23 Quantitative data from microscopic specimens
Traditional approaches
Stereology is a technique developed for analysis of metals and minerals, where generally the properties being measured relate to number, size, and distribution of some particle in the sample. It is based on geometry and probability theory and, using statistical mathematics, stereology makes specific assumptions about the object being analyzed. A general discussion of the theoretical basis of stereology can be found in DeHoff and Rhines (1968), and in Underwood (1970). Stereological techniques have been applied to many biological images, both light and electron microscopical. General principles and applications can be found in Weibel (1979, 1980, 1990), in Elias and Hyde (1983), and in Elias et al. (1978). Although there is a long history of use of stereology in histology and histopathology, the use of this technique makes assumptions about the specimen that may not be applicable. Since the foundation of stereology is statistical, the general nature of the distribution of whatever is being measured should be describable using some statistic. This condition may be met under specific conditions, such as examining the distribution of chromatin ‘clumps’ within a cell nucleus, where the only object being examined is a single nucleus. For highly ordered structures, such as gland elements within an organ, the organization of the structure implies that there is no statistical distribution. Stereology can make estimates of some parameters of specimens, such as area of a total image occupied by some particular component. Note that this is an estimate. The use of stereology to derive measures of the three-dimensional structure of cell and tissue specimens may provide misleading information, since the probabilities used in the mathematics assume that the entire volume of the specimen is accurately reflected in the portion measured. Due to the polarization of cell organelles, and the arrangement of tissues and organs, this is generally not the case.
Electronic light microscopy
An electronic measurement of light transmitted through microscopic specimens has a long history, and roughly parallels the development of photometers, spectrophotometers, and light-detecting devices. Until recently (1980s), these devices simply detected light, and did not produce images. To use these early devices to produce images, the portion of the specimen visible to the light detector had to be restricted, and the specimen or image moved across this restricted area to generate an actual image. Many mechanisms were developed to acquire images using such techniques (Wied 1966; Wied & Bahr 1970). These mechanisms tended to be expensive, since they required high precision, and were also slow, as the image had to be acquired a small area at a time, and then ‘put together’ or reconstructed into a recognizable image. The majority of literature relating to quantitative light microscopy which utilized electronic measurement of light therefore was actually related to photometric and spectrophotometric studies, rather than analysis of images as currently defined.
Microscope photometry
A detailed theoretical account of microscope photometry can be found in Piller (1977). The hardware systems for obtaining data described in Piller have been superseded by modern devices, but the theoretical foundations are sound. The fundamental requirement for photometry is described by the Beer-Lambert law:
Recognition of distributional error was the reason that quantitative microscopes employed various devices to permit restricting the area of the specimen seen by the detector to a small area. This strategy was successful because of the optics of the microscope itself. A given lens resolution is defined as the ability to separate two points (point resolution). If a lens cannot separate two points, then they look like a single object. If a light detector sees an area of the specimen that is smaller than the point resolution of the microscope lens combination in use, then, by definition, that area is homogeneous, since the lens cannot see any structure in that area. For microscope lenses of 40x magnification and above, general ‘area examined’ sizes for photometry range from 0.25 to 0.5 µm diameter spots. Such spot sizes generally avoid significant distributional error. When such spots are generated by mechanical ‘stops’ or pinholes in the optical path of the microscope, they may introduce other, significant, sources of error, such as edge diffraction. These sources of error, as well as many others, such as glare within the optical system, are discussed in detail in Piller (1977). The above discussion applies to absorbing images only: that is, microscope images obtained with transmitted light microscopy. Fluorescence emission microscopy and particle reflectance (autoradiography) are based on different physical principles, and require different optics and sensor configurations.
Image acquisition
Solid-state cameras, whether CCD or CMOS, are available in either monochrome or color versions. Color cameras may use two different techniques to generate a color image. In one approach, there are three separate detector arrays, each with a color filter in front of the array. A prism or mirror system is used to split the image coming from the microscope into three separate but identical images, so each detector sees the same image. The color filters are red, green, and blue, since a red image, a green image, and a blue image can be combined to create a full color image. This type of camera is called a three-chip color camera. The second approach to color cameras uses a single detector chip, and places a pattern of color dots over the individual pixels. Again, these color dots are red, blue, and green. The most common pattern for these dots is the Bayer pattern (Fig. 23.1). Note that in the Bayer pattern there are actually four dots per ‘repeat’, since for each red and each blue dot there are two green dots. This type of camera is called a single-chip color camera.
