Fig. 7.1
Sample stimuli. a Sample of original images derived from the Ekman and Friesen collections of neutral face and the facial emotions of anger, disgust, fear, happiness, sadness, and surprise. The images were cropped with the same outline (6° × 8°). Numbers were designated 0 % as the neutral face and 100 % as the intensity of the original image of the facial emotion. b Transitional images from the neutral face to the emotion disgusted are indicated as the 10 % increment values
Besides the recording of subjective rating from the subjects of experiment, the brain signals of the subjects were also recorded during the whole experiments. These two different data, subjective rating and brain signals, will be inter-correlated in order to elucidate the temporal effect of facial expressions in humans’ brain. Besides that, the task of rating the presented facial expression subjectively is one way to keep the subject stay awake and alert during the experiment.
We presented facial expressions as still photo images at the center of a CRT 21′′ (1,280 × 1,024, 100 Hz) where monitor placed approximately 70 cm from the subjects, with a visual horizontal angle 4° and a vertical angle of 6°. To fixate the subjects’ gaze, a white dot was presented at the center of the display during the transition between image presentations or between trials. The subject was told to stare at the fixation point, to carefully watch the stimulus and to do the press-button tasks as quickly as possible after the instruction was shown. Detail of the experimental design is shown in Fig. 7.2.
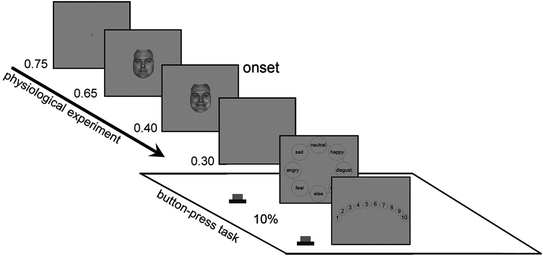
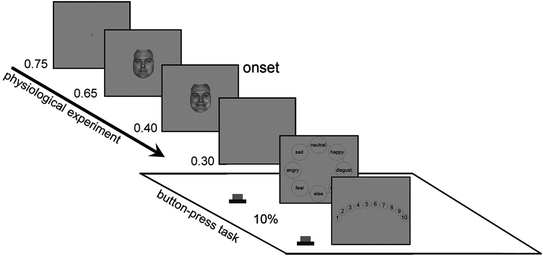
Fig. 7.2
Experimental design of psychological experiment. A trial was started by the presentation blank gray screen with white dot in the center of the screen as fixation point. A neutral face was then presented followed by the presentation of a randomly selected transitional image of one of the emotional facial expressions from the same actor. The subject was required to identify the type of emotion and to assess the intensity of each image. Presentation time [s] is shown at the left sides of each panel
7.4 Brain Signal Recordings
Despites many medical imaging techniques can be used for recording the brain activities, electroencephalography is still the least dangerous, the less expensive, and yet the most temporally accurate for elucidating the brain activities based on its signals. Electroencephalography is a science of recording and analyzing the electrical activity of the brain. The spontaneous brain’s electrical activities are recorded in the time domain from several electrodes placed on the scalp. The electrodes are then linked to an electroencephalograph, which is an amplifier connected to a mechanism that converts electrical impulses into digital data and displayed on a computer screen. The digital data or the print out of spontaneous brain’s activity is called an electroencephalogram (EEG) (see Fig. 7.3).
