The Importance of Sample Size
If the number of patients in our study is small, we may have inadequate power (Chapter 18) to detect an important existing effect, and we shall have wasted all our resources. On the other hand, if the sample size is unduly large, the study may be unnecessarily time-consuming, expensive and unethical, depriving some of the patients of the superior treatment. We therefore have to choose the optimal sample size which strikes a balance between the implications of making a Type I or Type II error (Chapter 18). Unfortunately, in order to calculate the sample size required, we have to have some idea of the results we expect in the study.
Requirements
We shall explain how to calculate the optimal sample size in simple situations; often more complex designs can be simplified for the purpose of calculating the sample size. If our investigation involves a number of tests, we concentrate on the most important or evaluate the sample size required for each and choose the largest.
Our focus is the calculation of the optimal sample size in relation to a proposed hypothesis test. However, it is possible to base the sample size calculation on other aspects of the study, such as on the precision of an estimate or on the width of a confidence interval (the process usually adopted in equivalence and non-inferiority studies, Chapter 17).
To calculate the optimal sample size for a test, we need to specify the following quantities at the design stage of the investigation.
- Power (Chapter 18) – the chance of detecting, as statistically significant, a specified effect if it exists. We usually choose a power of at least 80%.
- Significance level, α (Chapter 17) – the cut-off level below which we will reject the null hypothesis, i.e. it is the maximum probability of incorrectly concluding that there is an effect. We usually fix this as 0.05 or, occasionally, as 0.01, and reject the null hypothesis if the P-value is less than this value.
- Variability of the observations, e.g. the standard deviation, if we have a numerical variable.
- Smallest effect of interest – the magnitude of the effect that is clinically important and which we do not want to overlook. This is often a difference (e.g. difference in means or proportions). Sometimes it is expressed as a multiple of the standard deviation of the observations (the standardized difference).
It is relatively simple to choose the power and significance level of the test that suit the requirements of our study. The choice is usually governed by the implications of a Type I and a Type II error, but may be specified by the regulatory bodies in some drug licensing studies. Given a particular clinical scenario, it is possible to specify the effect we regard as clinically important. The real difficulty lies in providing an estimate of the variation in a numerical variable before we have collected the data. We may be able to obtain this information from published studies with similar outcomes or we may need to carry out a pilot study. Although a pilot study is usually a distinct preliminary investigation, we may incorporate the data gathered in the pilot study into the main study using an internal pilot study 1, provided all details of it are documented in the protocol. We determine the optimal sample size on the best, although perhaps limited, information available at the design stage of the study. We then use the relevant information from a pilot study (the size of which is pre-specified, may be relatively large and is usually determined through practical considerations) to revise our estimated sample size for the main study. (Note: the calculation must be based on the originally defined smallest effect of interest, not on the effect observed in the pilot study, and the revised sample size estimate utilized only if it exceeds the original estimate.) In such situations, the information gathered in the internal pilot study may be used in the final analysis of the data.
Methodology
We can calculate sample size in a number of ways, each of which requires essentially the same information (described in Requirements) in order to proceed:
- General formulae2 – these can be complex but may be necessary in some situations (e.g. to retain power in a cluster randomized trial (Chapters 14 and 41), we multiply the sample size that would be required if we were carrying out individual randomization by the design effect equal to [1 + (m − 1)ρ], where m is the average cluster size and ρ is the intraclass correlation coefficient (Chapter 42)).
- Quick formulae – these exist for particular power values and significance levels for some hypothesis tests (e.g., Lehr’s formulae3, see next page).
- Special tables2 – these exist for different situations (e.g. for t-tests, Chi-squared tests, tests of the correlation coefficient, comparing two survival curves, and equivalence studies).
- Altman’s nomogram – this is an easy-to-use diagram which is appropriate for various tests. Details are given in the next section.
- Computer software – this has the advantage that results can be presented graphically or in tables to show the effect of changing the factors (e.g. power, size of effect) on the required sample size.
Altman’s Nomogram
Notation
We show in Table 36.1 the notation for using Altman’s nomogram (Appendix B) to estimate the sample size of two equally sized groups of observations for three frequently used hypothesis tests of means and proportions.
Table 36.1 Information for using Altman’s nomogram
Method
For each test, we calculate the standardized difference and join its value on the left-hand axis of the nomogram to the power we have specified on the right-hand vertical axis. The required sample size is indicated at the point at which the resulting line and sample size axis meet.
Note that we can also use the nomogram to evaluate the power of a hypothesis test for a given sample size. Occasionally, this is useful if we wish to know, retrospectively, whether we can attribute lack of significance in a hypothesis test to an inadequately sized sample. In such post hoc power calculations, the clinically important treatment difference must be that which was decided a priori; it is not the observed treatment effect. Remember, also, that a wide confidence interval for the effect of interest indicates an imprecise estimate, often due to an insufficiently sized study (Chapter 11).
Quick Formulae
For the unpaired t-test and Chi-squared test, we can use Lehr’s formula3 for calculating the sample size for a power of 80% and a two-sided significance level of 0.05. The required sample size in each group is
If the standardized difference is small, this formula overestimates the sample size. Note that a numerator of 21 (instead of 16) relates to a power of 90%.
Power Statement
It is often essential and always useful to include a power statement in a study protocol or in the methods section of a paper (see CONSORT Statement, Chapter 14) to show that careful thought has been given to sample size at the design stage of the investigation. A typical statement might be ‘84 patients in each group were required for the unpaired t-test to have a 90% chance of detecting a difference in means of 2.5 days (SD = 5 days) at the 5% level of significance’ (see Example 1).
Adjustments
We may wish to adjust the sample size:
- to allow for losses to follow-up by recruiting more patients into the study at the outset. If we believe that the drop-out rate will be r%, then the adjusted sample size is obtained by multiplying the unadjusted sample size by 100/(100 − r).
