Survival data are concerned with the time it takes an individual to reach an endpoint of interest (often, but not always, death) and are characterized by the following two features.
- It is the length of time for the patient to reach the endpoint, rather than whether or not she or he reaches the endpoint, that is of primary importance. For example, we may be interested in length of survival in patients admitted with cirrhosis.
- Data may often be censored (see below).
Standard methods of analysis, such as logistic regression or a comparison of the mean time to reach the endpoint in patients with and without a new treatment, can give misleading results because of the censored data. Therefore, a number of statistical techniques, known as survival methods 1, have been developed to deal with these situations.
Censored Data
Survival times are calculated from some baseline date that reflects a natural ‘starting point’ for the study (e.g. time of surgery or diagnosis of a condition) until the time that a patient reaches the endpoint of interest. Often, however, we may not know when the patient reached the endpoint, only that she or he remained free of the endpoint while in the study. For example, patients in a trial of a new drug for HIV infection may remain AIDS-free when they leave the study. This may either be because the trial ended while they were still AIDS-free, because these individuals withdrew from the trial early before developing AIDS, or because they died of non-AIDS causes before the end of follow-up. Such data are described as right-censored. These patients were known not to have reached the endpoint when they were last under follow-up, and this information should be incorporated into the analysis.
Where follow-up does not begin until after the baseline date, survival times can also be left-censored.
Displaying Survival Data
A separate horizontal line can be drawn for each patient, its length indicating the survival time. Lines are drawn from left to right, and patients who reach the endpoint and those who are censored can be distinguished by the use of different symbols at the end of the line (Fig. 44.1). However, these plots do not summarize the data and it is difficult to get a feel for the survival experience overall.
Figure 44.1 Survival experience of 105 patients following admission with cirrhosis. Filled blank circles indicate patients who died, open circles indicate those who remained alive at the end of follow-up.

A survival curve, usually calculated by the Kaplan–Meier method, displays the cumulative probability (the survival probability) of an individual remaining free of the endpoint at any time after baseline (Fig. 44.2). The survival probability will only change when an endpoint occurs, and thus the resulting ‘curve’ is drawn as a series of steps, starting at a survival probability of 1 (or 100%) at baseline (time 0) and dropping towards 0 as time increases. We may also display the cumulative incidence of the endpoint; this is calculated as (1 − survival probability) at each time point and the resulting curve is the inverse of the survival curve (i.e. it starts at a survival probability of 0 and moves up towards 1 as time increases). Although the information contained in both displays is the same, the cumulative incidence curve is generally preferred to the cumulative survival curve when the endpoint is rare (and so the survival probability remains high throughout the study) as this allows maximum detail to be shown without a break in the scale. An alternative method of calculating survival probabilities, using a lifetable approach, can be used when the time to reach the endpoint is only known to within a particular time interval (e.g. within a year). The survival probabilities using either the Kaplan–Meier or lifetable approaches may be obtained easily from most statistical packages.
Figure 44.2 Kaplan–Meier curves showing the survival probability, expressed as a percentage, following admission for cirrhosis, stratified by baseline HVPG measurement (see Example).

Summarizing Survival
We often summarize survival by quoting survival probabilities (with confidence intervals) at certain time points on the curve, for example, the 5-year survival rates in patients after treatment for breast cancer. Alternatively, the median time to reach the endpoint (the time at which 50% of the individuals have progressed) can be quoted.
Comparing Survival
We may wish to assess the impact of a number of factors of interest on survival, e.g. treatment, disease severity. Survival curves can be plotted separately for subgroups of patients; they provide a means of assessing visually whether different groups of patients reach the endpoint at different rates (Fig. 44.2). We can test formally whether there are any significant differences in progression rates between the different groups by, for example, using the log-rank test or regression models.
The Log-Rank Test
This non-parametric test addresses the null hypothesis that there are no differences in survival times in the groups being studied, and compares events occurring at all time points on the survival curve. We cannot assess the independent roles of more than one factor on the time to the endpoint using the log-rank test.
Regression Models
We can generate a regression model to quantify the relationships between one or more factors of interest and survival. At any point in time, t, an individual, i, has an instantaneous risk of reaching the endpoint, often known as the hazard or λi(t), given that she or he has not reached it up to that point in time. For example, if death is the endpoint, the hazard is the risk of dying at time t. This instantaneous hazard is usually very small and is of limited interest. However, we may want to know whether there are any systematic differences between the hazards, over all time points, of individuals with different characteristics. For example, is the hazard generally reduced in individuals treated with a new therapy compared with those treated with a placebo, when we take into account other factors, such as age or disease severity?
