KEY TERMS
The science of epidemiology rests on statistics. In fact, all public health, because it is concerned with populations, relies on statistics to provide and interpret data. The chapter on the role of data in public health discusses the kinds of data governments collect to assess the need for public health programs and evaluate public health progress. The term statistics refers to both the numbers that describe the health of populations and the science that helps to interpret those numbers.
The science of statistics is a set of concepts and methods used to analyze data in order to extract information. The public health sciences discussed in this book depend on the collection of data and the use of statistics to interpret the data. Statistics makes possible the translation of data into information about causes and effects, health risks, and disease cures.
Because health is determined by many factors—genes, behavior, exposure to infectious organisms or environmental chemicals—that interact in complex ways in each individual, it is often not obvious when or whether specific factors are causing specific health effects. There are ethical and logistical limits to the kinds of studies that can be conducted on human populations and there are limits to the conclusions that can be drawn from biomedical studies on animals. Only by systematically applying statistical concepts and methods can scientists sometimes tease out the one influence among many that may be causing a change in some people’s health. Often, however, statistics indicates that an apparent health effect may be simply a random occurrence.
The problems and limits of epidemiology are defined in large part by the uncertainties that are the subject of the science of statistics. This chapter discusses the science of statistics in more detail, describing how it is used to clarify conclusions from a study or a test, to put numbers into perspective so that researchers can make comparisons and discern trends, and to show the limits of human knowledge.
The Uncertainty of Science
People expect science to provide answers to the health questions that concern them. In many cases, science has satisfied these expectations. But the answers are not as definitive as people want them to be. Science has shown that the human immunodeficiency virus (HIV) causes AIDS. But that does not mean that a woman will definitely contract AIDS from having sex with an HIV-positive man. Her chance of becoming infected with the virus from one act of unprotected intercourse is about one in 1000.1 Similarly, scientific studies show that as a treatment for early breast cancer, a lumpectomy followed by radiation is as effective as a mastectomy. However, a woman who chooses the lumpectomy still has a 10 percent chance of cancer recurrence.2 Both the woman who had unprotected intercourse and the woman who chose the lumpectomy would dearly like to believe that they will be one of those in the majority of cases who will have a positive outcome, but science cannot promise them that. It can only say, statistically, that if 1000 women like her have unprotected sex with an HIV-positive man, 999 probably will fare well while one will not, and if 100 women with early breast cancer have a lumpectomy with radiation, 90 probably will be cancer-free after 12 years while 10 will have a recurrence.
In many cases, there are not enough data even to give us that degree of certainty, or the data that exist are too ambiguous to allow a valid conclusion. In 1995, the New England Journal of Medicine published a report that the Nurses’ Health Study (a cohort study), which had monitored 122,000 nurses for 14 years, found a 30 to 70 percent increased risk of breast cancer in women who had taken hormone replacement therapy after menopause.3 One month later, the Journal of the American Medical Association published the results of a case-control study that found no increased risk from the hormones. Some 500 women who had newly diagnosed breast cancer were no more likely to have taken postmenopausal hormones than a control group of 500 healthy women.4 In The New York Times article reporting on the studies, each researcher is quoted suggesting possible flaws in the other study.5 There was little comfort in these results for women seeking certainty on whether the therapy would improve their health. According to one view, postmenopausal estrogen was clearly worth the possible risk of cancer because it appeared to decrease a woman’s risk of heart disease and osteoporosis. In the opposing argument, women could achieve similar benefits without the possible risk through exercise, avoiding smoking, eating a low-fat diet, maintaining a normal weight, and taking aspirin. Now a clinical trial has contradicted some of the findings of each of these studies; hormone replacement therapy has been found to increase cancer risk and not to benefit the heart.
Contradictory results from epidemiologic studies are common. There are many possible sources of error in this kind of research, including bias and confounding, which are factors irrelevant to the hypothesis being tested that may affect a result or conclusion. Later in this chapter, additional factors to be considered in assessing whether to believe a study’s conclusions are examined.
