Fig. 5.1
(a) Far-UV CD spectra of protein 1–4 (spectral similarity compared to that of protein 1 is 100% protein 1; 5.0% protein 2; 44.9% protein 3; 28.7% protein 4). (b) Near-UV CD spectra of protein 1–4 (spectral similarity compared to that of protein 1 is 100% protein 1; 41.0% protein 2; 3.1% protein 3; 12.9% protein 4)
The results from Li et al.’s qualification study indicate that CD spectroscopy can be qualified for characterizing protein secondary and tertiary structures and for comparability studies of biopharmaceuticals. With proper calibration of the instruments and cuvettes, and standardized good operating practices, CD spectroscopy is quite reproducible and able to detect changes induced in the secondary and tertiary structure of a protein by lower pH and other possible denaturing reagents that are present during therapeutic protein manufacturing processes. The sensitivity of the technique to detect the changes in conformation that might be induced by the manufacturing process is dependent on the experimental variability and the nature and extent of the structural changes in the particular protein being analyzed.
The results shown above provide the ground work on how the CD method should be calibrated and qualified and demonstrate that the method is sufficiently precise to monitor protein structure changes beyond the variability of the method.
5.3 Qualification of FTIR
Fourier transform infrared (FTIR) spectroscopy can be used to obtain information about the higher-order structure of a protein both in solution and in the solid state (Carpenter et al. 1998; Fu et al. 1999; Haris and Chapman 1995; Kong and Yu 2007). It is routinely employed as a characterization method in the biopharmaceutical industry to determine the higher-order structure of protein therapeutics (Gross and Zeppezauer 2010). It is often included as part of the biophysical characterization methods to assess the secondary structure of protein therapeutics before and after changes to process, formulation, manufacturing, and storage conditions, and the data are included in regulatory filings (Jiang and Narhi 2006; Jiang et al. 2008). However, the qualification of the FTIR method for protein secondary structure analysis has mostly remained elusive, mainly due to the lack of a consistent method applied to quantitatively determine the similarity of the FTIR spectra and hence the comparability of the protein samples analyzed. Visual comparisons of a sample spectrum to a reference have typically been used as a way to verify proper folding of a protein sample. To objectively qualify the FTIR method, a mathematical algorithm is needed to compare the FTIR spectra quantitatively and demonstrate the precision and sensitivity of the method for protein secondary structure analysis.
Work has been done to quantify the percentages of different secondary structural components for proteins in solution by FTIR (Vonhoffa et al. 2010; Susi and Byler 1987). There are two primary ways of doing this. The first uses curve fitting of the second-derivative or self-deconvoluted spectra to obtain the relative amounts of different types of secondary structure based on the band areas (Susi and Byler 1986; Byler and Susi 1986). The second involves peak fitting of the non-deconvolved and baseline-corrected amide I bands and then obtaining the percentage of secondary structures by correlating with the shape and intensity using an interval partial least squares algorithm (Vedantham et al. 2000; Surewicz and Mantsch 1988). These methods are helpful in determining the major secondary structure components and estimating their percentages in a protein. However, these methods are not optimized for assessing overall similarity of protein structure between samples from different processes or formulations. The quantification of the secondary structure composition can vary depending on the methods and parameters used, and it involves considerable mathematical manipulations including spectra manipulation, curve fitting, area integration, and normalization. Therefore, they are not routinely carried out for protein secondary structure assessment and qualification of FTIR by analysts in the biopharmaceutical industry.
One other mathematical approach explored by Prestrelski et al. (1993) is to compare the FTIR spectra quantitatively by using the correlation coefficient function. Occasionally, the correlation coefficient value of the uncorrected spectra did not agree with a visual assessment of the spectral similarity due to an offset in baselines which led to an artificially low value. Conversely, if the spectra were baseline corrected and peak positions were similar, but there were differences in relative peak heights, the correlation value would be unreasonably high. To avoid this inconsistency, Kendrick has developed a method to quantify the overlap of second-derivative FTIR spectra to determine structural similarity between proteins (Kendrick et al. 1996). In this approach area-normalized second-derivative spectra were used and compared. The authors found that quantifying the area of overlap between area-normalized spectra provides a reliable, objective method to compare overall spectral similarity and avoids the problems associated with calculation of the correlation coefficient. However, due to normalization of the spectra, the area of overlap approach cannot distinguish between true differences in the magnitude of FTIR signals. Recently, D’antonio et al. 2012 showed a modified area of overlap method that improved the differentiation power for quantitative comparison of FTIR spectra. The authors also compared four different algorithms including the correlation coefficient and area of overlap and summarized their strength and weakness for studying comparability of protein therapeutics.
