Chapter Contents
Likelihood Ratios for Tests With Two Outcomes 96
Why Bother? 97
Shift Happens 98
Choosing Cut Points 98
Fagan Nomogram 99
Two-Step Fagan Nomogram 100
Nomogram Alternatives 100
Size Matters 100
Some Large Likelihood Ratios 102
Likelihood Ratios for Tests With Multiple Outcomes 102
The Importance of Accurate Pretest Probability 103
Diagnostic Thresholds 103
Limitations of Likelihood Ratios 104
Conclusion 105
Likelihood ratios can refine clinical diagnosis on the basis of signs and symptoms; however, they are grossly underused for patient care. A likelihood ratio is the percentage of ill people with a given test result divided by the percentage of well individuals with the same result. Ideally, abnormal test results should be much more frequent in ill individuals than in those who are well (high likelihood ratio), and normal test results should be more frequent in well people than in sick people (low likelihood ratio). Likelihood ratios near unity have little effect on decision making; by contrast, high or low ratios can shift (sometimes dramatically) the clinician’s estimate of the probability of disease. Likelihood ratios can be calculated not only for dichotomous (positive or negative) tests but also for tests with multiple levels of results, such as helical computerised tomography (CT) scans. When combined with an accurate clinical diagnosis, likelihood ratios from ancillary tests improve diagnostic accuracy in a synergistic manner.
Despite their usefulness in interpretation of clinical findings, laboratory tests, and imaging studies, likelihood ratios remain little used. Most doctors are unfamiliar with likelihood ratios, and few use them in practice. In a survey of 300 doctors in different specialties, only two (both internists) reported using likelihood ratios for test results. The underuse of likelihood ratios may reflect the dominance of therapeutic studies in the medical literature, as opposed to diagnostic studies. Because simple descriptions help clinicians to understand such ideas, we will try to make likelihood ratios both simple and clinically relevant. Our aim is to enhance clinicians’ familiarity with and use of likelihood ratios.
If everyone could be categorised as diseased or healthy, and if a dichotomous test for that disease were universally administered, then all seven billion of us will fit (albeit crowded) into one such table ( Fig. 9.1 ). Regrettably, neither life nor tests are so simple; grey zones abound. Likelihood ratios help clinicians to navigate these seas of uncertainty.

A likelihood ratio, as its name implies, is the likelihood of a given test result in a person with a disease compared with the likelihood of this result in a person without the disease. Stated alternatively, a likelihood ratio is simply the percentage of sick people with a given test result divided by the percentage of well individuals with the same result. Percentage and likelihood are used interchangeably here. The implications are clear: ill people should be much more likely to have an abnormal test result than healthy individuals, and vice versa . The size of this discrepancy has clinical importance.
Likelihood Ratios for Tests With Two Outcomes
The simple 2 × 2 table in the bottom of Fig. 9.1 shows the calculation for the likelihood ratio. In this example, 15 people are sick and 12 (80%) have a true-positive test for the disease. By contrast, 85 are well but five (6%) have a false-positive test. Thus the likelihood ratio for a positive test is simply the ratio of these two percentages (80%/6%), which is 13. Stated in another way, people with the disease are 13 times more likely to have a positive test than are those who are well. For a dichotomous test (positive or negative), this is called the positive likelihood ratio (abbreviated LR +). The flip side, the negative likelihood ratio (LR–), is calculated similarly. Three of 15 sick people (20%) have a false-negative test, whereas 80 of 85 healthy individuals (94%) have a true-negative test. So LR– is the ratio of these percentages (20%/94%), which is 0.2. Thus a negative test is one-fifth as likely in someone who is sick than in a well person. Panel 9.1 outlines three approaches to calculate likelihood ratios for dichotomous data.
If sensitivity and specificity have already been determined, then:
LR + is sensitivity/(1 – specificity)
LR– is (1 – sensitivity)/specificity
If raw numbers for the 2 × 2 table are available, then:
LR + is (a/[a + c])/(b/[b + d])
LR– is (c/[a + c])/(d/[b + d])
If mathematical formulas are unappealing, then:
LR + is the true-positive percentage divided by the false-positive percentage
LR– is the false-negative percentage divided by the true-negative percentage
The Prospective Investigation of Pulmonary Embolism Diagnosis III (PIOPED III) study examined the usefulness of gadolinium-enhanced magnetic resonance angiography in diagnosing this disease. A total of 371 patients were included. This imaging technique proved technically inadequate in a quarter of patients, which limits its clinical usefulness ( Panel 9.2 ). The reference standard incorporated clinical assessment, D-dimer tests, and other imaging studies.
For patients with adequate imaging studies ( Panel 9.3 ), the sensitivity was 0.78 and the specificity was 0.99. The distribution of results is presented, and the likelihood ratios are calculated in the right column. A positive test had a high LR+, useful for establishing the diagnosis. The LR− approached the threshold of 0.1.
Why Bother?
As most doctors are generally familiar with terms such as ‘sensitivity’ and ‘specificity’, is learning to use likelihood ratios worth the additional effort? Likelihood ratios have several attractive features that the traditional indices of test validity ( Chapter 8 ) do not share.
First, not all tests have dichotomous results. Formulae for test validity do not work when results are anything other than just positive or negative. Many tests in clinical medicine have continuous results (e.g., blood pressure) or multiple ordinal levels (fine-needle biopsy of breast masses).
