Various regression methods can be used for the analysis of the two-level hierarchical structure described in Chapter 41, in which each cluster (level 2 unit) contains a number of individual level 1 units. For example, in a study of rheumatoid arthritis, we may measure the flexion angle on both the left and right knees (level 1) of every patient (level 2). Alternatively, we may have a longitudinal data set with a measurement (e.g. total cholesterol) observed at successive times (level 1) on each patient (level 2). The main advantages and disadvantages of each method are summarized in Table 42.1. Most of these methods are unreliable unless there are sufficient clusters, and they can be complicated to perform and interpret correctly; we therefore suggest you consult a specialist statistician for advice.
Table 42.1 Main advantages and disadvantages of regression methods for analysing clustered data.
Method | Advantages | Disadvantages |
Aggregate level analysis |
|
|
Robust standard errors that allow for clustering |
|
|
Random effects model |
|
|
Generalized estimating equations (GEE) |
|
* These points may sometimes be regarded as advantages, depending on the question of interest.
Aggregate Level Analysis
A very simple approach is to aggregate the data and perform an analysis using an appropriate numerical summary measure (e.g. the mean) for each cluster (e.g. the patient) (Chapter 41). The choice of this summary measure will depend on features of the data and on the hypotheses being studied. We perform an ordinary least squares (OLS) multiple regression analysis using the cluster as the unit of investigation and the summary measure as the outcome variable. If each cluster has been allocated a particular treatment (in the knee example, the patient may be randomly allocated one of two treatments – an exercise regimen or no exercise), then, together with other cluster level covariates (e.g. sex, age), we can incorporate ‘treatment’ in the regression model as a dummy variable using codes such as 0 and 1 (or as a series of dummy variables if we have more than two treatments (Chapter 29)).
Robust Standard Errors
If the clustering is ignored in the regression analysis of a two-level structure, an important assumption underlying the linear regression model – that of independence between the observations (see Chapters 27 and 28) – is violated. As a consequence, the standard errors of the parameter estimates are likely to be too small and, hence, results may be spuriously significant.
To overcome this problem, we may determine robust standard errors of the parameter estimates, basing our calculation of them on the variability in the data (evaluated by appropriate residuals) rather than on that assumed by the regression model. In a multiple regression analysis with robust standard errors, the estimates of the regression coefficients are the same as in OLS linear regression but the standard errors are more robust to violations of the underlying assumptions, our particular concern being lack of independence when we have clustered data.
Random Effects Models
Random effects models1 are also known as (for example) hierarchical, multilevel, mixed or cluster-specific models, and as cross-sectional time series, panel or repeated measures models when the data are longitudinal. They can be fitted using various comprehensive statistical computer packages, such as SAS and Stata, or specialist software such as MLwiN (www.cmm.bristol.ac.uk), all of which use a version of maximum likelihood estimation. The estimate of the effect for each cluster is derived using both the individual cluster information as well as that of the other clusters so that it benefits from the ‘shared’ information. In particular, shrinkage estimates are commonly determined whereby, using an appropriate shrinkage factor, each cluster’s estimate of the effect of interest is ‘shrunk’ towards the estimated overall mean. The amount of shrinkage depends on the cluster size (smaller clusters have greater shrinkage) and on the variation in the data (shrinkage is greater for the estimates when the variation within clusters is large when compared to that between clusters).
A random effects model regards the clusters as a sample from a real or hypothetical population of clusters. The individual clusters are not of primary interest; they are assumed to be broadly similar with differences between them attributed to random variation or to other ‘fixed’ factors such as sex, age, etc. The two-level random effects model differs from the model which takes no account of clustering in that, although both incorporate random or unexplained error due to the variation between level 1 units (the within-cluster variance, σ2), the random effects model also includes random error which is due to the variation between clusters, . The variance of an individual observation in this random effects model is therefore the sum of the two components of variance, i.e. it is
.
Particular Models
When the outcome variable, y, is numerical and there is a single explanatory variable, x, of interest, the simple random intercepts linear two-level model assumes that there is a linear relationship between y and x in each cluster, with all the cluster regression lines having a common slope, β, but different intercepts (Fig. 42.1a). The mean regression line has a slope equal to β and an intercept equal to α, which is the mean intercept averaged over all the clusters. The random error (residual) for each cluster is the amount by which the intercept for that cluster regression line differs, in the vertical direction, from the overall mean intercept, α (Fig. 42.1a). The cluster residuals are assumed to follow a Normal distribution with zero mean and variance . Within each cluster, the residuals for the level 1 units are assumed to follow a Normal distribution with zero mean and the same variance, σ2. If the cluster sizes are similar, a simple approach to checking for Normality and constant variance of the residuals for both the level 1 units and clusters is to look for Normality in a histogram of the residuals, and to plot the residuals against the predicted values (see Chapter 28).