People sometimes demand certainty even when science cannot provide it, as occurred in 1997 over the issue of whether women ages 40 through 49 should be screened for breast cancer using mammography. Studies had shown that routinely testing women aged 50 and over with the breast x-rays could reduce breast cancer mortality in the population. However, studies done on younger women had not demonstrated a life-saving benefit overall for this group. Routine screening of these women increases their radiation exposure, perhaps raising their risk of cancer. It also yields many false alarms, leading to unnecessary medical testing, and major expense. The follow-up testing itself may cause complications, and many of the women remain anxious even after cancer is ruled out.6
When Dr. Richard Klausner, the director of the National Cancer Institute (NCI), called together a panel of experts in early 1997 to advise him on the issue, the panel concluded that, for younger women, the benefit did not justify the risks and costs, and recommended that each woman make the decision in consultation with her doctor, considering her own particular medical and family history. The public and political response was heated: After a barrage of media publicity, the Senate voted 98 to 0 to endorse a nonbinding resolution that the NCI should recommend mammography for women in their 40s. A letter signed by 39 congresswomen stated that, “without definitive guidelines, the lives of too many women are at risk to permit further delay,” assuming that screening could save lives despite the lack of evidence.7(p.1104) In the end, director Klausner, with the support of President Clinton and Secretary of Health and Human Services Donna Shalala, recommended that women in their forties should be screened. It seems clear that pressure from politicians eager to get credit for supporting women’s health led to a pretense of scientific certainty where none existed.
On this question, further analysis supported the politicians, although the benefit is weaker for the younger age group. While the “melee that followed the meeting will not qualify for a place in the history of public health’s most distinguishing scientific or policy moments,” in the words of one analyst, there is now a far better understanding of the issue and evidence that screening may be life-saving for some younger women.8(p.331) However, because the incidence of breast cancer is lower in women in their 40s, and the effectiveness of mammography is also lower in the denser breasts of the younger women, the benefit of screening is less for them. In a review of the evidence published in 2007, the conclusion seems to echo the NCI’s original recommendation that individual women, in consultation with their doctor, should decide whether to be screened. The authors suggest that, “a woman 40 to 49 years old who had a lower-than-average risk for breast cancer and higher-than-average concerns about false-positive results might reasonably delay screening. Measuring risks and benefits accurately enough to identify these women remains a challenge.”9(p.522)
Remarkably, the whole political uproar was repeated in 2009, when an independent panel of experts, appointed by the Department of Health and Human Services, issued a recommendation that routine breast cancer screening begin at age 50, not 40. Because the recommendation was published in the midst of the public debate over health care reform, conservative politicians cried “rationing.” As science reporter Gina Kolata pointed out in a New York Times article, the dispute gives many people “a sense of déjà vu.”10 The data hadn’t changed much since the earlier debate, except that new evidence was published in 2008 suggesting that some invasive breast cancers may spontaneously regress, supporting the argument that screening may lead to unnecessary treatment.
Many people concerned about how to protect their health find it frustrating when today’s news seems to contradict yesterday’s. As this example shows, science is a work in progress. In the words of Dr. Arnold Relman, former editor of the New England Journal of Medicine, “Most scientific information is of a probable nature, and we are only talking about probabilities, not certainty. What we are concluding is the best opinion at the moment, and things may be updated in the future.”11(p.11)
Probability
Scientists quantify uncertainty by measuring probabilities. Since all events, including all experimental results, can be influenced by chance, probabilities are used to describe the variety and frequency of past outcomes under similar conditions as a way of predicting what should happen in the future. Aristotle said that, “the probable is what usually happens.” Statisticians know that the improbable happens more often than most people think.11(p.19)
One concept scientists use to express the degree of probability or improbability of a certain result in an experiment is the p value. The p value expresses the probability that the observed result could have occurred by chance alone. A p value of 0.05 means that if an experiment were repeated 100 times, the same answer would result 95 of those times, while 5 times would yield a different answer. If a person tosses a coin 5 times in a row, it is improbable that it will come up the same—heads or tails—every time. However, if each student in a class of 16 conducts the experiment, it is probable that 1 student will get the identical result in all 5 tosses. The probability of that occurrence is 1 chance in 16, or 0.0625 (p = 0.0625). Thus a p value of 0.05 says that the probability that an experimental result occurred by chance alone is less than the probability of tossing 5 heads or 5 tails in a row. A p value of 0.05 or less has been arbitrarily taken as the criterion for a result to be considered statistically significant.
Another way to express the degree of certainty of an experimental result is by calculating a confidence interval. This is a range of values within which the true result probably falls. The narrower the confidence interval, the lower the likelihood of random error. Confidence intervals are often expressed as margins of error, as in political polling, when a politician’s support might be estimated at 50 percent plus or minus 3 percent. The confidence interval would be 47 percent to 53 percent.11
While p values and confidence intervals are useful concepts in deciding how seriously to take an experimental result, it is wrong to place too much confidence in an experiment just because it yields a low p value or a narrow confidence interval. There may be up to 10,000 clinical trials of cancer treatment under way at any time. If a p value of 0.05 is taken to imply statistical significance, 5 out of every 100 ineffective treatments would appear to be beneficial, errors caused purely by chance.11 Thus, large numbers of cancer treatments could be in clinical use that are actually not effective. Other reasons that a low-p-value study could lead to an erroneous conclusion could be bias or confounding, which are systematic errors. The results of the study that linked coffee drinking with pancreatic cancer were statistically significant with a p value of 0.001.12 The conclusion is thought to be wrong not because of random error but because the cancer was caused by smoking rather than coffee drinking.13
The fact that the probable is not always what happens leads to the Law of Small Probabilities.11 The most improbable things are bound to happen occasionally, like throwing heads 5 times in a row, or even—very rarely—99 times. This means, for example, that a few people with apparently fatal illnesses will inexplicably recover. They may be convinced that their recovery was caused by something they did, giving rise—if their story is publicized—to a new vogue in quack therapies. But because their recovery was merely a random deviation from the probable, other patients will not get the same benefit.
