15.1 Two types of interaction
Types of interaction
Epidemiologists speak of two distinct types of interaction: biological interaction and statistical interaction. Although these concepts share the term “interaction,” these are separate phenomena that should not be confused. Biological interaction (biological interdependence, causal interaction, synergism) is the interdependent operation of two or more causes to produce or prevent an effect. Statistical interaction (effect measure modification, measure of association heterogeneity) refers to a statistical model that does not adequately predict the joint effects of two or more exposures. Whereas a biological interaction describes a property of causality, statistical interaction does not have a universal causal interpretation.
Biological interaction
Because most diseases are caused by multiple causal factors acting together, every cause must be viewed in relation to other causal components. Biological interaction is almost always present. Take as an example exposure to the polio virus. The effect of exposure to the polio virus depends not only on the virus, but also on the immune status of the individual. In an immune individual, the virus will have no effect. In a susceptible individual, the virus is causal. The interdependence between the effect of the viral agent and the host factor, which we call immunity, is an example of a biological interaction.
As a second example, consider smoking and lung cancer. Smoking causes lung cancer in only a fraction of exposed individuals. This is because genetic factors and perhaps contributing environmental factors are needed to complete a sufficient causal mechanism for lung cancer. Therefore, we say there is a biological interaction between smoking and complementary contributors to lung cancer. (See Section 2.3 for additional discussion about causal interactions).
Knowledge of biological interactions have direct health relevance. When two factors interact biologically, their combined presence has a more potent effect than either of them singly. Consider as an example the biologic interaction between oral contraceptives and smoking. Both cause cardiovascular disease in women. However, their combined use is a much more potent cause than either alone. Oral contraceptive use is therefore discouraged in smokers because of its causal consequences.
Understanding biological interdependences is useful in helping to focus prevention efforts in susceptible populations. For example, the biological dependency between Down syndrome and pregnancies after the age of 40 allows “older pregnancies” to be targeted for screening; the dependency between influenza and mortality in older people and in people with pre-existing cardiorespiratory disease allows vaccination programs to be directed toward these groups; the dependency between driving and alcohol consumption motivates programs against drunk driving.
Statistical interaction
Distinct from biological interaction, statistical interaction (effect measure heterogeneity, measure of association heterogeneity) occurs when a statistical description does not adequately describe the joint effects of two or more exposures. In contrast to biological interaction, statistical interactions are measure of association specific. For instance, a given risk factor may cause statistical interactions with risk differences but not with risk ratios. The data in Table 15.1 will help illuminate this point. This table lists risks of arterial thromboembolic diseases (myocardial infarction, ischemic stroke) in oral contraceptive user and nonusers according to smoking status. The risk ratio in smokers is 2 (12 ÷ 6); the risk ratio in nonsmokers is also 2 (4 ÷ 2). Therefore, the risk ratios are homogenous across smoking categories. When a measure of association is homogeneous according to levels of another factor, we say that statistical interaction is absent. When statistical interaction is absent in the risk ratios, risks plotted on logarithmic graph paper will form parallel lines (Figure 15.1).
Table 15.1 One-year risk (per 10 000) of thromboembolic diseases according to oral contraceptive use and cigarette smoking, hypothetical values.
Oral contraceptive users | No oral contraceptive use | |
Smokers | 12 | 6 |
Nonsmokers | 4 | 2 |
Figure 15.1 One-year risk of thromboembolic disease in oral contraceptive nonusers and users according to smoking status plotted on semi-log paper. Parallel lines indicate homogeneity of the risk ratio.

Let us now use the data in Table 15.1 to consider whether smoking causes interaction in the risk differences. Among smokers, the risk difference (per 10 000) is 12 − 6 = 6. Among nonsmokers, the risk difference (per 10 000) is 4 − 2 = 2. Thus, the risk differences are heterogeneous across smoking categories and statistical interaction is present. When statistical interaction is present in risk differences, risks plotted on arithmetic graph paper will not be parallel (Figure 15.2).
Figure 15.2 One-year risk of thromboembolic disease in oral contraceptive nonusers and users according to smoking status plotted on nonlogarithmic paper. Nonparallel lines indicate heterogeneity of the risk difference.

This illustrates that statistical interaction depends on the measure of association used to describe the relation being studied. Whereas risk ratios in the above example demonstrated no statistical interaction, risk differences demonstrated statistical interaction. Statements about statistical interaction are therefore measure of association specific.
15.2 Chi-square test for statistical
When statistical interaction is present, we expect measures of association to differ in population strata. Since measures of association in epidemiology are also called measures of effect, statistical interaction is also called effect measure heterogeneity. Such heterogeneity is often evident upon inspection. However, even in the absence of statistical interaction, effect measures in different strata will vary randomly. To help distinguish random and systematic heterogeneity, the epidemiologist may perform a statistical test for interaction.
Let MA represent the measure of association under consideration. This may be a risk ratio, risk difference, rate ratio, odds ratio, or whatever. We test the following statistical hypotheses:
H0: MA1 = MA2 = … = MAK (“homogeneity”)
H1: at least one strata-specific measure of association differs (“heterogeneity”) where MAk represents measure of association parameter in stratum k { k: 1, 2, …, K.} For example, in testing odds ratios from two strata, the null and alternative hypotheses are H0: ψ1 = ψ2 vs. H1: ψ1 ≠ ψ2.
An ad hoc variance-based chi-square interaction statistic takes the following general form:
15.1
where
= measure of association estimate in stratum k
SE = standard error of the measure of effect in stratum k
= adjusted or summary measures of association
(e.g., as a Mantel–Haenszel estimate)
Under the null hypothesis, the statistic has a chi-square distribution with K −1 degrees of freedom. Two cautions must be made in reference to this test.




