Biomedicalizing Difference in Post-Genomic Subjects
Introduction: The Values of Inclusion and Interaction
What determines who gets sick and who does not, who dies and who lives? What accounts for the often systematic and stratified patterns of disease distribution across human populations? How do we know – or think we know – where and how to intervene in these patterns of disease and risk, and on whom? In turn, what drives how we shape our healthcare systems, our clinical practices and our public health recommendations? Our answers to these questions have profound impacts on the design of our health programs, the kinds of health-related advice we give to individuals and families, and our conceptions of the distribution of responsibility for promoting and maintaining health and addressing disease. Understandably then, these questions have preoccupied the many scientific disciplines that have contributed to our knowledge of health and illness.
In the twenty-first century, a confluence of unique trends in the landscape of health research has shifted the ways in which these questions are being posed and the approaches taken to answer them. First, “complexity” has emerged as a new keyword in discourses about and research on disease causation. However, the ways in which scientists interpret the notion of causal complexity and put it into practice encompass widely variable ideas, models and frameworks for thinking about environment, genes, biology, social factors and culture. Second, new scientific research technologies and capacities – in genomics, clearly, but also in computing and statistics – enable new and different kinds of questions to be asked and pursued. Finally, there have been significant shifts in scientists’ and the public’s understandings of the research enterprise, from the growing appeal of inter- and transdisciplinary approaches (that aim for true integration rather than merely side-by-side multidisciplinary efforts), to consortium models of research, to cohorts of participants becoming research platforms for multiple studies. These new modes of research work to codify many different versions and variations of “complexity” (Shim et al. 2014a).
To trace these post-genomic transformations of race and ethnicity, from 2010 to 2014 we recruited US-based genome scientists conducting research that fit a number of eligibility criteria: (a) identifiable as gene–environment interaction (GEI) research – that is, studies that try to account for the interactions of genetic with environmental factors; (b) inclusion of at least one non-white population, that is, those who had designed their studies in a way to enable comparisons between racial/ethnic groups; and (c) research on the etiology of heart disease, type 2 diabetes and cancer – all conditions for which there exists wide agreement that they are caused by multiple, interacting genetic, behavioral and environmental determinants. We conducted in-depth, semi-structured interviews with these scientists to gather data on their actual research practices – including how and why specific study designs, samples, variables, measures, measurement tools and analytic procedures are selected and implemented – and how these practices have changed over the course of a study. We also conducted observations and informal interviews at scientific conferences where findings from GEI research are presented. This chapter is based on fifty-four in-depth interviews with thirty-three GEI scientists (twenty-one of these interviews were follow-up interviews conducted approximately one year after the first interview), and over 200 hours of observation and additional informal interviews at nine scientific conferences where findings from GEI research were presented. All interview transcripts, observation field notes and selected verbatim transcriptions of podium presentations and question-and-answer sessions were uploaded to the qualitative data analysis software ATLAS.ti. Initial codes were first generated inductively through a collaborative reading and analysis of a subset of interviews, and then finalized through successive waves of coding into approximately eighty categories and codes. The ATLAS.ti query tool was used to extract data tagged with particular codes and we wrote, circulated and revised memos on these queries.
In addition to the changing landscape of biomedical research in the post-genomic era, two other touchstones serve as points of departure for this project. The first is Steven Epstein’s (2007) Inclusion. Epstein traces, from the 1990s onward, a transformative shift in our medical “common sense,” from the notion that health research conducted on white, middle-aged men could be broadly generalizable to other human populations, to a new “inclusion-and-difference” paradigm that held that diverse individuals must be included in biomedical research to investigate group differences. As Epstein states, we are now fully in an age of inclusion, in which the regulatory mandate to include underrepresented populations has become both scientific and social common sense.
All of the populations that were being studied … were Caucasian. Why? Because it was easy to collect those families … [We] said, “This is ridiculous. We know there are huge health disparities. And no one is making an effort to find out why. We have got to start a study that is going to enroll large numbers of African American men and their families and try and figure this out.” And that’s what we did … [We] went out and found terrific mostly African American clinicians around the country. Put out a contract. These guys got together. They designed their study. They collected these families from all across the country and were phenomenally successful in enrolling African American families so that we finally have a decent number.
Another researcher summarized: “So right now, I think there’s no doubt that there is a sense of trying to spread the biomedical dollars to assess questions and other ethnic groups and populations simply because it exposes a much greater diversity of the problem.” Thus our scientists seem to be guided by a moral and scientific sensibility that inclusion is necessary to pose questions of significant public health impact, to examine questions of genomic and environmental causation within a broadly representative population, and/or to fulfill an ethical mandate to address health disparities.