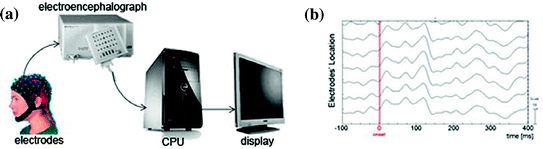
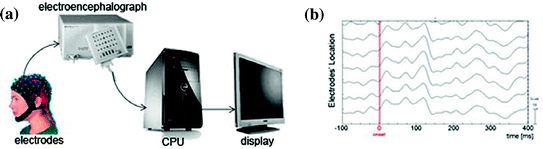
Fig. 7.3
a EEG system. b Samples of raw EEG
7.4.1 Brief History of Electroencephalography
Experiment by applying electricity to dead frogs’ nerve trunks which induced the movement of their legs was the first demonstration to prove that information in the nervous system may be electrically transmitted. This experiment was done by Italian physiologist Luigi Galvani during the eighteenth century. In 1870, two Prussian (current: German) physicians, Gustav Theodor Fritsch and Eduard Hitzig confirmed Galvani’s work by electrically stimulating areas of motor cortex of the dog’s brain which caused involuntary muscular contractions of specific parts of its body. It was not until 1875, however, that the Liverpool physician, Dr. Richard Caton, became the first person to record electrical activity in the brain by placing electrodes directly on brains of vivisected rabbits and monkeys. Using a primitive measuring device known as a mirror galvanometer, in which a moving mirror was used to amplify very small voltages, he reported finding feeble currents in the cerebral cortex, the outermost layer of the brain (Finger 1994).
Electrophysiological recordings became much more fashionable after Hans Berger (1873–1941) published his human EEG in 1929. The first human EEG was recorded using electrodes (made of lead, zinc, platinum, etc.) attached to the intact skull and connected to an oscillograph. Berger made 73 EEG recordings from his fifteen-year-old son, Klaus. The first frequency he encountered was the 10-Hz range (8–12 Hz), which at first was called the Berger rhythm, currently called alpha rhythm brain wave. He reported that the brain generates electrical impulses or ‘brain waves.’ The brain waves changed dramatically if the subject simply shifts from sitting quietly with eyes closed (short or alpha waves) to sitting quietly with eyes opened (long or beta waves). Furthermore, brain waves also changed when the subject sat quietly with eyes closed, ‘focusing’ on solving a math problem (beta waves). That is, the electrical brain wave pattern shifts with attention (O’Leary 1970). The publication of Hans Berger’s in 1929 changed neurophysiology forever, and because of it, he earned the recognition of ‘Father of Electroencephalography.’
7.4.2 The Electroencephalograph
The system for recording the spontaneous brain’s activity is electroencephalograph. It is safe, and very few risks are associated with it. Some locations on the scalp will be cleaned up by removing the dead cells and oils before attaching the electrodes. The placement of electrodes depends on the purpose of the study. In this writing, we would like to discuss based on the American 10–10 system of electrode nomenclature and placement for electrodes positioning. It contains 73 recording electrodes plus one electrode for ground and another for system reference at nose tip. All of these electrodes’ positioning is embedded in a cap. Besides those electrodes, we attached two different pairs of electrodes to record horizontal and vertical movement of the eyes. We placed these electrodes on the outer canthi of the two eyes for detecting the horizontal eye movements and on the infra- and supra-orbital ridges of the left eye for the vertical eye movements (see Fig. 7.4).
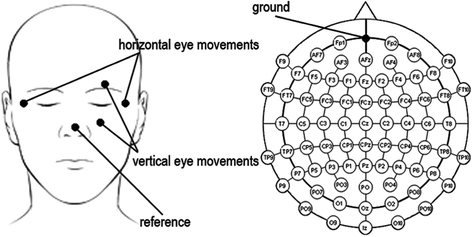
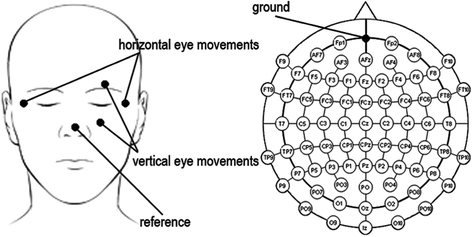
Fig. 7.4
Electrodes nomenclature and placement
For this writing, we used amplifiers of SynAmps system (Neuroscan) with amplification of 25,000 times of the voltage between the active electrode and the reference. The amplified signal is digitized via an analog-to-digital converter, after being passed through an anti-aliasing filter. Electroencephalograms (EEGs) and electrooculograms (EOGs) for the eyes were recorded continuously with a band pass filter of 0.1–100 Hz and digitization rate of 1,000 Hz.
