The Problem
We have two samples that are related to each other and one numerical or ordinal variable of interest.
- The variable may be measured on each individual in two circumstances. For example, in a cross-over trial (Chapter 13), each patient has two measurements on the variable, one while taking active treatment and one while taking placebo.
- The individuals in each sample may be different, but are linked to each other in some way. For example, patients in one group may be individually matched to patients in the other group in a case–control study (Chapter 16).
Such data are known as paired data. It is important to take account of the dependence between the two samples when analysing the data, otherwise the advantages of pairing (Chapter 13) are lost. We do this by considering the difference between the values for each pair, thereby reducing our two samples to a single sample of differences.
The Paired t-Test
Assumption
In the population of interest, the individual differences are Normally distributed with a given (usually unknown) variance. We have a reasonable sample size so that we can check the assumption of Normality.
Rationale
If the two sets of measurements were the same, then we would expect the mean of the differences between each pair of measurements to be zero in the population of interest. Therefore, our test statistic simplifies to a one-sample t-test (Chapter 19) on the differences, where the hypothesized value for the mean difference in the population is zero.
Additional Notation
Because of the paired nature of the data, our two samples must be of the same size, n. We have n differences: their sample mean is and estimated standard deviation sd.
Refer t to Appendix A2.
Interpret the P-value and calculate a confidence interval for the true mean difference in the population. The 95% confidence interval is given by
If the Assumption is not Satisfied
If the differences do not follow a Normal distribution, the assumption underlying the t-test is not satisfied. We can either transform the data (Chapter 9) or use a non-parametric test such as the sign test (Chapter 19) or Wilcoxon signed ranks test to assess whether the differences are centred around zero.
The Wilcoxon Signed Ranks Test
Rationale
In Chapter 19 we explained how to use the sign test on a single sample of numerical measurements to test the null hypothesis that the population median equals a particular value. We can also use the sign test when we have paired observations, the pair representing matched individuals (e.g. in a case–control study, Chapter 16) or measurements made on the same individual in different circumstances (as in a cross-over trial of two treatments, A and B, Chapter 13). For each pair, we evaluate the difference in the measurements. The sign test can be used to assess whether the median difference in the population equals zero by considering the differences in the sample and observing how many are greater (or less) than zero. However, the sign test does not incorporate information on the sizes of these differences.
The Wilcoxon signed ranks test takes account not only of the signs of the differences but also their magnitude, and therefore is a more powerful test (Chapter 18). The individual difference is calculated for each pair of results. Ignoring zero differences, these are then classed as being either positive or negative. In addition, the differences are placed in order of size, ignoring their signs, and are ranked accordingly. The smallest difference thus gets the value 1, the second smallest gets the value 2, etc., up to the largest difference, which is assigned the value n′, if there are n′ non-zero differences. If two or more of the differences are the same, they each receive the mean of the ranks these values would have received if they had not been tied. Under the null hypothesis of no difference, the sums of the ranks relating to the positive and negative differences should be the same (see following box).
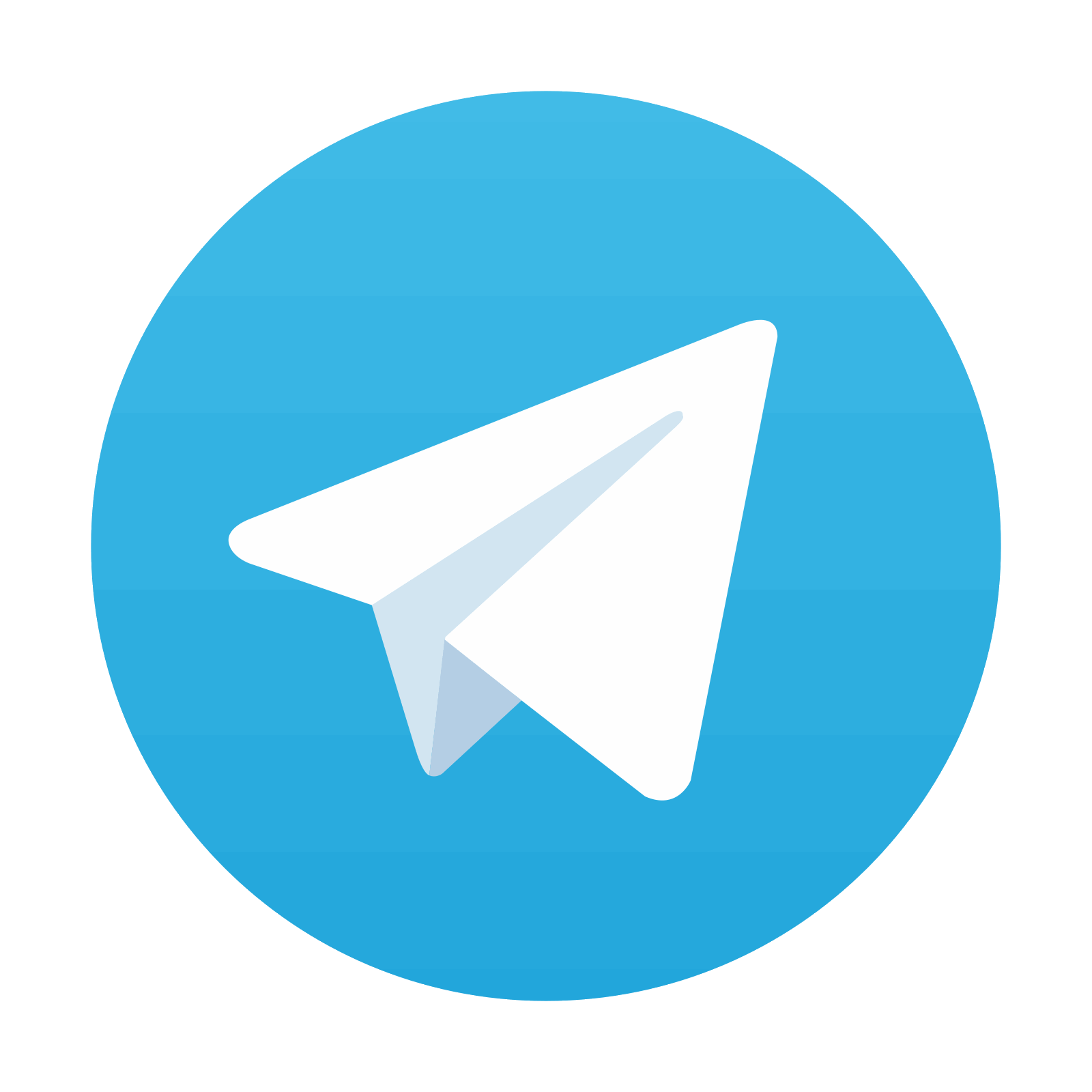
Stay updated, free articles. Join our Telegram channel

Full access? Get Clinical Tree
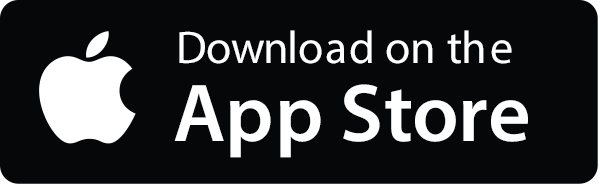