We can use the Cox proportional hazards model to test the independent effects of a number of explanatory variables (factors) on the hazard. It is of the form
where λi(t) is the hazard for individual i at time t, λ0(t) is an arbitrary baseline hazard (in which we are not interested), x1, …, xk are explanatory variables in the model and β1, …, βk are the corresponding coefficients. We obtain estimates, b1, …, bk, of these parameters using a form of maximum likelihood known as partial likelihood. The exponential of these values (i.e. ) are the estimated relative hazards or hazard ratios. For a particular value of x1, the hazard ratio is the estimated hazard of disease for (x1 + 1) relative to the estimated hazard of disease for x1, while adjusting for all other x’s in the equation. The relative hazard is interpreted in a similar manner to the odds ratio in logistic regression (Chapter 30) or the relative rate in Poisson regression (Chapter 31); therefore values above one indicate a raised hazard, values below one indicate a decreased hazard and values equal to one indicate that there is no increased or decreased hazard of the endpoint. A confidence interval can be calculated for the relative hazard and a significance test performed to assess its departure from one.
The relative hazard is assumed to be constant over time in this model (i.e. the hazards for the groups to be compared are assumed to be proportional). It is important to check this assumption1: we can, for example;
- use graphical methods – the simplest approach is to check that the two or more curves corresponding to the categories of a single covariate in a Kaplan–Meier survival plot move apart progressively over time. Alternatively, a plot of ln[−ln(survival probability)] versus ln(time) for each category of the covariate (sometimes referred to as a log log plot, and available in most statistical packages) should exhibit roughly parallel lines. In particular, lines that cross indicate a serious deviation from proportional hazards;
- incorporate an interaction between the covariate and ln(time) in the model and ensure that it is non-significant;
- perform a formal test, such as the global Chi-squared test based on Schoenfeld residuals, usually available in statistical packages.
If the proportional hazards assumption is violated, it may be possible to split the follow-up time into two or more intervals over which the hazards are known to be proportional. We can then perform a separate Cox regression analysis in each interval and report the results from each.
Other models can be used to describe survival data, e.g. the Exponential, Weibull or Gompertz models, each of which assumes a specific probability distribution for the hazard function. Frailty models are used when the observations on survival are not independent (i.e. there is correlation within clusters, such as within geographical areas because of shared environmental factors). However, all these models are beyond the scope of this book1.
Problems Encountered in Survival Analysis
Informative Censoring
In any survival analysis we make the assumption that the probability that an individual’s follow-up is censored is independent of (i.e. unrelated to) the probability that the individual will develop the outcome of interest (e.g. death). For example, an individual’s follow-up may be censored because she or he moved from the area and was lost to follow-up; at the time of censoring, this person has the same chance of developing the outcome of interest as individuals who had been followed for the same period of time but whose follow-up was not censored. Where this assumption is violated, we say that we have informative censoring (and we must accommodate this in our statistical analysis). For example, in a study of the survival of patients with acute liver failure, patients who undergo liver transplantation may be withdrawn from the study early and their follow-up censored. As these individuals are likely to have a different prognosis to those who did not undergo transplantation, their follow-up will have been informatively censored. Administrative censoring, whereby patient follow-up is censored simply because the study ends on a particular date, is generally non-informative.
Competing Risks
Occasionally, a study may have a number of different outcomes of interest. If the development of one or more of these outcomes precludes the development (or measurement) of any of the others, the outcomes are termed competing risks. For example, individuals with HIV infection are diagnosed as having AIDS once they experience any one of a list of clinical events (including Pneumocystis carinii pneumonia, Kaposi’s sarcoma and cytomegalovirus retinitis). If we are interested in assessing the risk factors for a specific clinical condition (e.g. Kaposi’s sarcoma) as an AIDS-defining event (the outcome of interest) then the development of any of the other listed conditions will act as a competing risk (i.e. once the patient has developed one of the other conditions, she or he will be diagnosed with AIDS and a subsequent development of Kaposi’s sarcoma will no longer be AIDS-defining). An analysis of the risk factors associated with Kaposi’s sarcoma as a first AIDS-defining event may reach different conclusions from an analysis of the risk factors for Kaposi’s sarcoma whether it occurs as a first or subsequent event.