7.4.3 The EEG Artifacts
Although EEG is designed to record cerebral activity, it also records electrical activities arising from sites other than the brain. The recorded activity that is not of cerebral origin is termed artifact and can be divided into physiological and non-physiological artifacts. Physiological artifacts are generated from the subject him/herself and include cardiac, glossokinetic, muscle, eye movement, respiratory, and pulse artifact among many others. The EEG recording can be contaminated by numerous non-physiological artifacts generated from the immediate patient surroundings. Common non-physiological artifacts include those generated by monitoring devices, infusion pumps, electric power system, and electrode pops; spikes originating from a momentary change in the impedance of a given electrode may also contaminate the EEG record.
Severe contamination of EEG activity by the artifacts is a serious problem for EEG interpretation and analysis. The easiest way to remove the artifacts is simply rejecting contaminated EEG epochs, but it causes a considerable loss of collected information. In this study, we apply independent component analysis (ICA) to multi-channel EEG recordings and remove a wide variety of artifacts from EEG records by eliminating the contributions of artifactual sources onto the scalp sensors (Jung et al. 2000a, b). ICA-based artifact correction can separate and remove a wide variety of artifacts from EEG data by linear decomposition. The ICA method is based on the assumption that firstly, the time series recorded on the scalp are spatially stable mixtures of the activities of temporally independent cerebral and artifactual sources, secondly the summation of potentials arising from different parts of the brain, scalp, and body is linear at the electrodes, and thirdly, the propagation delays from the sources to the electrodes are negligible. The second and third assumptions are quite reasonable for EEG data. Given enough input data, the first assumption is reasonable as well. The method uses spatial filters derived by the ICA algorithm and does not require a reference channel for each artifact source. Once the independent time courses of different brain and artifact sources are extracted from the data, artifact-corrected EEG signals can be derived by eliminating the contributions of the artifactual sources.
7.4.4 Reducing the Non-physiological Artifacts
Reducing the artifacts in EEG record is an important process to be done. Before the experiment, it is highly recommended to clean up the experimental room from any electrical devices that are not related with the experiment, and it is better to do the experiment in an electronically shielded room. For the artifact from electric power supply or display monitor, applying specific digital notch filter before or after EEG recording might be the easiest and the best way to reduce its effect. For the electrode-pop and electrostatic artifact, treatment after EEG recording is the only way to reduce its effect in EEG record in this study. These kinds of artifacts are originating in electrodes; the electrode-pop artifact is caused by a drying electrode or slight mechanical instability that changes the area of electrode surface in contact with the skin, and the electrostatic artifact is caused by the movement of electrode wires between the electrode on the head and the electrode board or other objects moving in relation to the input electrode leads. We cannot prevent these kinds of artifacts to be happens, but we might be able to reduce its effect in our EEG by always using clean electrodes and ask subjects to sit still and less moving during the recording. Besides that, it is a necessity to have stable electrodes. In this study, ICA decomposition was applied to detect the ICA components with electrode-pop and electrostatic artifacts. We rejected those components to reduce the effect of these artifacts from the EEG record. The process of eliminating these ICA components was carried out under EEGLAB toolbox in Matlab (Delorme and Makeig 2004).
7.4.5 Reducing the Physiological Artifacts
Myogenic or muscle potentials are the most common artifacts in EEG recordings. Frontalis and temporalis muscles (e.g., clenching of jaw muscles) are common causes. Generally, the potentials generated in the muscles are of shorter duration than those generated in the brain and are identified easily on the basis of duration, morphology, and rate of firing (e.g., frequency). Other common physiological artifact is eye movement. Eye movement can simulate a plausible EEG slow wave having eyeball origin. Eyeball artifacts are caused by the potential difference between the cornea and retina, which is quite large compared with cerebral potentials. When the eye is completely still, this is not a problem. But, there are nearly always small or large reflexive eye movements, which generates a potential that is picked up in the frontopolar and frontal leads. Involuntary eye movements, known as saccades, are caused by ocular muscles, which also generate electromyographic potentials. Purposeful or reflexive eye blinking also generates electromyographic potentials, but more importantly, there is reflexive movement of the eyeball during blinking which gives a characteristic artifactual appearance of the EEG.
