10.1 Introduction
This chapter considers the accuracy of diagnostic tests and procedures. It also considers implications of diagnostic test accuracy in population-based screening programs.
The accuracy of a diagnostic test is a function of the procedure and technology used to collect information. Data can be derived by personal interview, self-administered questionnaire, abstraction of medical records, or direct examination of study subjects. Direct examinations may be based on symptoms, signs, and diagnostic test results. Symptoms are subjective sensations, perceptions, and observations made by the patient. Examples of symptoms are pain, nausea, fatigue, and dizziness. Signs are perceptions and observations made by an examiner. Although signs tend to be more objective than symptoms, they are still influenced by the skill and judgment of the examiner. Diagnostic tests are measures of physical, physiologic, immunologic, and biochemical processes. Tests can range from the mundane (e.g., body temperature) to the technical (e.g., clinical chemistry). It is important to note that different methods of case ascertainment may derive different epidemiologic results (Table 10.1).
Table 10.1 Comparison of prevalence estimates of selected chronic conditions as determined by household interviews and clinical evaluations, all ages combined.
Prevalence per 1000 | ||
Condition | Household interview | Clinical evaluation |
Heart disease | 25 | 96 |
Hypertension | 36 | 117 |
Arthritis (any type) | 47 | 75 |
Neoplasms (any type) | 8 | 55 |
Source: Adapted from Lilienfeld and Lilienfeld (1980, p. 150); data from Commission on Chronic Illness (1957).
Even objective procedures demonstrate intra- and inter-observer variability. Figure 10.1 displays blood glucose determinations on a single pooled blood specimen sent to ten different clinical laboratories. Values derived by each lab were compared with the true glucose level determined by a definitive (“gold-standard”) technique with no known sources of error, that is, isotope dilution-mass spectrometry. The true value determined by this state-of-the-art method was 5.79mmol/l. However, readings within clinical labs (intra-observer reliability) and between clinical labs (inter-observer reliability) varied widely.
The accuracy of any diagnostic method is characterized by two distinct elements: its reliability (agreement upon repetition) and its validity (ability to discriminate between people with and without disease). These elements are considered separately.
10.2 Reliability (agreement)
Essential background
Reliability refers to the extent to which intra- or inter-rater ratings agree from one evaluation to the next. Thus, this parameter is also referred to as agreement and reproducibility.
Measurements that fail to agree with each other upon repetition are unreliable, whereas those with high levels of agreement are reliable. For example, if two physicians consistently agreed with each other on the diagnoses of a series of patients, this would indicate a high degree of inter-rater reliability. In contrast, if there were many diagnostic disagreements, this would indicate inter-rater unreliability.
Figure 10.1 Blood glucose determinations of a pooled sample of blood according to ten clinical laboratories in Sweden. The horizontal dashed line represents the actual glucose level of the samples as determined by a definitive method known as isotope dilution-mass spectrometry (Based on data in Björkhem et al., 1981 and Ahlbom and Norell, 1990, p. 17).

A classic 1966 study of diagnostic reproducibility by Lilienfeld and Kordan found a significant number of discrepancies in the interpretation of chest X-rays read by radiologists. Using six diagnostic categories, the observed level of diagnostic agreement was a modest 65.1% (Table 10.2). When the diagnostic classification scheme was simplified to form only two diagnostic categories—significant pulmonary lesion, yes or no—diagnostic agreement improved to 89.4% (Table 10.3). These agreement levels are all the less impressive when one considers that among the 3558 X-rays labeled as positive by at least one of the radiologists, agreement was present in only 1467 (41.2%)—and this does not account for agreement due to chance.
Table 10.2 Comparison of two different radiologists’ interpretations of chest X-ray films; outlined diagonal represents areas of diagnostic agreement.

Table 10.3 Comparison of two different radiologists’ interpretations of chest X-ray filmsa.

