KEY TERMS
The ultimate goal of many epidemiologic studies is to determine the causes of disease. This is generally done first by observing a possible association between an exposure and an illness, second by developing a hypothesis about a cause and effect relationship, and third by testing the hypothesis through a formal epidemiologic study. While the formal study can strongly support the conclusion that a certain exposure causes a certain disease, there are many potential sources of error in drawing such a conclusion. Studies of chronic diseases, which often have multiple determinants and develop over long periods of time, are especially prone to error.
Problems with Studying Humans
All epidemiologic studies have the advantage of studying humans rather than experimental animals; but all are also limited by that fact. Each type of epidemiologic study has its own strengths and weaknesses.
Consider the design of an epidemiologic study to test the hypothesis that a low-fat diet reduces the risk of heart disease. The average American already eats a high-fat diet and has a high risk of heart disease compared with residents of many other countries, so it should be possible ethically to compare the health of people who eat this diet with others who have other dietary patterns.
The randomized controlled trial, the most rigorous form of intervention study, is the most similar in concept to a biomedical scientist’s experiment with rats. Suppose researchers choose a group of subjects who have been eating an average American diet and divide them randomly into an experimental group, who will be instructed to eat a strict low-fat diet over the next five years, and a control group, who will be told to continue eating normally. Researchers will monitor both groups, watching for signs of heart disease, and they expect that, if their hypothesis is correct, fewer people in the low-fat group will become ill.
In fact, researchers are likely to be disappointed with the results. The problem is that it is impossible to control the behavior of human beings under such circumstances. If the experiment was being conducted using rats, researchers would feed them the assigned diets and could thus be certain of the relative exposures of the two groups. With people, however, even if researchers could find enough of them who would agree to participate in the experiment, it is questionable whether they would remain on the appropriate diet over the necessary length of time. People in the experimental group might succumb to temptation and drop out of the study or lie about what they have eaten. People in the control group might become concerned about their health and voluntarily cut back on the amount of fat they eat. It is unrealistic to expect to succeed at a randomized controlled trial that requires people to alter their behavior over a significant period of time, unless the subjects have a special motivation to participate—if they are suffering from a serious disease, for example—and participation in a trial is their only chance to have access to a new, potentially more effective treatment.
To test the dietary hypothesis, researchers might try, instead of a randomized controlled trial, a cohort study. They would choose a large group of people who are free of heart disease, ask them detailed questions about their diets, and then, over the next five years, compare the health of those who already eat a low-fat diet with those who eat an average American diet. This would not require people to change their behavior. The problem with this scenario is that people who have voluntarily chosen to eat a low-fat diet may differ in other respects from the group who eat the average diet. The low-fat group members are likely be more health conscious in general. They may be less likely to smoke and more likely to exercise, for example. These people, therefore, would have a reduced risk of heart disease even if a low-fat diet did not have a protective effect.
The third type of study, the case-control study, has its own difficulties. In this study, researchers would choose a group of people who already have heart disease; perhaps they would go to a hospital and interview patients recovering from a heart attack. A comparable group of people who do not have heart disease would serve as the control group. Researchers would question people in both groups about their diets over the past five years and decide whether the diets should be classified as high-fat or low-fat. If the researchers’ hypothesis is correct, the patients who have had a heart attack will report a diet higher in fat than the control group. This approach also has obvious problems. People are not likely to remember what they ate in the past, or they might be embarrassed to admit how self-indulgent they have been. The information researchers obtain concerning exposure in the case-control trial may not be reliable.
These difficulties do not mean that no valid conclusion can be drawn from any kind of epidemiologic study. However, they demonstrate the types of errors to which different kinds of studies may be prone and alert researchers about what to watch out for in choosing a study design and in interpreting the results.
Sources of Error
News reports of new health studies can often be confusing. Sometimes there are conflicting reports on the health effects of various substances. Coffee is reported to cause heart disease; then it is reported that there is no such effect. Oat bran is reported to prevent cancer; then it is reported to make no difference. Fish is good for your heart; fish is full of toxic chemicals that may cause harm. All these contradictions tend to make people distrustful of the news and uncertain about how to protect their health. Since most of these news reports are based on epidemiologic studies, it is useful to understand possible sources of error in such studies and how to look for the truth in the reports.
One of the most common reasons for a study to lead to a wrong conclusion is that the reported result is merely a random variation and that the association is merely due to chance. As a general rule, epidemiologic studies of chronic diseases require large numbers of subjects to draw valid conclusions. Causes of these diseases are usually complex, and there are usually long periods between exposures to possible causes and the development of illness, making it difficult to draw conclusions about associations between exposure and disease. The cause-and-effect relationship is not obvious—as it is, for example, when a bullet in the heart causes death, or exposure of an unvaccinated child to the measles virus causes the child to develop measles in 10 to 12 days. The weaker the relationship between exposure and disease, the larger the group of people that must be studied for the relationship to be evident. If the group being studied is too small, a cause-and-effect relationship is likely to be missed or a spurious relationship will show up by chance alone. One of the reasons that the Doll–Hill and Hammond–Horn results concerning smoking and lung cancer are so convincing is that they involved such large numbers of subjects.
