The over-arching goal of public health is to maximise the health of the population and for this we need evidence about what works and what doesn’t work. In Chapters 4, 6, 7 and 8 we looked at the different epidemiological study designs and examined the various misfortunes that can befall them. Good studies are difficult to design and implement, and interpretation of their results and conclusions is not always as straightforward as we might hope. How, then, can we make the best use of this information? In the next three chapters we will look at ways to identify, appraise, integrate and interpret the literature to generate the evidence we need to inform policy and practice. In this chapter we will focus on interpreting the results from a single study, while Chapter 10 will consider some of the issues involved when we try to decide if an observed association might be causal. Finally, Chapter 11 will look at how we conduct and interpret reviews and how we can bring all of this information together to make evidence-based recommendations.
The central question we have to answer when we read a study report is ‘Are the results of the study valid?’ If the authors report an association between exposure and outcome, is it real? If they find nothing, do we accept this? Or could there be an alternative explanation for the results, namely chance, bias and/or confounding? Then, if we think the results are valid, we should ask ‘so what?’ – are the results clinically or socially important? And ‘to whom do these results apply’ – can we assume that they will apply more generally than in that particular study population?
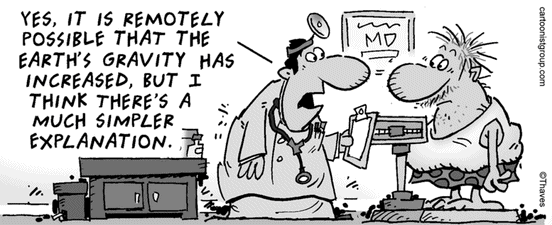
Much of the following discussion will pick up and integrate the core epidemiological issues covered in the previous chapters. We will concentrate mainly on analytic studies looking for associations between ‘cause’ and ‘effect’, the study designs that you met in Chapter 4, but the same general principles apply equally to descriptive epidemiology. To extract the maximum information from a paper we need a systematic approach to identifying its strengths and weaknesses. Some quite detailed sets of guidelines for ‘critical appraisal’ of the health literature exist already (e.g. see Box 9.2 on page 259) and we do not intend to add to this list (although we do offer a flowchart for more general guidance). Instead, we will focus on the essence of the challenge: what are the practical effects of the ways in which subjects were selected and information collected, and the likely influence of confounding and chance on the results we see? While the elements of the general strategy we propose are universal, the approach can (and should) be tailored to suit your own personal style. In practice you will almost certainly have to read individual papers and reports and, if you are involved in research, you may write some of your own. Both activities demand a very practical approach and this is what we will focus on here. We will emphasise the perspective of the reader, but the writer should be thinking about exactly the same things, because good writing demands that the readers’ needs and perspectives are kept firmly in mind.
It is obviously easier to assess the results of a study if they are presented in a clear and systematic way. A number of checklists have been developed to assist authors with this; we will discuss these under ‘Writing papers’.
The research question and study design
When reading a paper, the first step is to identify the research question that the authors set out to answer and then the strategy they used to attempt to answer that question. Was the study design appropriate to answer the question posed? This involves consideration of what the ideal type of study would be and also what would be practical in that particular situation.
As you have seen, the ideal study to answer a question of cause and effect would usually be some sort of randomised trial, as this is the best way to ensure that the groups we are comparing are exchangeable, but in many situations this will be impossible for numerous ethical and/or practical reasons. Next best would generally be a cohort study in which exposure is measured prior to the development of disease, but again the resources, time and money required to conduct a large enough study often make it unfeasible. So from a practical viewpoint, the key question should be ‘Was the research design the best that could have been done in the circumstances to answer that particular question?’ If it was not the best, can it still provide useful information? Are there other studies addressing the same issue that were of better design?
Many studies are not conducted to give a definitive answer to direct questions about causation, but because they can answer other more indirect questions of interest. For example, the results from the ecological study of Helicobacter pylori infection and stomach cancer rates in China shown in Figure 3.8 cannot directly answer the question ‘Does H. pylori infection cause stomach cancer?’, but they can answer the question ‘Are stomach cancer rates higher in areas where H. pylori infection is more common?’ If H. pylori infection does cause stomach cancer then we would expect this to be the case and evidence to this effect supports the hypothesis that the relation is causal. Although non-randomised studies provide more circumstantial evidence than RCTs, if the results are valid each can increase our understanding of the relation between an exposure and outcome. As an example, ecological and migrant studies conducted across countries with widely differing levels of solar ultraviolet (UV) radiation have consistently revealed an association between sun exposure in childhood and melanoma rates. In contrast, case–control studies, which have generally been conducted within a single country or region with a narrow range of UV exposures, have not given consistent results (Whiteman et al., 2001). In this particular situation ecological studies with their wide variety of exposure levels provide a valuable addition to the case–control studies.
