Chapter 3
Molecular Pathology via Infrared and Raman Spectral Imaging
3.1 Introduction
Histopathology, the microscopic visual inspection of stained tissue sections from biopsies, has been the first line of diagnosis in the treatment of many diseases, in particular cancer. Modern histopathology dates back to the late nineteenth century, when Virchow [1, 2] first used microscopic techniques to gain insight into the changes in cellular morphology that accompany disease. Gross morphological changes that occur with the transition from healthy to diseased tissue include abnormal tissue architecture, the appearance of cells at locations where they are normally not found (metaplasia), the presence of inflammatory cells, abnormal cell morphology including changed nucleus to cytoplasm (N/C) ratio, abnormal nuclear membrane morphology, abnormal chromatin distribution, the number of nucleoli in the nucleus, the presence of an unusually large number of mitotic figures indicating rapid cell division, and a plethora of other often ill-defined features such as foaminess of the cytoplasm [3]. To arrive at these diagnostic descriptors, tissue sections, generally about 5 μm thick, are obtained by cutting, via a microtome, either as formalin-fixed paraffin-embedded tissue blocks or as unfixed, flash-frozen tissue sections. However, in either case, the tissue sections present themselves as white or grayish deposits on microscope slides, and show very little contrast under the microscope. Thus, stains with different affinities for cellular components have been used as early as the late nineteenth century to create a visual contrast in histopathology. The most common stain in histopathology is the hematoxylin and eosin (H&E) combination stain [4], which visualizes the basic proteins of the histones (mainly arginine) in dark bluish or purple color, whereas the eosin imparts a pinkish color to the cytoplasm of cells. We may think of the H&E staining procedure as a very basic “optical method of diagnosis,” as the two stains bind differentially to different cellular structure, making these structures absorb light of different wavelengths, thereby permitting their distinction. Thus, classical histopathology, which uses the human eye as a detector and databases stored in the pathologist’s mind as a diagnostic discriminator, has been the earliest form of optical detector to detect disease. A current trend is to shift conventional histopathology toward digital integration [5].
In order to reduce the inter-observer variability of classical histopathology, digital imaging acquisition (at one or more different visible wavelengths) and subsequent computer analysis of the resulting image planes of histopathological specimens have been introduced and commercialized; these methods are generally referred to as digital pathology or whole-slide imaging [6]. However, the analysis of such datasets is still carried out in the “morphology domain” rather than in the “spectral domain,” because the few wavelengths at which the images are acquired do not permit a true spectral discrimination, and because the images are based on external stains with low inherent discrimination between normal and abnormal structures in tissue.
Although histopathology has been extraordinarily successful as a first-line diagnostic tool, modern medicine, especially oncology, requires more than the subjective descriptors available from classical histopathology in order to decide on a suitable treatment regimen for disease. This requirement gave rise to the development of a new methodology that explores the abundance and localization of specific target molecules, such as cancer markers. The most common of these cancer-marker-specific techniques is immunohistochemistry (IHC), which was first described in 1942 [7]. This approach is generally described as a method of “molecular pathology,” as it reduces the reliance on morphological features, and instead uses changes in chemical composition – the presence or overexpression of certain marker molecules – as a primary means of distinguishing normal from diseased tissues. In IHC, an optical chromophore – generally a fluorescent label – is attached to a mono- or polyclonal antibody, to an antigen, or to other protein markers. Upon binding the antibody–chromophore complex to the target molecules in a cell or tissue, these binding sites can be detected by fluorescence microscopy. IHC has very high sensitivity and specificity, but is restricted by the fact that only markers for which an antibody is applied can produce a detectable signal; that is, unknown cancer markers cannot be detected in this approach.
Thus, different approaches have been developed, mostly by the biophysical and spectroscopic community. These may be based on detecting either inherent spectral signatures of cellular components or external stains and labels (as discussed above), but are not restricted to the visible wavelengths of light. Miss rates up to 25% have been reported for white-light endoscopy, which led to treatment of polyps without malignant potential and, consequently, without benefit but additional costs and risks to the patient [8]. Therefore, fluorescence-based techniques, such as the detection of inherent tissue markers, have gained acceptance mostly in endoscopic diagnoses, and use mostly ultraviolet excitation. Because of the few tissue components that exhibit significant autofluorescence, tissues are often stained with specific fluorophores such as 5-aminolevulinic acid (5-ALA) [9] or indocyanine green (ICG) [10] to increase sensitivity and specificity, particularly for in vivo observations.
During the last 15 years, methods have been developed that can be viewed as molecular pathology methods and depend on sampling the entire genome, proteome, and metabolome of cells and tissues, rather than probing for the presence of selected markers [11, 12]. These methods rely on the detection of the inherent spectral signatures of the biochemical components in a pixel of tissue. This is possible using vibrational spectroscopy, which comprises two well-established spectroscopic techniques. As the name implies, vibrational spectroscopy monitors the internal motion of atoms in molecules, which are in continuous thermal motion. These atomic motions in molecules are well understood and follow physical laws similar to those that govern the motions of spring-coupled masses. Vibrational spectroscopy can be observed in both absorption (infrared (IR) absorption spectroscopy) and light scattering (Raman spectroscopy), which differ in the way the molecular vibrations are excited and detected; however, both may be described as “fingerprint” techniques, as every molecule exhibits a distinct and specific fingerprint spectrum that allows identification of the molecular compound and quantitation of its abundance in a sample [13, 14].