Figure 42.1 Two-level random effects linear regression models with a single covariate, x.

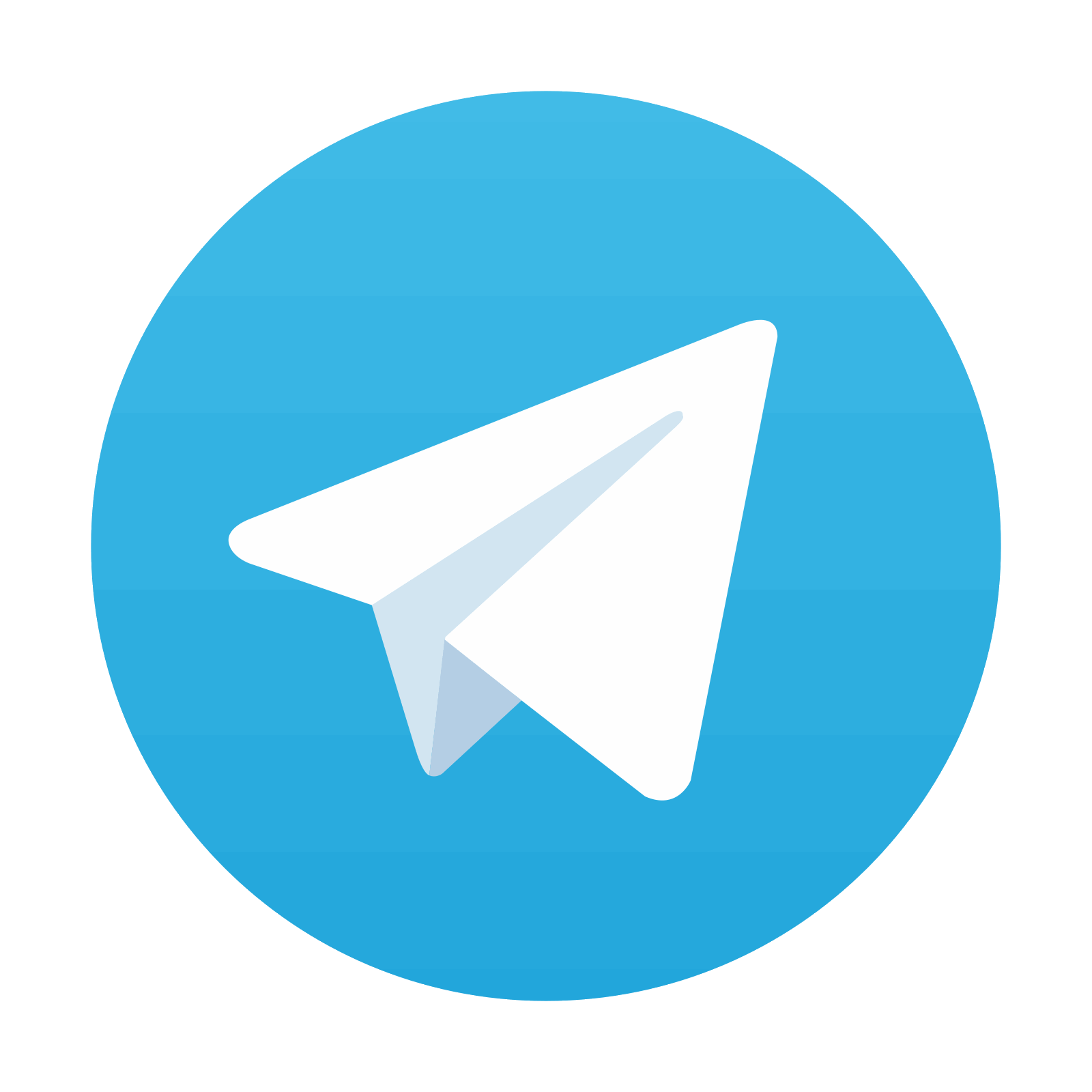
Stay updated, free articles. Join our Telegram channel

Full access? Get Clinical Tree
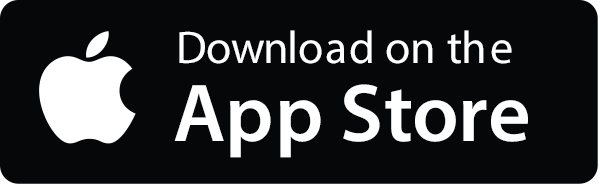