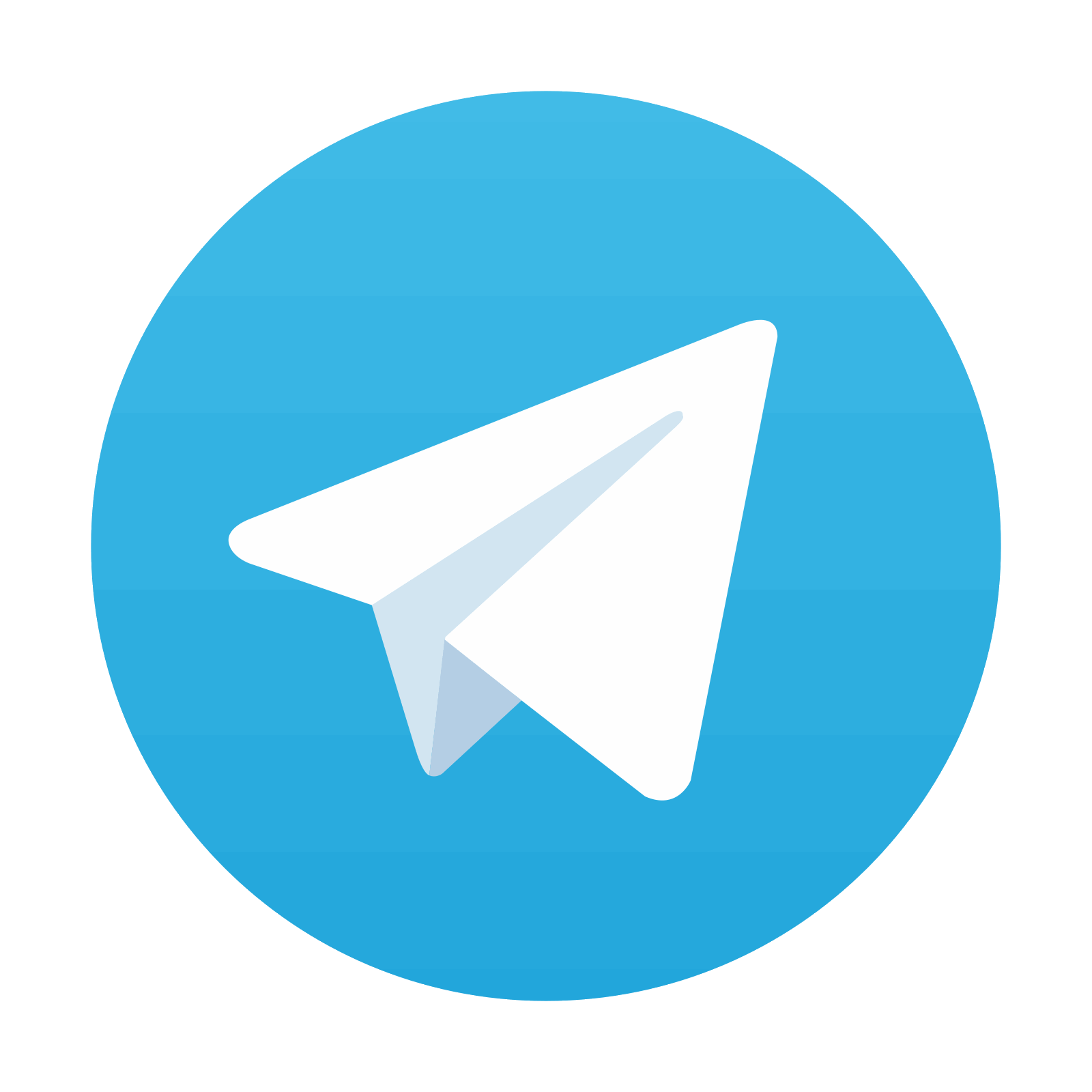
Stay updated, free articles. Join our Telegram channel

Full access? Get Clinical Tree
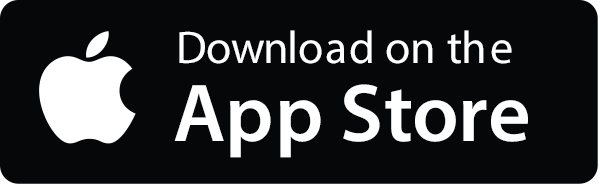