For a complex sample, such as human cells or tissues, the observed IR, or Raman spectra are a superposition of all the spectra of the individual biochemical components, and the daunting task of utilizing these techniques for medical diagnosis is to decode the complex spectral signatures. Modern methods of mathematical data analysis, aided by computer algorithms, have succeeded in this decoding process, and the spectral methods are now poised to enter the mainstream diagnostic arena. Preliminary results from a number of laboratories worldwide [15] have indicated that these novel spectral methods can distinguish between normal tissue types, disease types, and stages, and even identify the primary tumors from spectral patterns observed in metastatic cells. Furthermore, these techniques can analyze single unstained and unlabeled cells, and therefore, can be used in the detection of circulating tumor cells (CTCs) [16, 17].
3.2 Background
The discussion of spectral methods of diagnosis has to start with a short review of the spectroscopies underlying the new methodologies, namely IR and Raman spectroscopy. In the former, broadband IR radiation (with wavelength between about 2 and 20 μm) is focused onto a sample, which absorbs IR radiation at specific wavelengths. The light transmitted by the sample lacks these absorbed wavelengths, and thus, presents “absorption spectra” similar to the ones shown in Figure 3.1. In this figure, several IR absorption spectra are shown for different compounds expected to be found in a cell or tissue. Here, the abscissa is given in “wavenumber” units, where the wavenumber is defined as the reciprocal of the wavelength, and indicates the number of waves per centimeter. Thus, the units of the wavenumber axis are 1/cm or cm−1. (The reason for choosing this unit is that the wavelength of light is indirectly proportional to the light energy, whereas the wavenumber is directly proportional to energy. Thus, a higher wavenumber corresponds to a higher photon energy.) The ordinate is a logarithmic plot of the amount of light absorbed by a specific vibration, and is presented in optical density (OD) units. One OD unit corresponds to 90% of the incident radiation absorbed. Because of instrumental operational parameters, it is not advisable to collect data spectra with more than 1 OD. The spectra reproduced in Figure 3.1 were “normalized” (see Section 3.3.3) to account for sample thickness; thus their ordinate values are arbitrary. Furthermore, they were offset along the Y-axis for easier presentation [15].

Figure 3.1 Infrared absorption spectra of selected cellular components.
The spectra shown in Figure 3.1 can be divided into two regions. The high-wavenumber region (2500–4000 cm−1, or 4–2.5 μm) corresponds to absorptions due to light atom stretching motions: at 3400 cm−1, the O–H stretching vibration is observed, whereas the N–H stretching vibration of the amide backbone is found between 3270 and 3310 cm−1, depending on the hydrogen-bonding properties of this proton. C–H stretching vibrations of aromatic, olefinic, and aliphatic C–H bonds occur between about 2800 and 3050 cm−1. The 700–1800 cm−1 region, frequently referred to as the fingerprint region, contains in the 1500–1700 cm−1 range the vibrations of the protein amide linkage, the “amide I” vibration at about 1650 and the “amide II” vibration at about 1550 cm−1 (see top three traces of Figure 3.1). For a listing and discussion of many protein vibrational frequencies, the reader is referred to a comprehensive review by Barth [18].
Inspection of Figure 3.1 reveals that proteins with different secondary structures exhibit quite different IR absorption spectra: helical proteins (such as albumin) exhibit a sharp amide I band at about 1655 cm−1, whereas pleated sheet structures exhibit a lower amide I frequency with a distinct high-frequency shoulder. This shoulder can be resolved into an individual peak by using a second-derivative methodology (see Section 3.3.3). Other biomolecular components of cells and tissues are found in the lower four traces of Figure 3.1, including polymeric sugars (glycogen), which exhibit strong C–O stretching and C–O–H deformation vibrations between 1000 and 1200 cm−1; DNA and RNA which can be identified by the vibrations of the phosphodiester vibrations (1095 and 1235 cm−1) and aromatic base vibrations between 1600 and 1700 cm−1; and phospholipids with distinct C–H stretching peaks between 2850 and 3000 cm−1 in addition to their own phosphodiester vibrations. IR absorption spectroscopy has long been applied to study the conformational and other aspects of proteins and biomolecules [18] and can be considered a mature and well-established field.
The companion technique to IR absorption spectroscopy is Raman spectroscopy [19, 20], which samples the same vibrations that give rise to IR spectra but using a different excitation mechanism. Instead of illuminating the sample with broadband IR radiation, the sample is illuminated by intense, monochromatic visible light (typically from a laser). The laser photons are scattered by the molecules of the sample; in the scattering process, the photons lose energy. A plot of the number of photons scattered at given photon energy (wavenumber) yields “Raman spectra,” as shown in Figure 3.2. Again, the abscissa is in wavenumber units (photon energy) and the ordinate indicates the number of scattered photons. Raman and IR spectra are, because of their different excitation mechanism, somewhat complementary and exhibit different sensitivities toward different moieties in the sample. Other differences include the sensitivity of the two methods toward water: IR spectroscopy is strongly confounded by water in the sample, as water is one of the strongest absorbers of IR radiation, whereas Raman spectroscopy is quite insensitive to the presence of water. Raman spectroscopy yields more detail on C–H vibrations, which are particularly strong in this technique. However, Raman spectra are generally weaker, and more difficult to acquire; hence, Raman spectra are generally noisier than IR spectra. IR spectra contain more conformational information on proteins (see Figure 3.1). Finally, Raman spectra can be collected from significantly smaller spots than IR spectra, an aspect that will be further elaborated in Section 3.3.2.

Figure 3.2 Raman spectra of selected cellular components.
When a spectrum of a voxel (pixel) of tissue or a cell is acquired, the spectral information collected depends on the biochemical composition, which varies between tissue types, cell types, location within a cell, state of disease, and so on. For example, the cytoplasm of squamous cells may contain glycogen, which manifests itself by a contribution as shown in Figure 3.1. The nucleus, on the other hand, may show signatures of nucleic acids, in particular during mitosis of a cell. Similarly, structural tissues (such as the stroma, ligaments, and cartilage) are rich in collagen which has a distinct spectral signature. Thus, when IR or Raman spectra are collected from distinct regions or areas of cells or tissue sections, variations in the spectral patterns are observed that can be correlated to the tissue types from which the spectra were collected.