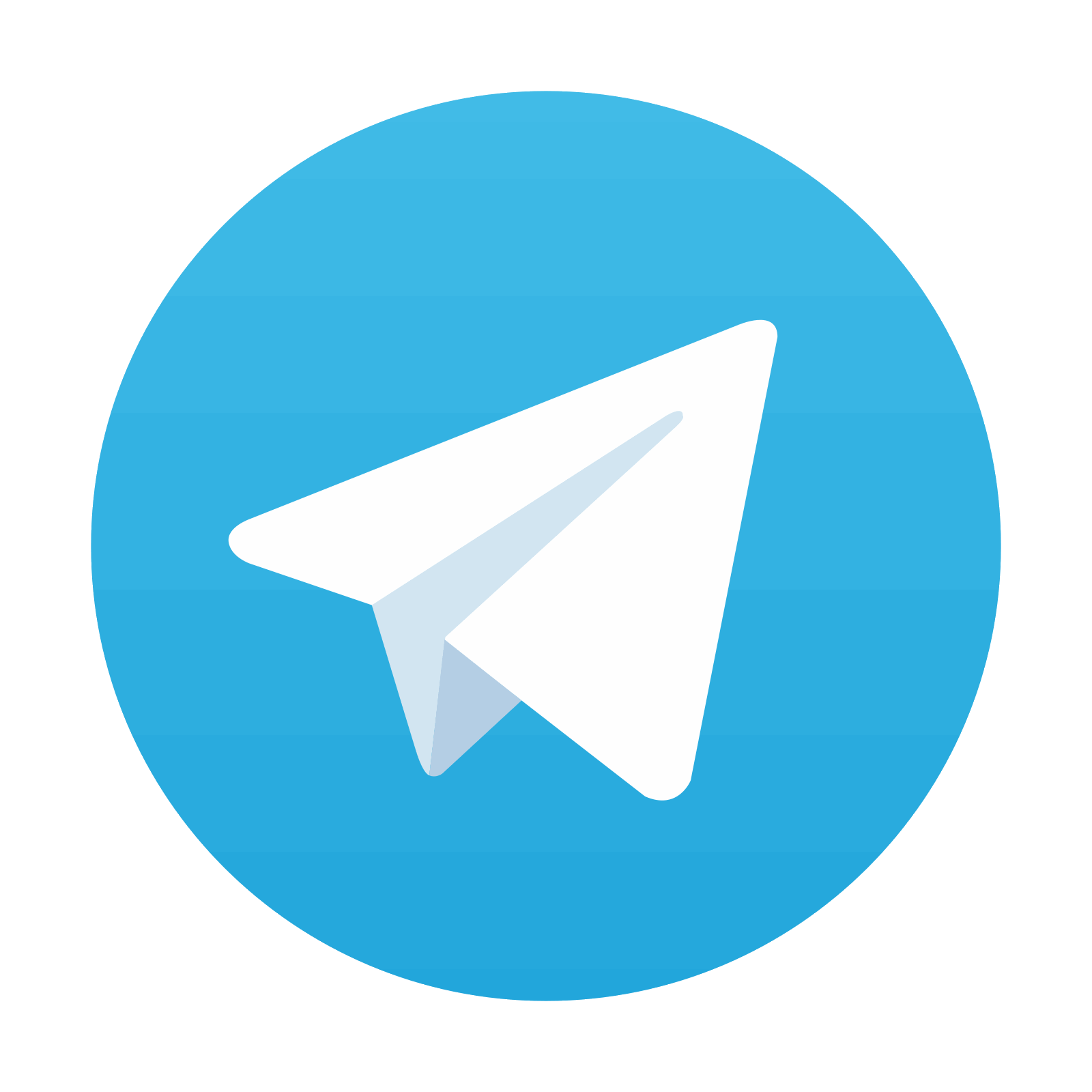
Stay updated, free articles. Join our Telegram channel

Full access? Get Clinical Tree
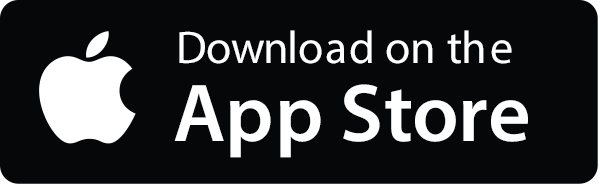