Proportion of agreement in subjects labeled positive by at least one radiologist
The kappa statistic
The kappa statistic (κ) was developed to measure the level of agreement between rates that occurs beyond that due to chance (Cohen, 1960). Consider an experiment that simultaneously flips two coins (Figure 10.2). We expect that the two coins would agree heads or tails half of the time. Thus, the expected level of agreement due to chance is 50%. The kappa statistic is constructed so that when the observed agreement is no greater than that which is expected due to chance, κ = 0. Greater than chance agreement leads to positive values of κ. When there is complete agreement, κ = + 1. One widely used benchmark scale for characterizing the strength of agreement indicated by kappa values is shown as Table 10.4.
Table 10.4 Benchmark scale for interpreting kappa according to Landis and Koch (1977).
Kappa statistic | Strength of agreement |
<0.0 | Poor |
0.0–0.20 | Slight |
0.21–0.40 | Fair |
0.41–0.60 | Moderate |
0.61–0.80 | Substantial |
0.81–1.00 | Almost perfect |
Table 10.5 Notation for measuring agreement for a binary diagnostic test.

To calculate kappa for a binary outcome (condition present or absent), data are laid out in a two-by-two table with the notation shown in Table 10.5. Using this notation, the observed proportion of agreement is
10.1
the expected proportion of agreement due to chance is
10.2
and Cohen’s kappa statistic is
10.3
For the X-ray inter-rater agreement data presented in Table 10.3:



The kappa paradox
The κ has an important limitation: It is affected by the prevalence of the condition being studied. This causes an effect by which two raters can have high agreement but still emerge with a low kappa value. This problem is referred to as the kappa paradox (Feinstein and Cicchetti, 1990).
Table 10.6 Demonstration of the kappa paradox (Feinstein and Cicchetti, 1990).

The data in Table 10.6 demonstrate a kappa paradox. Table 10.6A demonstrates observed proportions of agreement (pobs) of 0.85 and kappa of 0.70 (“substantial agreement”). Table 10.6B also demonstrates observed proportions of agreement pobs of 0.85, but in this case the kappa statistic is 0.32 (“fair agreement”).
Several options have been offered as solutions to the kappa paradox. One approach uses alternative measures of agreement that are resistant to the kappa paradox. Two such alternatives are the Brennan–Prediger kappa coefficient (also called the G index; Holley and Guilford, 1964; Brennan and Prediger, 1981) and Gwet’s AC1 (Gwet, 2010).a
These statistics can be calculated with WinPEPI’s PairsEtc → “A. ‘Yes-no’ (dichotomous) variable” program.
One practical solution to the kappa paradox is to accompany the kappa statistic with the proportion of specific positive agreement (ppos), which is
10.4
and the proportion of specific negative agreement (pneg)
10.5
Use of these statistics to complement κ provides a more complete picture of the agreement between the two raters.
In Illustrative Example 10.1 (Table 10.3) we calculated and observed a level of agreement of 89.4% and κ statistic of 0.52. The proportion of positive agreement for these data is
The proportion of negative agreement is
This indicates that the agreement for positive diagnoses is inferior to that of negative diagnoses, suggesting that further work is needed to decrease the observers’ disparities in the positive direction.
10.3 Validity
We use the term validity to describe the ability of a test or diagnostic procedure to accurately discriminate between people who do and do not have the disease of interest. A perfectly reliable and valid test would correctly discriminate between people with and without disease without fail.
We will discuss four measures of diagnostic test validity: sensitivity, specificity, predictive value positive, and predictive value negative. To calculate these measures, we must first classify test results into one of the following four categories:
- True positives (TP) have the disease in question and show positive test results.
- True negatives (TN) do not have the disease in question and show negative test results.
- False positives (FP) do not have the disease in question but show positive test results.
- False negatives (FN) have the disease but show negative test results.
This assumes there is a definitive “gold standard” means of identifying individuals who have and do not have the disease in question by which to make these classifications. After each result is classified into one of the above four categories, the frequency of results is cross-tabulated to form a table similar to the one shown in Table 10.7.
Table 10.7 Notation for calculating sensitivity, specificity, predictive value positive, and predictive value negative.