Such spatially resolved spectral data can be collected by carrying out either IR or Raman measurements through a microscope [21]. For Raman microscopy, the instrumentation is very similar to confocal fluorescence microscopy, and utilizes a basic visible microscope to which a laser source and a monochromator for the analysis of the scattered light have been added. The spatial resolution achievable (cf. Section 3.3.2) in Raman microscopy is similar to that of confocal fluorescence microscopy [22].
However, the instrumentation for IR microspectroscopy is quite different, as IR radiation is not transmitted by glass. Thus, all optical components, including the microscope objectives, are mirror-based. Furthermore, the long wavelength in IR microscopy prevents objects smaller than about 10 μm in size to be resolved. Nearly all IR microscopic measurements are performed interferometrically, that is, the signal observed initially is not the desired IR spectrum but its Fourier transform. Conversion of the interferogram to the spectral domain is carried out automatically via the instrument control software.
In the context of this chapter, we shall refer to IR-spectroscopy-based medical diagnostics as infrared spectral histopathology (IR-SHP). Most of this chapter deals with this subject. The methodology used in IR-SHP will be elaborated on in more detail in Section 3.3; may it suffice here to present a very short introduction to this methodology. Unstained tissue sections are used to collect an IR “spectral image,” that is, an image where each tissue pixel is not just represented as a color value (as, for example, in digital photography), but by an entire IR spectrum. Analysis of such a spectral image, to be described in Section 3.3, yields a pseudocolor rendition of the tissue section, which is based on the biochemical composition of the tissue, rather than on the morphology, tissue architecture, or staining patterns.
When IR spectral data acquisition is applied to the diagnostics of individual exfoliated cells, we refer to this technology as infrared spectral cytopathology (IR-SCP). IR-SCP can be carried out by the collection of one spectrum for each cell, or by imaging a cell at a pixel size much smaller than the cell and reconstructing a cellular spectrum from the individual pixel spectra. This latter approach lends itself to the automatic acquisition of cellular data (see Section 3.3), and was patented under the name of “PapMap” by some of the authors of this review [23, 24]. The PapMap approach has allowed the automatic collection of datasets containing over a million cellular spectra, as well as their analysis in terms of the origin of the cells, disease state, viral infection, and so on.
If Raman spectral data acquisition is applied to tissues, we refer to this method logically as Ra-SHP. As Raman and IR spectroscopy are complementary techniques, both Ra-SHP and IR-SHP give similar information. However, because of the smaller sampling area in Ra-SHP (see Section 3.3), the acquisition of Ra-SHP data from large tissue sections is impractical. However, Ra-SHP affords much higher spatial resolution [25, 26], which allows individual cellular components, such as the nucleus [27], or even mitochondria [28], to be detected in the spectral images. Furthermore, it has the enormous advantage of relative immunity from water interference; thus it is readily amenable to data collection of live tissue or in vivo applications. In fact, several research groups have published numerous reports on Raman histopathology of hollow organs or extracellular matrix [29], or acquired through needles fitted with fiber optics [30–33]. Although the spatial resolution of these methods is less than that of microscopic Ra-SHP, the fact that an entire tissue fingerprint can be collected in a few seconds of data acquisition time bears an enormous advantage for in vivo tissue diagnosis.
Finally, Ra-SCP, the study of individual cells by Raman microscopy, will be discussed. Ra-SCP can be carried out in two ways. First, one can utilize the high spatial resolution of the method to collect imaging data sets from one cell, consisting of 10 000 or more individual spectra, and reconstruct a spectral image of this cell. This results in images that can differentiate the individual cellular components such as the membrane, the cytoplasm, the nucleus and nucleoli, membrane-rich structures in the cytoplasm, and mitochondria [34–36]. This method can be viewed as a label-free analog of confocal fluorescence microspectroscopy, and can produce images with similar high spatial resolution. For example, the dynamics, subcellular origin, and chemical composition of lipid droplets were monitored in living hepatocytes [37]. However, Ra-SCP imaging does not offer the same contrast as is afforded by antibody-bound fluorophores. Second, Ra-SCP can also be carried out by collecting spectra from individual cells by not utilizing the high spatial resolution (by using longer wavelength excitation and lower numerical aperture (NA) objectives) but aiming the exciting laser beam at specific targets in the cell, typically the nucleus. This method is more labor intensive, but yields in a relatively short time hundreds of spectra of selected cellular features that show enormous sensitivity toward many biochemical processes, such as the metabolic activity in live yeast cells [38, 39]. For example, this approach has been used very successfully to follow stem cell differentiation [40–42], photochemical changes in melanosomes [43], or for the detection of CTCs in flow cytometry [16, 17]. Finally, because of the very high spatial resolution of Ra-SCP, spectral information from individual bacterial cells can be collected [44, 45]. This aspect may be of tremendous importance for tracking the origin of bacterial contaminations or outbreaks of infectious diseases, as Raman spectroscopy can, in principle, identify one bacterial particle at the strain level.