A new approach using Thermo Electron OMNIC software QC compare function (Cover and Hart 1967; TQ Analyst Algorithm 2007–2010) has been applied to directly compare FTIR spectra with minimal mathematical manipulation (Fig. 5.2), which led to the identification of important performance characteristics for the qualification of the FTIR method (Jiang et al. 2011). The QC compare function was originally intended and used as a library searching tool for small-molecule identification by FTIR (TQ Analyst Algorithm 2007–2010). It correlates the spectral information in the specified region of the sample and the reference spectra to determine the similarity between the two. The results of the method are reported as a value between 0% and 100%, which indicates how well the sample spectrum matches the reference spectrum. A spectral similarity value of 100% indicates the two spectra are identical.
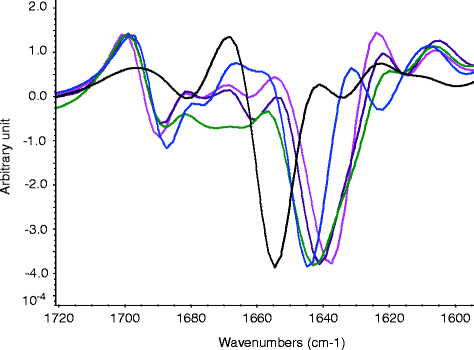
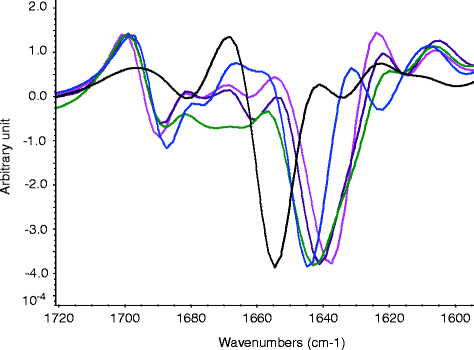
Fig. 5.2
Second-derivative FTIR spectra of protein 1–5 (spectral similarity compared to that of protein 1 is 100% protein 1 (pink); 46.3% protein 2 (purple); 36.3% protein 3 (green); 9.7% protein 4 (blue); 12.1% protein 5 (black))
The selection of an appropriate approach to numerically compare the spectra should depend on the capability of the method, the ease of access and use of the method, and the purpose of the study.
With the OMNIC QC compare algorithm, Jiang and colleagues evaluated the precision and sensitivity of FTIR for the analysis of protein secondary structure through a multisite/instrument and multi-analyst study in an effort to qualify the method (Jiang et al. 2011). Proteins containing different types of secondary structures such as alpha-helical and beta-sheet were included to ensure that the precision assessment would apply to secondary structure analysis of all proteins regardless of the specific structural type. In addition inter-day repeatability and the effect of protein concentration differences on the precision of the method were also evaluated. The overall FTIR spectral similarities of these analyses on the same proteins were compared. The sensitivity of the method was evaluated by comparing the reference spectrum of a protein to that of the partially or fully unfolded protein after exposure to the denaturing condition and also by blending studies where the spectrum of a reference was mixed with that of a denatured protein. Standard curves were generated where the spectral similarity was plotted as a function of the percentage of the unfolded protein.
