html xmlns=”http://www.w3.org/1999/xhtml” xmlns:mml=”http://www.w3.org/1998/Math/MathML” xmlns:epub=”http://www.idpf.org/2007/ops”>
A study is about information. Information is obtained from data. Investigators collect data, examine this data, describe the values obtained, and make inferences and conclusions based on this data. The conclusions are the information from the study. The individual items collected are the study variables and the values of these variables, which are collected during the study from each participant, are the study data. As the name implies, variables are the items that can vary between study participants. The variables may have different uses in the study. Although you as the investigator usually know why you are collecting specific variables, an understanding of their role helps formulate analysis plans, understand the implications of statistical findings, and present results. In this chapter we define these different uses of variables. The following two chapters discuss these concepts with detailed examples.
14.1 Types of Variables
The study variables are based on measurements taken in the study. These include physical measurements such as height or weight, biological variables measured through chemical assay, genetic information, and emotional and psychological measurements through questionnaires and visual scales. The variables may be these measurements as taken or may be new variables derived from these measurements, such as differences between the baseline and final value of a variable, or composite scores. A variable may be a simple Yes or No response to a question (a binary or dichotomous variable), an evaluation at several levels that may be ordered (from best to worst in steps – an ordered categorical variable), a category (such as race) that is not ordered, a rate or percent, a count, or a continuous measurement that can, theoretically, take on any value in a given range. One variable may be measured or calculated at several time points. Frequently the measurement at the earliest time in a study, usually immediately before the intervention begins, is called the “baseline” value, and values at later time points can be used either as the observed value or as the calculated change from baseline.
A randomized parallel group study of testosterone replacement in hypogonadal men compared a new delivery method, given at two dosages, to a currently approved method of delivery. The participants were treated for six months. Testosterone levels were measured several times during the study, and several summary measures, such as the maximum level and the area under the curve for a given time interval, were calculated. Other hormones, bone markers, body composition, and bone mineral density were measured at the beginning and end of the study and, for some variables, several times during the study. The testosterone measurement was used to create a new dichotomous variable – within or not within the normal range. The primary hypothesis of this study was that the new method would yield testosterone levels equivalent to those calculated for the currently approved method. A secondary hypothesis was that the new method would have fewer side effects than the current method. Mood scores and sexual activity scores were calculated from a weekly diary by averaging each item over the week and then computing a summary score from several items. Expected side effects, such as skin irritation, were measured and recorded at regular intervals if they occurred at or between scheduled examinations.
14.2 Role of Variables in an Interventional Study
A study protocol, along with the Manual of Procedures (MOP: Section 29.3), should specify what variables are to be collected, how they will be measured, what derived variables will be used, why they are considered important, and how these variables will be used in the study. The variables may be grouped into four classes: outcome variables, predictor variables, confounding variables, and safety variables, based on the planned usage in the analysis of the study. The role of these variables depend on the assumptions and objectives of the investigator and are not inherent to any particular variable or method of measurement.
Outcome variables are the basic results of the study. The outcome variables are frequently referred to as endpoints, and the statistical name for them is the dependent variables. Most studies have multiple outcome variables. Frequently one variable is described as the primary outcome variable, and the rest are called secondary outcomes.
The primary outcome variable for this study was the measured testosterone level, dichotomized to normal or not normal. There were also secondary outcome variables spanning many areas expected to be affected by testosterone replacement. The occurrence and severity of certain side effects were compared to test the assumption that the new method would be easier to use than the current method. The other secondary outcome variables, including bone markers, sexual function, mood changes, body strength, body composition, and bone mineral density, were analyzed as changes from the first day of the study.
We discuss outcome variables in more detail in Chapter 15.
Predictor variables are the variables that you think will affect the outcome variables. The statistical name for them is independent variables. In general, the main purpose of a study is to examine the relationship between the predictor variables and the outcome variables. In an interventional study with more than one group, the primary predictor variable is the intervention used, often a treatment for a medical problem. There is frequently a hierarchy of predictor variables similar to the outcome variables, with some being of primary interest. The study design often focuses on the effect of primary predictors on primary outcome variables, particularly when determining sample size. Analysis of the effects of secondary predictors is often seen as exploratory.
The primary predictor variable for both the primary outcome and all the secondary outcomes was the intervention that the participant received. The treatment was assigned randomly when participants entered the study (Chapters 20 and 21).
Investigators are interested in whether a program of diet and exercise, which has previously been shown to reduce cholesterol levels in participants, will reduce the incidence of cardiac events in a group of high-risk individuals. To do this they use a parallel group interventional study, with two groups: one receiving the diet and exercise program, and one following their routine diet and exercise. The incidence of cardiac events would be the primary endpoint, while other outcomes, such as all-cause mortality, would be secondary. The primary predictor is the randomized treatment group assignment.
Confounding variables, also referred to as concomitant variables, are variables that are not manipulated by the investigator and are not a focal point of the study, but may affect the relationship between predictors and outcomes. In other words, they are variables that have to be tested but that usually the investigator has not specified in his hypotheses. These are often demographic variables, such as age, sex, and ethnicity, but other variables may also be confounders in a study. For example, knowing someone is male may predict that he will be heavier that someone who is female.