3.3 Methods
This chapter differs from the “Materials and Methods” section in typical research papers in that the methods used in IR and Raman diagnostic applications are described on a qualitative level without going into the experimental details. In particular, the mathematical steps will be described without equations, but rather in a pictorial way to introduce the concept. This chapter discusses aspects of
- 3.3.1 Sample preparation
- 3.3.2 Collection of hyperspectral data cubes
- 3.3.3 Data pre-processing
- 3.3.4 Data pre-sorting (cluster analysis)
- 3.3.5 Visual imaging
- 3.3.6 Annotation
- 3.3.7 Diagnostic data analysis
- 3.3.8 Factor methods
3.3.1 Sample Preparation
Tissue samples for both Raman and IR data acquisition are prepared as follows: Tissue sections, either from formalin-fixed paraffin-embedded tissue blocks or from frozen tissue, are cut using a microtome and mounted on suitable substrates. For IR-SHP, the thickness of the sample should not exceed about 5 μm if the sample is examined in transflection mode (see below) or 10 μm for transmission measurements (Figure 3.3).

Figure 3.3 Measurement in infrared transmission (left) and transflection (right) mode. In the transflection mode, the IR beam passes through the sample, gets reflected by the Ag layer covering the substrate, and passes the sample again, before reaching the detector.
For the former case, the substrate of choice is the “low emissivity” (generally referred to as low-e) slide, which consists of glass with a thin silver coating and an inert overcoat. These slides are completely reflective in the IR spectral region and nearly completely transparent in the visible region; thus, samples on these substrates can be imaged in the IR spectral region, and subsequently stained and imaged with visible light for correlation between classical and spectral histopathology (SHP). Unstained tissue sections on the low-e slide are used for IR data acquisition (molecular stains would interfere with data collection), and, subsequently, they are stained and cover-slipped and used for classical histopathological analysis. Recently, an electric field standing-wave artifact has been reported for FTIR microspectroscopy of biological material [46]. As this artifact causes confounding spectral variations related to the sample thickness, a low-e slide should be used with care. Classical histopathology, along with IHC, is used to train algorithms for the automatic diagnosis of IR spectral datasets. For Ra-SHP, tissue sections may be thicker than 10 μm if the Raman data acquisition is carried out confocally, as the thickness of the sampled image slice is determined by the optical arrangement. The substrate for Ra-SHP ideally is a fluorescence-free quartz or CaF2 slide, although we have recently collected good Ra-SHP datasets from 5 μm thick tissue sections mounted on low-e slides as well [47].
After mounting the tissue sections on appropriate substrates, the samples are air-dried, which can be considered an effective way of fixation because the samples become resistant to further degradation and remain virtually unaffected for days or weeks [48, 49]. On the other hand, dehydration causes denaturation of biomolecules such as lipids, proteins, and nucleic acids. Hydrophobic lipids such as cholesterol and cholesterol ester form microcrystals, proteins precipitate and become insoluble, and DNA changes conformation from the normal B-form to A- or unordered forms [50]. This denaturation can be prevented by keeping the samples moist or transferring them into an aqueous buffer during data acquisition. While it is more challenging to collect IR data from such samples because of the strong mid-IR absorbance of water, Raman data can easily be collected, and enable to transfer the results to in vivo conditions.
For IR-SCP, we have found that spin-depositing cells from a suspension, using a cyto-centrifuge, onto a low-e slide works very well. Depending on the concentration of cells in the original suspension and the deposition time, sparse monolayers of (unstained) cells can be produced. For relatively large squamous epithelial cells, we typically aim for about 50 cells mm−2. Cells generally adhere well to the low-e slides, which may be coated by poly-l-lysine to improve adhesion. This method lends itself to the automatic collection of SCP datasets. Cultured cells can be grown directly onto IR-compatible substrates. Cells may be stained after IR image acquisition for classical cytopathological analysis.
For Ra-SCP, a few microliters of a cell suspension typically is dropped onto a quartz or CaF2 slide and allowed to dry. Cells are selected under a microscope for data acquisition. This mode of data acquisition lends itself to the analysis of large numbers of cells, and has been used widely [40–42, 51]. However, in the context of this chapter, another imaging-based method for Ra-SCP data acquisition will be introduced. As we were interested in studying the uptake and distribution of drugs into cells, we have allowed cells to attach themselves to a substrate before they are treated, in vivo, with drugs or drug delivery systems. After incubation times that vary between a few minutes to 24 h, cells were fixed, and imaging datasets were collected, using a water-immersion objective, of cells in their native aqueous surrounding. The use of imaging methods (see next section) allows the exact locations of drug and drug carriers to be determined.
Nonadherent cells such as blood cells or cells in other body fluids (saliva, urine, and cerebrospinal fluid) can be studied by Raman spectroscopy with minimal sample preparation. If the Raman excitation laser is focused onto the cell, the high electric field acts as laser tweezers, retaining the cell inside the focus during data acquisition. Optical traps are formed by two counterpropagating lasers, which enables the separation of Raman excitation and micromanipulation of cells. These optical tools are compatible with microfluidic devices and have paved the way toward Raman-activated cell sorting (RACS): cells are injected into microfluidic channels, optically trapped, identified by their Raman spectroscopic signature, and subsequently sorted.
3.3.2 Collection of Hyperspectral Data Cubes
IR and Raman imaging methods contain both spatial and compositional information in one measurement, similar to immunohistochemical imaging technology. However, as pointed out above, Raman and IR data are collected from inherent spectral fingerprint signatures, and do not require any external dyes or labels. In IR-SCP and IR-SHP, spectra are collected from voxels of tissue about 10 μm × 10 μm in the lateral (x,y) dimensions and about 5 μm in the axial (z) direction. The axial dimension is determined by the thickness of the tissue or cell, whereas the lateral dimensions are determined by a physical quantity known as the diffraction limit, which depends on the wavelength of light and the NA of the microscope objective. In RA-SHP and RA-SCP, the wavelength of light is much smaller than in the corresponding IR methods; consequently, smaller voxels can be illuminated. For the high-resolution work from the LSpD to be described later, the lateral dimensions were about 300 nm × 300 nm, with an axial resolution of about 1 μm. For the RA-SCP studies reported by other groups, the lateral dimensions often exceeded 1 μm × 1 μm [27].