Results by Jiang et al. (2011) demonstrate that the FTIR method for the analysis and characterization of protein secondary structure is precise with the standard deviations of the characteristic FTIR band frequencies <1.1 cm−1 and the spectral similarity >93% for replicate measurements. The FTIR spectra remain the same whether collected on the same day or during a three-day period and when the protein concentration deviates from the target concentration (≥30 mg/mL) by ≤10% regardless of the maker of the instrument used or the structural family of the protein. The authors also demonstrate that the method is sensitive and appropriate for assessing protein secondary structure and changes in conformation resulting from stresses that can be encountered during common biopharmaceutical manufacturing processes. The sensitivity of the FTIR method is dependent on the extent of the structural changes induced and the magnitude of the resulting changes in the spectra.
The precision of the FTIR method is closely related to the signal-to-noise ratio of the instrument, the sample concentration, the buffer components, and the spectral region of interest. The sensitivity of the method to detect structural change depends on the nature of the sample and relative spectral changes corresponding to the structure changes. Therefore, protein-specific qualification/verification of the method may need to be performed to ensure the understanding of precision and sensitivity of the method.
5.4 Qualification of DSC
DSC has been used widely to study thermal dynamics of protein folding. The melting/thermal transition temperature(s) of proteins can be used to understand the energy barrier for protein unfolding and thermal stability of proteins (Pyrpassopoulos et al. 2006; Johnson et al. 1995). DSC has also been used to study the binding interactions of proteins with other molecules and the effect of mutations and the presence of carbohydrates on protein thermal stability (Celej et al. 2006; Bruylants et al. 2005; Johnston et al. 2011; Protasevich et al. 2010; Wen et al. 2008). The application of DSC has been reviewed extensively in the area of characterization of macromolecules and their interactions during pharmaceutical product development (Chiu and Prenner 2011; Bruylants et al. 2005). However, even though the method has been used extensively to monitor and show changes in protein thermodynamic parameters such as enthalpy and melting temperature (T m), there are very limited published data on the systematic qualification of the method to show that the DSC measurements are precise, accurate, and sensitive for the analysis of protein conformation and thermal stability. A protocol on measuring protein thermostability by DSC was published by Makhatadze (Coligan et al. 2001). It provides a detailed procedure for conducting DSC analysis including sample preparation and interpretation of the results, as well as calibration of the instrument and maintenance of DSC cells. Calibration of DSC was described by Gmelin and Sarge (Gmelin and Sarge 1995). They provided recommendations for instrument-independent calibration of temperature, heat, heat flow rate of the scanning calorimeter, operation procedures, calibration substance, and data treatment algorithms which can be used for all instruments.
In the early days of DSC analysis, various data analytical algorithms were developed and used to assess the effect of instrument configuration and scan rate on DSC measurement results (Lopez and Freire 1987). During the use of DSC for purity analysis of drug product, instrumental differences, sample size, heating rate, and details of the calculation of such analysis were examined (Yoshii 1997). It was found that changing the sample size or heating rate resulted in a difference in the effect of purity value between two instruments. Collectively controlling the heating rate during DSC measurement appears to be critical in obtaining reproducible results.
The precision of DSC measurements across different instruments and labs using the same sample (polymeric material) and parameters has been assessed. The study was organized by Empa (Swiss Federal Institute for materials testing and research) (Schmid 2012; Affolter et al. 2001). It collected measured data on glass transition (T g) and melting point (T m) and evaluated those using statistical methods to assess the precision of DSC. The results show that variability of DSC measurements is mainly caused by differences in the analyst, instrument, and calibration of the instrument. For one-point temperature measurements such as T g and T m, good agreements were observed with a standard deviation of repeatability at 0.3–1.0°C and that of reproducibility at 1.0–2.1°C. The standard deviation increases significantly with user-defined data evaluation.