Those two artifacts above are the focus of physiological artifact in this study. Preventing action like asking subjects to control their blinking and taking the break between the recordings might reduce the occurrence of these artifacts. Applying ICA-based artifact correction can separate and remove these artifacts from EEG data (Jung et al. 2000a, b). Several useful heuristics can be used to discriminate them. For the eye movements, they should project mainly to frontal sites with a low-pass time course. For the eye blinks, they also project to frontal sites, but they have large punctate activations. For muscle artifacts, they usually project to temporal sites with a spectral peak above 20 Hz. Sample of specific topography for artifacts can be seen at Fig. 7.5.
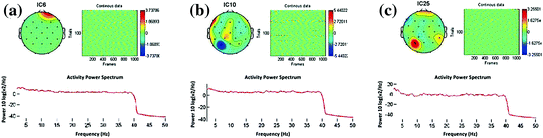
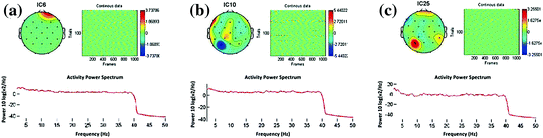
Fig. 7.5
Topography of EEG artifacts. a Eye artifact. b Muscle artifact. c Electrode-pop artifact
7.4.6 The Windowing
Brain electrical activity recordings consist of time-varying measurements of the scalp electric potential field, performed for spontaneous activity (EEG) or for ERPs. In studies of ERPs, these recordings are interpreted as being formed by a sequence of components. Each component appears as a peak or trough in the voltage versus time plot, characterized by a certain amplitude and latency value. The different components are assumed to reflect different functional states of the brain, corresponding to different stages of information processing. Therefore, the determination of the functional states and their time sequencing constitutes an important problem of electrophysiology.
7.4.7 K-Means Clustering
In studies of ERPs, the brain signals are interpreted as being performed by a sequence of components. Instead of viewing these sequences of components in the waveforms, k-means clustering technique tries to segment the brain activities into a sequence of momentary potential distribution maps (microstates). In simple way, k-means clustering technique tries to view the multi-channel records of EEG data as a sequence of microstates. Microstate is a stable topographical scalp field persists during an extended epoch or time segment (Lehmann and Skrandies 1984). Each microstate presumably reflects the different step or mode or content of information processing (Michel et al. 1992). But, we have to be careful, because the successive occurrence of microstates does not imply that brain information processing is strictly sequential. The underlying mechanism by which the brain enters a microstate with a given neuronal generator distribution may be composed of any number of sequential or parallel physiological sub-processes.
The scalp electromagnetic field reflects the source distribution in the brain. Due to the non-uniqueness of the electromagnetic inverse problem, it may occur that different source distributions produce exactly the same microstate. However, changes in the microstate are undoubtedly due to changes in the source distribution. Therefore, brain electrical activity can then be seen as a sequence of non-overlapping microstates with variable duration and variable intensity dynamics (Pascual-Marqui et al. 1995). In this technique, the EEG data are assumed to be reference free, and the entire data set at all-time points are examined simultaneously. Therefore, this technique should be applied to averaged EEG (or ERP) data after re-referenced to an average reference. Mathematical and statistical detail of this method can be seen at the study of Pascual-Marqui et al. (1995). Sequential process of this segmentation or windowing with k-means clustering technique can be seen at Table 7.1. Result of windowing with k-means clustering technique can be seen at Fig. 7.6.
Table 7.1
Sequential process of windowing with k-means clustering technique
1. Raw EEG data |
2. Artifact reduction process |
3. Re-referencing the ‘clean’ EEG data to average reference |
4. Averaging the ‘clean’ EEG data over subjects and conditions |
5. Applying k-means clustering technique |
5.1. Basic k-means clustering |
5.1.1. Checking data |
5.1.2. Setting criteria: initializing microstates |
5.1.3. Labeling each measurement to the closest microstate |
5.1.4. Computing new microstates based on labeling results |
5.1.5. Computing noise variance for the new microstates |
5.1.6. Checking for the convergence of noise variance
![]() Stay updated, free articles. Join our Telegram channel![]() Full access? Get Clinical Tree![]() ![]() ![]() |