Sensitivity and specificity
Sensitivity (SEN) is the probability that a test result will be positive when the test is administered to people who actually have the disease or condition in question. Using conditional probability notation, we define sensitivity as Pr(T+ |D+), where Pr denotes “probability,” T+ denotes “test positive,” D+ denotes “disease positive,” and the vertical line (|) denotes “conditional upon.” Thereby, Pr (T+ |D+) is read as “the probability of being test positive conditional upon being disease positive.”
Sensitivity is calculated by administering the test to subjects who have the disease in question. The number of diseased people who test positive is divided by the total number of diseased people tested:
10.6
Specificity (SPEC) is the probability that a test will be negative when administered to people who are free of the disease or condition in question. In other words, specificity is the probability of being test negative conditional upon being disease negative: SPEC = Pr(T– |D–).
Specificity is calculated by administering the test to disease-free subjects. The number of people testing negative is divided by the total number of disease-free people tested:
10.7
To illustrate sensitivity and specificity, let us consider a hypothetical survey of teen smoking in which a questionnaire is used as a screening instrument to help determine whether subjects smoke. We are concerned that many teen smokers will feel compelled to falsely answer in the negative, so we compare the results of the questionnaire to a more reliable method of ascertainment based on testing for cotinine in the saliva. (Cotinine, a major detoxication product of nicotine, is a biomarker for tobacco smoke.) Thus, the questionnaire serves as a rapid and inexpensive screening toll and the salivary cotinine test serves as the “gold standard” method of ascertainment. Results of our study are shown in Table 10.8. Thus
and
Table 10.8 Data for Illustrative Examples 10.4–10.6. Results of a smoking survey questionnaire and definitive salivary cotinine test: fictitious data.