A second point of departure for our project is Pamela Sankar’s (2008) call to move beyond “the two-race mantra.” She argues that it is time to let go of the notion that there are two – and only two – conceptions of race: that it is either biological or social.2 Relatedly, Catherine Bliss (2012; 2015, Chapter 9, this volume) has also traced the emergence of a new “sociogenomic” paradigm in genomic research that calls for a combination of both social and biological inquiry, a race-conscious genomics that explicitly accounts for the social realities of race.
Again, this call is also something we have heard among our participants. To be sure, given that we recruited those engaged in GEI research, it is no surprise that we would see a commitment to examining disease determinants all along the causal spectrum. But at the scientific conferences where we conducted observations, this was also a widely invoked refrain among researchers. Indeed the mandate to examine the influence of race along both biological and social dimensions was framed as part of the solution to the issue of “missing causality.” That is, for complex diseases like heart disease, cancer and diabetes, all of the known contributors to disease still do not fully explain who develops the condition and who does not. Thus a great deal of energy and debate is spent on how to solve the etiological puzzle of complex diseases, in both genetic and environmental respects. The key intervention that was articulated to locate the “missing causality” was the inclusion of diverse populations in etiologic studies. Our study therefore sought to trace how this diversity of populations – mobilized as an important solution to health research – is being accounted for by investigators of GEI research.
Situating Race in Post-Genomic Science
Our aim is to contribute to an expanding literature on the invocation, uses and constructions of race and population differences in genomic research (e.g., Reardon 2005, 2009; Lee 2006; Abu El-Haj 2007; Fullwiley 2007, 2008, 2011; Braun and Hammonds 2008; Lee et al. 2008; Whitmarsh 2008; Fujimura and Rajagopalan 2011; Montoya 2011; Bliss 2012). One widespread claim in the genomics literature is that the approaches and techniques of human population genetics enable a shift from typological notions of race to statistical notions of difference among populations – that is, from an understanding of race as defined categories or groups, to one that conceptualizes racial differences as more continuous without discernable boundaries or divisions (e.g., Dobzhansky 1963; Lewontin 1972; Cavalli-Sforza and Bodmer 1999[1974]). However, multiple scholars who have analyzed genomic and post-genomic research practices take serious issue with the claim that “old” ideas about racial categories arrayed in a hierarchy have been eclipsed by a “new” logic of populations whose differences greatly overlap and are much more gradual and continuous.
Gannett (2001) argues that the notion of population has not attenuated scientists’ inclination to identify and measure group differences that incorporate cultural meanings of race, or precluded stereotypical and racist interpretations of statistical patterns. Similarly, even when scientists are equipped with statistical software that in theory allows them to identify and define population clusters without an a priori use or conceptualization of race, Fujimura and Rajagopalan (2011) found that researchers still brought together notions of population, race and genetic ancestry in ways that were difficult to disentangle. In fact, Lee (2005) identified an “infrastructure of racialization” in post-genomic research wherein biobanks incorporated racialized labels3 for groups whose DNA samples they store. In so doing, they privileged the body as the grounds in and from which racial difference can be read and genomics as the official language for reading race.
Finally, Bliss (2011, 2012) found in her study of elite genomic scientists that even while these scientists acknowledged the great heterogeneities within racial groups, they attended to race in research participation as a means to ensure racial equality in research and drug development. In the shift “from practicing colorblind science to race positive inquiry” (2012:67), Bliss found that elite scientists were no longer content to use “race” as a catch-all proxy or black box variable for other potential disease determinants. Instead, they were committed to measuring those previously uninterrogated factors in and of themselves. Bliss argues that within an “anti-racist racialism,” researchers strategically incorporate racial populations into genome science with the goals of deconstructing biological race and producing an inclusive genomic science.