In both Raman and IR-based imaging methods, spectra from the sampled pixels are collected and arranged as “spectral hypercubes” or “hyperspectral data cubes,” shown in Figure 3.4. The bottom of this graph shows the pixels (or voxels) from which the spectral data were collected along with the voxel coordinates; the spectra themselves are indicated by the vertical wavelength (or wavenumber) axis, and the spectral intensities as shown. Further dimensions can be introduced, such as the axial coordinate in confocal microscopy or the time domain for time-dependent experiments. Such a spectral hypercube contains all the pertinent information of the tissue composition and its spatial variations; however, in its raw form, it is useless for medical diagnostics. Thus, data processing is carried out on this hypercube to present the spectral information in a suitable form for medical diagnosis. Each spectrum in the data cube is stored as a “spectral vector,” which typically contains a column of wavenumber values, each followed by the intensity (Raman scattered intensity or IR absorption) values. If the data points in a spectral vector are equidistant, it is sufficient to store an initial wavenumber, the number of points, and an increment value to save storage space.

Figure 3.4 Schematic diagram of a hyperspectral data cube.
The size of a hyperspectral data cube depends, of course, on the size of the tissue or cell to be imaged. Tissue sections often measure several square millimeters in size. Given the pixel (voxel) dimensions discussed above, a 3 mm × 3 mm tissue section would produce a hyperspectral dataset consisting of 300 × 300 (=90 000) spectra. As each spectrum typically consists of about 1500 intensity/wavelength data points, such a dataset easily occupies around a GByte 1 GB of disk space. Owing to the much smaller pixel size in Raman imaging, a 100 × 100 pixel dataset will typically only cover an area of 100 μm × 100 μm to about 1 mm × 1 mm.
3.3.3 Data Preprocessing
A number of recent publications [23, 52, 53] have dealt with data preprocessing steps and methods, particularly for IR-based measurements, which will not be detailed here. These steps are necessary because any experimental data are contaminated by noise, unwanted spectral features, distortions, and so on, the source of which are well understood. Most important are corrections for Mie scattering contributions which can shift the observed peaks and cause intensity distortions [54, 55]. Furthermore, data sometimes are collected in domains that are not suitable for further data analysis (time vs frequency domains, for example) and need to be converted from one to the other by a procedure known as Fourier transformation (FT). All magnetic resonance imaging (MRI) data, for example, are recorded in the time domain and converted, via FT, to the frequency domain. Similarly, raw IR spectra are produced by FT from interferometrically acquired data; hence, IR methodologies are often referred to as FTIR spectroscopy.
IR spectra may be superimposed on sloping baselines, which confound computer interpretation of spectral features. Thus, spectral interpretation is frequently carried out on second-derivative spectra; the process of calculating the second derivative of the intensity with respect to the wavenumber removes baseline fluctuations and reduces the apparent width of the spectral peaks. The intensity of IR spectra depends directly on the path the IR beam travels through the sample; consequently, the sample thickness determines the observed absorption strength. To account for variations in sample thickness of a cell or tissue section, the second-derivative spectra are normalized.
Raman spectral data need to be corrected for spectral spikes that result from the interaction of cosmic rays and the detector elements, and should be converted to linear wavenumber scales. As Raman data are generally too noisy to warrant conversion to second derivatives, a baseline is subtracted from the spectra to correct for the auto-fluorescence background. Although it has been noted that the auto-fluorescence can contribute to the distinction of tissue types (e.g., normal vs non-normal), it usually exhibits too low a specificity for accurate differential diagnosis. Then, the spectra are normalized to compensate for confounding variations in sample thickness, laser focus, and penetration depth.
The preprocessing procedures listed above generally are applied consecutively to each spectrum in a dataset; given the size of the hyperspectral datasets (see Section 3.3.2), preprocessing amounts to a substantial computational effort and may take a few minutes of computation time.
3.3.4 Presorting (Cluster Analysis)
The goal of spectral diagnostic methodology is the recognition of tissue spectral features, and the utilization of these features to aid in medical diagnoses. As pointed out previously, the raw data contained in a spectral hypercube cannot, a priori, be used for this purpose, and methods need to be developed to accomplish this task. Most of the researchers in the field of optical diagnosis via vibrational spectroscopy have adopted a diagnostic scheme that is summarized in Figure 3.5. The data-processing step starts with the preprocessed hyperspectral (Raman or IR) dataset of the unstained tissue, and converts it into a pseudocolor image. This step, in principle, could be performed by color coding an intensity value (e.g., red: high and blue: low) at a given wavelength and plotting the color codes at the pixel coordinates. Similarly, intensity ratios, band positions (wavenumbers), or half-width could be encoded as well. However, these so-called univariate methods (because only one intensity value of a spectrum is used) are counterproductive in the sense that the rest of the information contained in the spectra is not utilized. Multivariate methods, in contrast, utilize the entire spectral vectors to create images from the hyperspectral datasets. One commonly used multivariate method is unsupervised hierarchical cluster analysis (HCA) [21, 56], which calculates the similarity of all spectra in a dataset and assigns color codes to spectral groups, or clusters, based on their similarity. Figure 3.5 shows such an HCA-based spectral image and, for comparison, an H&E stained image of the same tissue section (see Section 3.3.5). It should be emphasized at this point that the conversion of the hyperspectral data cube into the HCA image is completely “unsupervised,” which in this context implies that the HCA algorithm needs no training, but arrives at the pseudocolor HCA image strictly by a mathematical comparison of spectral similarity.