Another consequence of the Law of Small Probabilities is the phenomenon of cancer clusters. Every now and then a community will discover that it is the site of an unusual concentration of some kind of cancer, such as childhood leukemia, and everyone will be highly alarmed. Is there a carcinogen in the air or the drinking water that is causing the problem? Could the cause be electromagnetic fields, which residents blamed for the cluster of six cases of childhood cancer between 1981 and 1988 among the pupils of an elementary school in Montecito, California?14 Under great political pressure, the local and state government will investigate, but no acceptable explanation will be found. In the case of the electromagnetic fields, it could not be proven that they were not responsible for the cluster, but as more studies are done the evidence is still ambiguous. Most such clusters are due to statistical variation, like an unusual run of tails in a coin toss. Such an explanation tends to be unsatisfactory to community residents, who may accuse the government of a cover-up; but after the investigation the number of new cases usually returns to more or less normal levels, and the sense of alarm subsides.
If a cluster is very large, it is likely not to be a random variation—just as in coin tossing, 50 heads in a row is a much less likely outcome than five heads unless there is something wrong with the coin. A large number of cases is said to confer power on a study. Power is the probability of finding an effect if there is, in fact, an effect. Thus, an epidemiologic study that includes large numbers of subjects is more powerful than a small study, and the results are more likely to be valid, although systematic errors due to bias or confounding can be present in even the largest studies.
In designing studies of any kind, statisticians can calculate the size of the study population necessary to find an effect of a certain size if it exists. Studies with low power are likely to produce false-negative results (i.e., to find no effect when there actually is one). False-positive results occur when the study finds an effect that is not real (e.g., when a random variation appears to be a true effect). In a study of epidemiologic studies, a statistician examined the power of each of 71 clinical trials that reported no effect. He concluded that 70 percent of the studies did not have enough patients to detect a 25 percent difference in outcome between the experimental group and the control group. Even a 50 percent difference in outcome would have been undetectable in half of the studies.11 This common weakness in epidemiologic studies is probably one reason for the contradictory results so often reported in the news.
In the review of high-dose chemotherapy and bone marrow transplant for advanced breast cancer, the authors addressed the question of whether the studies had enough power to detect a significant improvement in survival for the treated women. They concluded that at least one of the individual studies did have sufficient power, and that the systematic review of all studies combined had the power to detect a 10 percent difference after five years.15 Although some subgroups of women appeared to have benefited slightly from the high dose treatment, further studies would be necessary to demonstrate this, and no such studies are planned. The question remains of how much difference would be clinically relevant. Would it be acceptable for a woman to undergo the arduous treatment if her chance of survival was only 10 percent better? That is a question that cannot be answered by statisticians.
The Statistics of Screening Tests
In public health’s mission to prevent disease and disability, secondary prevention—early detection and treatment—plays an important role. When the causes of a disease are not well understood, as in breast cancer, little is known about primary prevention. The best public health measure is to screen the population at risk so as to detect the disease early, when it is most treatable. Screening is also an important component of programs to control HIV/AIDS by identifying HIV-infected individuals so that they can be treated and counseled about how to avoid spreading the virus to others. As discussed later in this volume in the section of genetic diseases, newborn babies are routinely screened for certain congenital diseases that can be treated before permanent damage is done to the infants’ developing brains and bodies.
While laboratory tests to be used in screening programs should ideally be highly accurate, most are likely to yield either false positives or false negatives. Tests may be highly sensitive, meaning that they yield few false negatives, or they may be highly specific, meaning that they yield few false positives. Many highly sensitive tests are not very specific and vice versa. For most public health screening programs, sensitive tests are desirable in order to avoid missing any individual with a serious disease who could be helped by some intervention. However, inexpensive, sensitive tests chosen to encourage testing of as many at-risk individuals as possible are often not very specific. When a positive result is found, more specific tests are then conducted to determine if the first finding was accurate. For example, if a sensitive mammogram finds a suspicious spot in a woman’s breast, the test is usually followed up with a biopsy to determine whether the spot is indeed cancerous.