The primary confounding variable in this study was the underlying cause of the hypogonadism. Since this was a multi-center study, the study site also was a possible confounder. Age was also tested as a confounder. Since this study only involved males, sex was not an issue.
In this study of diet and exercise, age and sex were considered as confounding variables. The inclusion criteria specified a limited age range and the ages were recorded in 10-year intervals. The interaction of age and sex was also tested for an effect on cardiac events.
Predictor and confounding variables are discussed further in Chapter 16.
Finally, safety variables are variables that are not related to the efficacy aims of the study, but are measured to make sure that the intervention is not doing any harm. They are needed only for interventional studies. They commonly include vital signs, lipids, liver enzymes, electrolytes, hematology, glucose and insulin, other measurements commonly included in a chemical panel, and significant adverse events, hospitalization, and death. Other safety variables can be required depending on what effects the treatment could possibly have. The investigators may have to track actual values, or simply categorize a condition as normal or abnormal. A description of adverse events should also be recorded as part of the safety information
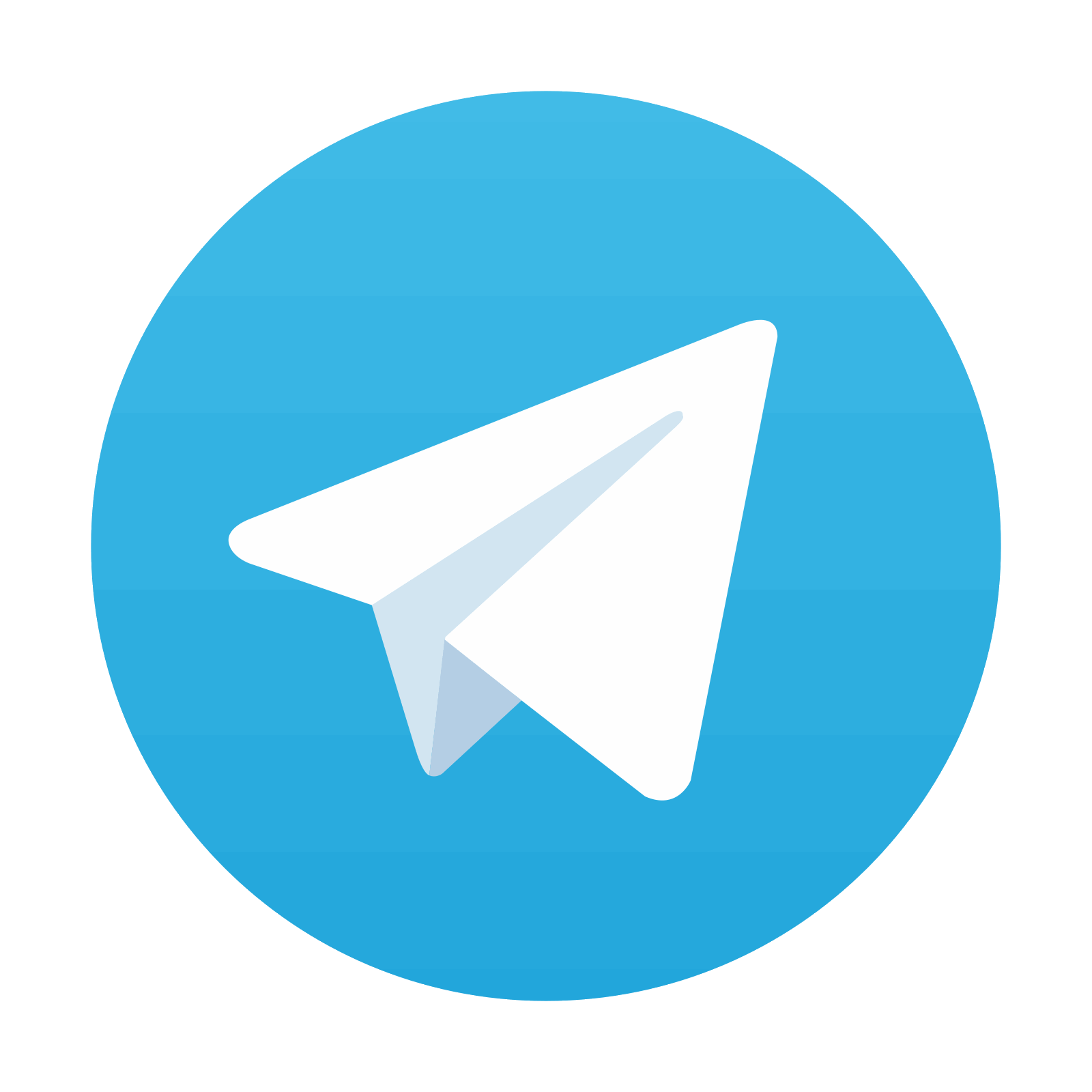
Stay updated, free articles. Join our Telegram channel

Full access? Get Clinical Tree
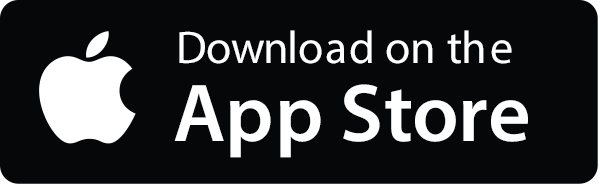