Given these emergent and enduring connections between genome science and race, ethnicity and ancestry, we summarize the shifting practices of using self-identified race and ethnicity (SIRE) and ancestry informative markers (AIMs), and scientists’ specific concerns about and commitments to the investigation of human and social differences. These results have been reported in full elsewhere (Shim et al. 2014b). We argue that GEI researchers’ uses of SIRE and AIMs both construct and reflect particularly individualizing understandings of race and human bodies that echo various processes of biomedicalization (Clarke et al. 2003, 2010b).4 We suggest that bringing both biomedicalization and feminist theories to bear on these phenomena shows how these compulsions shape, in deeply consequential ways, our understandings of human difference in relative rather than relational terms. That is, human differences are perceived to be characteristics of individuals, arrayed on a continuum and relative to one another, rather than the outcomes of unequal relationships and interactions between socially defined groups. Thus researchers were preoccupied with technical questions of how to classify and measure genetic variation, and how to standardize such classifications. These concerns underscore how tropes of precision, individualization and the continuity of human variation serve to promote genetic expressions of ancestry and to displace techniques that attend to group-based processes and social relations of power. In contrast, we argue that biomedicalization and feminist theories help to highlight and problematize the individualization and personalization of difference.
SIRE: The Convenience and Imprecision of Standardized Categories
As noted above, SIRE indicates the use of self-identification as a means to determine race and ethnicity. For the vast majority of the scientists we observed and interviewed, SIRE refers to the placement of oneself into one or more of the racial and ethnic categories defined by the US Office of Management and Budget (OMB) and required for use in biomedical research funded by the US National Institutes of Health (NIH). This use of SIRE is seen by our participants as now embedded and entrenched in the social practices of conducting research, as mandated by the NIH. They speak of these categories as “what we have” and what they “have to use” because “all NIH research requires [that]”; they “make that question [of race and ethnicity] fit those requirements” in order to “conform with what we have to report to the NIH.” These characterizations of SIRE by the scientists underscore its use as a technique of convenience precisely because its collection is compelled by government funding agencies. One scientist rationalized, “I think the path of least resistance is to stick with self-identified race ethnicity,” and another explained that, “When we’re measuring it de novo … I try to ask it in categories that conform to the NCI [National Cancer Institute, one of the institutes that make up the NIH] reporting requirements. Make it easy on everybody.”
Thus, because of its institutionalization into biomedical data collection practices and standardization across research studies, SIRE is largely considered methodologically robust and consistent, at least in the USA. However, its conceptual and analytic utility and the kind of questions it answers about etiology are often seen as inadequate. Thus while the measure may exhibit reliability in a technical sense, it is often not perceived to be valid in a scientific sense (see also Shim 2014). Researchers interviewed for this study therefore describe SIRE as having potential but also potential pitfalls, on a spectrum from necessary and convenient, to limited and questionable. While many justify the use of SIRE for various reasons, mostly having to do with regulatory requirements and expediency, they also feel compelled to question its adequacy in these very regards.
On the other hand, while the categories by which scientists measure self-identified race and ethnicity may have been standardized and intended to be used in specific ways, the particular practices surrounding their use often do vary. For example, several of the scientists mentioned the confusion regarding the status of “Hispanic/Latino” as an ethnicity, but not a race.5 One scientist said explicitly that while they report Hispanic ethnicity to the NIH as mandated, when they do data analyses in their study, they consider Hispanic to be another race. Multiple researchers also described the dilemmas they face when subjects select multiple categories: “If people report multiple things, then we say African-American trumps everything else. Then Hispanic then trumps everything else that’s remaining. And then the Asian trumps everything else that’s remaining. That’s kind of what I think the standard thing that people do.” Another participant recalled:
We’ve often gone to try to get more granular self-report information … having lots of different possibilities, having lots of different multi-ethnic designations … What we do is we give them the opportunities, then we look at the numbers [of participants choosing those other designations], and it’s so small … that we ended up having to fold them back into the same categorization that we’ve talked about here that has been used for a long time.
Thus even for those who offer multiple possibilities for self-identification that try to go beyond just picking one box, the numbers of subjects who took advantage of such opportunities were so small as to render those more capacious identifications irrelevant when it came to data analysis. Notably, these scientists on the one hand affirm the right for subjects to self-identify as multiracial, but then on the other hand, need to assign them to only one category in the analysis phase.
SIRE thus reflects some of the defining attributes of standards and standardization (Bowker and Star 1999; Lampland and Star 2009; Timmermans and Epstein 2010) in that the racial and ethnic categories with which individuals are asked to identify are more or less made uniform through NIH regulations. And in a nominal way, the practice of self-identification itself has become a standard operating procedure, although as we describe above, the particulars of implementation are by no means widely agreed upon. However, we argue that it is precisely the attributes of standards – and in particular, of the standardized categories themselves – that elicit scientists’ ambivalence about the scientific utility of study subjects’ actual, contingent and complex self-identification, even as they express a commitment to individuals’ self-representation and self-determination.