Figure 3.5 Schematic of SHP process. (a) Pseudocolor image of tissue section obtained from hyperspectral data cube by HCA. (b) Photomicrograph of same tissue section, H&E stained after IR data acquisition. (c) Data bases extracted from HCA image during annotation process. (d) Diagnostic algorithm trained with database “C.” (e) Hyperspectral dataset from unknown sample. (f) Pseudocolor SHP image produced by a diagnostic algorithm to distinguish activated B lymphocytes from other tissue types.
3.3.5 Visual Imaging
At this point in the diagnostic procedure, the tissue section is stained (typically using H&E stain) and imaged, using a visual microscope at 20× or 40× magnification and a high-resolution digital camera. A comparison between the H&E digital image and the HCA image, based on the IR or Raman datasets, shows a very high correlation between the tissue boundaries apparent in the H&E image and the boundaries in the HCA image (see Figure 3.5). This indicates that IR spectra detect changes in tissue composition that parallel the different pathological features observed in the H&E image. The images generally are aligned with respect to each other using prominent tissue features.
3.3.6 Annotation
The next step toward training a diagnostic algorithm to automatically detect and assign tissue types or diseased areas is the correlation of spectral regions identified by the HCA map with regions that can be recognized by a pathologist in the H&E images. To this end, corresponding regions in the HCA image and the H&E image are selected, as indicated by the black arrows in Figure 3.5. Spectra from the selected areas are extracted and tagged with a diagnostic code, for example, activated B lymphocytes (red areas in Figure 3.5) or endothelial cells (light blue areas). The selected spectra are entered into a database for subsequent training of diagnostic algorithms. This annotation step is necessary only during the training phase of a diagnostic algorithm or to establish an annotated database for algorithm testing; however, this step is omitted for the diagnostic analysis of an unknown sample.
3.3.7 Diagnostic Data Analysis
As indicated earlier, the overall goal of the optical diagnostic methods is to provide medical information on a tissue section based on chemical composition and compositional variations rather than on morphological criteria. In order to correlate the compositional changes with disease, self-learning mathematical algorithms are utilized that correlate spectral (compositional) changes with any other available diagnostic information, such as immunohistochemical or – particularly in the early stages of development – classical staining and morphological changes, and disease outcome. Thus, the self-learning algorithms for analyzing spectral data may be based on morphological correlations, although the goal of these efforts is to move away from the morphological descriptors and utilize strictly compositional aspects of the tissue samples.
After establishing databases of annotated spectra from many patients with the same disease diagnosis, algorithms are trained to recognize the distinct spectral features associated with a particular disease. Although it is possible to train self-learning algorithms that recognize several disease states and tissue types, it is advantageous to design an algorithm tree, or a set of hierarchical algorithms that analyze the data in consecutive binary steps, as indicated in Figure 3.6. Depending on the tissue type and disease state, it may be advantageous to distinguish the most different class of spectra first. In breast cancer, for example, the most different tissue type may be adipose tissue, whereas in certain blood cancers it may be advantageous to test for the spectral class of erythrocytes first. Subsequently, spectral classes with the next highest variance may be differentiated, for example, molecular subtypes of breast cancer [57]. Thus, the diagnostic algorithm may be broken down into a set of binary decision steps. The order of the binary decision steps may be determined by classical methods of multivariate analysis via spectral covariance, or by performing hierarchical clustering of the spectra in the annotated database.

Figure 3.6 Schematic of a hierarchical decision tree for tissue diagnostics. Each of the endpoints (pure tissue type A, pure tissue type B, etc.) could be the starting point for further algorithms for a finer discrimination (tissue subtypes).
The training of each of the binary decisions consists of the following steps, explained here for the case of a binary decision between two spectral classes, A and BCD (see Figure 3.6). First, a “feature selection” is carried out that utilizes hundreds or thousands of spectra in each of the databases of classes A and BCD to determine which spectral intensities, or “features,” can reliably differentiate between the two classes. Although feature selection is a mathematical process, a pictorial view of this process is presented in Figure 3.7. Here, a set of spectra from two classes is shown, along with spectral features, indicated by blue arrows, that allow discrimination of the two classes. Note that other peaks, indicated by the white arrows, do not contribute to the discrimination, as the spectral differences are either weak or not unique. Thus, feature selection can be viewed as a process that removes irrelevant features from the data. A typical fingerprint region spectral vector may consist of about 500 intensity points; typically about 1/10 of those are useful in discriminating the spectral classes. Several mathematical methods exist, for example, as MATLAB library functions (The Mathworks, Natick, MA, USA), to carry out feature selection.

Figure 3.7 Feature selection to discriminate two spectral classes (red and blue), with relevant features given by blue arrows.
Next, a self-learning algorithm is trained to quantitatively discriminate between the spectral classes A and BCD. Recent progress in chemometric methods [58] has led to several algorithm types based on artificial neural networks (ANNs), support vector machines (SVMs), random forests (RFs), and others. Their performance, when properly trained and validated, is quite similar, and we shall use ANNs for an example for the following discussion. For unbiased training and test runs of the ANN, the dataset is split into completely independent training and test sets. A typical number of training spectra in applications reported by us would consist of 6000 spectra, from 12 patients, for tissue class A, and 6000 spectra of classes BCD, equally divided between classes B, C, and D (2000 spectra each, from four patients in each class). The ANN in the training phase “learns” to associate the selected spectral features with the diagnostic outcome; in particular, it assigns “weights” to each of the features to achieve an optimal balance between the sensitivity (which evaluates the percentage of true positive diagnoses) and the specificity (which evaluates the percentage of false negative diagnoses) against a gold standard. For the training set, the outcome (sensitivity and specificity) is generally very high, >98%. When applying such a trained algorithm to test the dataset, the sensitivity and specificity typically are better than 80%, often even better than 90%, if sufficiently large datasets are utilized and if the annotation (Section 3.3.6 above) is carried out sufficiently carefully by a pathologist to give pure class spectral databases.