Public-domain software will calculate indices of validity and likelihood ratios for 2 × 2 tables. For 2 × n tables, the software will calculate level-specific likelihood ratios, plot a receiver operating characteristic (ROC) curve, and determine the area under the curve ( http://www.openepi.com/DiagnosticTest/DiagnosticTest.htm , accessed 2 April 2017). Another useful calculator can be found at http://ebm-tools.knowledgetranslation.net/calculator/diagnostic/ (accessed 21 April 2017).
Likelihood ratios express the richness of test results and can influence patient management. Collapsing multiple categories into positive and negative sacrifices information. Likelihood ratios enable clinicians to interpret and use the full range of diagnostic test results instead of arbitrarily dichotomizing results. Although predictive values relate test characteristics to populations, likelihood ratios can be applied to a specific patient. Moreover, likelihood ratios, unlike traditional indices of validity, incorporate all four cells of a 2 × 2 table (see Panel 9.1 ).
Reliance on sensitivity and specificity frequently leads to exaggeration of the benefits of tests. In a comparison of two obstetrical tests (foetal fibronectin measurement to predict premature birth, and uterine artery Doppler wave-form analysis to predict pre-eclampsia), two-thirds of published reports overestimated the value of the tests. Use of likelihood ratios, rather than just sensitivity and specificity, might have prevented this misinterpretation.
Finally, and most important, likelihood ratios refine clinical judgement. Application of a likelihood ratio to a working diagnosis may change the diagnostic probability—sometimes radically.
When tests are done in sequence, the post-test odds of the first test become the pretest odds for the second test; one builds on the other. An example is sequential use of the D-dimer followed by a ventilation/perfusion scan or helical CT scan to diagnose pulmonary embolism.
Shift Happens
Likelihood ratios were traditionally thought to be invariable (i.e., unaffected by the prevalence of a disease). By their definitions, sensitivity, specificity, and likelihood ratios should not shift with different disease prevalences. However, numerous examples have now refuted this notion of invariability. This variability may reflect the patient spectrum involved, distorted inclusion of patients, verification bias, faulty reference standards, or other clinical issues.
A likelihood ratio derives from sensitivity and specificity, which are known to vary by disease prevalence, as discussed in Chapter 8 . Thus a likelihood ratio derived from a population with a low prevalence may not apply to one with a higher prevalence. Rather than being fixed values, sensitivity, specificity, and likelihood ratios reflect how a test performs in a specific population. Accordingly, clinicians should seek likelihood ratios derived from populations similar to their own.
Choosing Cut Points
ROC curves can help identify where cut points should be for continuous variables, like blood glucose, intraocular pressure, and blood pressure. An ROC curve ( Fig. 9.2 ) plots the true-positive rate (TPR) on the vertical axis and the false-positive rate (FPR) on the horizontal axis. For a worthless test, the plot is a diagonal line, and the area under the curve is 0.5. The closer the curve approaches the upper left-hand corner, the better is the test (and the greater is the area under the curve). In general, the best cut point is the point on the curve closest to the upper left-hand corner of the box.

ROC curve slopes have several useful features concerning their relationship to likelihood ratios. First, the slope (#1 in Fig. 9.2 ) of the tangent to any point on the curve is equal to the likelihood ratio of the test result for that point. Second, the slope (#2 in Fig. 9.2 ) between the origin (lower left-hand corner) and any point on the curve is the positive likelihood ratio when using that point as the threshold for test ‘positive’. Third, the slope (#3 in Fig. 9.2 ) between any two points on the curve ( x and y ) corresponds to the likelihood ratio for a test result using those two points to define an interval when multiple intervals are used.
Fagan Nomogram
Tests are not undertaken in a vacuum; a clinician always has an estimate (although usually not quantified) of the probability of a given disease before doing any test. According to Bayesian principles, the pretest odds of disease multiplied by the likelihood ratio gives the post-test odds of disease. For example, a pretest odds of 3/1 multiplied by a likelihood ratio of 2 would yield a post-test odds of 6/1. Unlike gamblers (or statisticians), most clinicians do not think in terms of odds; we usually use percentages. For example, a probability of 75% (75% yes/25% no) is the same as an odds of 3/1.
Although the conversion back and forth between odds and probabilities involves simple arithmetic, a widely used nomogram ( Fig. 9.3 A) skirts this step altogether. A nomogram is a graphical calculator that solves a specific equation; given two known values, the nomogram calculates a third value. A straight edge is placed on the pretest probability of disease (left column) and aligned with the likelihood ratio (middle column); the post-test probability (right column) can be read off this line. This procedure shows how much the test result has altered the pretest probability. For example, in the bottom of Fig. 9.1 , the likelihood ratio for a positive test was 13 and for a negative test, 0.2. Assume that the pretest probability of the hypothetical disease is 0.25 and that the test is positive. Placing a straight edge on a pretest probability of 0.25 and intercepting the likelihood ratio column at 13 yields a post-test probability of about 0.80, a large shift in diagnostic probability ( Fig. 9.3 B). This value is close to the post-test probability of 0.81 calculated with the Bayesian formula.
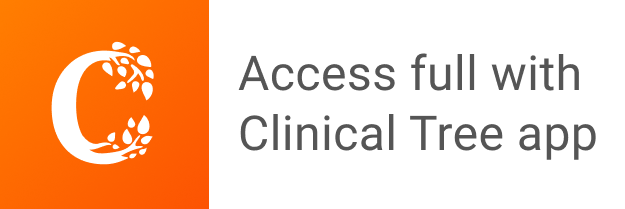