A recent paper by Wen et al. (2011) explored the qualification of DSC for its applications in thermal stability analysis of proteins. The authors assessed the precision and sensitivity of the DSC method through a multisite, instrument, and analyst study using several proteins from different structural families including monoclonal antibodies and cytokines and parameters including T m and profile similarity. The same experimental parameters such as heating/scan rate and similar protein concentrations were used for measurement on all instruments with the exception of one. The results show that the T m values obtained for the same protein by the same or different instruments and/or analysts are quite reproducible, varying generally <1.1°C with the same instrument and <1.5°C across different instruments. The profile similarity values obtained using the OMNIC QC compare function (discussed above in the CD and FTIR sections) for the same protein from the same instrument are also high (> ~95%). The variability of inter-day DSC measurements is very similar to that of the intra-day measurements (only slightly higher by ~0.1°C). The sensitivity of the DSC method for assessing protein thermal stability and conformational changes was evaluated by several experiments including analyzing samples perturbed by pH 3 or 6 M guanidine HCl (Gdn). The results show that DSC is able to detect changes in protein conformation caused by low pH and denaturant to levels as low as 10% denatured protein present in the sample. The authors then applied DSC to the analysis of pH stability and buffer screening of a protein and candidate screening. They demonstrated that DSC is an appropriate method for assessing protein thermal stability and conformational changes that may result from manufacturing processes, formulation, and storage conditions and can also be used to compare relative stability of candidate molecules.
Validation of DSC for pharmaceutical analysis under cGMP was described by Weissburg et al. (2002). The validation included development of a validation plan (i.e., scope of validation, criterion for completion of validation), design documents, user requirements, calibration and maintenance procedures, IQ/OQ, standard operation procedures, and test scripts. The authors provided details about validation of the computer-controlled system to be 21CFR Part 11 compliant and of the thermal accuracy and precision using a NIST-certified indium standard at 0.5 and 50°C per minute scan rate employing the DSC testing script. Validation of DSC was achieved when the test results obtained met the specifications. The validation showed that the DSC instrument and the computer program and system used met 21 CFR Part 11, cGMP, and the user predefined requirements.
The cases shown above demonstrate that DSC can be qualified and validated for the purpose of assessing protein higher-order structure changes for regulatory filings. The qualification and/or validation should focus on demonstrating the precision of the method and that the method is suitable for its applications. When the instrument is properly calibrated and maintained, DSC is accurate, precise, and sufficiently sensitive to detect changes in proteins resulting from process and formulation changes and point mutations of the protein primary structure.
5.5 Qualification of SV-AUC
Sedimentation velocity analytical ultracentrifugation (SV-AUC) is used as a characterization tool in biotherapeutic development primarily to assess product purity; that is, it measures the relative abundance of size variants in a protein solution. Although nonroutine, it is commonly applied in product comparability studies, protein reference standard qualifications, product quality investigations, and other product characterization activities (Berkowitz 2006; Shire 1994).
The SV-AUC analysis is an orthogonal method to size-exclusion (SE) HPLC, and it is in this context that it is perhaps most useful, because it does not suffer from many of the potential limitations of SE-HPLC: physical disruption of the sample, extensive dilution into what is often a different solution environment, and lack of separation of product size variants close to the column exclusion limit (Gabrielson et al. 2007a; Philo 2006; Carpenter et al. 2010). Because it employs a fundamentally different mode of separation, SV-AUC can be used to verify the accuracy of SE-HPLC methods when they are developed and remediated and to confirm the suitability of SE-HPLC for routine use.
In addition to measuring the relative concentration of size variants, SV-AUC can be used to determine their mass and shape. The sedimentation boundary profiles provide information about the sedimentation and diffusion rates of size variants present in the sample (Dam et al. 2004; Laue et al. 1992; Lebowitz et al. 2002; Schuck et al. 2002). The sedimentation coefficient is directly measured from the time-dependent displacement of the boundaries. For a size variant of known mass, consistency of the measured sedimentation coefficient provides confirmation that the overall shape and charge of the size variant has been maintained when multiple samples are measured under the same solution conditions. However, the accuracy and precision of the measured sedimentation coefficient decreases as the concentration of the size variant decreases (Gabrielson et al. 2007b). In very pure samples with low concentrations of fragmented and aggregated size variants (<1% of the total protein mass), only the sedimentation coefficient of the most abundant species (typically monomer) can be reliably determined.
In the biopharmaceutical industry, SV-AUC is primarily used as an orthogonal method to SE-HPLC to quantitatively measure protein purity and secondarily used to measure protein monomer sedimentation coefficients (Gabrielson et al. 2010). Qualification of an SV-AUC method should reflect the fact that it will be used to quantitatively measure these two attributes. Furthermore, two performance characteristics of an SV-AUC method should be considered during qualification: (1) evaluation of the method’s precision and (2) confirmation that the method is sufficiently sensitive to provide quantitative results, thereby allowing meaningful conclusions to be drawn.