Internal validity
Internal validity is the extent to which the results of a study reflect the true situation in the study sample. So how do we decide whether the results of a study are internally valid? We have to consider the three main alternative explanations that we discussed in the preceding chapters: chance, bias (both the selection of participants for the study and the information that was measured or collected from or about them) and confounding.
The study sample: selection bias
Who was included in the study, how were they selected and are there possible sources of selection bias? Specific questions to ask when reading a paper include those below.
Is the comparison group appropriate?
In a case–control study are the controls really representative of the population from which the cases arose? In a cohort study where the comparison cohort was recruited separately from the exposed cohort, are the two groups really comparable (i.e. are they exchangeable)?
What proportion of eligible participants actually took part in the study and, if appropriate, what proportion was lost to follow-up?
Low participation or follow-up rates may be cause for some concern. If the rates are lower than 80% or 90%, could participation (or loss to follow-up) be related to either the exposure or the outcome of interest? That is, could those who refused to take part (or who were lost to follow-up) have differed in some way from those who did take part? If so, might this have led to an overestimation or underestimation of the level of exposure and/or outcome? Most importantly, could this have differed between study groups?
As you saw in Chapter 7, high participation rates are very important for cross-sectional and case–control studies, but high follow-up rates are more important in cohort studies and trials.
Finally, what is the likely effect of any selection bias on the results of the study?
Ideally, the authors of the paper will have considered all of these issues in their discussion, but if they have not then it is up to the reader to decide whether bias might be present and, if so, what effect it may have had on the results. In practice there will almost certainly be some potential for selection bias. Participation rates are never 100% and in many developed countries it is becoming increasingly hard to persuade people to take part in research, especially when they see no benefit to themselves. This is a major issue in case–control studies when the motivation for a ‘case’ to take part may be much greater than that of an unaffected ‘control’. Also, people are becoming increasingly mobile, so follow-up in a cohort study that runs for more than a few years is never likely to be 100%. However, remember that selection bias will only affect the validity of the results if, in a case–control or cross-sectional study, the likelihood that someone agrees to take part differs by both case–control and exposure status or, in a cohort study, the likelihood of someone being lost to follow is related to both their exposure and their probability of developing the disease of interest.
If we were to reject all studies with less than 100% participation or follow-up rates, we would be left with nothing to review. In practice, participation or follow-up rates greater than 80% or 90% are generally considered to be good, but rates lower than this do not necessarily invalidate the findings (see Example 2 below). This is especially true for cohort studies and trials where low participation rates are less of a problem as long as the follow-up rate is high. The challenge for both investigator and reader is to think practically and to decide whether any potential biases related to selection might have compromised the study results (the internal validity) and, if so, how and to what degree the results might be biased. It is often impossible to quantify this, but sensitivity analyses making various assumptions about the size and direction of possible bias can be informative (see Chapter 7).
Example 1: case–control studies of blood transfusion and Creutzfeldt–Jakob disease
In five case–control studies of Creutzfeldt–Jakob disease (CJD) the controls were more likely to report having had a blood transfusion than cases (Riggs et al., 2001). Does this tell us that blood transfusions might protect against CJD (a finding contrary to the causal hypothesis)? If we consider the control groups, we find that in three of the five studies they were selected from among hospitalised patients and in another study more than 12,000 telephone calls were made in order to recruit just 784 controls.
People who are in hospital are more likely to have had a blood transfusion than those who are not; in addition, given the publicity surrounding ‘mad cow disease’, people who have had a blood transfusion may well have been more likely to agree to take part in a study of CJD. Indeed, in these four studies approximately 20% of controls reported having had a blood transfusion – an improbably high proportion, probably due at least in part to these selection pressures. So what can we conclude about the association between transfusion and CJD from these studies? Not much. The high transfusion rate in controls almost certainly overestimates the base rate in the population from which the cases came. Unless we have some knowledge of how common transfusion really is in the population, we have no idea whether the true background rate is similar to that in cases (i.e. there is no association) or lower than in cases (i.e. there is a positive association). Our next example shows how external information was used to help resolve such a dilemma.
Example 2: a case–control study of oesophageal cancer and smoking in Australia
In an Australian case–control study of oesophageal cancer, the authors considered the relation with smoking. In this study approximately 70% of eligible cases but only 49% of the controls who were contacted agreed to participate – this is a fairly typical response rate in many countries these days, but far from ideal. The authors found that current smoking rates were higher among cases with oesophageal adenocarcinoma than controls (OR compared to never smokers = 2.7; 95% CI 1.9–3.9), but could this be due to selection bias?
In general, smokers are less likely to agree to take part in a study than non-smokers. What effect might this have had on the odds ratio?