There are a number of other possible sources of error that well-designed studies may be able to avoid. For example, the cohort study of a low-fat diet proposed previously may be invalidated by the presence of confounding variables, like smoking and exercise. Confounding variables are factors that are associated with the exposure and that may independently affect the risk of developing the disease. Such an error may have occurred in a 1980s study that suggested coffee drinking could cause pancreatic cancer, a finding that has not been replicated in other studies. Since many heavy coffee drinkers were also smokers, there are suspicions that the cancer was caused by the smoking rather than the coffee.1 To eliminate the errors caused by smoking as a confounding variable, researchers might conduct the study only on nonsmokers. Alternatively, there are statistical techniques for adjusting the results to compensate for confounding variables as long as the investigator is clever enough to think of possible factors that may affect the result and to take them into consideration when collecting the data and calculating the results. While the investigators in the study of coffee corrected for smoking over the 5-year period before the cancer was diagnosed, the correction may have been inadequate.
An interesting example of confounding occurred in a study, published in 1999 and widely publicized, suggesting that small children were more likely to become myopic—nearsighted—if they slept in a lighted room. In a follow-up study, investigators asked the children’s parents about their own vision. It turned out that myopic parents were more likely to leave lights on in their children’s rooms than parents with better vision. Their children, therefore, were more likely to be nearsighted because they inherited the condition from their parents, not from the light exposure.2
Bias, or systematic error, may be introduced into a study in a number of ways. Selection bias is a particular problem in choosing subjects for a case-control study. For example, if the cases of heart disease are chosen from hospitalized patients recovering from heart attacks, and the controls include hospitalized patients being treated for a digestive disorder that causes extreme discomfort from eating fatty foods, the study may suggest an exaggerated effect of dietary fat on heart disease. The results would probably be different if the controls were patients recovering from the effects of motor vehicle crashes, whose diet might be more like the average American’s. Selection bias may also occur when there is a systematic difference between people who choose—or are chosen—to participate in a study and those who do not. For example, in a 1988 case-control study that found exposure to high electromagnetic fields (EMF) from power lines increased the risk of childhood cancer, the controls were chosen by a process of telephone random digit dialing until a child was located who matched a case by age and sex. Cases and controls were compared, and cases were found to have had a higher exposure to EMF. However, the cases also were also found to be of lower socioeconomic status; they were more likely to live in areas of high traffic density, and their mothers were more likely to smoke. The random-digit dialing had created a bias: Because poor families were less likely to have a telephone, or less likely to have an answering machine and to return calls, the control group was more affluent and consequently was less exposed to confounding poverty-associated factors.1
An extreme example of selection bias—one that no well-trained epidemiologist would make—was seen in the report of the author Shere Hite on male and female relationships. Out of 100,000 questionnaires on women’s attitudes about men and sex that Hite distributed, only 4500 replies were received. Hite reported that 84 percent of the women in the study were dissatisfied with their intimate relationships, results that were widely publicized. The low response rate suggests that selection bias was operating and that the most dissatisfied women were responding preferentially to the survey.3
Cohort studies, which tend to extend over many years, are likely to suffer from a form of bias caused by people dropping out or being untraceable when results are being sought. If people who get sick drop out at a different rate from those who remain healthy, the results will be compromised. Subjects who are lost to follow-up may be more likely than those who are traceable to have entered an institution or to have moved in with family, indicating a serious health problem. A high dropout rate casts doubt on the results of any epidemiologic study.
Reporting bias or recall bias is a common problem in case-control studies. It occurs if the study group and the control group systematically report differently even if the exposure was the same. Subjects’ reports of their dietary intake are notoriously unreliable. For example, underweight individuals consistently overreport their fat intake, while obese individuals underreport it.1 Similarly, studies attempting to relate certain diseases to alcohol consumption may suffer from reporting bias because people who drink heavily tend to underreport their consumption. Case-control studies that attempt to determine causes of birth defects are especially subject to recall bias, since the mother of a child born with a malformation is likely to have thought a great deal about what might have caused the problem, while mothers of healthy children would be less likely to notice an unusual exposure.
Proving Cause and Effect
For the most part, epidemiologic studies, no matter how well designed to avoid error, cannot prove cause and effect. In fact, that is why epidemiologists usually speak of risk factors rather than causes. However, there are several factors that can be combined to make the cause-and-effect relationship almost certain.