Assessing the precision of SV-AUC, and determining which type(s) of precision to evaluate, should be governed by how the method will be used. For example, if SV-AUC analysis can typically be completed by one analyst using one instrument for product characterization studies required during biotherapeutic development, then it may not be necessary to study the reproducibility of the method. However, if the number of samples requires multiple runs on different days, then understanding the intermediate precision of the method is critical.
Experimental factors that impact the precision of SV-AUC measurements have been reviewed elsewhere (Gabrielson et al. 2007b, 2010; Schuck et al. 2002; Gabrielson and Arthur 2011; Arthur et al. 2009; Pekar and Sukumar 2007) and include the instrument, rotor, centerpiece and other cell components, overall alignment of channels, temperature equilibration, analysis software and settings, and sample characteristics. Even under the tightest experimental control currently achievable, normal variation of these factors leads to variability in results of approximately 0.4% for size variant quantification (Gabrielson and Arthur 2011; Arthur et al. 2009) and about 0.02 S for sedimentation coefficient measurements (Gabrielson and coworkers, personal observation, unpublished data). Much of the imprecision arises from the specific centerpieces used (Arthur et al. 2009; Pekar and Sukumar 2007) and from the alignment of cells (Pekar and Sukumar 2007), factors that can vary as much within a run as they do across runs or even across laboratories. A robust qualification design for an SV-AUC method should therefore account for normal variations from all relevant experimental factors, that is, those factors that reflect how the method will be applied during development of a biotherapeutic.
Studies to evaluate SV-AUC method performance indicate that performance parameters including linear range, limit of detection (LOD), limit of quantitation (LOQ), and measurement precision are, in general, independent of the specific protein product analyzed (Gabrielson et al. 2007b; Gabrielson and Arthur 2011; Arthur et al. 2009). Variability in measured results is primarily due to experimental factors, such as centerpiece differences, and depends to a lesser extent on sample characteristics. Therefore, after sufficient data have been acquired to account for relevant experimental factors, it is possible to qualify SV-AUC analysis of a new protein product by simply verifying that the precision of sedimentation coefficient measurements and the precision of size variant measurements are within a range expected from previous experience with the method. Additional qualification experiments may be warranted if the expected method performance is not achieved for a particular product or sample type, for example, protein samples with reversible self-association. In those cases, protein-specific intermediate precision should be more rigorously defined.
The ability of SV-AUC to detect changes to the product, both to its size distribution profile and to the sedimentation coefficient of the monomer, is essential for successful application of the method in biotherapeutic development. Therefore, method qualification should include experiments demonstrating the suitability of SV-AUC for detecting such changes. This may be accomplished by manipulating a sample to perturb the protein structure and to produce higher levels of size variants. A suitable amount of degradation can usually be obtained from thermal, UV, or pH stresses. For example, subjecting a monoclonal antibody sample to low pH conditions will often cause the sedimentation coefficient of the monomer to shift and induce formation of high molecular weight size variants (aggregates), which can be used to confirm the sensitivity of the method.
The sensitivity of the method can best be determined when changes to the measured output are correlated with changes deliberately made to the product. Changes to the sedimentation coefficient of a protein and the amount of aggregation measured by SV-AUC for a different protein, both induced by exposure to low pH, are shown in Fig. 5.3.
