html xmlns=”http://www.w3.org/1999/xhtml” xmlns:mml=”http://www.w3.org/1998/Math/MathML” xmlns:epub=”http://www.idpf.org/2007/ops”>
In this chapter we discuss predictor and confounding variables, two categories of variables that are assumed to influence the values of the outcome variables. The difference is sometimes subtle and depends on the stated aims of the study. The effects of the predictor variables on the outcome are the focus of the study, whereas confounding variables are nuisance variables that must be considered but are not of main interest. Like outcome variables, predictor and confounding variables can be direct measurements, recoded values of measured variables, or composites derived from several variables. Like outcome variables, they should be specified in advance in the protocol.
16.1 Predictor Variables
We use the word “predictor” in a very general sense. It does not mean that the value of the predictor(s) will give you an exact value for the outcome. Rather, we assume that the value of the predictor(s) will influence the value of the outcome. From the statistical analysis you can obtain measures to estimate the strength of the predictor on the magnitude or other characteristics of the outcome. In an interventional study, the predictor variables are usually the ones that are manipulated as part of the intervention, and the objective of the study is to see if and how these manipulations affect the outcome. In an observational study, the exposures are the predictor variables. However, predictors may also include variables that describe the participant, such as age, sex, or ethnicity, when these are considered important to the outcome.
Example 15J described a randomized parallel group study to determine the efficacy of several different HIV treatments against each other. The primary predictor variable in this study is the treatment, assigned randomly by a computer program when participants are enrolled.
In Example 15G, a cohort study investigating whether participants who exercise before surgery have a better outcome after undergoing joint replacement than those who do not, the primary predictor was the amount of exercise the participant did prior to the surgery. Because this was not controlled by the investigator, it was necessary to develop standards to classify the type and amount of exercise. The most inclusive categories were endurance, strength training, both, or no exercise. The amount of time was estimated in terms of total hours per week and the number of separate occasions per week. Both the actual times and classifications such as infrequent, moderate, and frequent were used in the analysis.
In Example 15D, a multi-center study of depressed patients, the depressed participants were randomized to one of two drugs and followed for four weeks. Since both drugs were known to be effective overall in the treatment of depression, which drug was used was not considered a predictor of overall outcome. The specific aims were to show that the drugs affected different aspects of depression; therefore, the choice of drug was considered a predictor of change in these outcome variables. The drug was also tested as a predictor of change in biochemistry.
In practice, outcome variables can be used as predictor variables, to determine if their values are associated with the values of other outcome variables, but this must be done with caution. If the investigator modifies the value of a predictor variable and then observes an associated change in an outcome variable, it is reasonable to believe in a cause-effect relationship, with the change in the predictor variable causing the change in the outcome variable. However, when the predictor is an outcome variable that was not modified directly by the investigator, the only thing that can be said is that the values are associated. These findings may lead to another study where the investigator controls the value of the variable of interest to provide more evidence of a cause-effect relationship.
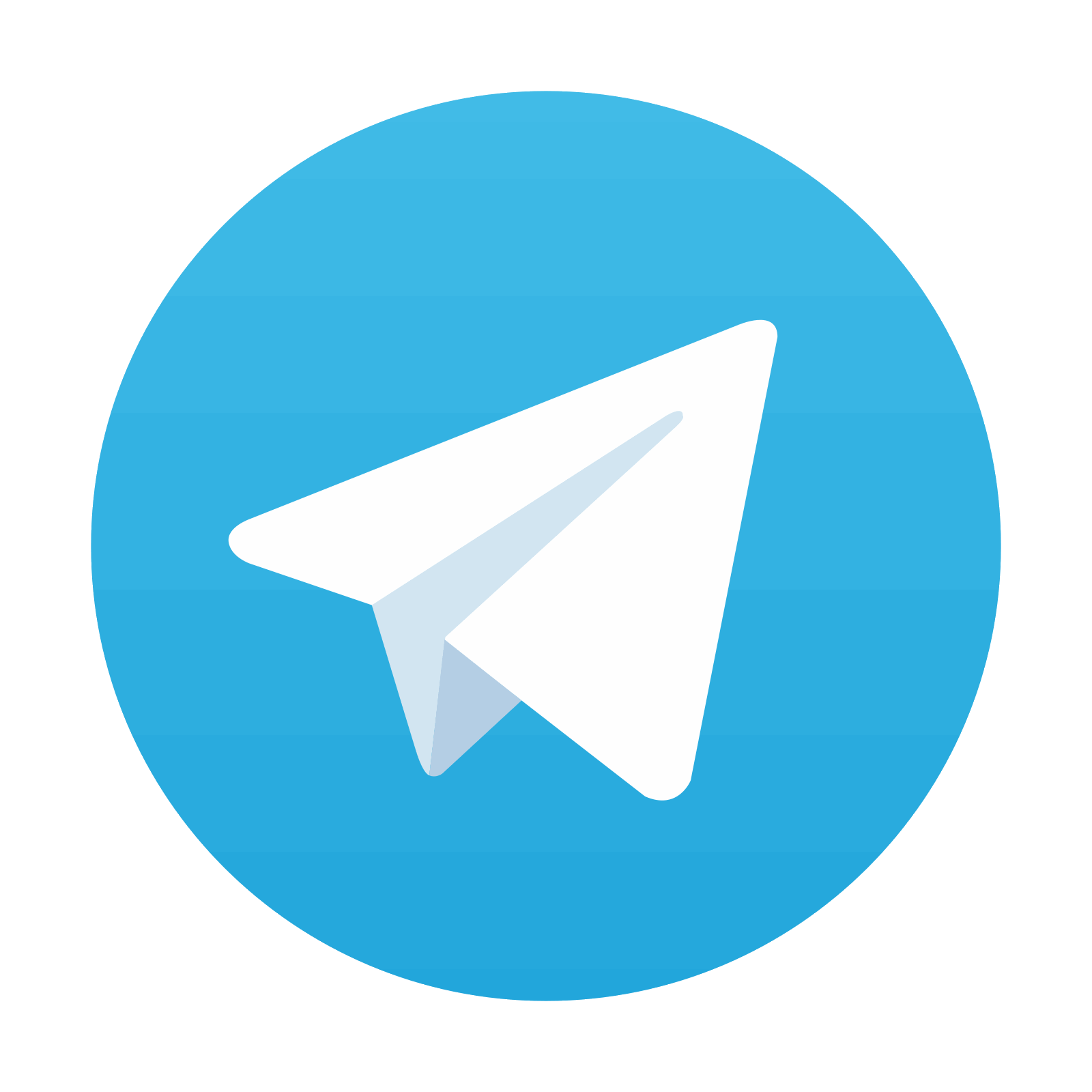
Stay updated, free articles. Join our Telegram channel

Full access? Get Clinical Tree
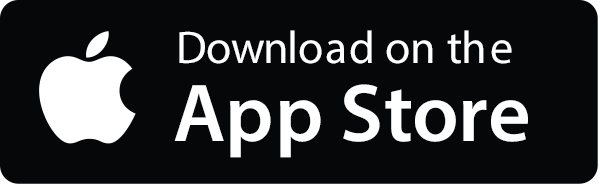