If smokers were less likely to take part the prevalence of smoking in the control group would be lower than that in the general population. This would exaggerate the difference between cases and controls and so increase the odds ratio, making it look as if smoking is associated with oesophageal adenocarcinoma when in reality it might not be. To address this issue the authors used data from a National Health Survey conducted at about the same time. If they assumed that the whole control population had a smoking rate equal to that seen in the national survey, they found that the odds ratio for the association between smoking and oesophageal adenocarcinoma was slightly weaker but still significantly greater than 1.0 (imputed OR = 2.4; 95% CI 1.7–3.4). This suggested that even though only about half of the controls invited to take part in the study actually agreed to participate, the overall results for the association with smoking were not seriously biased (Pandeya et al., 2009).
More about low participation rates: Investigators compared prevalence estimates and ORs for the association between selected exposures and health conditions calculated using baseline data from a cohort study with a participation rate of only 18%, with those from a population survey with a 60% response rate. The results suggested that any bias due to the low response rate in the study was minimal (Mealing et al., 2010).
Measuring disease and exposure: measurement bias
We also have to consider the information collected from or about the people in the study – particularly the measurement of ‘outcome’ and ‘exposure’ but also measurement of other factors that might be important confounders. Attention to unbiased measurement of outcome is crucial for cross-sectional, cohort and intervention studies. It is of relatively less importance in a case–control study, in which cases are selected because they have already experienced the outcome of interest (although a clear definition of what constitutes a case is still essential). Accurate measurement of exposure is important in every study, and in a case–control study it is critical to ensure that there are no systematic differences in measurement between cases and controls. Good measurement of confounders is often overlooked, but this is essential to enable optimal control of confounding in the analysis (see comments on residual confounding in Chapter 8).
Some questions to ask when reading a paper are the following.
Have all relevant outcomes and/or exposures and/or confounders been included and, if not, how important are those omitted?
Were the outcome/exposures/confounders clearly defined, and how were they measured?
Were the same definitions and methods of measurement used in all of the study groups?
Is measurement error likely to be a problem and, if so, could there be non-differential misclassification?
No measurement is perfect and some measurements are very poor. The effect of the ubiquitous random error and consequent non-differential misclassification must always be considered. The practical implication of this is that effects (OR, RR) estimated in the face of equal measurement error in the compared groups will usually appear weaker than they truly are, e.g. if the observed OR is 1.8 then, in all probability, the real association is even stronger, i.e. >1.8. Thus a finding of a positive association, despite poor measurement, should not be dismissed because of this – the true association is likely to be more impressive. On the other hand, a null finding or a very weak effect in the presence of non-differential misclassification is uninformative because it may reflect the imprecise measurement (thereby masking a true association) or there may truly be no effect. (Note that non-differential misclassification is unlikely to make it appear that an association exists when in reality there is none.)
Non-differential misclassification is particularly problematic in dietary studies because measurement of diet is very challenging and, as a result, misclassification is likely to be high. Furthermore the real effects are likely to be small.
If so, is it possible to predict what the differences might have been? For example, are cases more or less likely to have over-reported exposure? If cases overestimate their exposure then the OR is likely to be biased upwards, conversely if they underestimate their exposure (or controls overestimate theirs) then the bias is likely to be downwards. Could the observed association be due to misclassification? Or might the real association be stronger than that observed? Differential misclassification can bias results in either direction, it can make an association appear where there is none, it can make it seem that there is no association when in reality there is one and it can even make a positive association look like an inverse association, and vice versa. It is particularly important to consider this possibility in cross-sectional and case–control studies when exposure is measured after the outcome has occurred. In analytic research it is generally easier to distinguish clearly between outcome states (diseased versus non-diseased) than it is to measure exposures precisely, but the avoidance of differential outcome assessment is central to the integrity of cohort studies and trials, and again for cross-sectional studies.
Finally, what practical effects might any measurement bias (outcome or exposure) have had on the results of the study?
Example 3: a case–control study of body mass index (BMI) and asthma in Mexico
A significant association between asthma and obesity1 based on self-reported weight and height was observed among women (adjusted OR = 1.7; 95% CI 1.1–2.7), with a weaker non-significant association (adjusted OR = 1.3; 95% CI 0.6–2.9) among men (Santillan and Camargo, 2003); but how reliable are self-reported data on body size, and could measurement error have affected the results? The authors specifically addressed this question by weighing and measuring all of the participants. They found that, on average, people tended to report that they were taller and lighter than they really were, particularly the men. As a result, the true prevalence of obesity based on measured BMI was higher than that based on self-reported BMI and the difference was somewhat greater for cases (40% versus 24% for men and 44% versus 38% for women) than for controls (28% versus 22% for men; 24% versus 23% for women).
Is the error in the self-reported information on body-size differential or non-differential?
Assuming that the measured BMI values are correct, is the true association between obesity and asthma likely to be stronger or weaker than that seen for self-reported obesity?