- to have independent groups of different sizes. This may be desirable when one group is restricted in size, perhaps because the disease is rare in a case–control study (Chapter 16) or because the novel drug treatment is in short supply. Note, however, that the imbalance in numbers usually results in a larger overall sample size when compared with a balanced design if a similar level of power is to be maintained. If the ratio of the sample sizes in the two groups is k (e.g. k = 3 if we require one group to be three times the size of the other), then the adjusted overall sample size is
where N is the unadjusted overall sample size calculated for equally sized groups. Then N′/(1 + k) of these patients will be in the smaller group and the remaining patients will be in the larger group.
Increasing the Power for a Fixed Sample Size
If we regard the significance level and important treatment difference defined by a particular variable as fixed (we can rarely justify increasing either of them) and assume that our test is two-tailed (a one-tailed test has greater power but is usually inappropriate (Chapter 17)), we can increase the power for a fixed sample size in a number of ways. For example we might:
- use a more informative response variable (e.g. a numerical variable such as systolic blood pressure instead of the binary responses normal/hypertensive);
- perform a different form of analysis (e.g. parametric instead of non-parametric);
- reduce the random variation when collecting the data (e.g. by standardizing conditions or training observers (Chapter 39));
- modify the original study design in such a way that the variability in measurements is reduced (e.g. by incorporating stratification or using matched pairs instead of two independent groups (Chapter 13)).
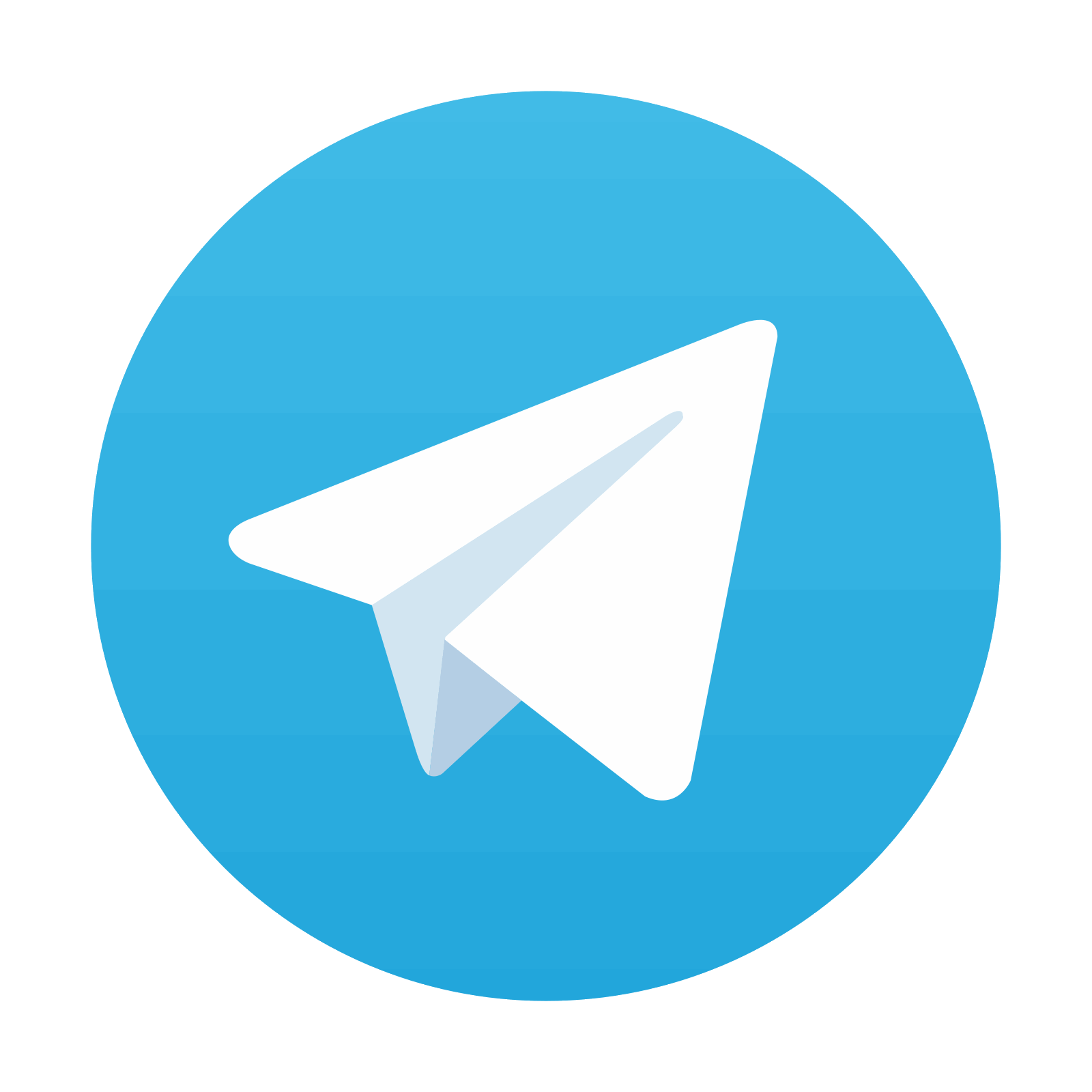
Stay updated, free articles. Join our Telegram channel

Full access? Get Clinical Tree
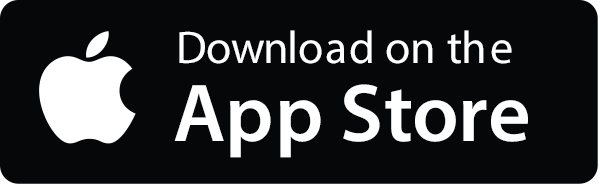