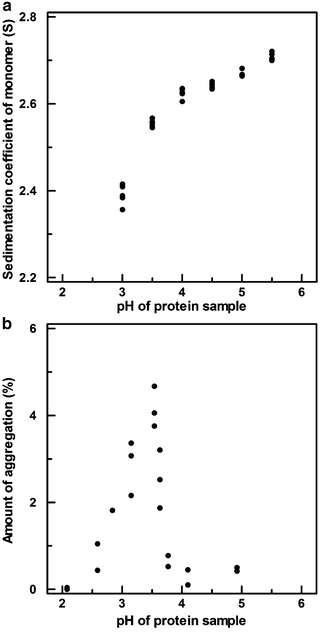
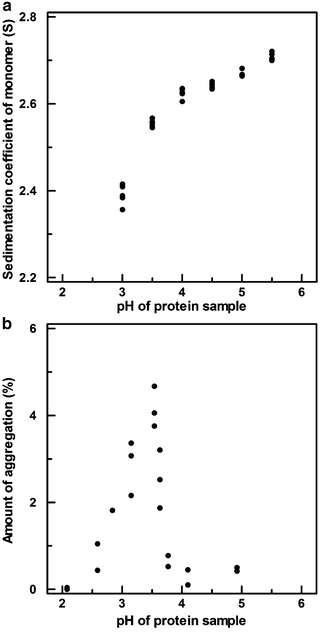
Fig. 5.3
Sedimentation coefficients (a) and aggregation levels (b) measured by SV-AUC for protein product samples across the pH range indicated
The data presented in Fig. 5.3 are instructive for several reasons. First, the response of the method to a given stress condition may or may not be linear. In panel A, the sedimentation coefficient responds nearly linearly to pH changes from 5.5 to 3.5 and then declines nonlinearly at more acidic pH, whereas in panel B the maximum aggregation levels are measured in a narrow pH range near 3.5; aggregation levels decline markedly at higher and lower pH. Second, it is often convenient to express the method sensitivity by normalizing the response, either by dividing by the range of the independent variable (i.e., by calculating the slope if the response is linear) or by dividing by an estimate of the variability of the method to express the change in response as a number of standard deviations. For example, in panel A of Fig. 5.3, a pH change from 5.5 to 3.5 results in a 0.2 Svedberg shift in the sedimentation coefficient. The sensitivity of the method to pH could then be expressed as 0.1 Svedberg per pH unit (or five standard deviations per pH unit when normalized by the method variability of 0.02 S). Finally, method sensitivity studies are most useful when they evaluate a range wider than what the product will likely encounter during manufacture, shipping, and long-term storage. This allows interpolation of the response, rather than extrapolation to conditions which have not been studied.
Any number of stress conditions can be used to demonstrate the sensitivity of SV-AUC. These may include thermal degradation, light/UV exposure, pH modification, chemical denaturation, or primary structural modifications (e.g., oxidation). Many considerations should inform the selection of product stress conditions with which to determine the sensitivity of the method, including the likelihood that the product will encounter a certain stress during manufacturing and the intended change to the product. That is, a general stress like elevated temperature may be desired to produce aggregation, whereas a more targeted stress like oxidation may be useful to correlate the sedimentation rate of the protein with changes to its primary structure. And finally, the intended purpose of the method should also be considered when selecting product stress conditions. A different stress condition may be desirable if the method will be used exclusively to characterize a drug substance manufacturing process compared to if the method will be used to evaluate drug product comparability.
5.6 Qualification of SE-HPLC-LS
During biotherapeutic development, it is often valuable to measure the molecular weights of size variants present in product samples. Molecular weight determination with sufficient accuracy facilitates identification of these size variants (such as dimer and larger aggregate species), which is required for comprehensive product characterization.
Addition of light scattering (LS) detection to a size separation method like SE-HPLC makes it possible to determine the molecular weights of size variants after elution from the column (Kendrick et al. 2001; Wen et al. 1996). Under some conditions, the LS detection is also more sensitive for large size variants than conventional detection (such as UV, RI) alone. Both of these LS capabilities arise from the fact that the intensity of the light scattered by an eluting species is proportional not only to its concentration but also to its molecular weight (Wen et al. 1996; Wyatt 1993). However, because the LS detector response is a function of two variables, molecular weight and concentration, neither can be calculated directly from the LS signal alone. The molecular weight of each eluting species can only be determined by using a separate measurement of protein concentration (i.e., a simultaneously collected UV or RI chromatogram) to deconvolute the molecular weight and concentration information for each peak.
