
Fig. 7.2
Highly overlapped samples could not be classified completely by linear and nonlinear discriminant function [7]
In the process of the KY methods, two different types of discriminant functions were created to determine positive, negative, and gray zones (Fig. 7.3). One of the discriminant functions is called as all-negative (AN) model and the other as all-positive (AP) model. The AN model classified AN samples in the sample set correctly and the AP model classified all-positive samples correctly. The samples which were classified as negative samples by AN model and positive samples by AP model belonged to the gray zone (Fig. 7.3).


Fig. 7.3
Classification results by AP and AN sample discriminant functions. Samples in positive zone and negative zone had high reliability of classification. Gray zone was not classified [7]
The KY methods focused on both sides of a sample space and found that there were special spaces, which included only correctly classified samples. These two areas have been defined as positive zone and the others as negative zone. The third zone was named as gray zone. All samples included in the positive zone belonged to a positive class, while all samples included in the negative zone belonged to a negative class. On the other hand, the samples included in the gray zone could not be determined whether they belonged to a positive or negative class since they were highly overlapped (Fig. 7.3).
If the gray zone (1) was determined by AN1 and AP1 discriminant functions, the gray zone (1) could be extracted and reclassified by AN2 and AP2 models to build a new sample set. If a new gray zone (2) was determined with respect to the new sample set, a further new sample set can be built as shown in Fig. 7.4. Repeating these steps, all samples in the original sample set can be classified correctly (Fig. 7.5). This is the basic concept of KY methods. The AN model and the AP model can be generated based on any conventional linear and nonlinear discriminant function. Therefore, KY methods can be categorized as a meta-algorithm approach.



Fig. 7.4
Improvement of classification rate by KY methods. Correctly classified positive and negative samples are removed and the gray zone samples were reconstructed and reclassified in the new sample space by new discriminant functions at the next step [7]

Fig. 7.5
Meta-algorithm repetition of reclassification of gray zone (KY methods). High reliability zone (correctly classified samples) was removed and gray zone was reclassified, and the sample space was reconstructed by new discriminant functions at the next step. All samples were correctly classified at the final step [7]
All data analyses were performed using ADMEWORKS/Model Builder software (Fujitsu Kyushu Systems Limited, Japan).
7.2.4 1 Model KY Methods
1 model KY methods are simple, easy, and delicately manipulated compared with ordinary 2 model KY methods (Fig. 7.6).


Fig. 7.6
1 model KY methods (overview)
7.3 Results
In this study, AN and AP discriminant functions at step 1 were generated by AdaBoost. The final discriminant function was generated by TILSQ. All 288 compounds were perfectly classified by two steps.
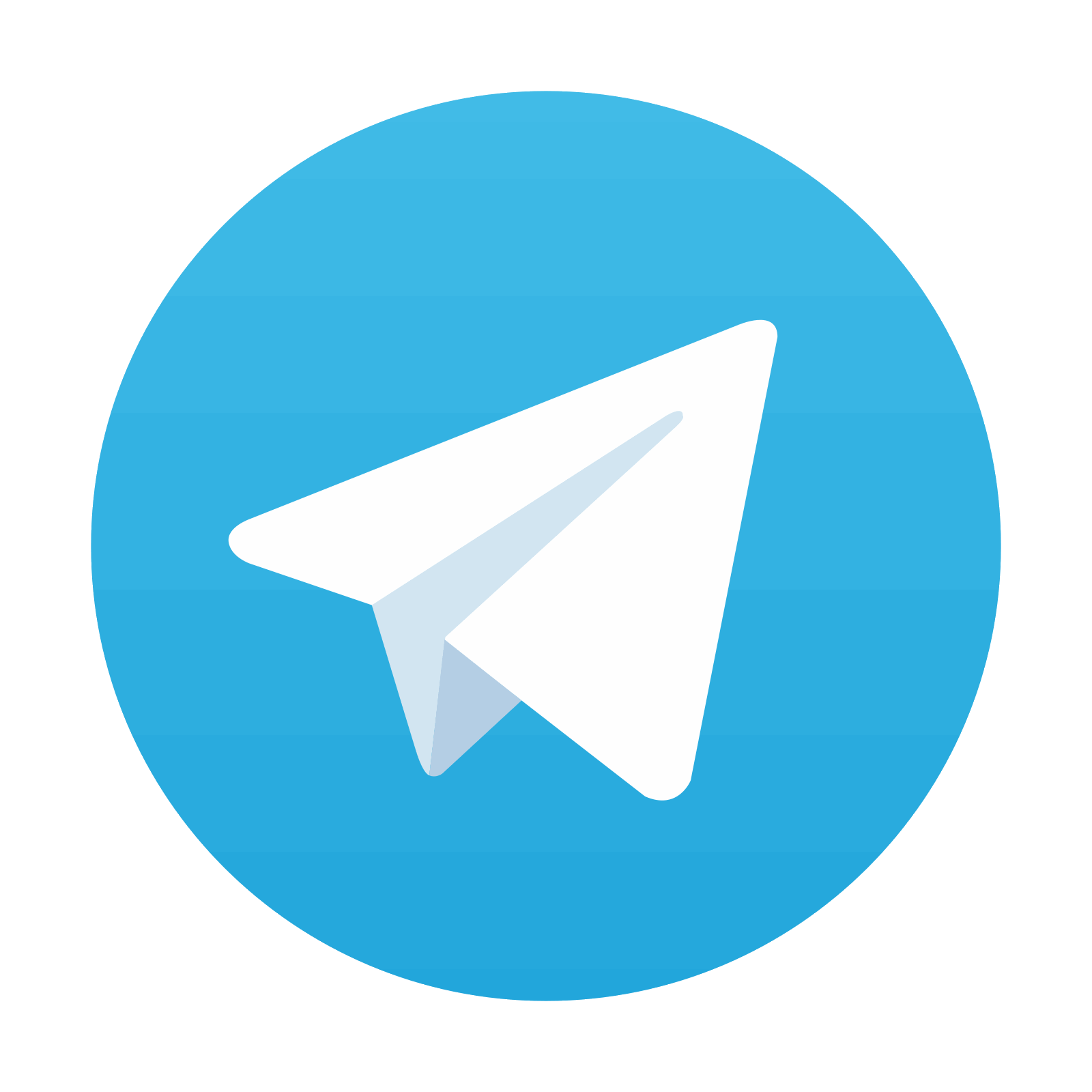
Stay updated, free articles. Join our Telegram channel

Full access? Get Clinical Tree
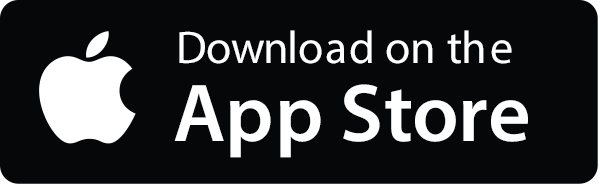