When screening is done for rare conditions, the rate of false positives may be as high as or higher than the number of true positives, leading to a lot of follow-up testing on perfectly normal people. Such a situation occurred in 1987 when the states of Illinois and Louisiana mandated premarital screening for HIV.16 With the rate of HIV infection in the general, heterosexual population quite low, a great many healthy people were unnecessarily alarmed and subjected to further tests, while very few HIV-positive people were identified. Some couples went to neighboring states to marry to avoid the nuisance. The programs were discontinued within a year. The problem of false positives is also the reason why mammography screening is questionable for women in their 40s, as discussed earlier.
There are other conditions for which screening may not be as beneficial as expected. One of these is prostate cancer, discussed elsewhere in this text. Another is lung cancer screening of smokers. Lung cancer is usually a fatal diagnosis; by the time most patients suffer symptoms, it is too late for medicine or surgery to make a difference. The idea of screening smokers so that cancers can be detected and treated earlier in the course of the disease has been around since the 1970s and 1980s. However, at that time, the only method of screening was to use chest x-rays, and it turned out that cancers detected by x-ray screening were almost always too far advanced to be treatable.
In fall 2006, a paper published in the New England Journal of Medicine reported that screening with spiral CT scans (a kind of three-dimensional x-ray) could detect lung cancers early enough that treatment allowed 80 percent of patients to survive for ten years, compared to a 10 percent survival rate for patients who had been diagnosed the usual way.17 A few months later, the Journal of the American Medical Association published another study, concluding that spiral CT scanning does not save lives and may actually cause more harm than good.18 An analysis of the findings of the first trial revealed two sources of bias: lead-time bias and overdiagnosis bias.19 The former may occur in all cancer screening and must be taken into consideration before concluding that screening saves lives. Lead-time bias occurs when increased survival time after diagnosis is counted as an indicator of success. If early detection of a cancer does not lead to a cure, the only result of early diagnosis is that patients will live longer with the knowledge that they are sick before dying at the same time they would have died anyway. This appears to be the case in the New England Journal of Medicine study of lung cancer screening. In fact, the effects of the additional diagnostic tests and surgeries that follow the early diagnosis may hasten the patients’ death.
Overdiagnosis bias occurs when the tumors that are detected by the screening are not likely to progress to the stage that they cause symptoms and be life-threatening. Such small tumors had also been found in the earlier lung cancer screening trials using x-rays. Overdiagnosis bias is also a problem with prostate cancer screening, and perhaps with breast cancer screening, as discussed earlier in this chapter. The only way to be sure that screening actually saves lives is to conduct randomized controlled trials, comparing mortality among patients who are screened with that of patients who are not screened. Such trials, together with data showing that breast cancer mortality overall has fallen in the United States by 24 percent since 1990, have shown that mammography does save lives.8,9,20
Rates and Other Calculated Statistics
Epidemiology makes extensive use of rates in studies of disease distribution and determinants. Rates put the raw numbers into perspective by relating them to the size of the population being considered. Vast quantities of health-related data are collected on the American population, data that are used to assess the people’s health and to evaluate the effectiveness of public health programs. For these purposes too, the raw numbers are subjected to statistical adjustments that yield various rates useful in making comparisons and identifying trends.
For example, knowing that a city has 500 deaths per year is not very informative unless the population of the city is known. Death rates are generally expressed as the number of deaths per 1000 people. Thus, 500 deaths per year is a low number for a city of 100,000, while it is high for a city of 50,000. The overall death rate in the United States was 8.2 per 1000 people in 2013.21 The same data may yield different rates depending on the population referred to. Rates are usually calculated using the population at risk for the denominator. In the case of death rates, the whole population is at risk. Birth rates are an exception; like the death rate, the birth rate is defined as the number of live births per 1000 people. The fertility rate, by contrast, does use the population at risk, giving the number of live births per 1000 women ages 15 to 44. Two communities with the same fertility rate may have quite different birth rates if one contains many young women and the other is older with a higher proportion of men. Both rates start with the same raw number—the number of live births—but use a different population for reference. In 2013, the birth of 3,932,181 babies in the United States led to a birth rate of 12.4 per 1000 people overall. The fertility rate ranged from 58.7 per 1000 non-Hispanic white women to 72.9 per 1000 Hispanic women.22

Full access? Get Clinical Tree
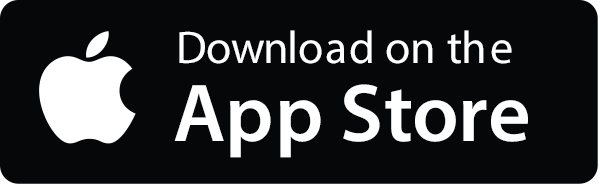