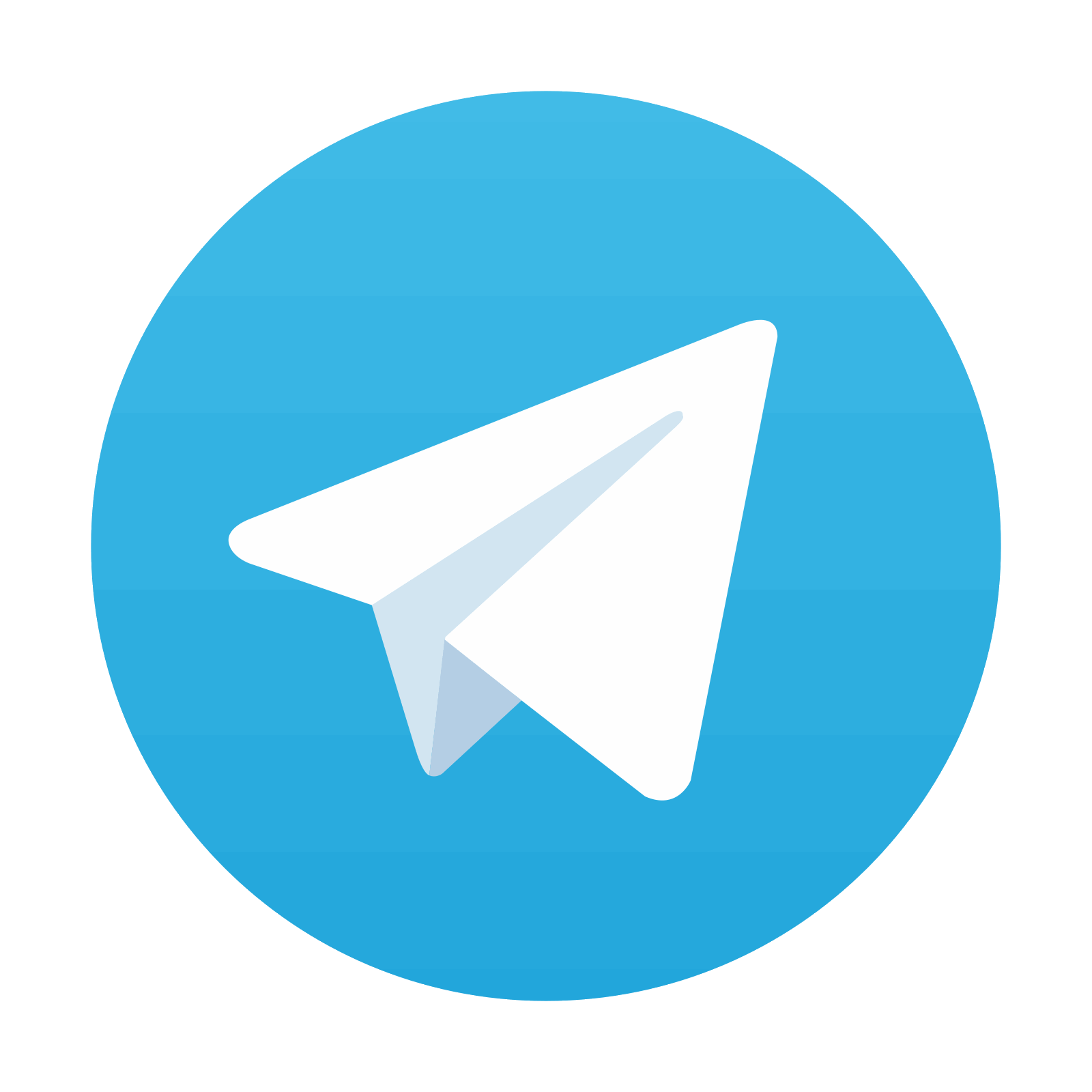
Stay updated, free articles. Join our Telegram channel

Full access? Get Clinical Tree
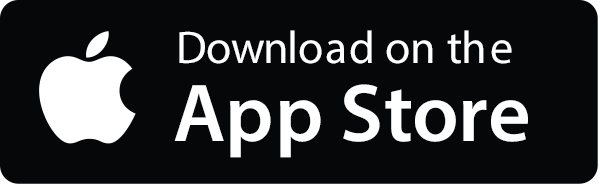

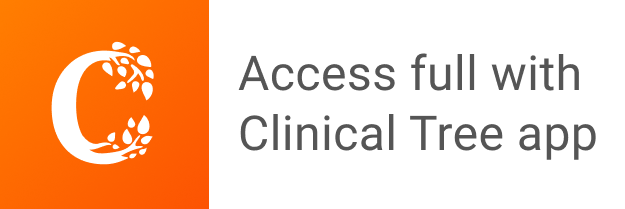