First, as discussed previously, a study with a large number of subjects is more likely to yield a valid result than a small study. Second, the stronger the association measured between exposure and disease—the higher the relative risk or odds ratio—the more likely that there is a true cause-and-effect relationship. For example, the Reye’s syndrome case-control study found a 42.7 odds ratio from exposure to aspirin during a viral infection. The British case-control study linking birth control pills to breast cancer found only a 2.3 odds ratio, while the Nurses’ Health Study—a cohort study—found at most a 1.5 relative risk of breast cancer from oral contraceptives. The much stronger association found in the Reye’s syndrome study makes it highly probable that aspirin causes the syndrome in children, while the breast cancer results could possibly be due to some error or alternative explanation. Nevertheless, exposure to hormones is generally accepted as a risk factor for breast cancer, as discussed in the next section.
Third, a dose–response relationship
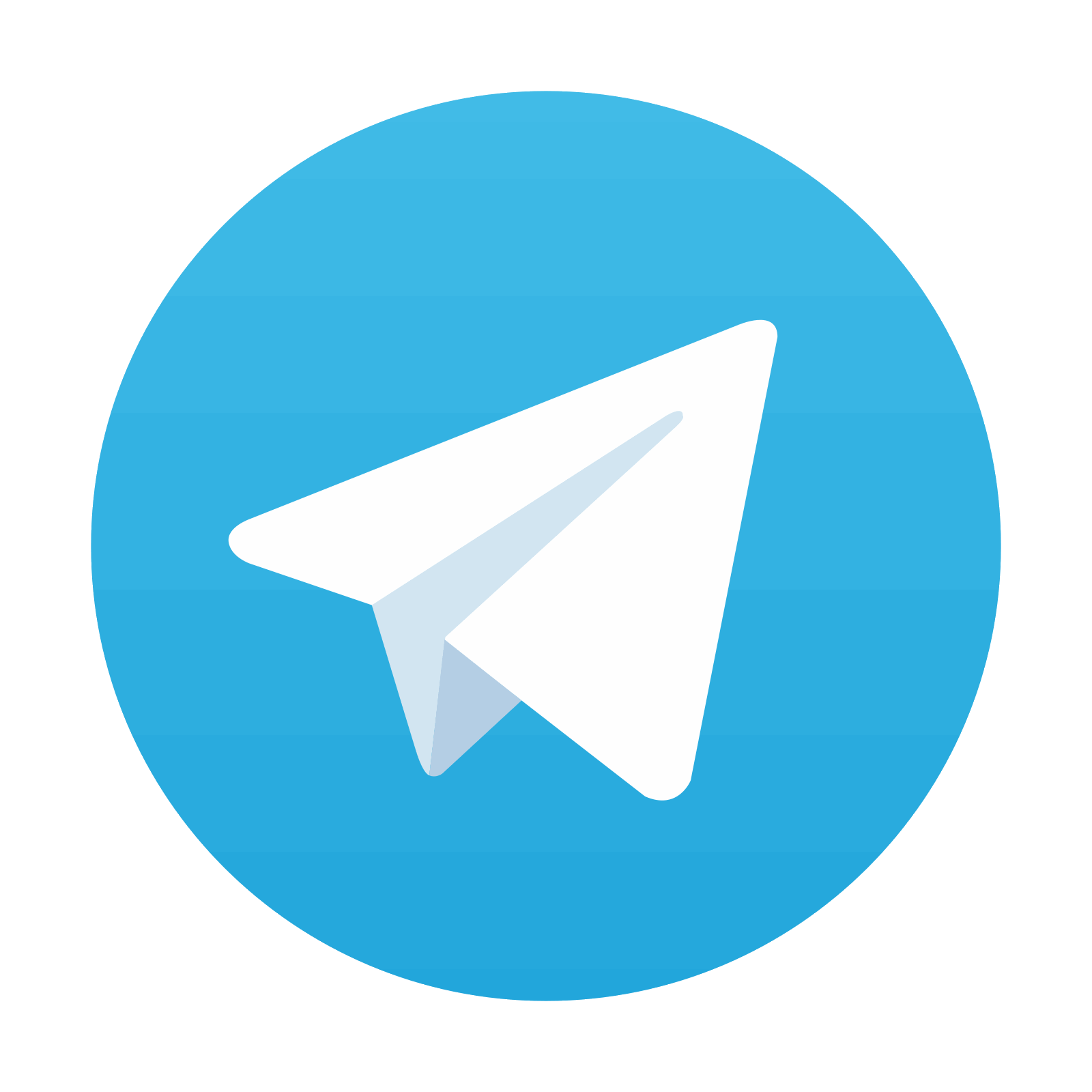
Stay updated, free articles. Join our Telegram channel

Full access? Get Clinical Tree
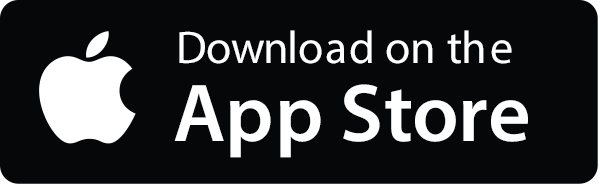
