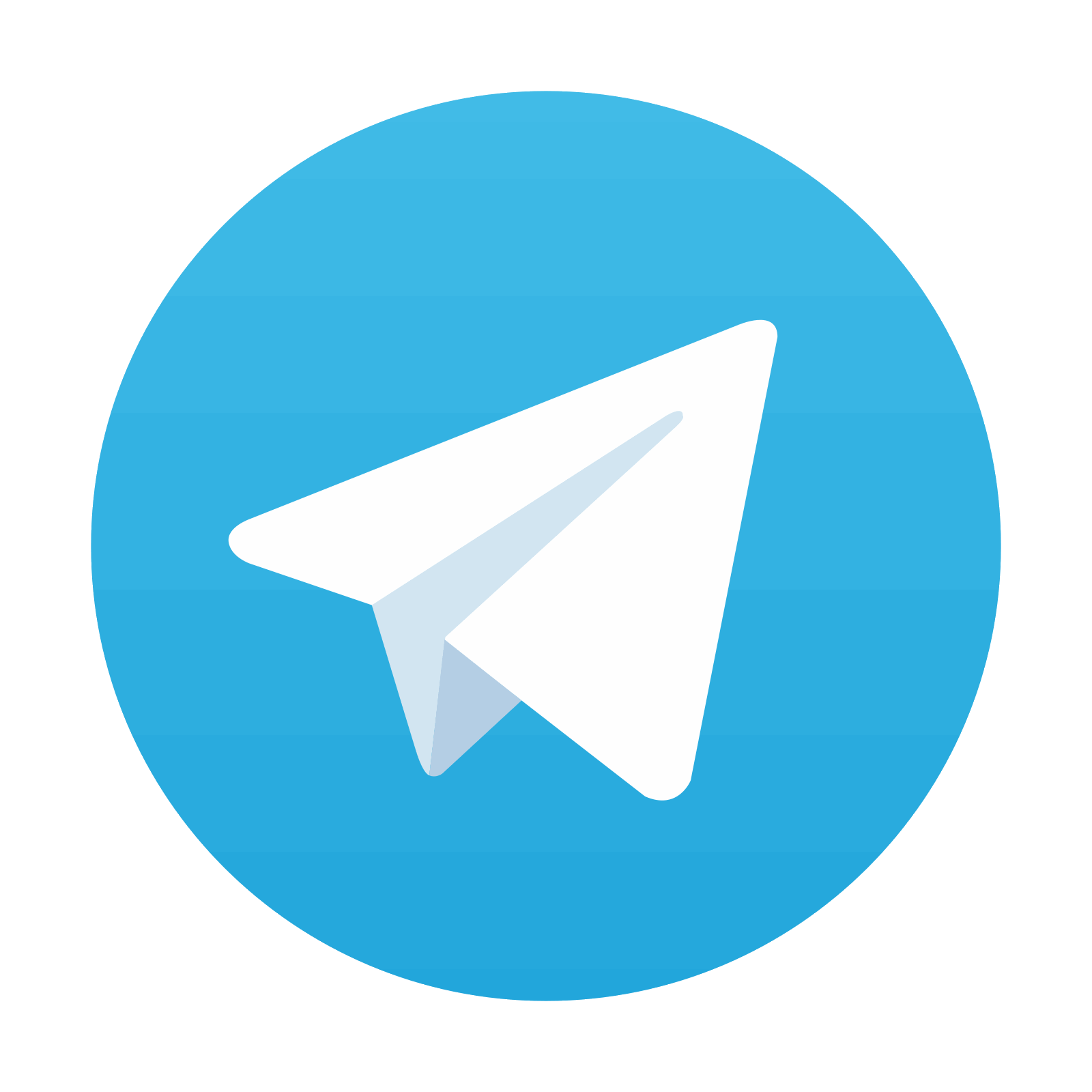
Stay updated, free articles. Join our Telegram channel

Full access? Get Clinical Tree
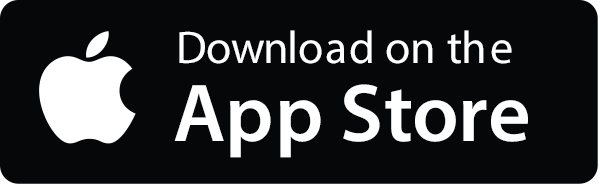