LS analysis is commonly applied as a characterization tool in product comparability studies, characterization studies to support product licensure, and product quality investigations. For molecular weight measurements by LS, the accuracy of the measured result is the key method performance parameter that should be evaluated during method qualification. The precision of the method may be determined in a straightforward manner and is useful to include in the design of qualification experiments. Accounting for different columns and mobile phases in these experiments is critical if characterization studies might be performed over multiple days and analysis sequences. Nonetheless, it is the accuracy of the method, that is, how much the measured mass differs from the true mass, which dictates the usefulness of the method for product characterization. Therefore, the method should be qualified to demonstrate that it is sufficiently accurate for the intended purpose of determining the molecular weights of size variants separated by SE-HPLC (or another flow separation method such as field flow fractionation).
Comparison of the measured mass of a protein standard to its known mass is an important way to assess the accuracy of SE-HPLC-LS. This confirms that the system is capable of providing results with sufficient accuracy. Nevertheless, the accuracy of results for unknown species cannot be inferred from the accuracy of a protein standard because the measurement accuracy depends on factors which may be different for each protein and size variant, such as the extinction coefficient and concentration. Therefore, propagation of error analysis provides a convenient approach by which to assess the accuracy of the measured result for any protein size variant.
First, the signal and noise associated with each detector (LS, UV, and/or RI) can be determined directly from a chromatogram. If a multiangle LS detector is used, signal and noise estimates should be determined from the 90° detector. (For isotropic scatters, such as proteins, the 90° detector has the highest signal-to-noise ratio.) Each detector’s signal is taken to be the maximum signal (in volts) of the peak of interest. The noise for each detector is estimated from a region without protein signal (i.e., a baseline region) using any one of several noise calculation approaches such as the square root of the mean squared error.
The governing equations that relate the signals of each detector to the physical attributes of the protein are provided in Eqs. 5.1, 5.2, and 5.3 (Wen et al. 1996):
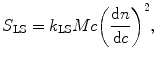
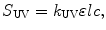
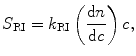
where k LS (V mol L mL−2), k UV (V), and k RI (V g mg−1) are detector proportionality constants and l (cm) is the path length of the UV cell, also a constant. The concentration, c (mg mL−1), of the size variant can be determined from either Eq. 5.2 or Eq. 5.3, depending on the choice of detector, when the extinction coefficient ε (mL mg−1 cm−1) and the refractive index increment dn/dc (mL g−1) are provided. The molecular weight of the size variant, M (g mol−1), is then determined from Eq. 5.1.
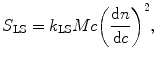
(5.1)
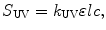
(5.2)
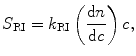
(5.3)
The error of the molecular weight measurement can be estimated by applying a propagation of error analysis to Eq. 5.1. The generalized form of the error propagation formula (Taylor 1997) is shown in Eq. 5.4 for a response, f, that is a function of independent variables, x, y, and z:
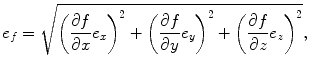
where e f is the expected error in the calculated value of f and e x , e y , and e z are error estimates for variables x, y, and z, respectively.
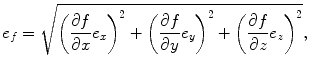
(5.4)
5.6.1 Case 1: LS-UV Detectors
When LS and UV detectors are connected in series, the size variant concentration is calculated from Eq. 5.2, which is then used to calculate the size variant molecular weight from Eq. 5.1. It is assumed that some degree of uncertainty exists in the protein extinction coefficient and refractive index increment, both of which are provided by the analyst. Propagation of error using Eq. 5.4 leads to the following expression for the expected error in the molecular weight calculation (Eq. 5.5):
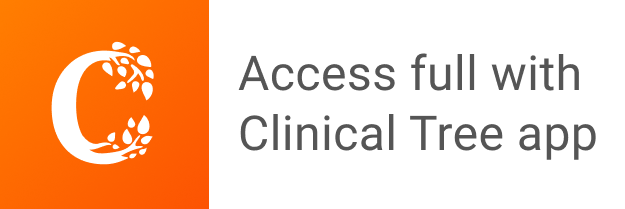