3.3.8 Visual Data Analysis: Factor Methods
Several factor methods have been developed for SCP and SHP to decompose datasets into a bilinear model of variables. Similar to the HCA discussed above, factor methods are unsupervised and can reveal small but recurring spectral differences in a dataset. Because different mathematical solutions exist for decompositions, further constraints are introduced. In principal component analysis (PCA) [58], a set of new spectra, known as principal components (PCs) or loading vectors, is calculated from the covariance matrix of the entire dataset. These PCs contain, in the order of decreasing magnitude, the variance in the dataset: the first PC might, for example, be the mean (average) of all spectra, the second PC, the largest deviation from the mean, and so on. Usually, only about 30 PCs are needed to capture nearly all (99.5%) the variance in the dataset, which implies that the original dataset can be expressed as linear combinations (mixing) of these 30 or so PCs. Furthermore, it is assumed that similar spectra in the original dataset require the same PCs, and the same abundances of these PCs, to reconstruct the spectra. When plotting the contributions (scores) of one PC versus the contributions of another PC, a “scores plot” is obtained that can show whether the spectra are related to each other or not.
Vertex component analysis (VCA) [59] and N-FINDR [60] are other factor methods that have recently been introduced. They decompose a hyperspectral dataset into linear combinations of endmember spectra which are considered the pure “component” spectra. The VCA algorithm, for example, finds the most different spectra in a dataset of spectra and considers them to be the endmembers. Subsequently, all spectra in the dataset are reconstructed in terms of these endmembers. This is similar to the decomposition of a spectral dataset into its principal components except that in VCA the most dissimilar spectra are used as endmembers, whereas in PCA the spectra containing the most variance are used as basis for the decomposition. One constraint in VCA is that the concentrations, or abundances, of the endmembers are non-negative. Further details can be found elsewhere [61, 62].
3.4 Results and Discussion
Our foray into SHP and SCP started, similar to the early steps taken by several other research groups, with attempts to detect and differentiate different stages of cervical disease, both in exfoliated cells and in cervical biopsies. In the former case, we quickly realized that the problem was untractable in the late 1990s and early 2000 due to the lack of understanding of many of the spectral problems associated with single-cell spectroscopy (see Section 3.4.3.1) and the need to collect 10 000–100 000 cell spectra to arrive at statistically significant results. For cervical tissue samples, the heterogeneity of normal tissue, with enormous variations in glycogen concentration in the squamous epithelium, and sometimes ill-defined diagnoses in the cases of mild cervical disease, presented serious problems in the original work. Thus, we decided on a problem that seemed better defined in terms of diagnosable disease, namely the detection of metastatic cancer cells in lymph nodes tissue. Indeed, this problem proved to be quite amenable to study via IR-SHP, and yielded interesting insights into the immune response of the body toward cancer cells.
3.4.1 IR-SHP: Detection of Metastases and Micro-Metastases in Lymph Nodes
The earliest results were obtained for lymph nodes with large metastatic cancer regions, which were easily visible in stained tissue, and which had a readily accessible medical diagnosis. One of the earliest results obtained is shown in Figure 3.8, which depicts a section from an excised lymph node with a well-defined region of metastatic colon adenocarcinoma (ADC). The images were obtained using a pixel size of 25 μm × 25 μm (larger than what is presently used) and unsupervised cluster analysis (see Section 3.3.4) to construct the pseudocolor images. Thus, the images shown in Figure 3.8 are not based on any diagnostic input, but strictly use the spectral information in the IR-SHP dataset to distinguish tissue types.

Figure 3.8 (a) Photomicrograph of an 8 mm × 8 mm lymph node tissue section with colon cancer metastases (in blue ellipse and circle). (b) HCA-based pseudocolor image of section shown in (a) depicting different tissue types, including the adenocarcinoma (dark red). (c) Photomicrograph of partial section of same lymph node, showing reactive lymph node follicles. (d) HCA-based pseudocolor image of the section shown in (c), demonstrating the distinction between activated (dark brown) and nonactivated (dark blue) B-lymphocytes (dark blue) by IR-SHP.
Panels a and b of Figure 3.8 depict an H&E stained photomicrograph of an entire lymph node section, measuring about 8 mm × 8 mm in size, and the corresponding IR-SHP pseudocolor image obtained by HCA, respectively. In Panel b, the tumor (dark red) is differentiated well from the medullary sinus (light blue), the germinal centers (red), the T lymphocytes (dark blue), and the capsule (yellow and blue-gray). Here, there is no pathological ambiguity in the diagnoses of cancer and normal tissue, and the IR-SHP results very well agree with the visual image. Panels c and d present enlarged sections of the same lymph node, containing only normal tissue. In Panel d, the germinal centers (dark brown), surrounded by the mantle zone (dark blue), are clearly visible, indicating, for the first time, the spectral differentiation of B-lymphocyte activation: the germinal centers in a lymph node are activated by an antigen, here most likely originating from the cancer in the adjacent tissue. This B-lymphocyte activation is accompanied by high proliferation rates, which change the chemical composition observable in IR-SHP. In Panel d, the capsule of the lymph node (orange region) is also recognizable; this region consists of fibro-connective tissue which has a significantly different chemical composition that is easily recognized in IR-SHP. These results demonstrated the enormous sensitivity of IR-SHP, because the biochemical differences between activated and nonactivated B lymphocytes are quite subtle, yet are recognized by spectral methods. These results also demonstrated unambiguously that a region of ADC has biochemical characteristics that are significantly different from those of B and T lymphocytes and normal structures in lymph nodes.