Predictive value positive and predictive value negative
Although sensitivity and specificity quantify a test’s accuracy in the presence of known disease status, they are unable to predict the performance of the test in the population. To accomplish this objective, the alternative indices of predictive value positive and predictive value negative are needed.
The predictive value positive of a positive test (PVPT) is the probability that a person with a positive test will actually have the disease in question. In other words, the predictive value positive is the probability of being disease positive conditional upon being test positive: PVPT = Pr(D+ |T+). This statistic is calculated by dividing the number of true positives by all those people who test positive:
10.8
The predictive value of a negative test (PVNT) is the probability that a person who shows a negative test will be disease negative—the probability of disease negative “given” test negativity: PVNT = Pr(D− |T−). The predictive value negative is calculated by dividing the number of true negatives by all those people who test negative:
10.9
The distinction between sensitivity/specificity and predictive value positive/predictive value negative may at first appear confusing. This becomes less confusing if one remembers that sensitivity and specificity quantify a test’s accuracy given the known disease status of study subjects, whereas predictive values quantify a test’s accuracy given only the test results.
Let us return to the data in Illustrative Example 10.4 on the validity of data derived from a smoking questionnaire. Data are in Table 10.8. In this example, we have 65 true positives and 1 false positive. Therefore,
This means that 98.5% of the study subjects that responded in the affirmative were actually smokers. The false positive rate is the complement of the PVPT. Therefore, the false positive rate was 1 – 0.985 = 0.015.
The questionnaire identified 35 false negatives and 99 true negatives. Since 99 of the 134 people who responded to the questionnaire in the negative were actual nonsmokers,
This means that 73.9% of the negative responders were nonsmokers. The false negative rate is the complement of the PVNT. Therefore, the false negative rate was 1 – 0.739 = 0.261.
True prevalence and apparent prevalence
The prevalence of disease can be calculated on the basis of the true number of people with the disease in the population or the apparent number of people with the disease based on screening test results. The true prevalence of the disease (P) represents the proportion of people who actually have the disease or condition:
10.10
where TP represents the number of true positives, FN represents the number of false negatives, and N represents all those tested.
The apparent prevalence of a disease (P*) represents the proportion of people who test positive on a screening test:
10.11
where TP represents the number of true positives, FP represents the number of false positives, and N represents all those tested.
The apparent prevalence and true prevalence will differ when the screening test is imperfect. In Illustrative Examples 10.3 and 10.4 (Table 10.8), the true prevalence of smoking is
In contrast, the apparent prevalence is
This discrepancy is due to the under-reporting of smoking on the questionnaire.
Relation between prevalence and the predictive value of a positive test
The predictive value of a positive (PVPT) test depends on the sensitivity of the test, the specificity of the test, and the prevalence of the disease in the population in which the test is used. Although the first two determinants of predictive value (sensitivity and specificity) are not surprising, many students are caught off guard by the important role prevalence plays in determining predictive value. In general, if the prevalence of disease is low, the predictive value positive will be low. If the prevalence of disease is high, the predictive value positive will be high. This relationship holds for all diagnostic tests that fall short of perfection.
Consider using a screening test with a sensitivity of 0.99 and specificity of 0.99 in two different populations. Population A has a prevalence of 1 in 10 (0.10). Population B has a prevalence of 1 in 1000 (0.001). Each population consists of 1 000 000 people.
Note that the number of people with disease in each population is equal to the prevalence of disease times the population size:
10.12
Thus, Population A has 0.1 × 1 000 000 = 100 000 cases, and Population B has 0.001 × 1 000 000 = 1000 cases.
Because the SEN of the test is 99%, it correctly identifies 99 000 (99%) of the 100 000 cases in Population A. This leaves 1000 false negatives in this population. In addition, because the SPEC of the test is 99%, it correctly identifies 891 000 (99%) of the 900 000 non-cases as true negatives, leaving 9000 false positives. Table 10.9A shows the results of the test in Population A Using these results, PVPT in Population A is 91.7% (calculations below Table 10.9A).
Using the same type of reasoning, the test correctly identifies 990 (99%) of the 1000 cases and leaves 10 false negatives in Population B. It also correctly identifies 989 010 (99%) of the non-cases as true negatives in Population B, leaving 9900 false positives. The predictive value positive of the test in Population B, therefore, is only 9.0% (Table 10.9B). Thus, the PVPT is substantially lower in Population B than in Population A. This is because of Population B‘s lower prevalence of disease.
Table 10.9 Data for Illustrative Example 10.7. Results of a screening test which has SEN = 0.99 and SPEC = 0.99 in two different populations.

Bayesian formulas for predictive value
The PVPT can be calculated directly from its sensitivity, specificity, and the prevalence of disease in the population it is being used in, if these quantities are known, according to the formula:
10.13
where PVP represents predictive value positive, P represents (true) prevalence, SEN represents sensitivity, and SPEC represents specificity. Because Formula (10.13) is derived using Bayes’s law of probability, it is called the “Bayesian formula for predictive value positive.”
Formula (10.13) is used to calculate the PVPT of the data in Table 10.6. Given the test’s sensitivity of 0.650 and specificity of 0.990, and the population prevalence of 0.500,
This calculated value matches the previously calculated value determined in Illustrative Example 10.5.
The Bayesian formula for the PVPT allows us to plot the predictive value of a positive test as a function of prevalence, sensitivity, and specificity. Figure 10.3 plots this relation for three different diagnostic tests. The sensitivity of all three tests is held constant at 0.99. Specificity varies between 0.80 and 0.99, as labeled in the figure. This figure indicates that all three tests have low predictive value positive when used in populations with low disease prevalence and that the predictive value positive increases as a function of prevalence. It also indicates that tests of low specificity add little new information about the population when the prevalence of disease is low.
Figure 10.3 PVPT as a function of prevalence. All three tests have a sensitivity of 0.99. Tests of three specificities are considered (indicated by SPEC).