Solid-state cameras used on microscopes are generally coupled to the microscope in a manner to optimize the area of the visible field (to a user looking through the eyepieces of the microscope) that is captured. As is true of photographic cameras, the solid-state camera captures a rectangular (or in a few cases, square) portion of the circular image displayed in the microscope. The camera sensor pixels are, as previously described, quite uniform in response to light intensity. The nature of microscope lens systems, even in those that have been carefully aligned, is a higher intensity along the optical axis (center of the image) than in the periphery of the field. For an adaptive sensor such as the eye, and one with a limited number of intensity step discriminations, the field of view of a carefully aligned microscope appears to be quite uniform. That this is not the case is amply illustrated in many lectures that display photomicrographs, where a common flaw is dark corners. In the case of solid-state cameras, the increased intensity level sensitivity, as compared to the eye, accentuates this problem. Since all solid-state cameras use some type of software, either within the capture system or on the capturing computer to control the camera, this software frequently contains some type of ‘field flattening’ or ‘background subtraction’ mechanism to correct this variation in intensity from center to edge of the image. Additional details of requirements for acquiring images through microscopes using electronic cameras can be found in Shotton (1993).
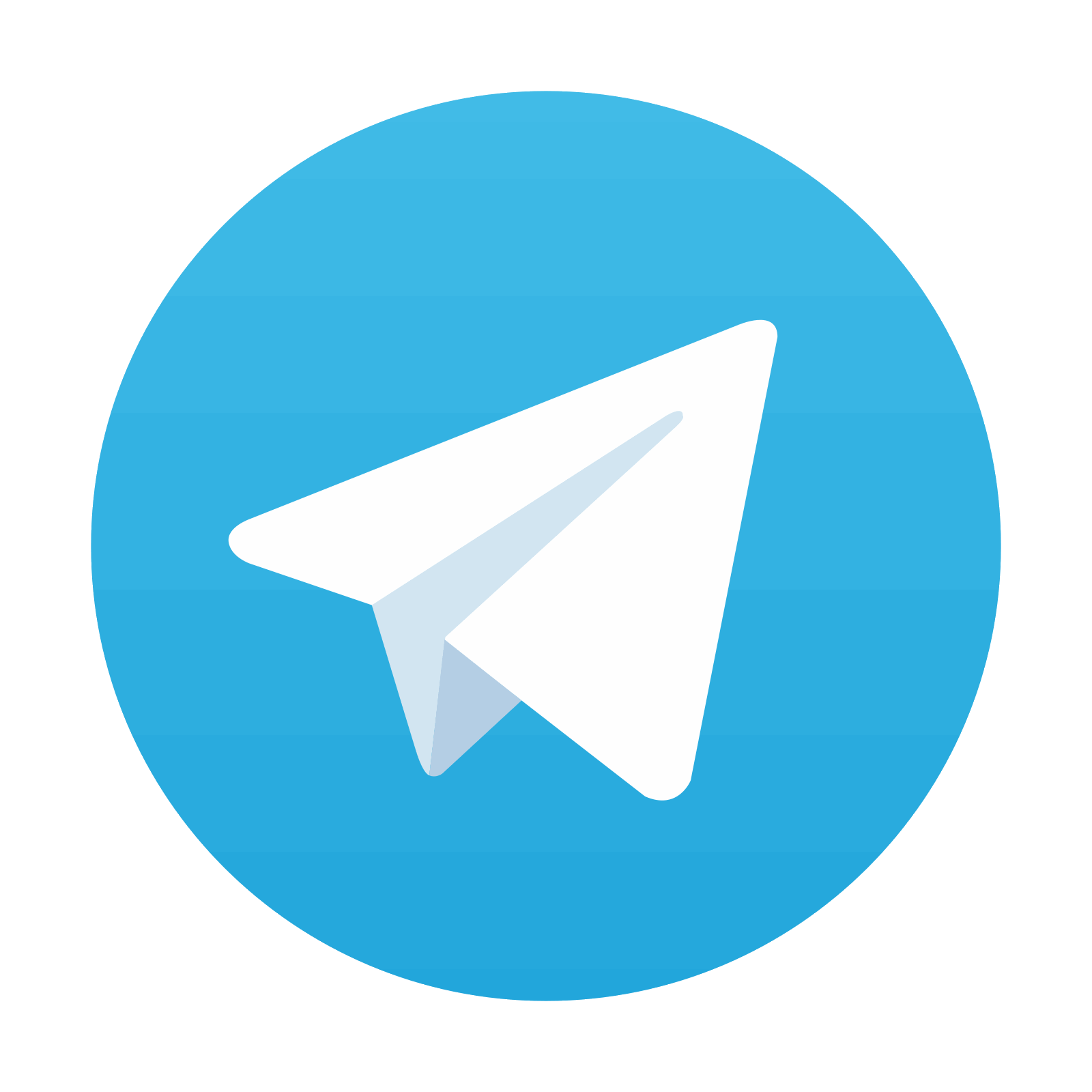
Stay updated, free articles. Join our Telegram channel

Full access? Get Clinical Tree
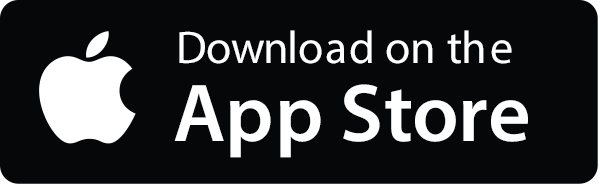