Figure 3.8 also demonstrates one limitation that was discovered early during this work. The cluster analyses shown in Panels b and d were carried out omitting the amide I spectral range, as the bands in this region were contaminated by spectral artifacts due to Mie scattering of the lymphocytes. Mie scattering is a well-known effect in classical physics and occurs when the light incident on spherical sample particles has about the same wavelength as the size of the particles (see Section 3.3.3). In addition to producing broad, undulating background features superimposed on the absorption spectra of the sample, Mie scattering also can mix the reflective and absorptive components of the interaction of radiation with the sample; this mixing, which has been referred to as resonance Mie (RMie) scattering [55, 63, 64], can cause severe distortion of the absorptive line shapes observed in IR spectroscopy. This distortion causes an apparent shift in the band position (up to 40 cm−1 in extreme cases) and severe distortions of observed intensities. The interference between absorption and scattering effects has been the subject of several recent papers [64, 65], and several approaches have been developed to account for them [55, 65, 66]. In more recent spectral images reported later in this review, these artifacts have been accounted for in the preprocessing methods described in Section 3.3.3.
3.4.1.1 IR-SHP of Micro-Metastases in Lymph Nodes
Although the detection and diagnosis of large metastases, such as the one shown in Figure 3.8
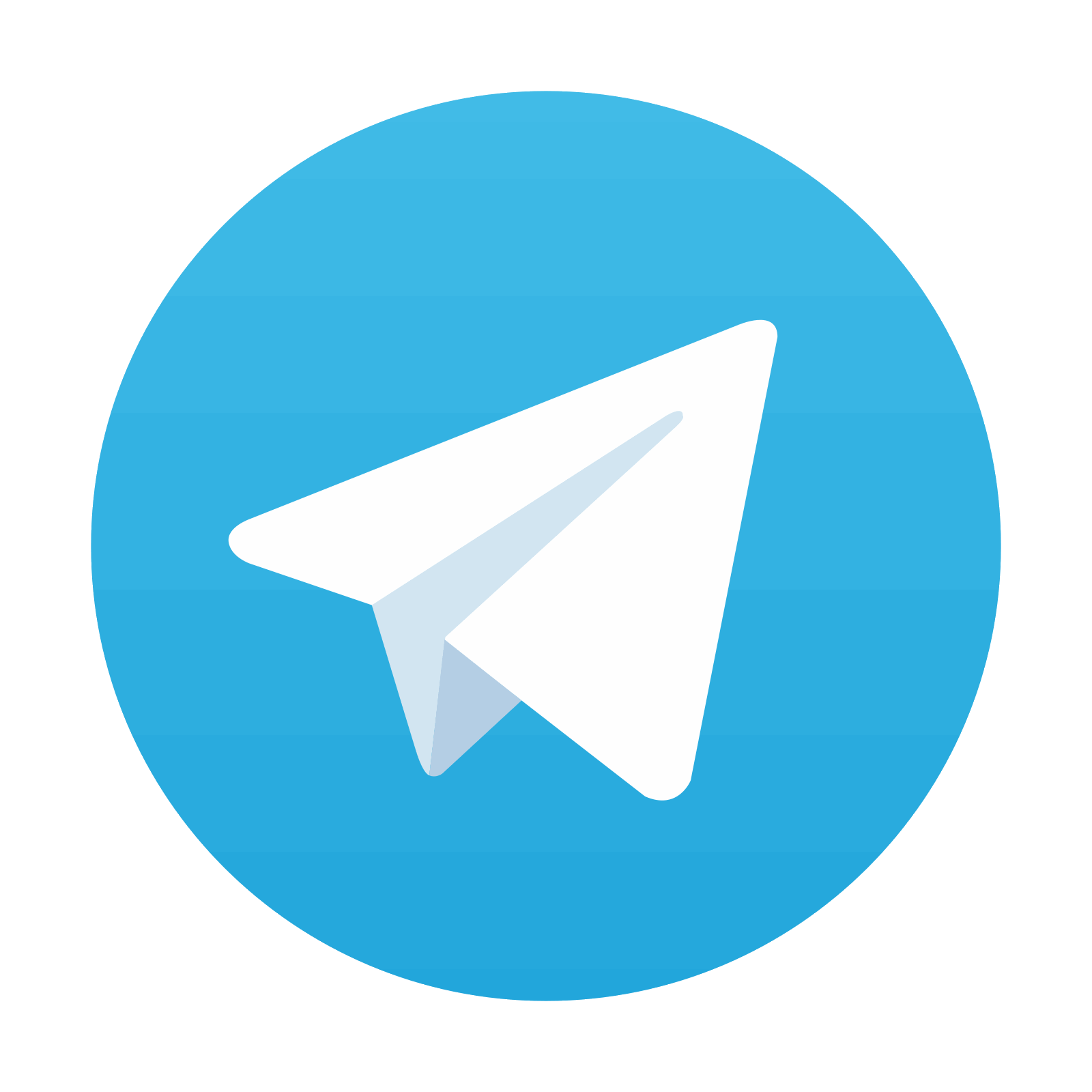
Stay updated, free articles. Join our Telegram channel

Full access? Get Clinical Tree
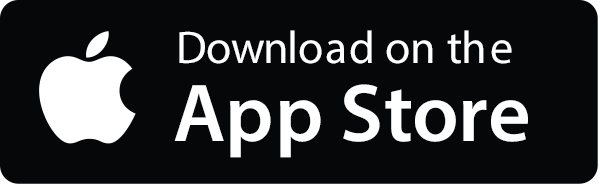