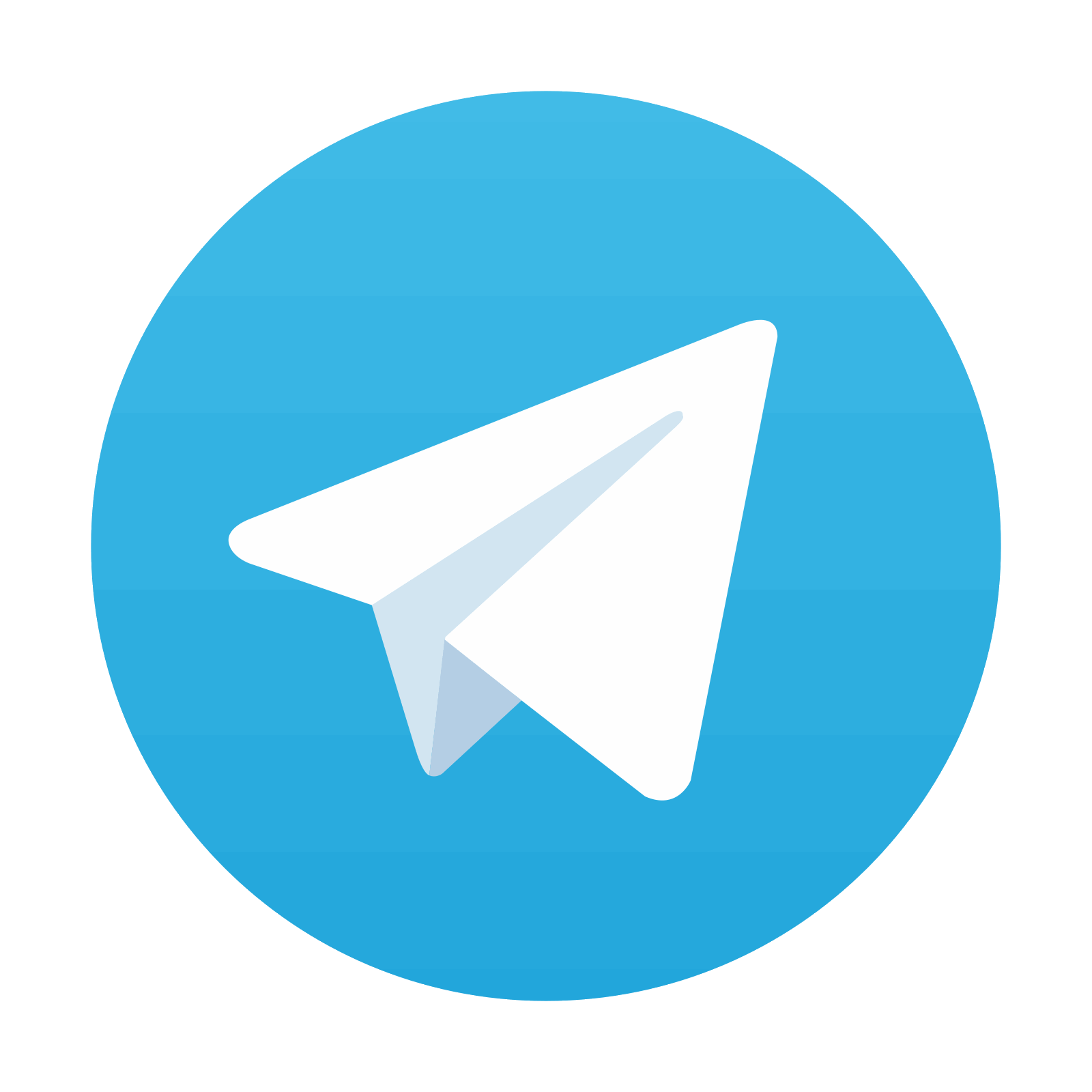
Stay updated, free articles. Join our Telegram channel

Full access? Get Clinical Tree
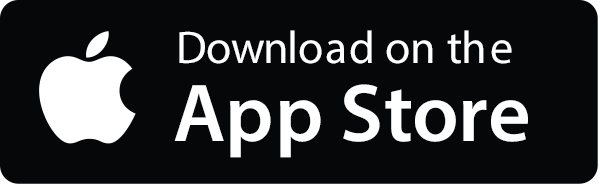
