19
Numerical Data: a Single Group
The Problem
We have a sample from a single group of individuals and one numerical or ordinal variable of interest. We are interested in whether the average of this variable takes a particular value. For example, we may have a sample of patients with a specific medical condition. We have been monitoring triglyceride levels in the blood of healthy individuals and know that they have a geometric mean of 1.74 mmol/litre. We wish to know whether the average level in the population from which our patients come is the same as this value.
The One-Sample t-Test
Assumptions
In the population of interest, the variable is Normally distributed with a given (usually unknown) variance. In addition, we have taken a reasonable sample size so that we can check the assumption of Normality (Chapter 35).
Rationale
We are interested in whether the mean, μ, of the variable in the population of interest differs from some hypothesized value, μ1. We use a test statistic that is based on the difference between the sample mean, , and μ1. Assuming that we do not know the population variance, then this test statistic, often referred to as t, follows the t-distribution. If we do know the population variance, or the sample size is very large, then an alternative test (often called a z-test), based on the Normal distribution, may be used. However, in these situations, results from both tests are virtually identical.
Additional Notation
Our sample is of size n and the estimated standard deviation is s.
Interpretation and use of the Confidence Interval
The 95% confidence interval provides a range of values in which we are 95% certain that the true population mean lies. If the 95% confidence interval does not include the hypothesized value for the mean, μ1, we reject the null hypothesis at the 5% level. If, however, the confidence interval includes μ1, then we fail to reject the null hypothesis at that level.
If the Assumptions are not Satisfied
We may be concerned that the variable does not follow a Normal distribution in the population. Whereas the t-test is relatively robust (Chapter 35) to some degree of non-Normality, extreme skewness may be a concern. We can either transform the data, so that the variable is Normally distributed (Chapter 9), or use a non-parametric test such as the sign test or Wilcoxon signed ranks test (Chapter 20).
The Sign Test
Rationale
The sign test is a simple test based on the median of the distribution. We have some hypothesized value, λ, for the median in the population. If our sample comes from this population, then approximately half of the values in our sample should be greater than λ and half should be less than λ (after excluding any values which equal λ). The sign test considers the number of values in our sample that are greater (or less) than λ.
The sign test is a simple test; we can use a more powerful test, the Wilcoxon signed ranks test (Chapter 20), which takes into account the ranks of the data as well as their signs when carrying out such an analysis.
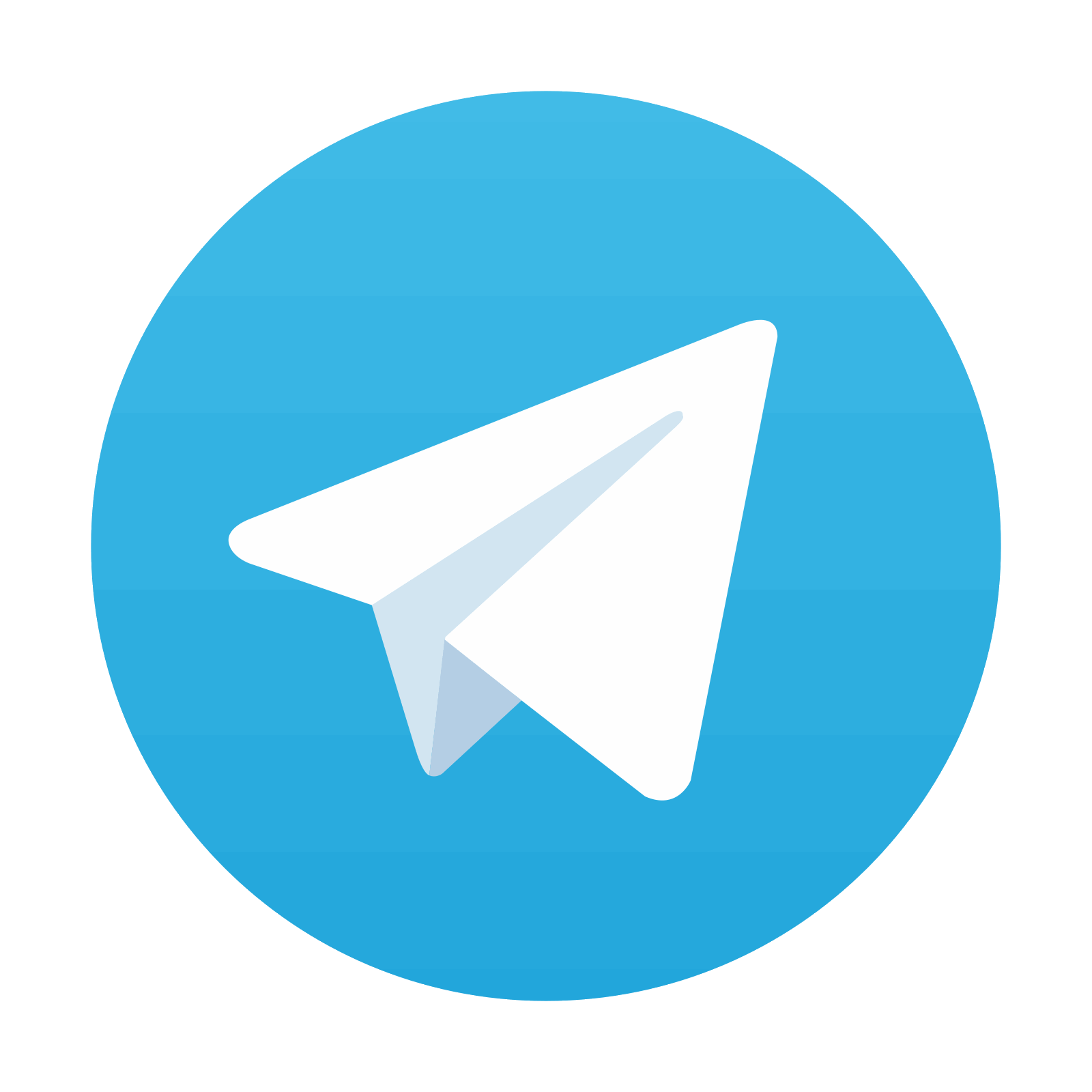
Stay updated, free articles. Join our Telegram channel

Full access? Get Clinical Tree
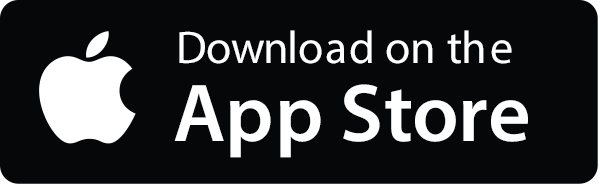