To illustrate this point, we elaborate on our scientist-participants’ critiques of SIRE. A central focus of their critique involved their understanding of SIRE as a crude, imprecise and overly confounded metric of human difference. One scientist noted:
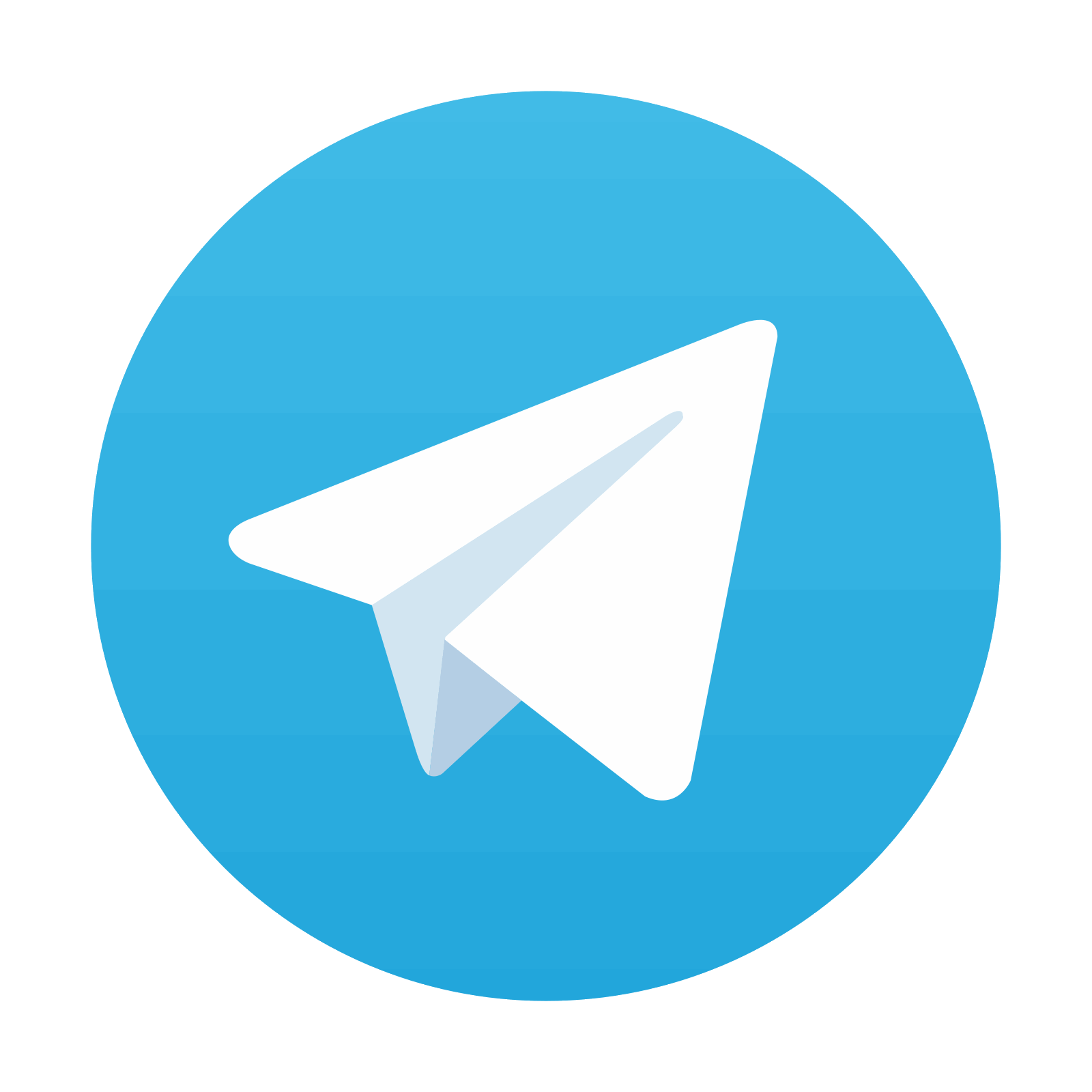
Stay updated, free articles. Join our Telegram channel

Full access? Get Clinical Tree
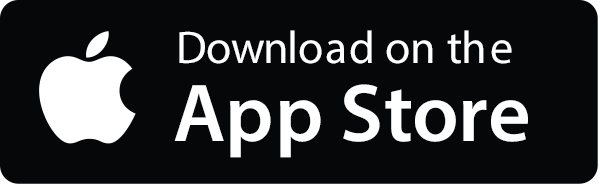
